没有合适的资源?快使用搜索试试~ 我知道了~
Introduction to Bayesian Statistics
需积分: 10 8 下载量 139 浏览量
2018-09-27
18:58:14
上传
评论
收藏 1.51MB PDF 举报
温馨提示
This course was originally developed by Dr Wayne Stewart (formerly of The University of Auckland) and was rst oered in 2009 (Figure 1.1). I joined the Department of Statistics in July 2012 and took over the course from him. It was good fortune for me that Wayne left the university as I arrived. If I had been able to choose which undergraduate course I would most like to teach, it would have been this one!
资源推荐
资源详情
资源评论












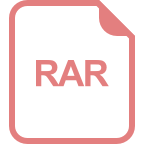



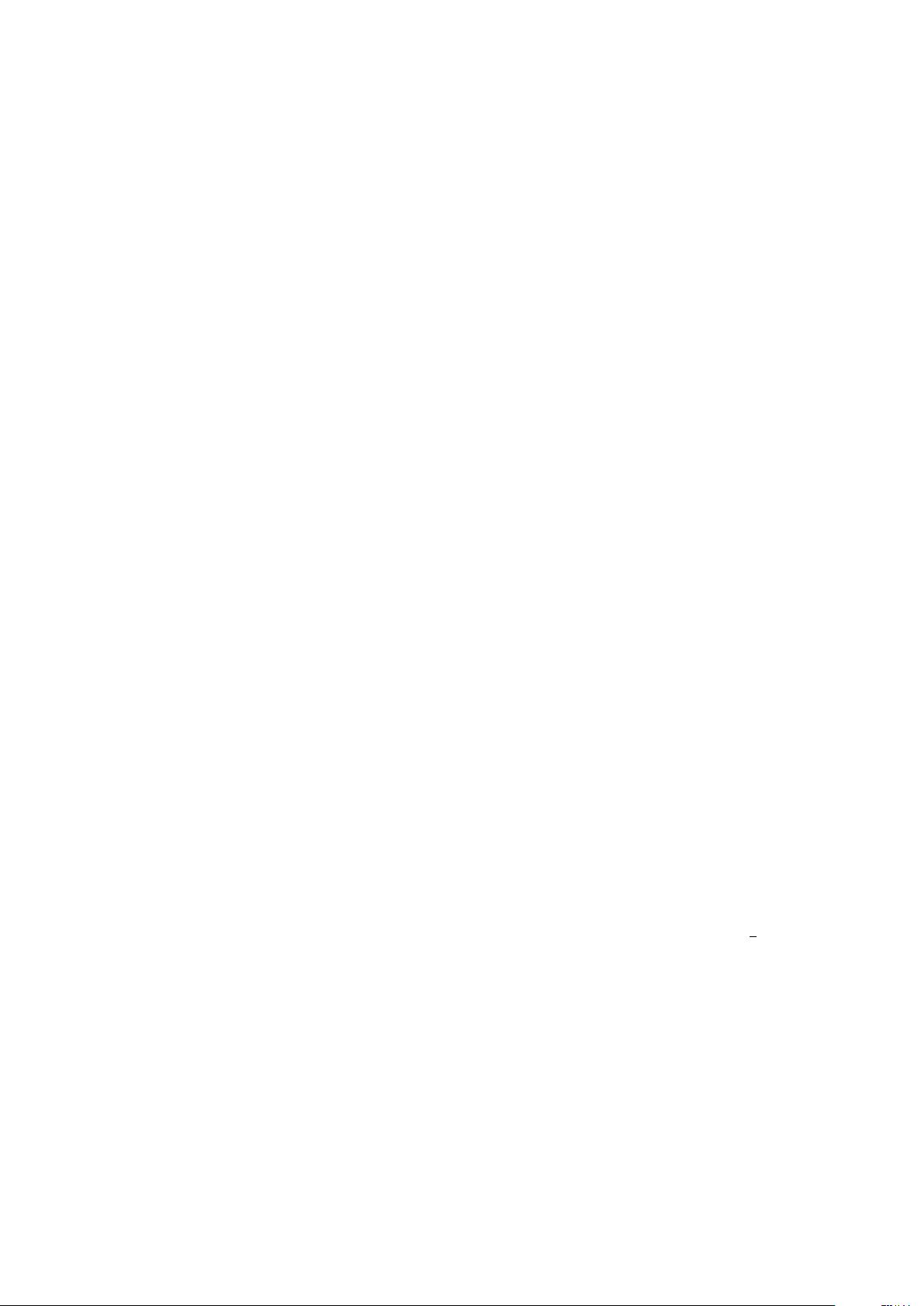
STATS 331
Introduction to Bayesian Statistics
Brendon J. Brewer
This work is licensed under the Creative Commons Attribution-ShareAlike
3.0 Unported License. To view a copy of this license, visit
http://creativecommons.org/licenses/by-sa/3.0/deed.en GB.
