In ROMA's ICML paper, we use [an old version of the SMAC benchmark](https://arxiv.org/pdf/1902.04043v1.pdf) for both ROMA and the baselines (QMIX, COMA, IQL, MAVEN, QTRAN), and their performance are different from that can be achieved by the latest version.
# ROMA: Multi-Agent Reinforcement Learning with Emergent Roles
## Note
This codebase accompanies the paper submission "ROMA: Multi-Agent Reinforcement Learning with Emergent Roles" ([ROMA website](https://sites.google.com/view/romarl)), and is based on [PyMARL](https://github.com/oxwhirl/pymarl) and [SMAC](https://github.com/oxwhirl/smac) codebases which are open-sourced.
The implementation of the following methods can also be found in this codebase, which are finished by the authors of [PyMARL](https://github.com/oxwhirl/pymarl):
- [**ROMA**: ROMA: Multi-Agent Reinforcement Learning with Emergent Roles](https://arxiv.org/abs/2003.08039)
- [**QMIX**: QMIX: Monotonic Value Function Factorisation for Deep Multi-Agent Reinforcement Learning](https://arxiv.org/abs/1803.11485)
- [**COMA**: Counterfactual Multi-Agent Policy Gradients](https://arxiv.org/abs/1705.08926)
- [**VDN**: Value-Decomposition Networks For Cooperative Multi-Agent Learning](https://arxiv.org/abs/1706.05296)
- [**IQL**: Independent Q-Learning](https://arxiv.org/abs/1511.08779)
## Installation instructions
Build the Dockerfile using
```shell
cd docker
bash build.sh
```
Set up StarCraft II and SMAC:
```shell
bash install_sc2.sh
```
This will download SC2 into the 3rdparty folder and copy the maps necessary to run over.
If you want to run the environments we designed, move all the SC2 maps in `src/envs/starcraft2/map/designed/` to `3rdparty/StarCraftII/Maps/SMAC_Maps/`.
It is worth noting that `bane_vs_bane1` corresponds to `6z4b`, `zb_vs_sz` corresponds to `10z5b_vs_2s3z`, and `sz_vs_zb`
corresponds to `6s4z_vs_10b30z` in the paper.
The requirements.txt file can be used to install the necessary packages into a virtual environment (not recomended).
## Run an experiment
```shell
python3 src/main.py
--config=qmix_smac_latent
--env-config=sc2
with
agent=latent_ce_dis_rnn
env_args.map_name=MMM2
t_max=20050000
```
To test other maps, add parameters
```shell
h_loss_weight=5e-2
var_floor=1e-4
```
The config files act as defaults for an algorithm or environment.
They are all located in `src/config`.
`--config` refers to the config files in `src/config/algs`
`--env-config` refers to the config files in `src/config/envs`
All results will be stored in the `Results` folder.
## Saving and loading learnt models
### Saving models
You can save the learnt models to disk by setting `save_model = True`, which is set to `False` by default. The frequency of saving models can be adjusted using `save_model_interval` configuration. Models will be saved in the result directory, under the folder called *models*. The directory corresponding each run will contain models saved throughout the experiment, each within a folder corresponding to the number of timesteps passed since starting the learning process.
### Loading models
Learnt models can be loaded using the `checkpoint_path` parameter, after which the learning will proceed from the corresponding timestep.
## Watching StarCraft II replays
`save_replay` option allows saving replays of models which are loaded using `checkpoint_path`. Once the model is successfully loaded, `test_nepisode` number of episodes are run on the test mode and a .SC2Replay file is saved in the Replay directory of StarCraft II. Please make sure to use the episode runner if you wish to save a replay, i.e., `runner=episode`. The name of the saved replay file starts with the given `env_args.save_replay_prefix` (map_name if empty), followed by the current timestamp.
The saved replays can be watched by double-clicking on them or using the following command:
```shell
python -m pysc2.bin.play --norender --rgb_minimap_size 0 --replay NAME.SC2Replay
```
**Note:** Replays cannot be watched using the Linux version of StarCraft II. Please use either the Mac or Windows version of the StarCraft II client.


不去幼儿园
- 粉丝: 1w+
- 资源: 44
最新资源
- 晋升SUNM2引擎专用微端完整源码2024最新定制Delphi D11.3.2版
- Pure Go WebRTC SFU.zip
- 基于Python、HTML、JavaScript、CSS的咖啡主题网站设计源码
- 基于java_springboot+大数据的智能家居销量数据分析系统设计实现(代码+数据库+演示录像+运行教学+软件下载)
- React.js Google Maps 集成组件.zip
- Ruby dotenv 库的 Go 端口(从 .env 文件加载环境变量).zip
- 基于Java语言的Git版本控制系统访问与核心算法设计源码库
- 基于java_springboot的web酒店客房管理系统设计实现(代码+数据库+论文+演示录像+运行教学+软件下载).zip
- Ruby 进程监视器.zip
- 第三次函数培训.zip
- tb_image_share_1733150361392.jpg.png
- Sarama 是 Apache Kafka 的 Go 库 .zip
- 基于Vue的刷脸支付系统及OEM定制设计源码
- Hexagon Fall 六角坠落跑酷模板+编辑器 Unity益智休闲火柴人游戏插件项目源码C#
- Sarasa Gothic , 更纱黑体 , 更纱黑体 , 更纱ゴshikku , 사라사 고딕.zip
- 基于java_springboot的web新能源充电系统设计实现(代码+数据库+论文+演示录像+运行教学+软件下载).zip
资源上传下载、课程学习等过程中有任何疑问或建议,欢迎提出宝贵意见哦~我们会及时处理!
点击此处反馈


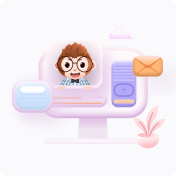