没有合适的资源?快使用搜索试试~ 我知道了~
Operations Research Applications and Algorithms

温馨提示
Operations Research Applications and Algorithms 4E
资源推荐
资源详情
资源评论
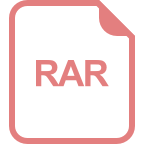
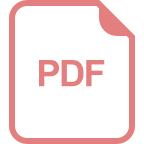
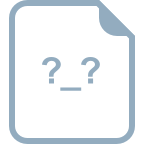
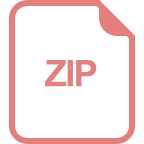
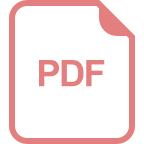
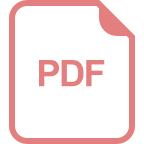
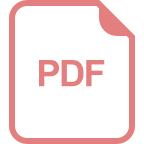
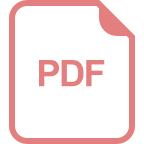
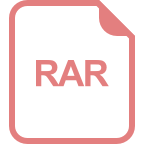
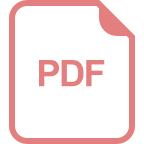
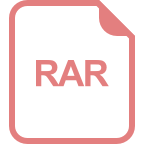
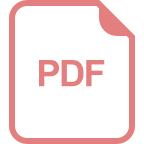
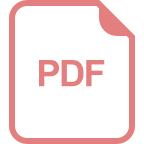
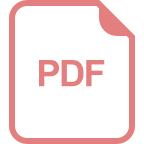
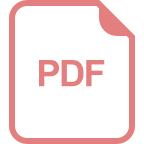
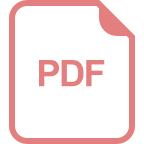
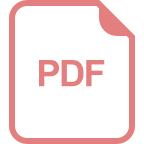
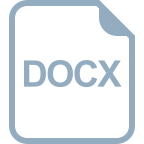
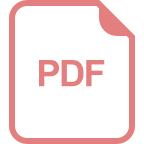
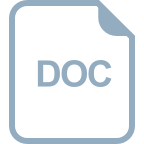
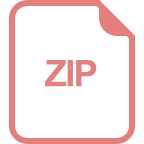
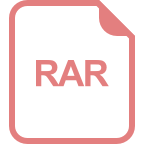
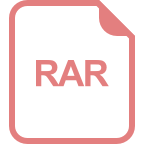
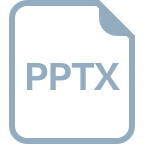
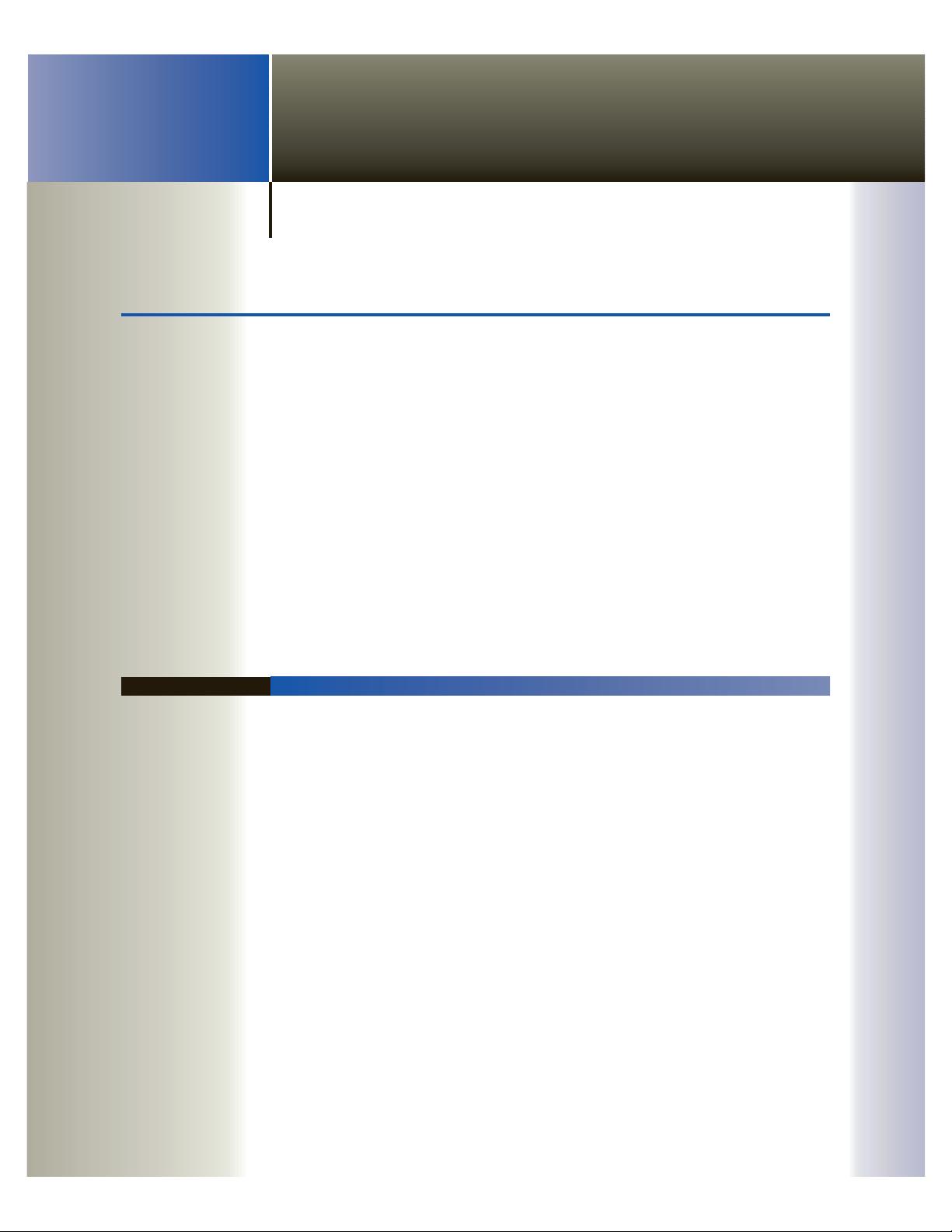
An Introduction to Model Building
1.1 An Introduction to Modeling
Operations research (often referred to as management science) is simply a scientific
approach to decision making that seeks to best design and operate a system, usually un-
der conditions requiring the allocation of scarce resources.
By a system, we mean an organization of interdependent components that work together
to accomplish the goal of the system. For example, Ford Motor Company is a system whose
goal consists of maximizing the profit that can be earned by producing quality vehicles.
The term operations research was coined during World War II when British military
leaders asked scientists and engineers to analyze several military problems such as the de-
ployment of radar and the management of convoy, bombing, antisubmarine, and mining
operations.
The scientific approach to decision making usually involves the use of one or more
mathematical models. A mathematical model is a mathematical representation of an ac-
tual situation that may be used to make better decisions or simply to understand the ac-
tual situation better. The following example should clarify many of the key terms used to
describe mathematical models.
Eli Daisy produces Wozac in huge batches by heating a chemical mixture in a pressur-
ized container. Each time a batch is processed, a different amount of Wozac is produced.
The amount produced is the process yield (measured in pounds). Daisy is interested in
understanding the factors that influence the yield of the Wozac production process. De-
scribe a model-building process for this situation.
Solution Daisy is first interested in determining the factors that influence the yield of the process.