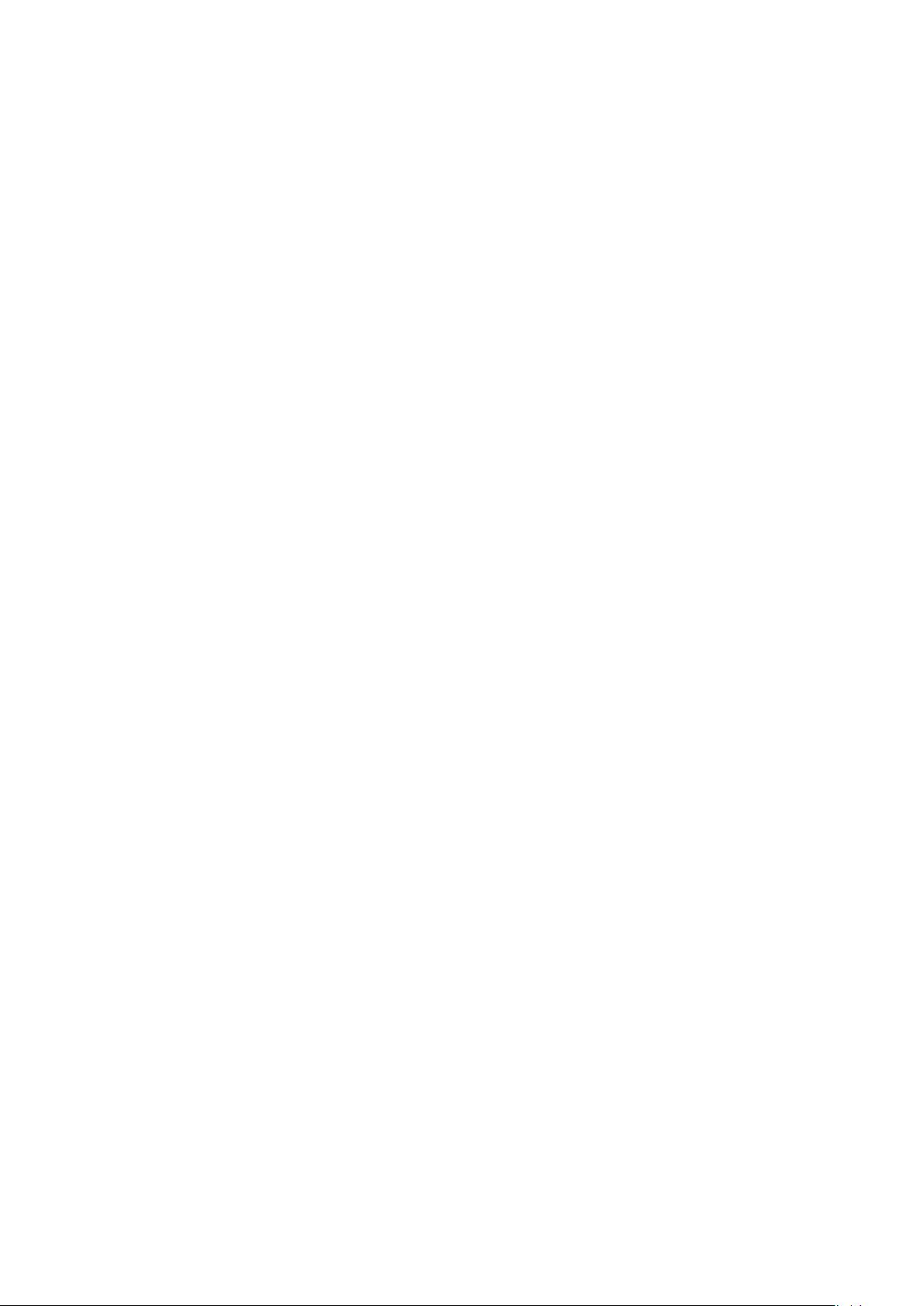
Contents
1 Prologue 4
1.1 Bayesian and Classical Statistics . . . . . . . . . . . . . . . . . . . . . . . . 5
1.2 This Version of the Notes . . . . . . . . . . . . . . . . . . . . . . . . . . . 6
1.3 Assessment . . . . . . . . . . . . . . . . . . . . . . . . . . . . . . . . . . . 6
2 Introduction 8
2.1 Certainty, Uncertainty and Probability . . . . . . . . . . . . . . . . . . . . 8
3 First Examples 11
3.1 The Bayes’ Box . . . . . . . . . . . . . . . . . . . . . . . . . . . . . . . . . 11
3.1.1 Likelihood . . . . . . . . . . . . . . . . . . . . . . . . . . . . . . . . 12
3.1.2 Finding the Likelihood Values . . . . . . . . . . . . . . . . . . . . . 13
3.1.3 The Mechanical Part . . . . . . . . . . . . . . . . . . . . . . . . . . 14
3.1.4 Interpretation . . . . . . . . . . . . . . . . . . . . . . . . . . . . . . 14
3.2 Bayes’ Rule . . . . . . . . . . . . . . . . . . . . . . . . . . . . . . . . . . . 15
3.3 Phone Example . . . . . . . . . . . . . . . . . . . . . . . . . . . . . . . . . 16
3.3.1 Solution . . . . . . . . . . . . . . . . . . . . . . . . . . . . . . . . . 17
3.4 Important Equations . . . . . . . . . . . . . . . . . . . . . . . . . . . . . . 19
4 Parameter Estimation I: Bayes’ Box 21
