<p align="center">
<img src="test/images/macadam_logo.png" width="480"\>
</p>
# [Macadam](https://github.com/yongzhuo/Macadam)
[](https://pypi.org/project/Macadam/)
[](https://travis-ci.com/yongzhuo/Macadam)
[](https://pypi.org/project/Macadam/)
[](https://github.com/yongzhuo/Macadam/stargazers)
[](https://github.com/yongzhuo/Macadam/network/members)
[](https://gitter.im/yongzhuo/Macadam?utm_source=badge&utm_medium=badge&utm_campaign=pr-badge&utm_content=badge)
>>> Macadam是一个以Tensorflow(Keras)和bert4keras为基础,专注于文本分类、序列标注和关系抽取的自然语言处理工具包。支持RANDOM、WORD2VEC、FASTTEXT、BERT、ALBERT、ROBERTA、NEZHA、XLNET、ELECTRA、GPT-2等EMBEDDING嵌入;
支持FineTune、FastText、TextCNN、CharCNN、BiRNN、RCNN、DCNN、CRNN、DeepMoji、SelfAttention、HAN、Capsule等文本分类算法;
支持CRF、Bi-LSTM-CRF、CNN-LSTM、DGCNN、Bi-LSTM-LAN、Lattice-LSTM-Batch、MRC等序列标注算法。
## 目录
* [安装](#安装)
* [数据](#数据)
* [使用方式](#使用方式)
* [TODO](#TODO)
* [paper](#paper)
* [参考](#参考)
# 安装
```bash
pip install Macadam
# 清华镜像源
pip install -i https://pypi.tuna.tsinghua.edu.cn/simple Macadam
```
# 数据
## 数据来源
* [ner_clue_2020](https://github.com/CLUEbenchmark/CLUENER2020), CLUENER2020中文细粒度命名实体识别
* [ner_people_1998](http://www.icl.pku.edu.cn/icl_res/), 《人民日报》标注语料库中的语料, 1998.01
* [baidu_qa_2019](https://github.com/liuhuanyong/MiningZhiDaoQACorpus), 百度知道问答语料
* [thucnews](http://thuctc.thunlp.org/), 新浪新闻RSS订阅频道2005-2011年间的历史数据筛
## 数据格式
```
1. 文本分类 (txt格式, 每行为一个json):
{"x": {"text": "人站在地球上为什么没有头朝下的感觉", "texts2": []}, "y": "教育"}
{"x": {"text": "我的小baby", "texts2": []}, "y": ["娱乐"]}
{"x": {"text": "请问这起交通事故是谁的责任居多小车和摩托车发生事故在无红绿灯", "texts2": []}, "y": "娱乐"}
2. 序列标注 (txt格式, 每行为一个json):
{"x": {"text": "海钓比赛地点在厦门与金门之间的海域。", "texts2": []}, "y": ["O", "O", "O", "O", "O", "O", "O", "B-LOC", "I-LOC", "O", "B-LOC", "I-LOC", "O", "O", "O", "O", "O", "O"]}
{"x": {"text": "参加步行的有男有女,有年轻人,也有中年人。", "texts2": []}, "y": ["O", "O", "O", "O", "O", "O", "O", "O", "O", "O", "O", "O", "O", "O", "O", "O", "O", "O", "O", "O", "O"]}
{"x": {"text": "山是稳重的,这是我最初的观念。", "texts2": []}, "y": ["O", "O", "O", "O", "O", "O", "O", "O", "O", "O", "O", "O", "O", "O", "O"]}
{"x": {"text": "立案不结、以罚代刑等问题有较大改观。", "texts2": []}, "y": ["O", "O", "O", "O", "O", "O", "O", "O", "O", "O", "O", "O", "O", "O", "O", "O", "O", "O"]}
```
# 使用方式
更多样例sample详情见test目录
## 文本分类, text-classification
```bash
# !/usr/bin/python
# -*- coding: utf-8 -*-
# @time : 2020/5/8 21:33
# @author : Mo
# @function: test trainer of bert
# 适配linux
import sys
import os
path_root = os.path.abspath(os.path.join(os.path.dirname(__file__), "../.."))
