# Implicit-Discourse-Relation-Recognition
Identifying IDRR in paragraph sentences using BERT models - 基于 BERT 类模型的隐式语篇关系识别(NLP)
<br>
## Abstract
Implicit discourse relationship identification aims to discover the semantic relationship between two sentences with missing discourse connectives. The discourse relationship between two text fragments plays a key role in many natural language processing tasks. Conjunctives strongly indicate the meaning of discourse relations, while discourses without conjunctives are called implicit discourse. Compared with explicit relations, implicit relations are harder to detect, and the key to their prediction is to correctly model the semantics of two discourse arguments, as well as the contextual interaction between them. This paper proposes several frameworks based on the BERT encoder model, including BERT base, ALBERT, DistilBERT base, RoBERTa and other pre-trained models.
<br>
## Background
A discourse usually contains one or more sentences to describe daily events and for people to exchange ideas and opinions. Since a chapter is usually composed of multiple text fragments, the relationship between text fragments should be considered in order to accurately understand the theme of the chapter. There are often connectives used to express relationships in the text, but there are no connectives between some text fragments, but there is an implicit relationship between them. The task of implicit textual relation recognition is to detect implicit relations and classify their meanings between two text fragments without connectives. The IDRR task has played a crucial role in various downstream natural language processing tasks such as text summarization, machine translation, etc. This paper provides solutions to various BERT pre-trained models for the implicit discourse relationship recognition task.
<br>
## Problem Description
Discourse Relation Recognition (DRR) aims to identify whether there is a logical relationship between two text fragments in a discourse; if it exists, it further classifies the relationship meaning into some predefined types, such as temporal (temporal), causal ( Contingency), comparison (comparison) and extension (expansion) and other relations. An article may contain one or more connective words between text fragments. A connective word is a vocabulary that can directly express the meaning of a certain relationship. For example, the connective word "because" usually expresses a causal relationship between two text fragments. If there are connectives in the discourse relation recognition, it is called Explicit Discourse Relation Recognition (EDRR), otherwise it is called Implicit Discourse Relation Recognition (IDRR). This experiment uses the Penn Discourse Tree Bank 2.0 (PDTB-2.0) corpus as the input sample of the model.
<br>
## Solution
Neural network experiments successively adopted different types of BERT models to train the PDTB-2.0 dataset and classify the implicit discourse relations of the chapters. Firstly, BERT BASE, ALBERT BASE, DistilBERT BASE and other models were used for training in the experiment, but the effect was not good, and the F1 values were 56.31, 56.81, and 50.19 respectively. After improvement, RoBERTa BASE, a model based on tag-dependent perceptual sequence generation, was used to achieve an F1 value of 62.08; and then tried to use the RoBERTa LARGE model, and through parameter adjustment, an F1 value of 69.76 was achieved. Experiments have verified that BERT can improve the accuracy and F1 value of many natural language processing and language modeling tasks, and it plays an important role in IDRR tasks.
<br>
## Experimental setup
Environment: Visual Studio Code 1.74.1 + Python 3.7.7 + NVDIA GeForce RTX 3090
Data: Penn Discourse Tree Bank 2.0(PDTB-2.0)
<br>
## Algorithm flowchart