4.1 Parameter Estimation: Bus Example . . . . . . . . . . . . . . . . . . . . . 22
4.1.1 Sampling Distribution and Likelihood . . . . . . . . . . . . . . . . . 25
4.1.2 What is the “Data”? . . . . . . . . . . . . . . . . . . . . . . . . . . 26
4.2 Prediction in the Bus Problem . . . . . . . . . . . . . . . . . . . . . . . . . 26
4.3 Bayes’ Rule, Parameter Estimation Version . . . . . . . . . . . . . . . . . . 27
5 Parameter Estimation: Analytical Methods 29
5.1 “∼” Notation . . . . . . . . . . . . . . . . . . . . . . . . . . . . . . . . . . 30
5.2 The Effect of Different Priors . . . . . . . . . . . . . . . . . . . . . . . . . 31
5.2.1 Prior 2: Emphasising the Extremes . . . . . . . . . . . . . . . . . . 32
5.2.2 Prior 3: Already Being Well Informed . . . . . . . . . . . . . . . . . 32
5.2.3 The Beta Distribution . . . . . . . . . . . . . . . . . . . . . . . . . 33
5.2.4 A Lot of Data . . . . . . . . . . . . . . . . . . . . . . . . . . . . . . 35
6 Summarising the Posterior Distribution 36
6.1 Point Estimates . . . . . . . . . . . . . . . . . . . . . . . . . . . . . . . . . 37
6.1.1 A Very Brief Introduction to Decision Theory . . . . . . . . . . . . 38
6.1.2 Absolute Loss . . . . . . . . . . . . . . . . . . . . . . . . . . . . . . 39
1
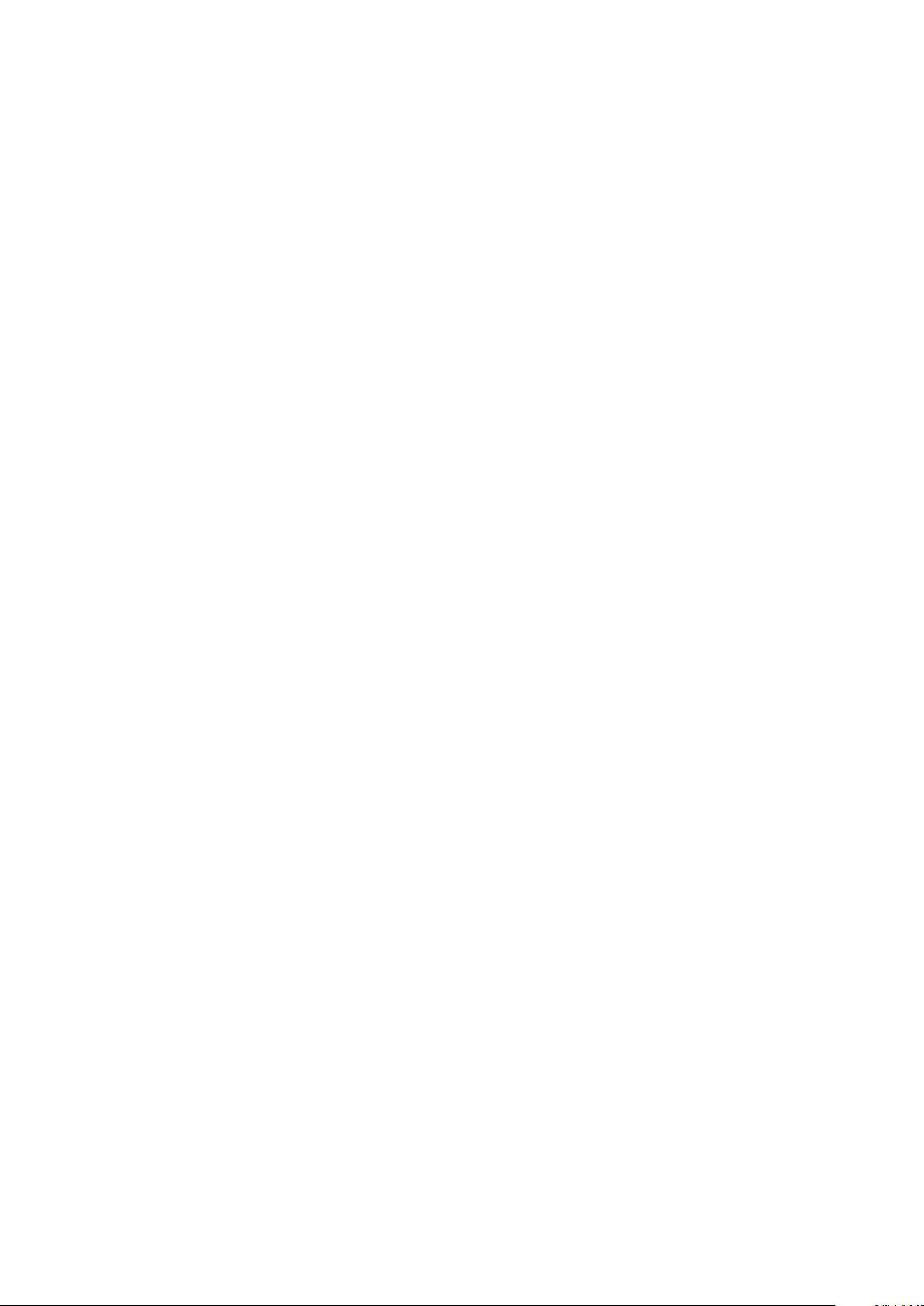
CONTENTS 2