This would be referred to as a descriptive model, because it describes the behavior of the
actual yield as a function of various factors. Daisy might determine (using regression
methods discussed in Chapter 24) that the following factors influence yield:
■ container volume in liters (V)
■ container pressure in milliliters (P)
■ container temperature in degrees Celsius (T)
■ chemical composition of the processed mixture
If we let A, B, and C be percentage of mixture made up of chemicals A, B, and C, then
Daisy might find, for example, that
(1) yield 300 .8V .01P .06T .001T*P .01T
2
.001P
2
11.7A 9.4B 16.4C 19A*B 11.4A*C 9.6B*C
Maximizing Wozac YieldEXAMPLE 1
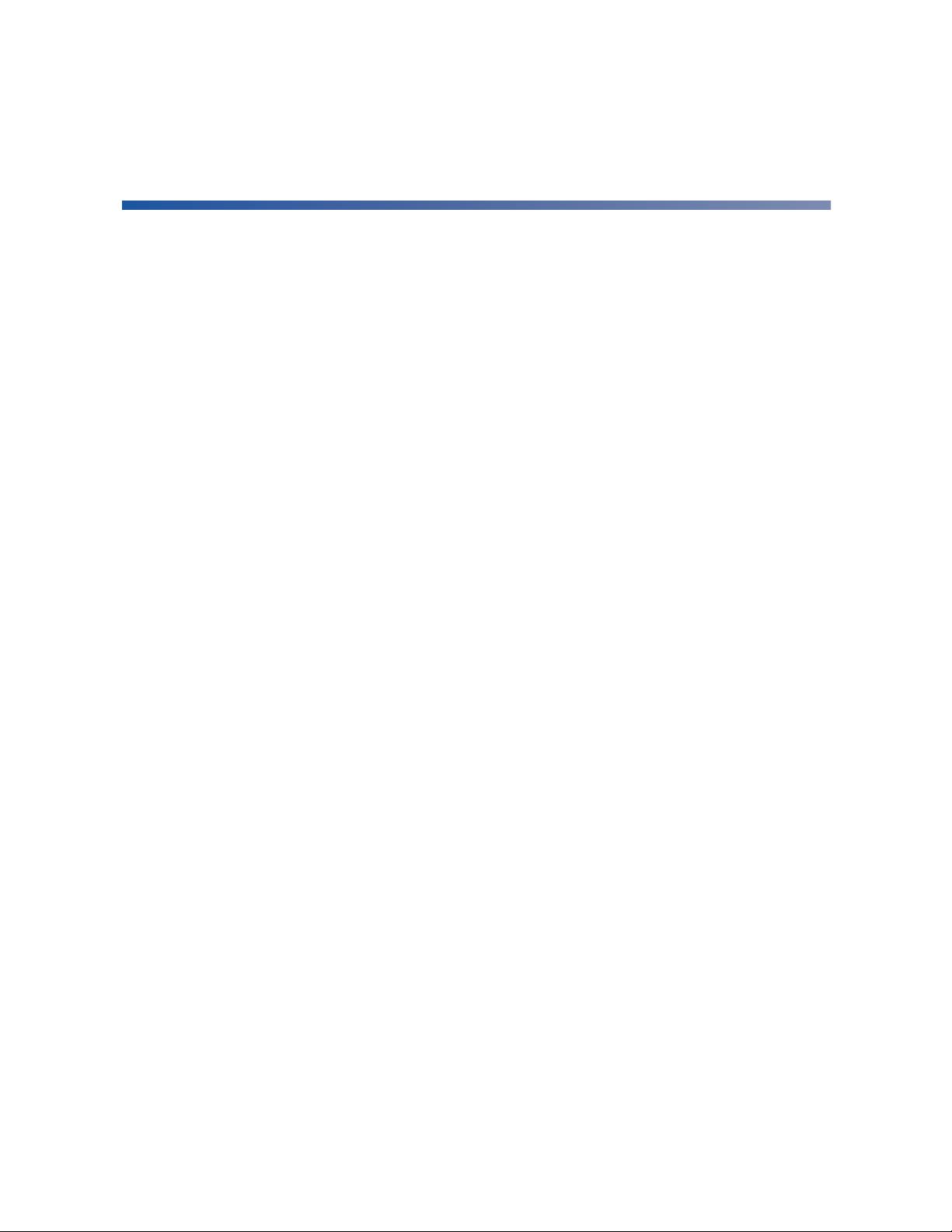
To determine this relationship, the yield of the process would have to be measured for
many different combinations of the previously listed factors. Knowledge of this equation
would enable Daisy to describe the yield of the production process once volume, pres-
sure, temperature, and chemical composition were known.
Prescriptive or Optimization Models
Most of the models discussed in this book will be prescriptive or optimization models.
