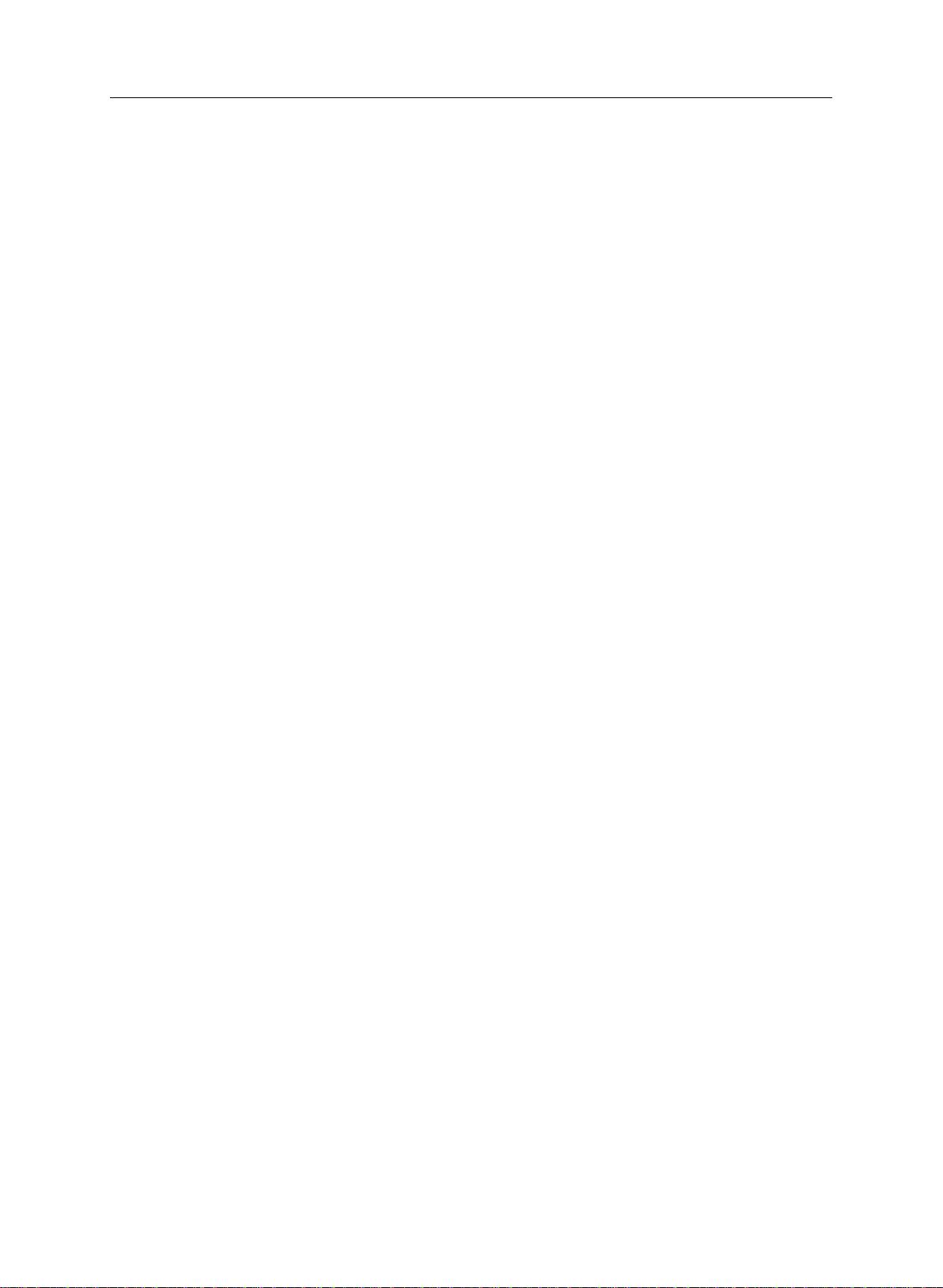
Abstract
MULTIPLE TESTING TECHNIQUE AND ITS APPLICATION IN
THE ANALYSIS OF MICROARRAY DATA
ABSTRACT
Multiple testing is an important method of data statistics analysis. It has a lot of
applications in bioinformatics, genomics and other aspects. Two kinds of problem are
considered in this paper. One is the control of false discovery rate control and the other is the
ratio of true null hypothesis. Finally they are used for the screening of differently expressed
genes in microarray data.
This paper first introduces the theoretical basis of multiple testing, and points out that the
most important is to control the type I error in this area. This can be solved by controlling
FWER and FDR. The old approach to multiplicity problem calls for controlling the family
wise error rate (FWER), but it is thought to be too strict. The FDR proposed by
Benjanimi&Hochberg(1995) ease strict rule of FWER, and it has more advantages in
distinguishing significant difference between two samples. Four classes of algorithms for
controlling FDR are listed, while the Bonferroni procedure are set as the reference of others.
In the control of false discovery rate, simulated data were used. In the algorithm, we need to
optimize the original p value, and compare the efficiency of each method under the new p
value set. Simulation results show that the q-value method can maintain the highest power
while controlling FDR.
How to correctly and effectively estimate the ratio of true null hypothesis m
0
is another
emphases of our work. Several estimation methods are reviewed and an improved average
method is proposed based on Jiang&Doerge(2008). The cubic spline method is used to
estimate the interval instead of bootstrap. Meanwhile the slope method is Li Wei(2014) is also
compared. In the simulation, we found that the improved mean value method can estimate m
0
.
We apply them in the data of breast cancer in Hendenfalk(2001) and the data of B cell in Feng
Pan,Tie-Lin Yang.etl(2009). Methods above are used to screen greens in microarray data.
Compared with the methods in Hochberg&Benjamini(2000), Storey&Tibshirani(2002)and the
convest decreasing density estimate in Langaas,M.et al.(2005), the improved average method
is able to find more genes, or to find the total gene fewer in number when finding the same
effective difference genes. Our improved average method is comparable to the method in Li
Wei considering the efficacy of the algorithm. Number of distinct gene is the same. This
proved the validity of the new average method in the estimation of true null hypothesis.
Key Words
multiple testing, FDR, p value adjustment, the ratio of true null hypothesis,
microarray
II
万方数据