6.1.3 All-or-nothing Loss . . . . . . . . . . . . . . . . . . . . . . . . . . . 40
6.1.4 Invariance of Decisions . . . . . . . . . . . . . . . . . . . . . . . . . 40
6.1.5 Computing Point Estimates from a Bayes’ Box . . . . . . . . . . . . 41
6.1.6 Computing Point Estimates from Samples . . . . . . . . . . . . . . 41
6.2 Credible Intervals . . . . . . . . . . . . . . . . . . . . . . . . . . . . . . . . 42
6.2.1 Computing Credible Intervals from a Bayes’ Box . . . . . . . . . . . 42
6.2.2 Computing Credible Intervals from Samples . . . . . . . . . . . . . 43
6.3 Confidence Intervals . . . . . . . . . . . . . . . . . . . . . . . . . . . . . . 43
7 Hypothesis Testing and Model Selection 45
7.1 An Example Hypothesis Test . . . . . . . . . . . . . . . . . . . . . . . . . 45
7.2 The “Testing” Prior . . . . . . . . . . . . . . . . . . . . . . . . . . . . . . 46
7.3 Some Terminology . . . . . . . . . . . . . . . . . . . . . . . . . . . . . . . 49
7.4 Hypothesis Testing and the Marginal Likelihood . . . . . . . . . . . . . . . 51
8 Markov Chain Monte Carlo 52
8.1 Monte Carlo . . . . . . . . . . . . . . . . . . . . . . . . . . . . . . . . . . . 52
8.1.1 Summaries . . . . . . . . . . . . . . . . . . . . . . . . . . . . . . . . 52
8.2 Multiple Parameters . . . . . . . . . . . . . . . . . . . . . . . . . . . . . . 54