A prescriptive model “prescribes” behavior for an organization that will enable it to best
meet its goal(s). The components of a prescriptive model include
■ objective function(s)
■ decision variables
■ constraints
In short, an optimization model seeks to find values of the decision variables that opti-
mize (maximize or minimize) an objective function among the set of all values for the
decision variables that satisfy the given constraints.
The Objective Function
Naturally, Daisy would like to maximize the yield of the process. In most models, there
will be a function we wish to maximize or minimize. This function is called the model’s
objective function. Of course, to maximize the process yield we need to find the values
of V, P, T, A, B, and C that make (1) as large as possible.
In many situations, an organization may have more than one objective. For example, in
assigning students to the two high schools in Bloomington, Indiana, the Monroe County
School Board stated that the assignment of students involved the following objectives:
■ Equalize the number of students at the two high schools.
■ Minimize the average distance students travel to school.
■ Have a diverse student body at both high schools.
Multiple objective decision-making problems are discussed in Sections 4.14 and 11.13.
The Decision Variables
The variables whose values are under our control and influence the performance of the
system are called decision variables. In our example, V, P, T, A, B, and C are decision
variables. Most of this book will be devoted to a discussion of how to determine the value
of decision variables that maximize (sometimes minimize) an objective function.
Constraints
In most situations, only certain values of decision variables are possible. For example, cer-
tain volume, pressure, and temperature combinations might be unsafe. Also, A B, and C
must be nonnegative numbers that add to 1. Restrictions on the values of decision vari-
ables are called constraints. Suppose the following:
2
CHAPTER 1 An Introduction to Model Building
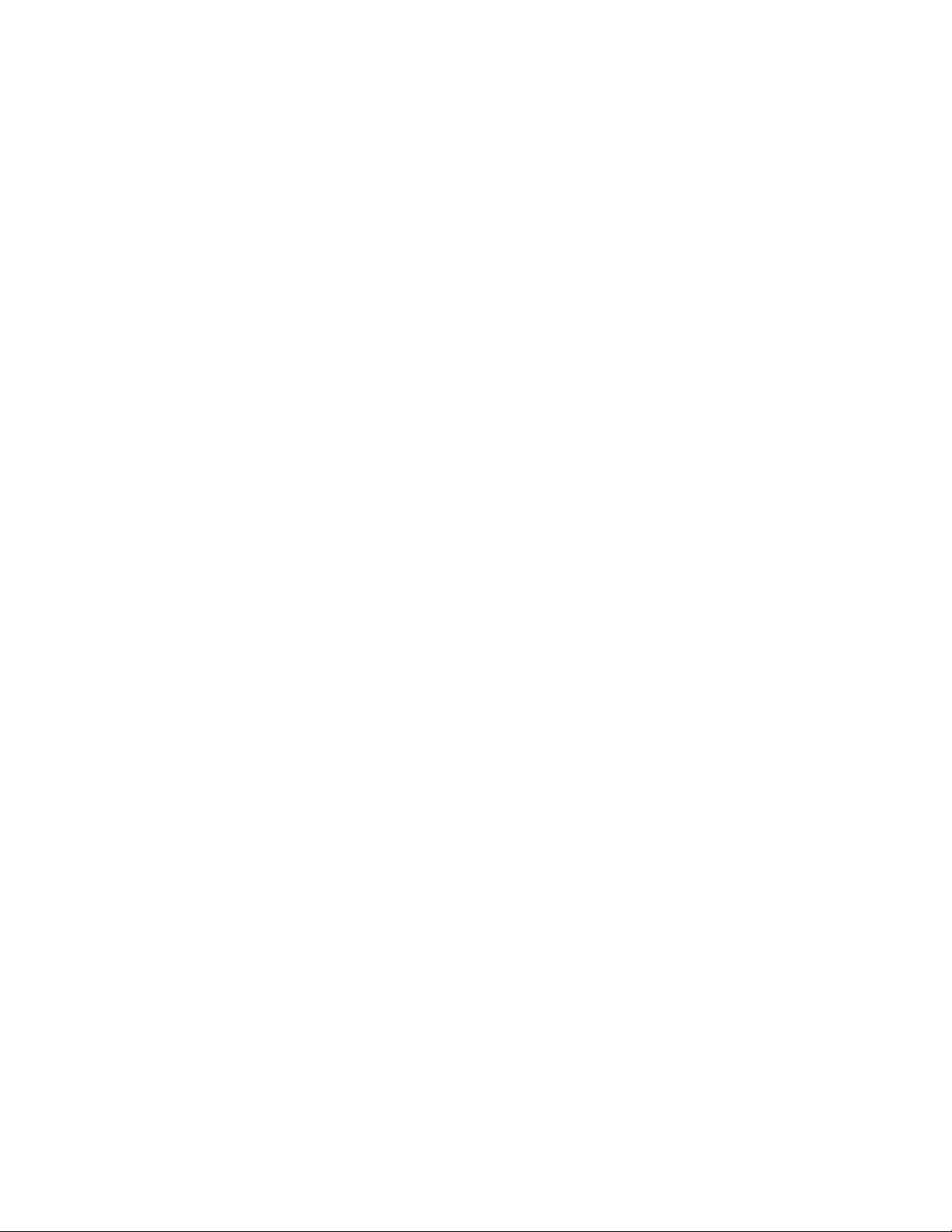
■ Volume must be between 1 and 5 liters.
