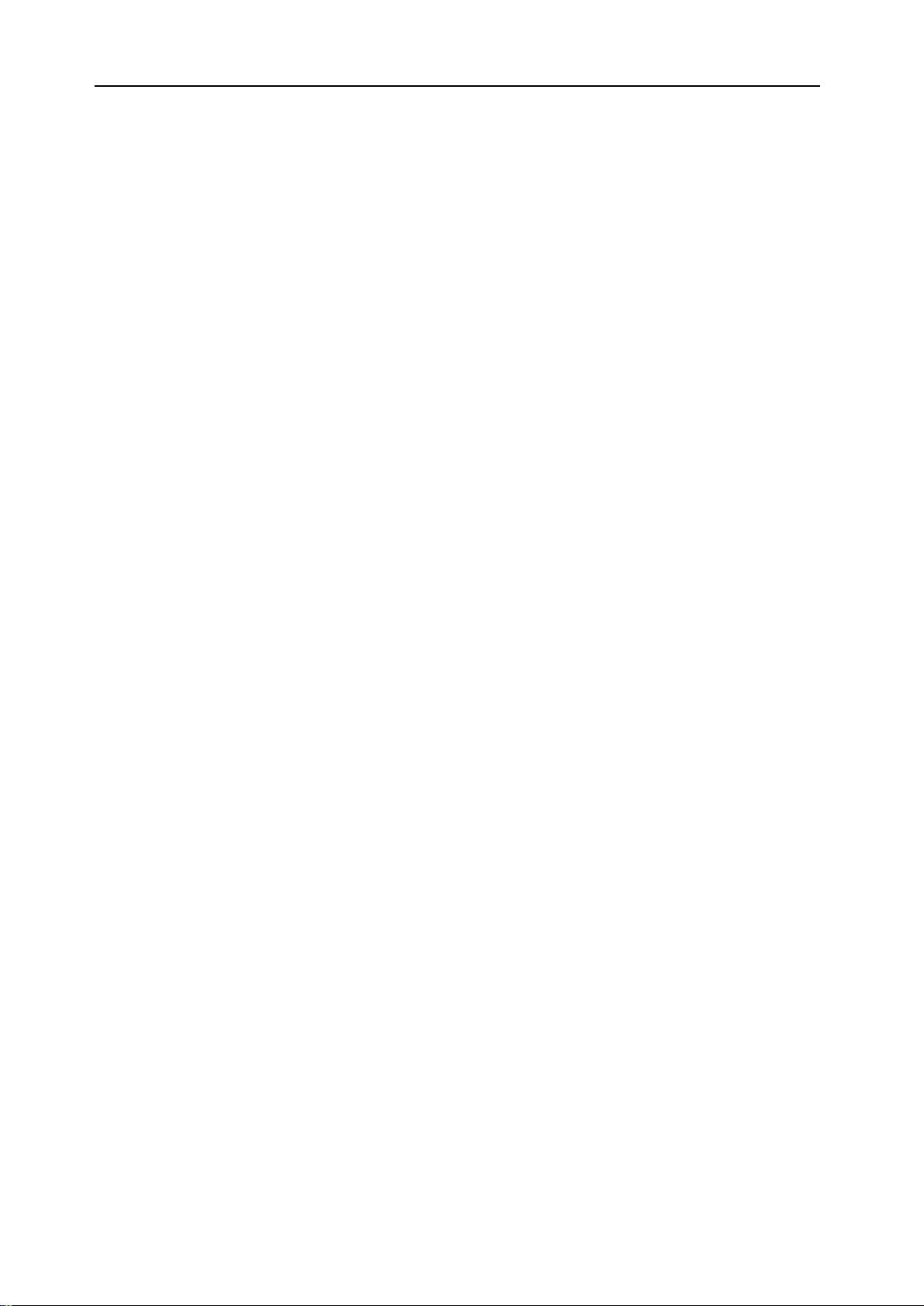
贵州大学硕士学位论文 图像前背景分离的机器学习算法及应用
II
Machine learning algorithms and applications of Image
foreground and background separation
Abstract
Images are the main carriers of information. With the development of computer technology,
using computers to collect, process and identify information contained in image has become a
popular research field. Image fore-background separation is a very important part of target
detection, tracking and recognition. As the name suggests, the fore-background separation is
used to separate interest part in image from background. The requirements for separation results
are different in various application scenarios. For example, when making expression packages
by using image editing tools such as a Tian Tian Pi Tu, MIX. We need accurate separation.
However. we need rough separation in driverless cars and image retrieval. Therefore, there are
many kinds of separation algorithms and their processing results are also different.
Since last century, scholars have proposed Knock, Bayesian, Grabcut and other algorithms.
They are uesd to segment image fore-background. Among them, Grabcut is one of the most
widely used and classic algorithms. But it requires human-computer interaction to process image,
the algorithm is less efficient and automated. In order to solve this problem, We improve the
traditional Grabcut algorithm and make it automotive. The main research work of this
dissertation is as follows:
Firstly, on the basis of introducing the principle of common machine learning algorithms
and analyzing their advantages and disadvantages, the principle of Grabcut algorithm is
introduced and experiments are conducted. Then analyzing and summarizing the experimental
results are provided.
Secondly, we propose an automatic image fore-background separation algorithm based on
Lab color space’s texture features. The image is divided and converted from RGB to Lab color
space, then extracting color and texture features of each image sub-blocks. After selecting seeds
for regional growth, in order to improve the over-segmentation caused by regional growth, we
use region merging to merge image blocks that are belong to the same region. Compared with
the traditional method, the proposed algorithm does not require manual interaction and sample
for training. The experimental results show that the proposed algorithm is not satisfied very
万方数据
评论0
最新资源