8.3 The Metropolis Algorithm . . . . . . . . . . . . . . . . . . . . . . . . . . . 56
8.3.1 Metropolis, Stated . . . . . . . . . . . . . . . . . . . . . . . . . . . 56
8.4 A Two State Problem . . . . . . . . . . . . . . . . . . . . . . . . . . . . . 58
8.5 The Steady-State Distribution of a Markov Chain . . . . . . . . . . . . . . 59
8.6 Tactile MCMC . . . . . . . . . . . . . . . . . . . . . . . . . . . . . . . . . 60
9 Using JAGS 61
9.1 Basic JAGS Example . . . . . . . . . . . . . . . . . . . . . . . . . . . . . . 62
9.2 Checklist for Using JAGS . . . . . . . . . . . . . . . . . . . . . . . . . . . 65
10 Regression 67
10.1 A Simple Linear Regression Problem . . . . . . . . . . . . . . . . . . . . . 67
10.2 Interpretation as a Bayesian Question . . . . . . . . . . . . . . . . . . . . . 67
10.3 Analytical Solution With Known Variance . . . . . . . . . . . . . . . . . . 68
10.4 Solution With JAGS . . . . . . . . . . . . . . . . . . . . . . . . . . . . . . 70
10.5 Results for “Road” Data . . . . . . . . . . . . . . . . . . . . . . . . . . . . 72
10.6 Predicting New Data . . . . . . . . . . . . . . . . . . . . . . . . . . . . . . 73
10.7 Simple Linear Regression With Outliers . . . . . . . . . . . . . . . . . . . . 75