■ Pressure must be between 200 and 400 milliliters.
■ Temperature must be between 100 and 200 degrees Celsius.
■ Mixture must be made up entirely of A, B, and C.
■ For the drug to properly perform, only half the mixture at most can be product A.
These constraints can be expressed mathematically by the following constraints:
V 5
V 1
P 400
P 200
T 200
T 100
A 0
B 0
A B C 1
A 5
The Complete Optimization Model
After letting z represent the value of the objective function, our entire optimization model
may be written as follows:
Maximize z 300 .8V .01P .06T .001T*P .01T
2
.001P
2
11.7A 9.4B 16.4C 19A*B 11.4A*C 9.6B*C
Subject to (s.t.)
V 5
V 1
P 400
P 200
T 200
T 100
A 0
B 0
C 0
A B C 1
A 5
Any specification of the decision variables that satisfies all of the model’s constraints is
said to be in the feasible region. For example, V 2, P 300, T 150, A .4, B
.3, and C .1 is in the feasible region. An optimal solution to an optimization model is
any point in the feasible region that optimizes (in this case, maximizes) the objective func-
tion. Using the LINGO package that comes with this book, it can be determined that the
optimal solution to this model is V 5, P 200, T 100, A .294, B 0, C .706,
and z 183.38. Thus, a maximum yield of 183.38 pounds can be obtained with a 5-liter
1.1 An Introduction to Modeling 3
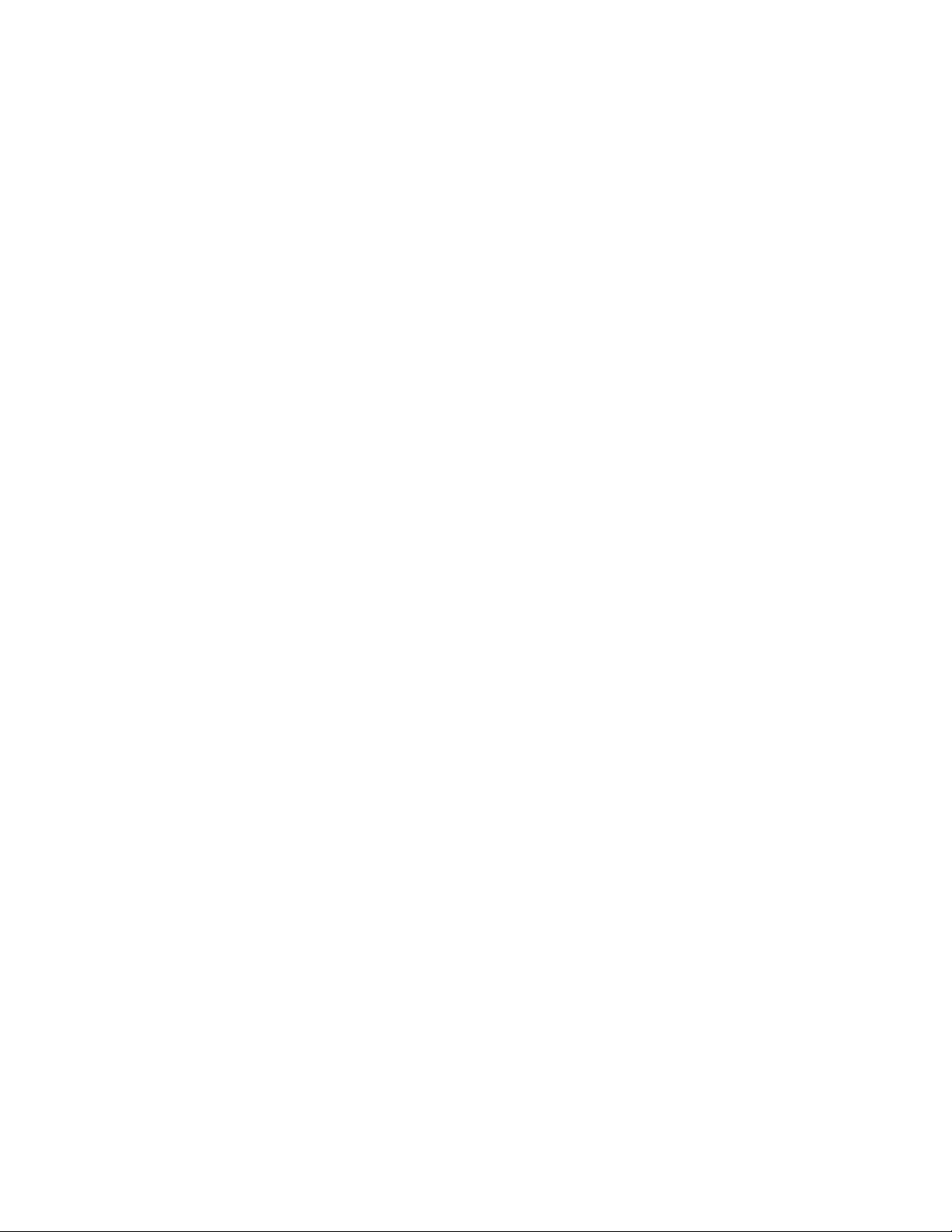
container, pressure of 200 milliliters, temperature of 100 degrees Celsius, and 29% A and
71% C. This means no other feasible combination of decision variables can obtain a yield
exceeding 183.38 pounds.
Static and Dynamic Models
A static model is one in which the decision variables do not involve sequences of deci-
