人工智能-数据挖掘-基于数据挖掘的企业财务风险预警研究.pdf
2.虚拟产品一经售出概不退款(资源遇到问题,请及时私信上传者)
"人工智能-数据挖掘-基于数据挖掘的企业财务风险预警研究" 本论文的主要内容是应用数据挖掘技术对企业财务风险进行预警,研究如何通过数据挖掘技术来解决企业财务风险管理中的难题。 本论文对财务风险的分析和预警进行了研究,包括财务风险的原因和特征,以及财务风险预警方法。财务风险是企业面临的一种重要风险,包括流动性风险、信用风险、市场风险、操作风险等。财务风险的预警是企业风险管理的重要组成部分,能够帮助企业避免或减少财务风险的影响。 本论文提出了一种基于频繁项集的并行数据挖掘算法,旨在提高数据挖掘的性能和效率。该算法将(K-1)频繁项集分组,各组生成K频繁项集,并将其组合。这样可以减少自连接和裁剪操作,提高搜索速度和效率。实验结果表明,改进后的算法性能有了顯著的改善。 此外,本论文还探讨了数据挖掘技术在企业财务风险预警中的应用,包括数据预处理、数据转换、模式评估和规则发现等。数据挖掘技术可以帮助企业从大量数据中挖掘出有价值的信息, identification of potential risks and opportunities,实现企业财务风险的预警和防控。 本论文的主要贡献在于提出了一种基于数据挖掘的企业财务风险预警方法,旨在帮助企业更好地管理财务风险,提高企业的财务稳定性和竞争力。 知识点: 1. 财务风险是企业面临的一种重要风险,包括流动性风险、信用风险、市场风险、操作风险等。 2. 财务风险预警是企业风险管理的重要组成部分,能够帮助企业避免或减少财务风险的影响。 3. 数据挖掘技术可以应用于企业财务风险预警,帮助企业从大量数据中挖掘出有价值的信息。 4. 基于频繁项集的并行数据挖掘算法可以提高数据挖掘的性能和效率。 5. 数据预处理、数据转换、模式评估和规则发现是数据挖掘技术在企业财务风险预警中的重要组成部分。 6. 