<br>
## Result
| Model | Results |
| :---------------: | :------------------------------------------------------------: |
| BERT BASE |  |
| ALBERT BASE |  |
| DistilBERT BASE |  |
| RoBERTa BASE |  |
| RoBERTa LARGE |  |
<br>
As shown in table above, it can be observed that the effect of using DistilBERT BASE at the beginning is not good, only reaching the F1 value of 50.19. This is due to the streamlined architecture of the DistilBERT model, which performs knowledge distillation in the pre-training phase, resulting in a 40% reduction in model size compared to the BERT model, a reduction in the number of layers, and much fewer trainable parameters. Among them, BERT's word segmentation embedding and pooling layers were deleted at the same time, and finally the number of DistilBERT BASE layers was reduced by 2 times. After trying to train the model on BERT BASE and ALBERT BASE, the experimental effect has been slightly improved, that is, the F1 values of 56.31 and 56.81 were respectively achieved, and the training time is equivalent to twice that of the DistilBERT BASE model.
In the experiment, the RoBERTa model was used again for IDRR task training. The BASE version achieved a higher F1 value of 62.08, while the performance improvement of the LARGE version was more significant, reaching an F1 value of 69.76. RoBERTa works much better than other BERT-like models because the model uses dynamic masking, where different parts of the sentence are masked at each iteration, making the model more performant; other BERT-like models use static masking. The batch size used by RoBERTa is much larger than that of BERT, and the training data set is also much larger than that of BERT, which is about 160 GB; while BERT only trains about 16 GB of data, so RoBERTa is more robust on IDRR tasks than Other BERT-like models are high.
<br>
## Conclusion
This project emphasizes the usage of various BERT-like models to train the Implicit Discourse Relation Discrimination (IDRR) task, and a deep understanding on variables such as model framework, number of parameters, model size, hyperparameters, etc. influence on the training effect. Compared with DistilBERT BASE, ALBERT BASE, BERT BASE, and RoBERTa BASE, RoBERTa LARGE model training has reached the highest F1 value, improving the overall model performance and accuracy.
没有合适的资源?快使用搜索试试~ 我知道了~
温馨提示
项目工程资源经过严格测试可直接运行成功且功能正常的情况才上传,可轻松复刻,拿到资料包后可轻松复现出一样的项目,本人系统开发经验充足(全领域),有任何使用问题欢迎随时与我联系,我会及时为您解惑,提供帮助。 【资源内容】:包含完整源码+工程文件+说明(如有)等。答辩评审平均分达到96分,放心下载使用!可轻松复现,设计报告也可借鉴此项目,该资源内项目代码都经过测试运行成功,功能ok的情况下才上传的。 【提供帮助】:有任何使用问题欢迎随时与我联系,我会及时解答解惑,提供帮助 【附带帮助】:若还需要相关开发工具、学习资料等,我会提供帮助,提供资料,鼓励学习进步 【项目价值】:可用在相关项目设计中,皆可应用在项目、毕业设计、课程设计、期末/期中/大作业、工程实训、大创等学科竞赛比赛、初期项目立项、学习/练手等方面,可借鉴此优质项目实现复刻,设计报告也可借鉴此项目,也可基于此项目来扩展开发出更多功能 下载后请首先打开README文件(如有),项目工程可直接复现复刻,如果基础还行,也可在此程序基础上进行修改,以实现其它功能。供开源学习/技术交流/学习参考,勿用于商业用途。质量优质,放心下载使用。
资源推荐
资源详情
资源评论
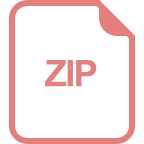
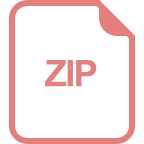
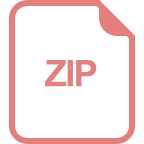
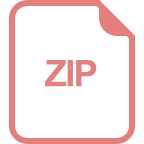
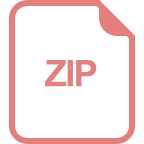
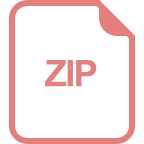
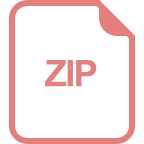
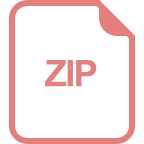
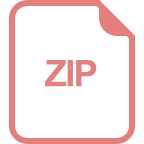
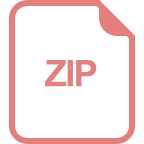
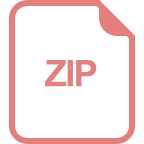
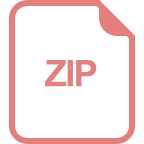
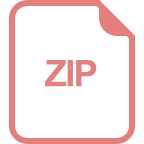
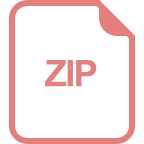
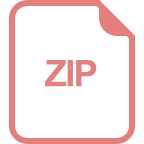
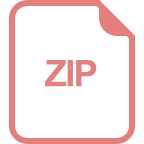
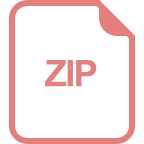
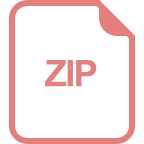
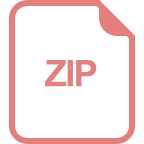
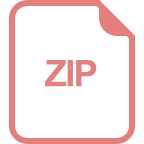
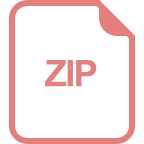
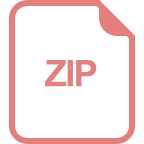
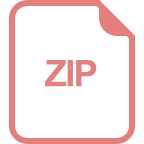
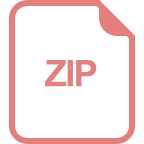
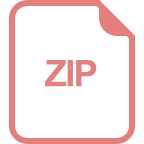
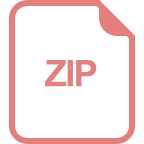
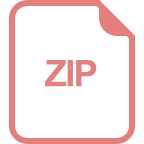
收起资源包目录