sions over multiple periods. A dynamic model is a model in which the decision variables
do involve sequences of decisions over multiple periods. Basically, in a static model we
solve a “one-shot” problem whose solutions prescribe optimal values of decision variables
at all points in time. Example 1 is an example of a static model; the optimal solution will
tell Daisy how to maximize yield at all points in time.
For an example of a dynamic model, consider a company (call it Sailco) that must de-
termine how to minimize the cost of meeting (on time) the demand for sailboats during
the next year. Clearly Sailco’s must determine how many sailboats it will produce during
each of the next four quarters. Sailco’s decisions involve decisions made over multiple pe-
riods, hence a model of Sailco’s problem (see Section 3.10) would be a dynamic model.
Linear and Nonlinear Models
Suppose that whenever decision variables appear in the objective function and in the con-
straints of an optimization model, the decision variables are always multiplied by constants
and added together. Such a model is a linear model. If an optimization model is not lin-
ear, then it is a nonlinear model. In the constraints of Example 1, the decision variables
are always multiplied by constants and added together. Thus, Example 1’s constraints pass
the test for a linear model. However, in the objective function for Example 1, the terms
.001T*P, .01T
2
, 19A*B, 11.4A*C, and 9.6B*C make the model nonlinear. In general,
nonlinear models are much harder to solve than linear models. We will discuss linear
models in Chapters 2 through 10. Nonlinear models will be discussed in Chapter 11.