企业财务风险预警需要通过数据挖掘技术来 identification of potential risks and opportunities,实现企业财务风险的预警和防控。 本论文对企业财务风险预警的研究具有重要的理论和实践意义,为企业财务风险管理提供了有价值的参考。
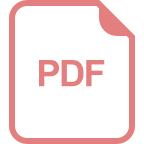
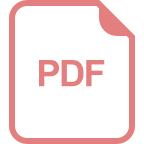
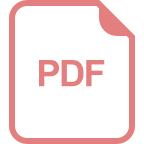
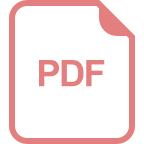
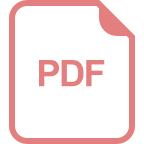
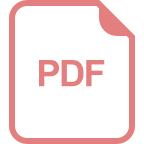
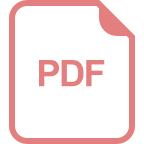
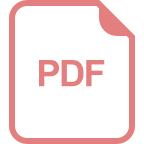
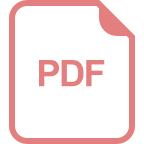
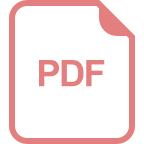
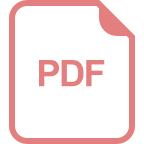
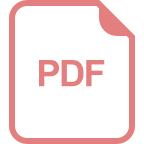
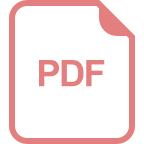
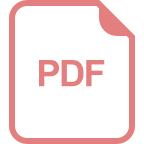
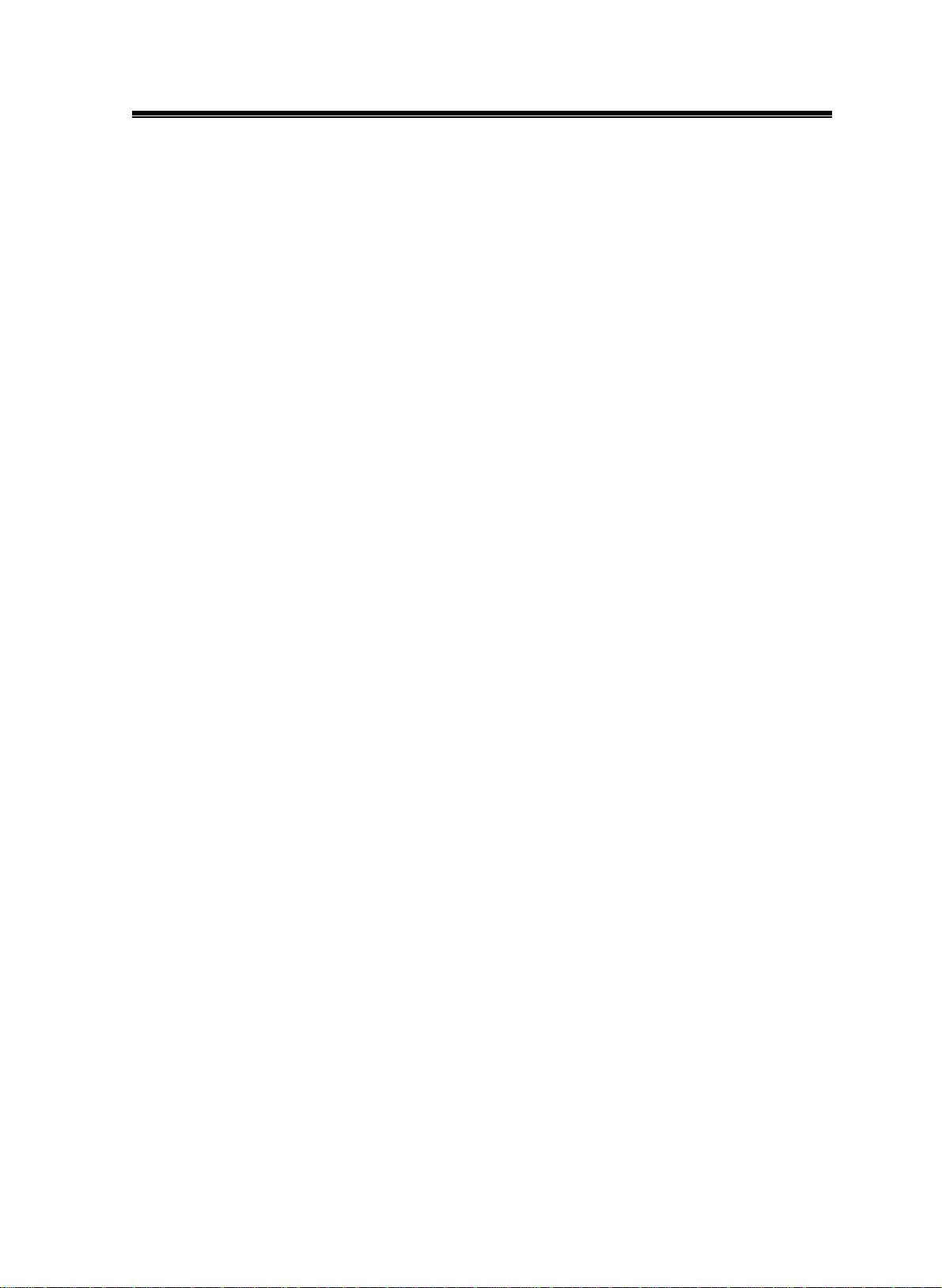
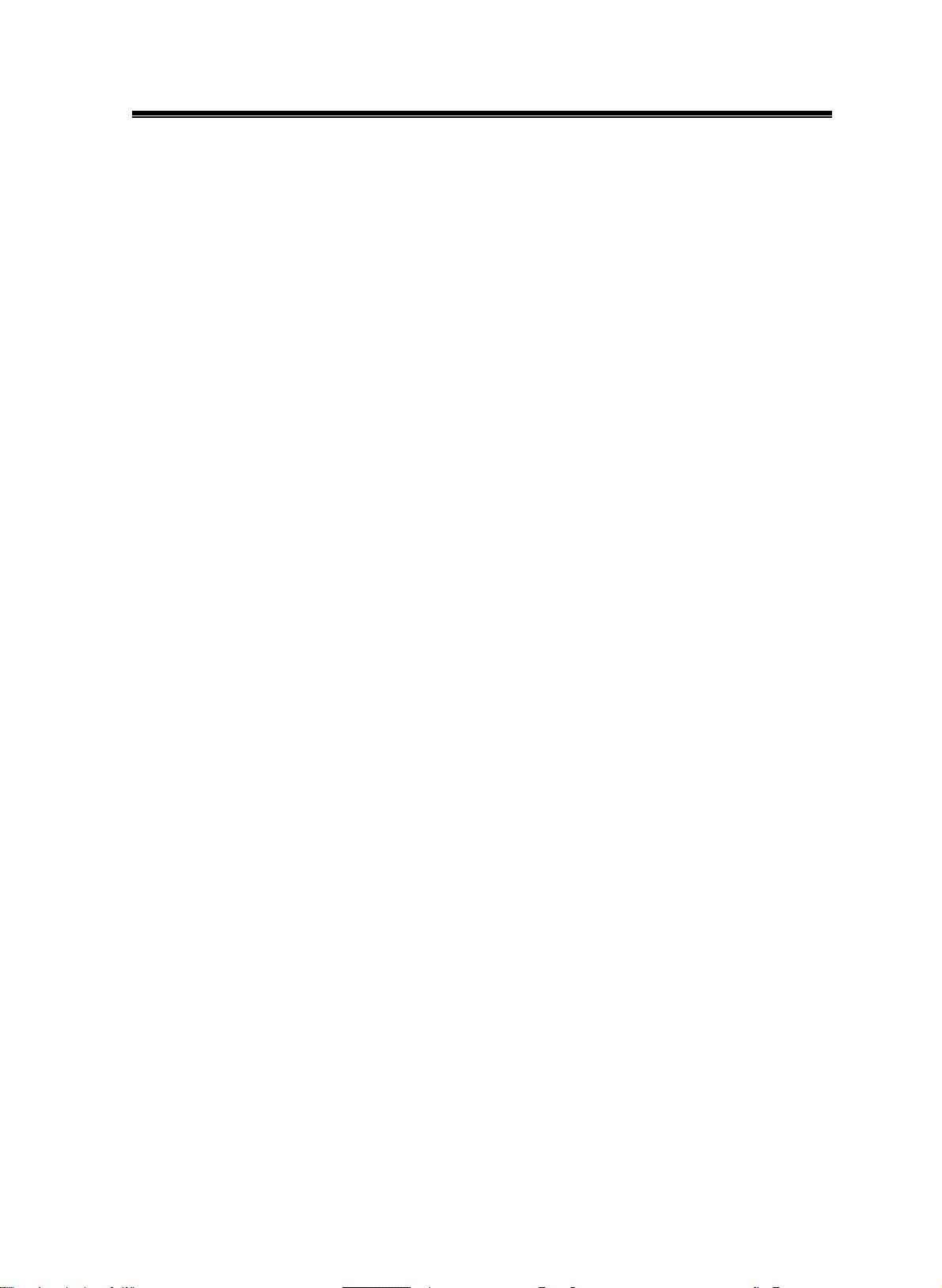
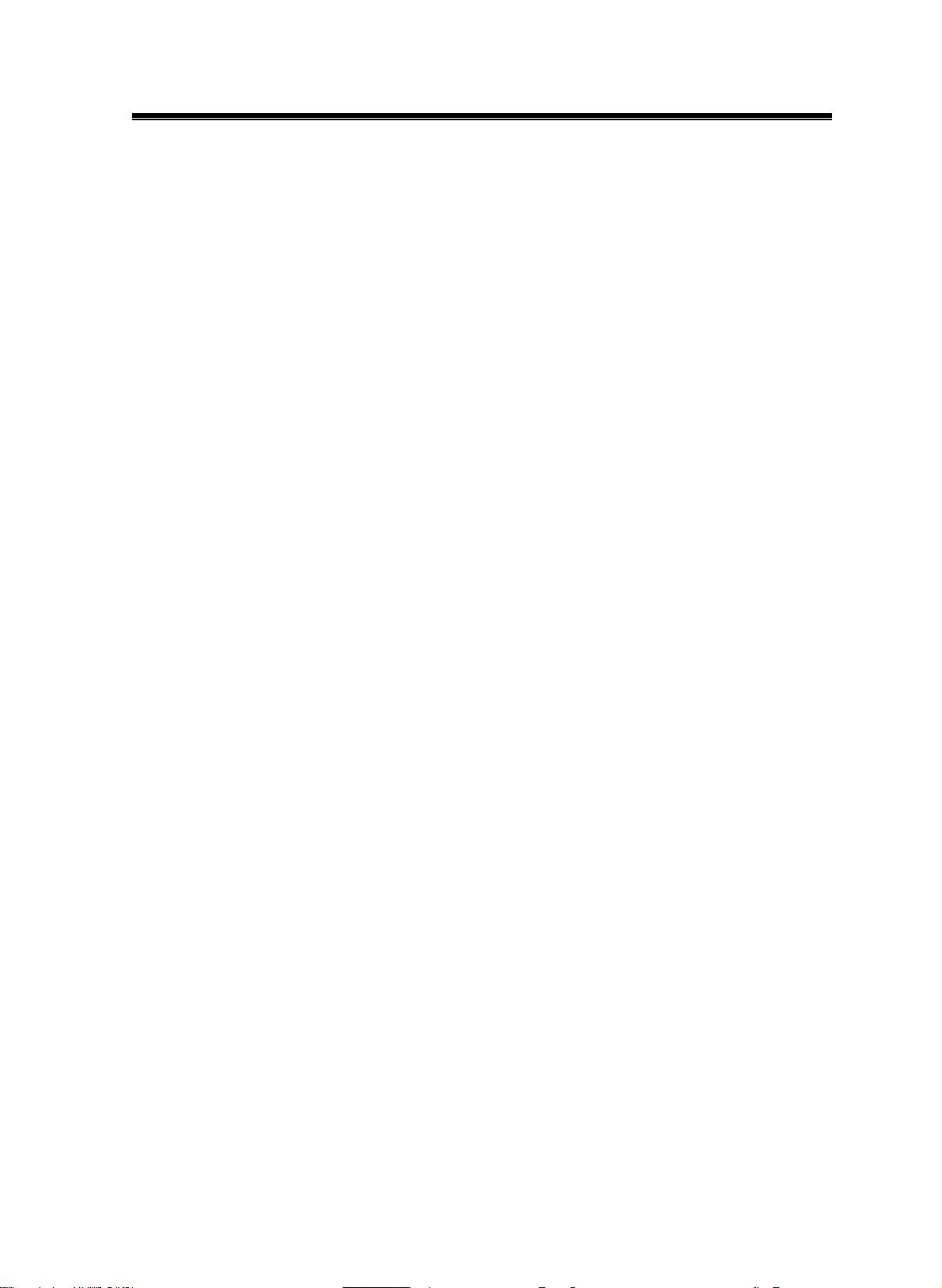
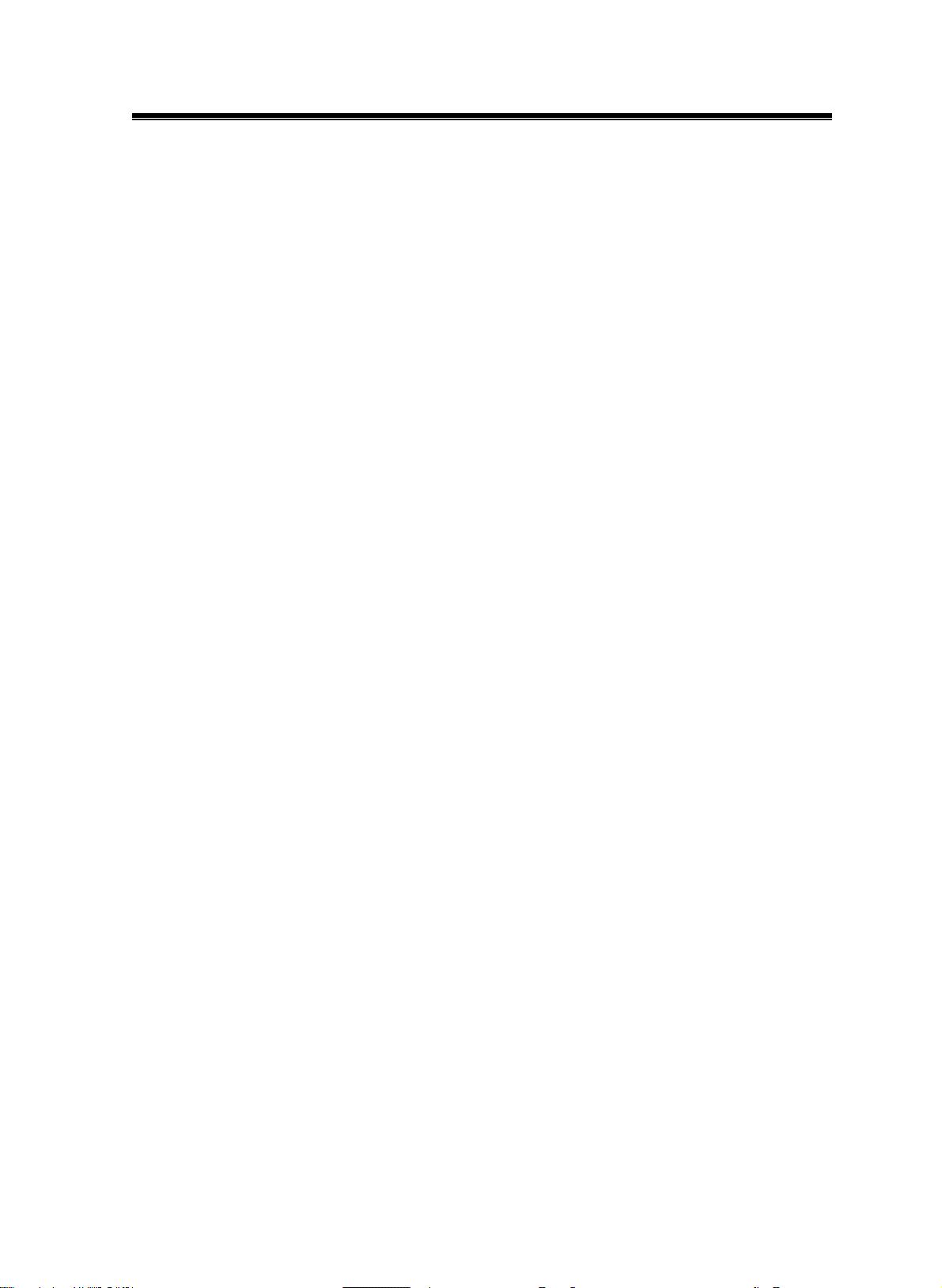
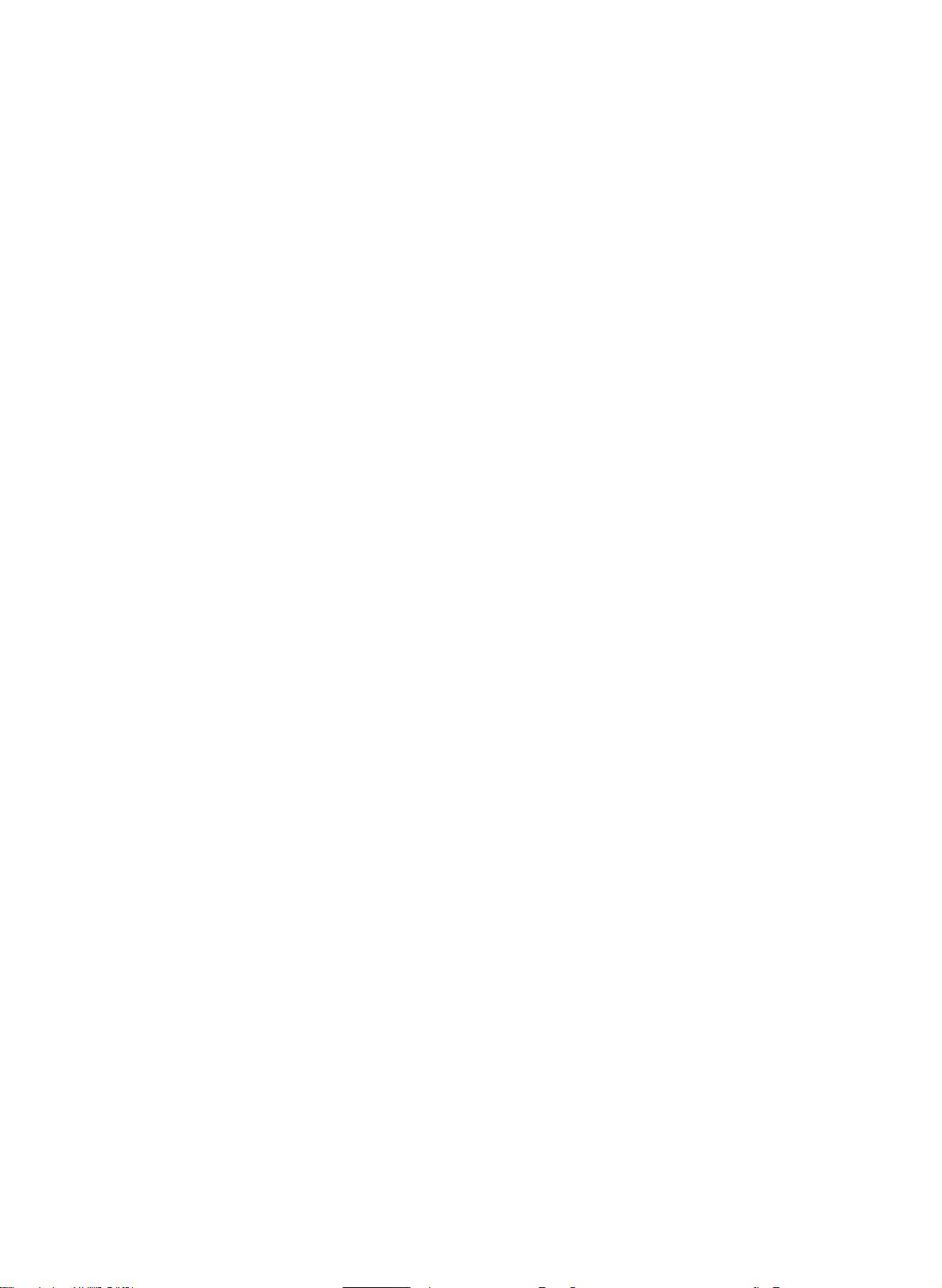