sys.path.append(path_root)
# cpu-gpu与tf.keras
os.environ["CUDA_VISIBLE_DEVICES"] = "0"
os.environ["TF_KERAS"] = "1"
# macadam
from macadam.conf.path_config import path_root, path_tc_baidu_qa_2019, path_tc_thucnews
from macadam.tc import trainer
if __name__=="__main__":
# bert-embedding地址, 必传
path_embed = "D:/soft_install/dataset/bert-model/chinese_L-12_H-768_A-12"
path_checkpoint = path_embed + "/bert_model.ckpt"
path_config = path_embed + "/bert_config.json"
path_vocab = path_embed + "/vocab.txt"
# 训练/验证数据地址, 必传
# path_train = os.path.join(path_tc_thucnews, "train.json")
# path_dev = os.path.join(path_tc_thucnews, "dev.json")
path_train = os.path.join(path_tc_baidu_qa_2019, "train.json")
path_dev = os.path.join(path_tc_baidu_qa_2019, "dev.json")
# 网络结构, 嵌入模型, 大小写都可以, 必传
# 网络模型架构(Graph), "FineTune", "FastText", "TextCNN", "CharCNN",
# "BiRNN", "RCNN", "DCNN", "CRNN", "DeepMoji", "SelfAttention", "HAN", "Capsule"
network_type = "TextCNN"
# 嵌入(embedding)类型, "ROBERTA", "ELECTRA", "RANDOM", "ALBERT", "XLNET", "NEZHA", "GPT2", "WORD", "BERT"
embed_type = "BERT"
# token级别, 一般为"char", 只有random和word的embedding时存在"word"
token_type = "CHAR"
# 任务, "TC"(文本分类), "SL"(序列标注), "RE"(关系抽取)
task = "TC"
# 模型保存目录, 必传
path_model_dir = os.path.join(path_root, "data", "model", network_type)
# 开始训练, 可能前几轮loss较大acc较低, 后边会好起来
trainer(path_model_dir, path_embed, path_train, path_dev, path_checkpoint, path_config, path_vocab,
network_type=network_type, embed_type=embed_type, token_type=token_type, task=task)
mm = 0
```
## 序列标注, sequence-labeling
```bash
# !/usr/bin/python
# -*- coding: utf-8 -*-
# @time : 2020/5/8 21:33
# @author : Mo
# @function: test trainer of bert
# 适配linux
import sys
import os
path_root = os.path.abspath(os.path.join(os.path.dirname(__file__), "../.."))
sys.path.append(path_root)
## cpu-gpu与tf.keras
# os.environ["CUDA_VISIBLE_DEVICES"] = "0"
os.environ["TF_KERAS"] = "1"
# 地址, tf.keras
from macadam.conf.path_config import path_embed_bert, path_embed_word2vec_word, path_embed_word2vec_char
from macadam.conf.path_config import path_root, path_ner_people_1998, path_ner_clue_2020
from macadam.sl import trainer
if __name__=="__main__":
# bert-embedding地址, 必传
path_embed = path_embed_bert # path_embed_bert, path_embed_word2vec_word, path_embed_word2vec_char
path_checkpoint = os.path.join(path_embed + "bert_model.ckpt")
path_config = os.path.join(path_embed + "bert_config.json")
path_vocab = os.path.join(path_embed + "vocab.txt")
# # 训练/验证数据地址
# path_train = os.path.join(path_ner_people_1998, "train.json")
# path_dev = os.path.join(path_ner_people_1998, "dev.json")
path_train = os.path.join(path_ner_clue_2020, "ner_clue_2020.train")
path_dev = os.path.join(path_ner_clue_2020, "ner_clue_2020.dev")