Integer and Noninteger Models
If one or more decision variables must be integer, then we say that an optimization model
is an integer model. If all the decision variables are free to assume fractional values, then
the optimization model is a noninteger model. Clearly, volume, temperature, pressure,
and percentage composition of our inputs may all assume fractional values. Thus, Exam-
ple 1 is a noninteger model. If the decision variables in a model represent the number of
workers starting work during each shift at a fast-food restaurant, then clearly we have an
integer model. Integer models are much harder to solve than nonlinear models. They will
be discussed in detail in Chapter 9.
Deterministic and Stochastic Models
Suppose that for any value of the decision variables, the value of the objective function
and whether or not the constraints are satisfied is known with certainty. We then have a
deterministic model. If this is not the case, then we have a stochastic model. All mod-
els in the first 12 chapters will be deterministic models. Stochastic models are covered in
Chapters 13, 16, 17, and 19–24.
4
CHAPTER 1 An Introduction to Model Building
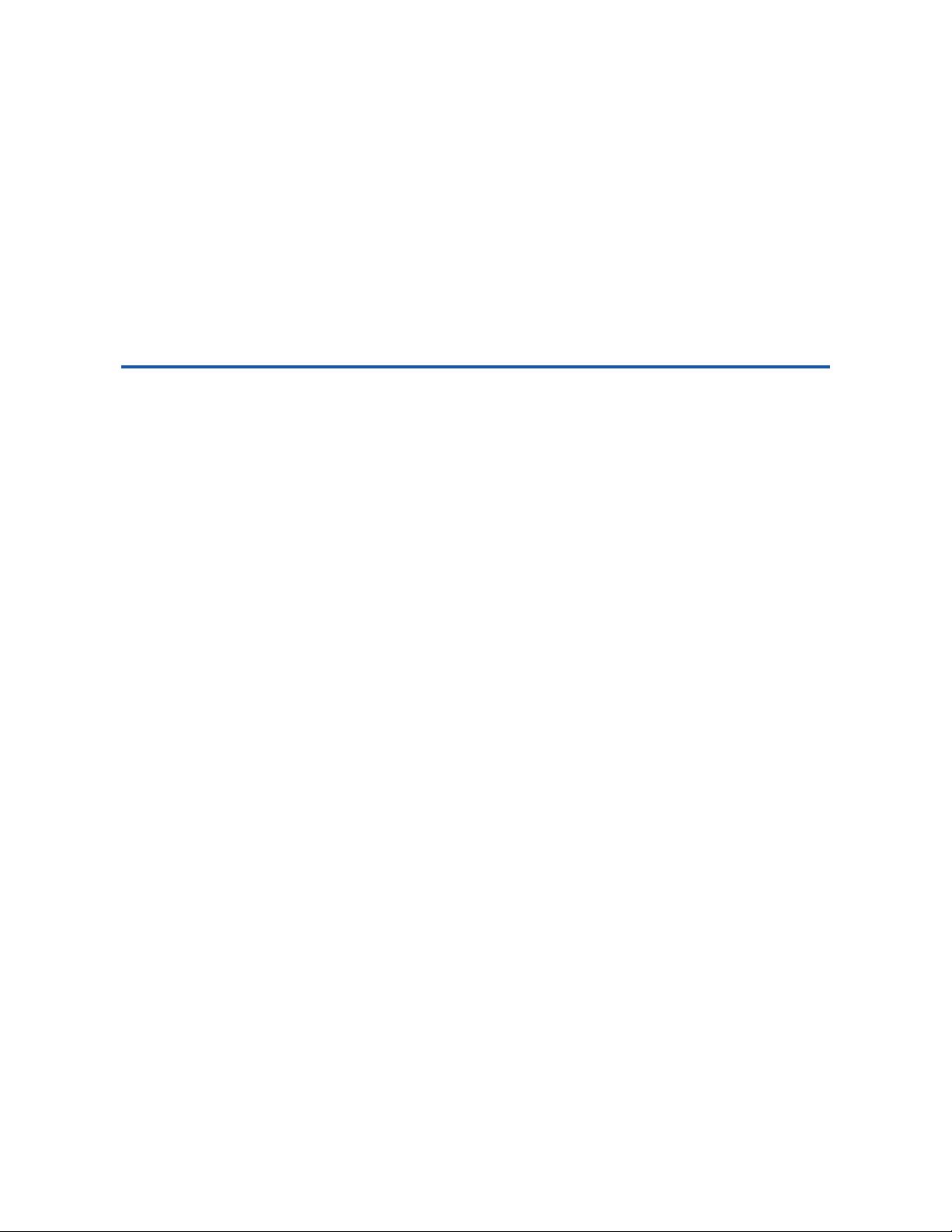
If we view Example 1 as a deterministic model, then we are making the (unrealistic)
assumption that for given values of V, P, T, A, B, and C, the process yield will always be
the same. This is highly unlikely. We can view (1) as a representation of the average yield
of the process for given values of the decision variables. Then our objective is to find val-
ues of the decision variables that maximize the average yield of the process.