剩余81页未读,继续阅读
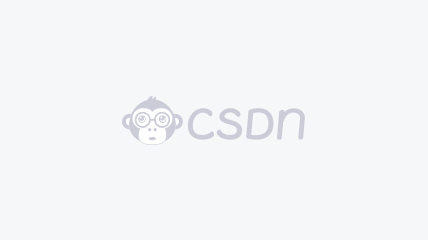

- 粉丝: 90
- 资源: 9323
我的内容管理 展开
我的资源 快来上传第一个资源
我的收益
登录查看自己的收益我的积分 登录查看自己的积分
我的C币 登录后查看C币余额
我的收藏
我的下载
下载帮助

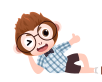
最新资源
- Python基于toad实现生成评分卡 完整的示例代码和数据集
- 基于PID控制器的电动汽车充放电系统的Simulink建模与仿真 包括程序操作录像+说明+参考paper 使用matlab2022a或者高版本,运行tops.m或者main.m 具体操作观看提供的程
- 密码学课程设计源代码,包括了数字签名、DES核心算法、Hash算法、RSA加解密
- stm32h743使用TSG时间
- 红绿灯识别项目代码,包括了一步一步的训练步骤,以及展示结果
- 基于视频通用内容特性的高效编码器参数优化模型研究(视频编码领域,HEVC标准,复杂度与性能优化)
- 基于显著性阈值的自适应视频流每场景比特率梯度优化预测方案
- 永磁同步电机旋转高频信号注入法零低速无位置控制仿真,相比高频方波信号注入法,旋转高频信号注入法噪声更小损耗更低,该模型注入1000Hz旋转高频电压信号到电机中用于产生激励电流,在低速100rpm下无感
- 异形插件机(sw21可编辑+工程图)全套技术资料100%好用.zip
- 光场图像编码新技术:基于线性近似先验的高效压缩方案
- 双足机器人强化学习项目.zip
- 双足机器人逆运动学解算.zip
- 双足机器人正运动学计算.zip
- RobotBit双足机器人.zip
- TITA双足机器人实机强化学习控制.zip
- 双足机器人:设计,建模,仿真,控制.zip

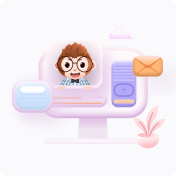