10.8 Multiple Linear Regression and Logistic Regression . . . . . . . . . . . . . 76
11 Replacements for t-tests and ANOVA 77
11.1 A T-Test Example . . . . . . . . . . . . . . . . . . . . . . . . . . . . . . . 77
11.1.1 Likelihood . . . . . . . . . . . . . . . . . . . . . . . . . . . . . . . . 78
11.1.2 Prior 1: Very Vague . . . . . . . . . . . . . . . . . . . . . . . . . . 79
11.1.3 Prior 2: They might be equal! . . . . . . . . . . . . . . . . . . . . . 79
11.1.4 Prior 3: Alright, they’re not equal, but they might be close . . . . . 80
11.2 One Way Anova . . . . . . . . . . . . . . . . . . . . . . . . . . . . . . . . . 82
11.2.1 Hierarchical Model . . . . . . . . . . . . . . . . . . . . . . . . . . . 83
11.2.2 MCMC Efficiency . . . . . . . . . . . . . . . . . . . . . . . . . . . . 85
11.2.3 An Alternative Parameterisation . . . . . . . . . . . . . . . . . . . 86
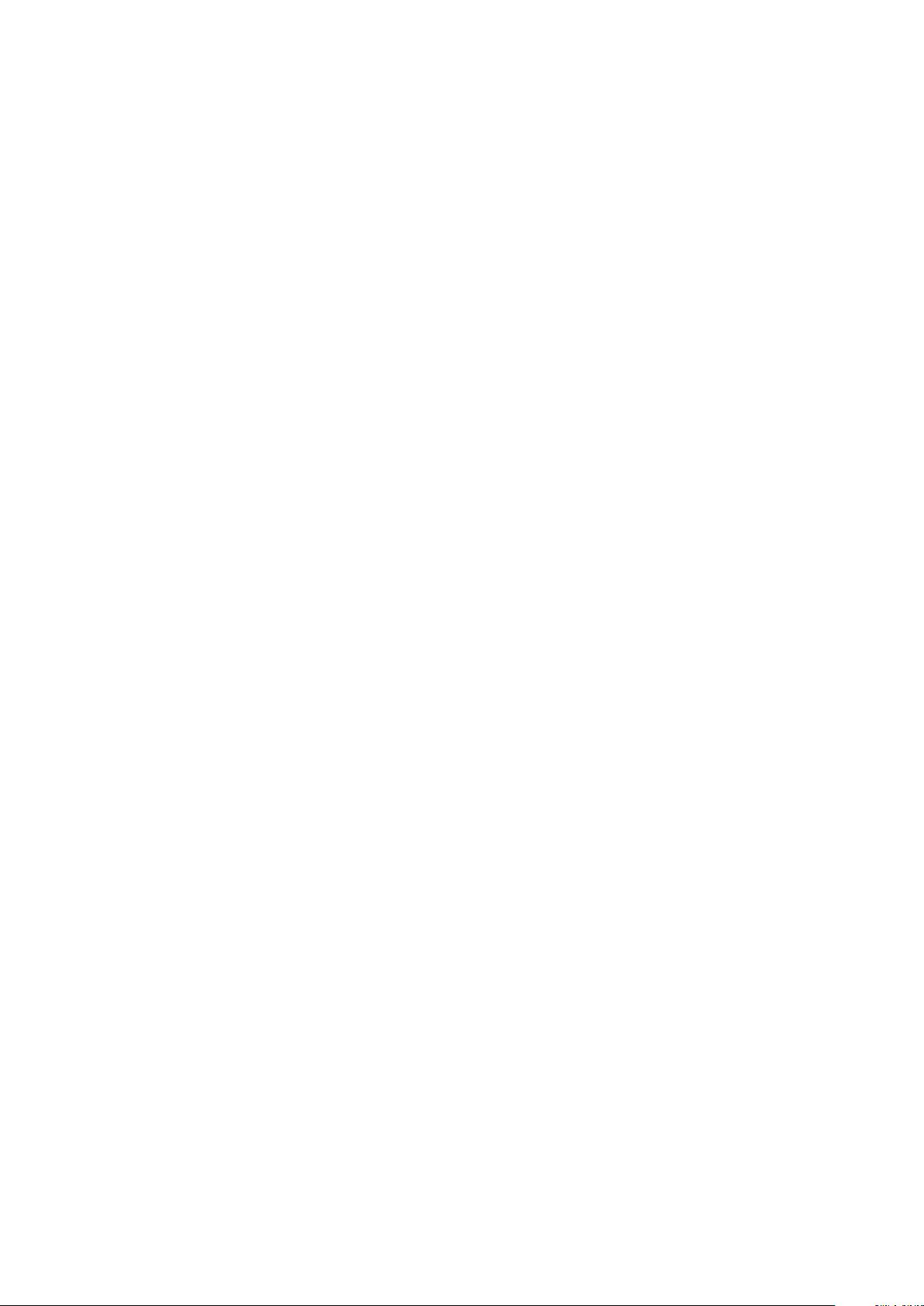
CONTENTS 3
12 Acknowledgements 88
A R Background 89
A.1 Vectors . . . . . . . . . . . . . . . . . . . . . . . . . . . . . . . . . . . . . . 89
A.2 Lists . . . . . . . . . . . . . . . . . . . . . . . . . . . . . . . . . . . . . . . 90
A.3 Functions . . . . . . . . . . . . . . . . . . . . . . . . . . . . . . . . . . . . 90
A.4 For Loops . . . . . . . . . . . . . . . . . . . . . . . . . . . . . . . . . . . . 91