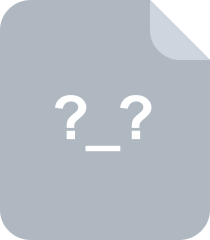
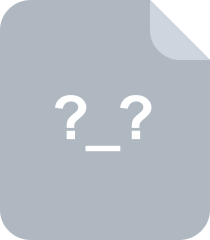
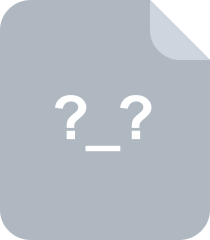

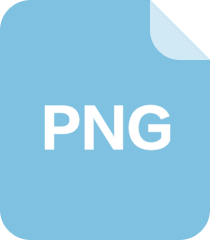
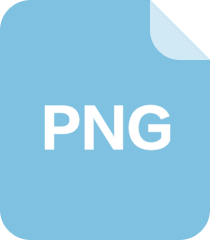
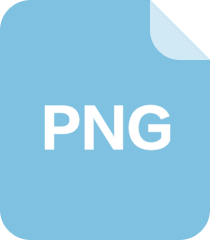
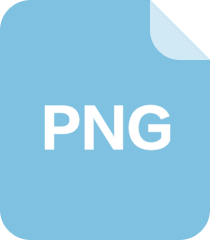
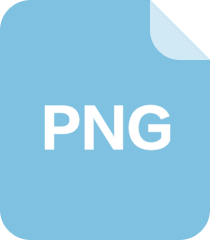
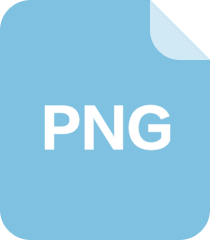
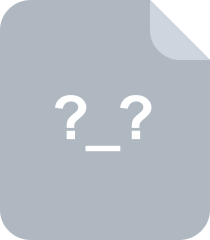
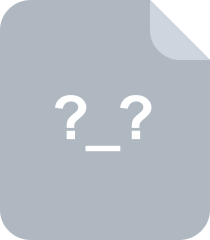
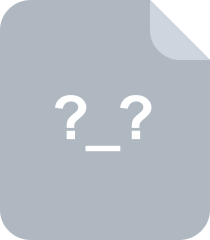
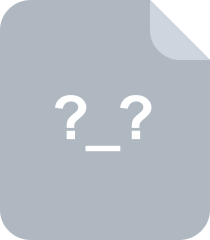