We can often gain useful insights into optimal decisions by using a deterministic model
in a situation where a stochastic model is more appropriate. Consider Sailco’s problem of
minimizing the cost of meeting the demand (on time) for sailboats. The uncertainty about
future demand for sailboats implies that for a given production schedule, we do not know
whether demand is met on time. This leads us to believe that a stochastic model is needed
to model Sailco’s situation. We will see in Section 3.10, however, that we can develop a
deterministic model for this situation that yields good decisions for Sailco.
1.2 The Seven-Step Model-Building Process
When operations research is used to solve an organization’s problem, the following seven-
step model-building procedure should be followed:
Step 1: Formulate the Problem The operations researcher first defines the organization’s
problem. Defining the problem includes specifying the organization’s objectives and the
parts of the organization that must be studied before the problem can be solved. In Ex-
ample 1, the problem was to determine how to maximize the yield from a batch of Wozac.
Step 2: Observe the System Next, the operations researcher collects data to estimate the
value of parameters that affect the organization’s problem. These estimates are used to de-
velop (in step 3) and evaluate (in step 4) a mathematical model of the organization’s prob-
lem. For example, in Example 1, data would be collected in an attempt to determine how
the values of T, P, V, A, B, and C influence process yield.
Step 3: Formulate a Mathematical Model of the Problem In this step, the operations re-
searcher develops a mathematical model of the problem. In this book, we will describe
many mathematical techniques that can be used to model systems. For Example 1, our
optimization model would be the result of step 3.
Step 4: Verify the Model and Use the Model for Prediction The operations researcher now
tries to determine if the mathematical model developed in step 3 is an accurate represen-
tation of reality. For example, to validate our model, we might check and see if (1) accu-
rately represents yield for values of the decision variables that were not used to estimate
(1). Even if a model is valid for the current situation, we must be aware of blindly ap-
plying it. For example, if the government placed new restrictions on Wozac, then we might
have to add new constraints to our model, and the yield of the process [and Equation (1)]
might change.
Step 5: Select a Suitable Alternative Given a model and a set of alternatives, the operations
researcher now chooses the alternative that best meets the organization’s objectives.
(There may be more than one!) For instance, our model enabled us to determine that yield
was maximized with V 5, P 200, T 100, A .294, B 0, C .706, and z
183.38.
Step 6: Present the Results and Conclusion of the Study to the Organization In this step, the
operations researcher presents the model and recommendation from step 5 to the decision-
making individual or group. In some situations, one might present several alternatives and
let the organization choose the one that best meets its needs. After presenting the results
1.2 The Seven-Step Model-Building Process 5
剩余1347页未读,继续阅读

shadow1096
- 粉丝: 1
- 资源: 4
上传资源 快速赚钱
我的内容管理 展开
我的资源 快来上传第一个资源
我的收益
登录查看自己的收益我的积分 登录查看自己的积分
我的C币 登录后查看C币余额
我的收藏
我的下载
下载帮助

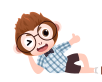
最新资源
资源上传下载、课程学习等过程中有任何疑问或建议,欢迎提出宝贵意见哦~我们会及时处理!
点击此处反馈


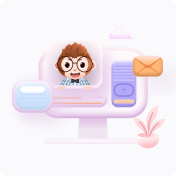
安全验证
文档复制为VIP权益,开通VIP直接复制

- 1
- 2
前往页