A.5 Useful Probability Distributions . . . . . . . . . . . . . . . . . . . . . . . . 91
A Probability 92
A.1 The Product Rule . . . . . . . . . . . . . . . . . . . . . . . . . . . . . . . . 92
A.1.1 Bayes’ Rule . . . . . . . . . . . . . . . . . . . . . . . . . . . . . . . 93
A.2 The Sum Rule . . . . . . . . . . . . . . . . . . . . . . . . . . . . . . . . . . 93
A.3 Random Variables . . . . . . . . . . . . . . . . . . . . . . . . . . . . . . . . 94
A.3.1 Discrete Random Variables . . . . . . . . . . . . . . . . . . . . . . . 94
A.3.2 Continuous Random Variables . . . . . . . . . . . . . . . . . . . . . 94
A.3.3 Shorthand Notation . . . . . . . . . . . . . . . . . . . . . . . . . . . 95
A.4 Useful Probability Distributions . . . . . . . . . . . . . . . . . . . . . . . . 95
A Rosetta Stone 96
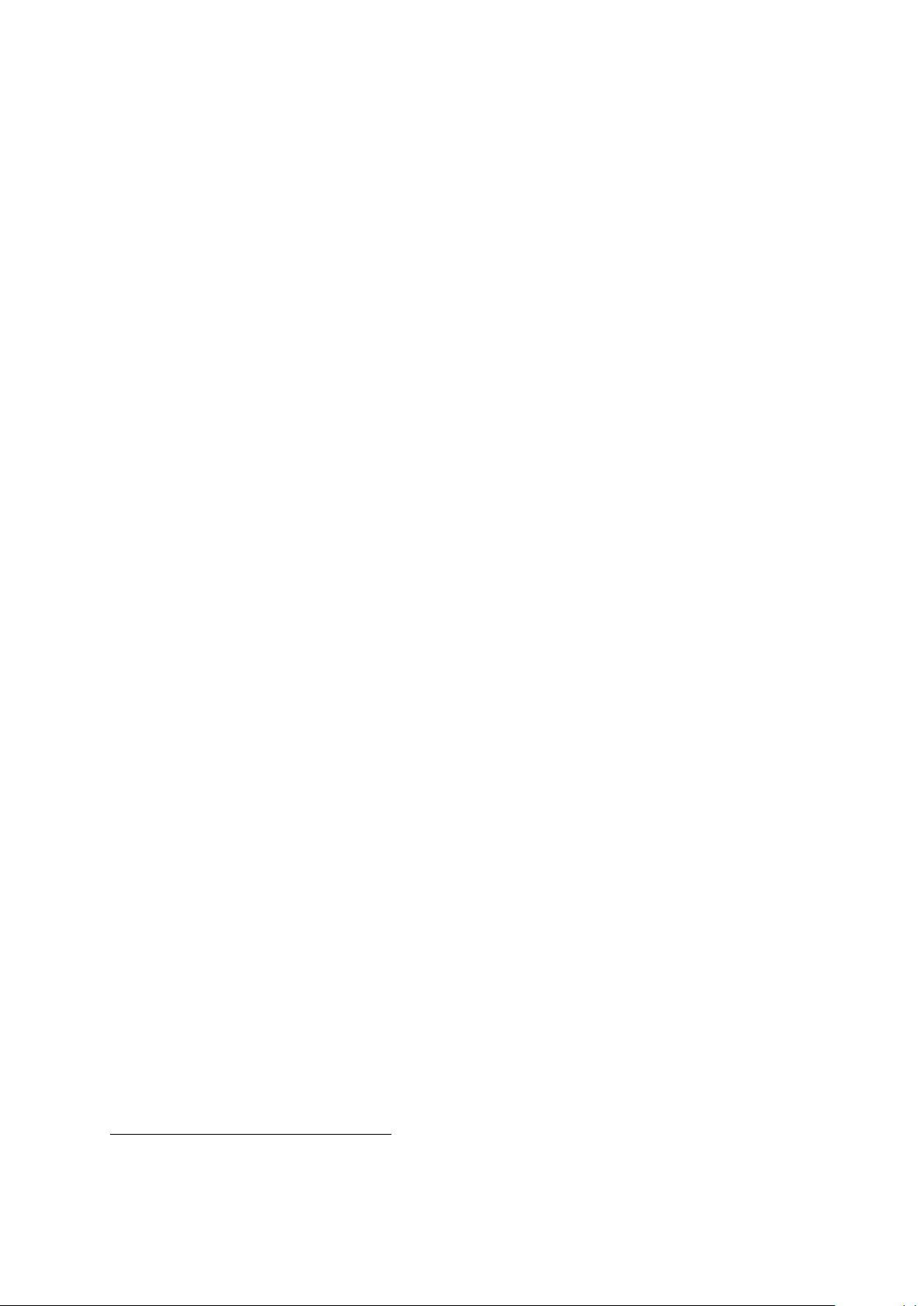
Chapter 1
Prologue
This course was originally developed by Dr Wayne Stewart (formerly of The University of
Auckland) and was first offered in 2009 (Figure 1.1). I joined the Department of Statistics
in July 2012 and took over the course from him. It was good fortune for me that Wayne
left the university as I arrived. If I had been able to choose which undergraduate course I
would most like to teach, it would have been this one!