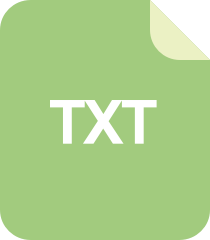
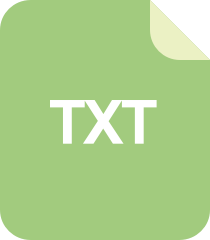
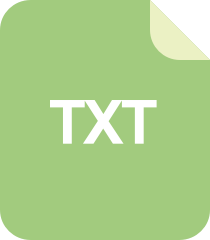
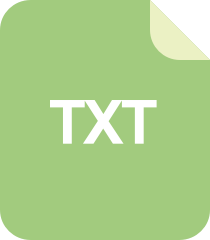
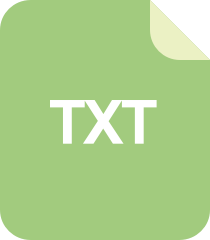
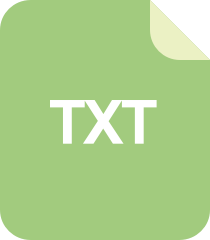

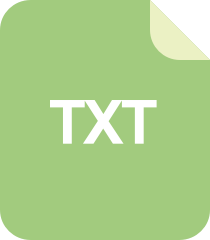

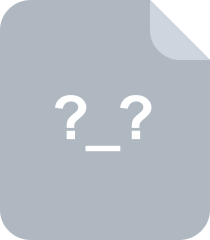
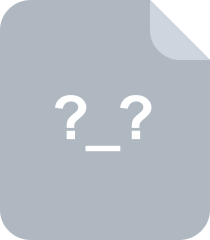
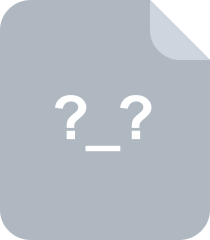
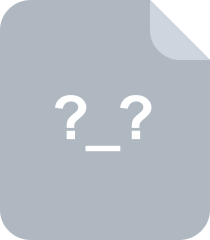
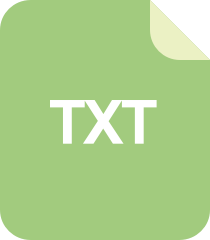
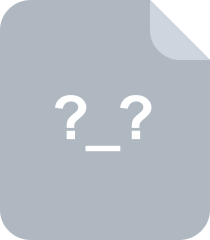
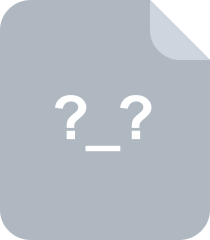
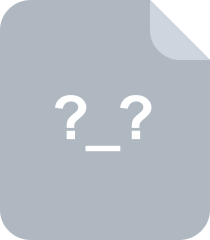
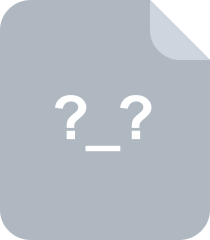
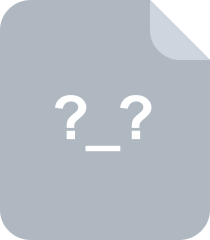

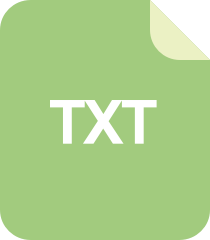
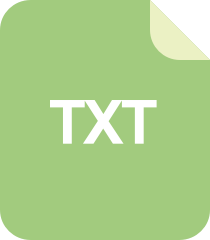
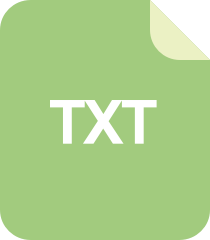
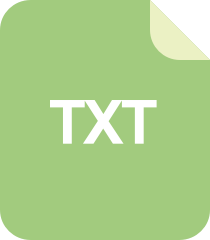
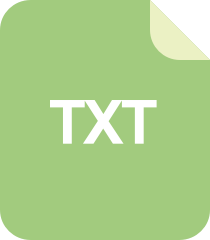

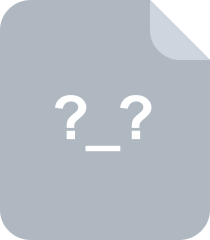
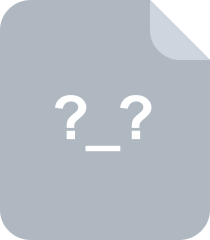
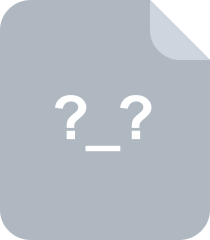
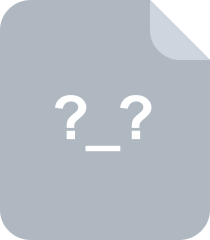
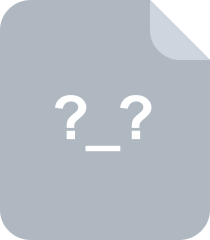
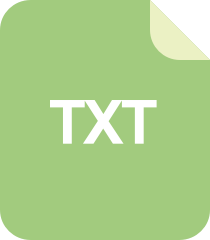
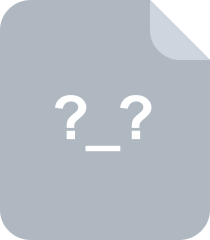
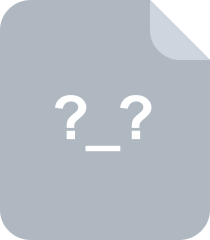
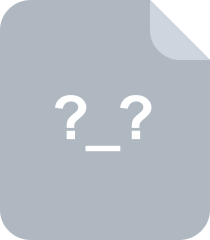
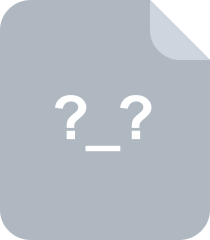
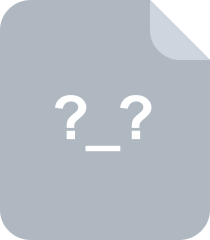
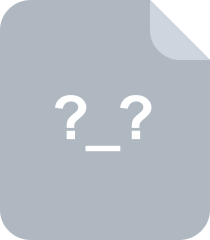
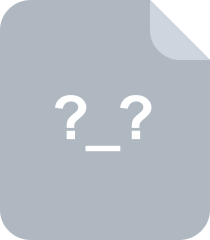

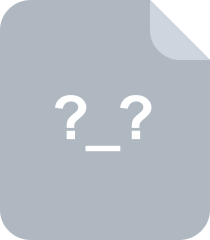
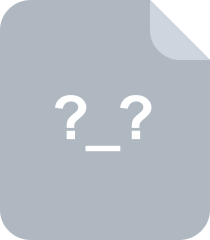
共 50 条
- 1
资源评论
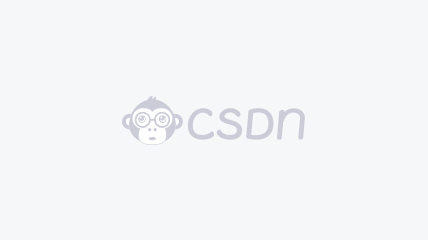

热爱技术。
- 粉丝: 2486
- 资源: 7862
上传资源 快速赚钱
我的内容管理 展开
我的资源 快来上传第一个资源
我的收益
登录查看自己的收益我的积分 登录查看自己的积分
我的C币 登录后查看C币余额
我的收藏
我的下载
下载帮助

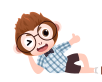
最新资源
资源上传下载、课程学习等过程中有任何疑问或建议,欢迎提出宝贵意见哦~我们会及时处理!
点击此处反馈


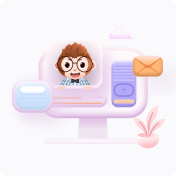
安全验证
文档复制为VIP权益,开通VIP直接复制