Wayne is a passionate Bayesian
1
and advocate for the inclusion of Bayesian statistics in
the undergraduate statistics curriculum. I also consider myself a Bayesian and agree that
this approach to statistics should form a greater part of statistics education than it does
today. While this edition of the course differs from Wayne’s in some ways
2
, I hope I am
able to do the topic justice in an accessible way.
In this course we will use the following software:
• R (http://www.r-project.org/)
• JAGS (http://mcmc-jags.sourceforge.net/)
• The rjags package in R
• RStudio (http://www.rstudio.com/)
You will probably have used R, at least a little bit, in previous statistics courses. RStudio
is just a nice program for editing R code, and if you don’t like it, you’re welcome to use
any other text editor. JAGS is in a different category and you probably won’t have seen
it before. JAGS is used to implement Bayesian methods in a straightforward way, and
rjags
allows us to use JAGS from within R. Don’t worry, it’s not too difficult to learn
and use JAGS! We will have a lot of practice using it in the labs.
These programs are all free and open source software. That is, they are free to use, share
and modify. They should work on virtually any operating system including the three
1
Bayesian statistics has a way of creating extreme enthusiasm among its users. I don’t just use Bayesian
methods, I am a Bayesian.
2
The differences are mostly cosmetic. 90% of the content is the same.
4
剩余97页未读,继续阅读
资源评论
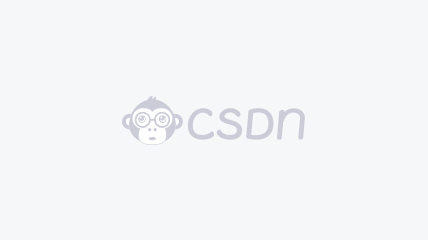

Ribbery
- 粉丝: 1
- 资源: 3
上传资源 快速赚钱
我的内容管理 展开
我的资源 快来上传第一个资源
我的收益
登录查看自己的收益我的积分 登录查看自己的积分
我的C币 登录后查看C币余额
我的收藏
我的下载
下载帮助

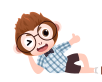
最新资源
- MATLAB: 双重改进的蚁狮优化算法-连续性边界收缩与动态权重系数的融合优化,MATLAB实现:双改进策略的蚁狮优化算法(IALO)与原始ALO算法的对比分析,matlab:一种改进的蚁狮优化算法
- 流程图-demo-总结的一些流程图
- 管家婆辉煌食品普及版TOP13.11.zip
- 基于人工势场算法的Matlab路径规划系统:自定义起始与目标点,栅格地图路径规划的优化实践,基于人工势场算法的Matlab路径规划算法研究与实现:栅格地图路径规划的自定义起始与目标点设置,人工势场算法
- 管家婆辉煌食品普及版TOP13.22.zip
- 管家婆辉煌食品普及版TOP13.32.zip
- AccessPort-ReceLend-2048
- 自定义A星算法路径规划与二维度码路径优化研究指南:附有详尽注释的代码,本地图自由更换指南 ,深入解析:自定义A星算法二维路径规划的代码实现与地图替换指南,A星算法路径规划 自己编写的Astar二维路
- 紫光拼音输入法-紫光拼音(Ziguang Pinyin)是一款中文拼音输入法软件,由清华大学和紫光集团联合开发
- osg、osgEarth加载tms瓦片数据只显示一个白球,缩放过程中图层消失
- 个人理财系统springboot+Thymeleaf+mysql
- Python网站自动登录项目+采用selenium+webdriver
- 基于A*算法的Matlab多AGV路径规划仿真系统:地图自定义导入,算法优化平滑路径,实时输出时空图与坐标信息 ,基于A*算法的Matlab多AGV路径规划仿真系统:地图自定义导入,优化路径平滑处理
- 基于混合整数二阶锥规划的配电网重构求解与代码详解-计及高比例清洁能源接入与需求响应,《高比例清洁能源接入下计及需求响应的配电网重构算法:混合整数二阶锥优化实现与代码解析》,EI复现高比例清洁能源接
- 管家婆辉煌食品版TOP+12.9.zip
- 管家婆辉煌食品普及版TOP15.0.zip
资源上传下载、课程学习等过程中有任何疑问或建议,欢迎提出宝贵意见哦~我们会及时处理!
点击此处反馈


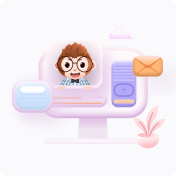
安全验证
文档复制为VIP权益,开通VIP直接复制