# 网络结构
# "CRF", "Bi-LSTM-CRF", "Bi-LSTM-LAN", "CNN-LSTM", "DGCNN", "LATTICE-LSTM-BATCH"
network_type = "CRF"
# 嵌入(embedding)类型, "ROOBERTA", "ELECTRA", "RANDOM", "ALBERT", "XLNET", "NEZHA", "GPT2", "WORD", "BERT"
# MIX, WC_LSTM时候填两个["RANDOM", "WORD"], ["WORD", "RANDOM"], ["RANDOM", "RANDOM"], ["WORD", "WORD"]
embed_type = "RANDOM"
token_type = "CHAR"
task = "SL"
lr = 1e-5 if embed_type in ["ROBERTA", "ELECTRA", "ALBERT", "XLNET", "NEZHA", "GPT2", "BERT"] else 1e-3
# 模型保存目录, 如果不存在则创建
path_model_dir = os.path.join(path_root, "data", "model", network_type)
if not os.path.exists(path_model_dir):
os.mkdir(path_model_dir)
# 开始训练
trainer(path_model_dir, path_embed, path_train, path_dev,
path_checkpoint, path_config, path_vocab,
network_type=network_type, embed_type=embed_type,
task=task, token_type=token_type,
is_length_max=False, use_onehot=False, use_file=False, use_crf=True,
layer_idx=[-2], learning_rate=lr,
batch_size=30, epochs=12, early_stop=6, rate=1)
mm = 0
```
# TODO
* 文本分�
没有合适的资源?快使用搜索试试~ 我知道了~
Macadam是一个以Tensorflow和bert4keras为基础,专注于文本分类、序列标注和关系抽取的自然语言处理工具包
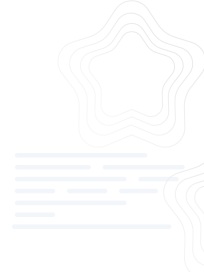
共108个文件
py:83个
txt:7个
json:7个

需积分: 5 0 下载量 69 浏览量
2024-05-22
13:44:04
上传
评论
收藏 1001KB ZIP 举报
温馨提示
LSTM (Long Short-Term Memory) 是一种特殊的循环神经网络(RNN)架构,用于处理具有长期依赖关系的序列数据。传统的RNN在处理长序列时往往会遇到梯度消失或梯度爆炸的问题,导致无法有效地捕捉长期依赖。LSTM通过引入门控机制(Gating Mechanism)和记忆单元(Memory Cell)来克服这些问题。 以下是LSTM的基本结构和主要组件: 记忆单元(Memory Cell):记忆单元是LSTM的核心,用于存储长期信息。它像一个传送带一样,在整个链上运行,只有一些小的线性交互。信息很容易地在其上保持不变。 输入门(Input Gate):输入门决定了哪些新的信息会被加入到记忆单元中。它由当前时刻的输入和上一时刻的隐藏状态共同决定。 遗忘门(Forget Gate):遗忘门决定了哪些信息会从记忆单元中被丢弃或遗忘。它也由当前时刻的输入和上一时刻的隐藏状态共同决定。 输出门(Output Gate):输出门决定了哪些信息会从记忆单元中输出到当前时刻的隐藏状态中。同样地,它也由当前时刻的输入和上一时刻的隐藏状态共同决定。 LSTM的计算过程可以大致描述为: 通过遗忘门决定从记忆单元中丢弃哪些信息。 通过输入门决定哪些新的信息会被加入到记忆单元中。 更新记忆单元的状态。 通过输出门决定哪些信息会从记忆单元中输出到当前时刻的隐藏状态中。 由于LSTM能够有效地处理长期依赖关系,它在许多序列建模任务中都取得了很好的效果,如语音识别、文本生成、机器翻译、时序预测等。
资源推荐
资源详情
资源评论
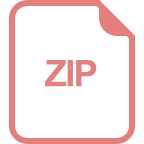
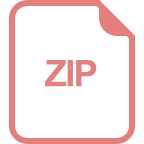
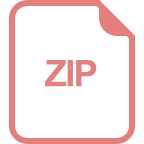
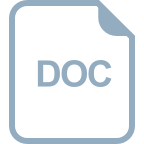
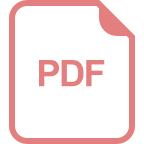
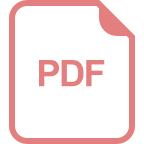
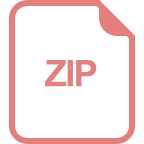
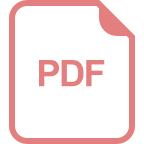
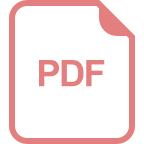
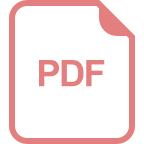
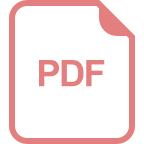
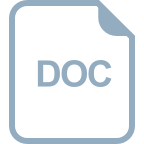
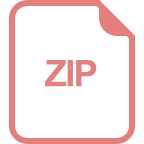
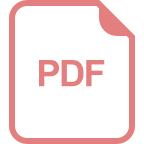
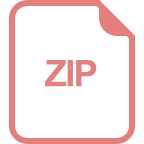
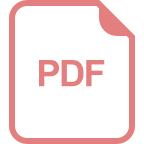
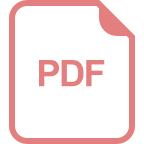
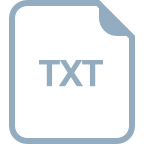
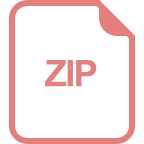
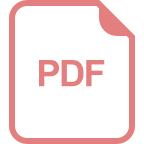
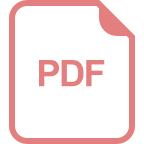
收起资源包目录

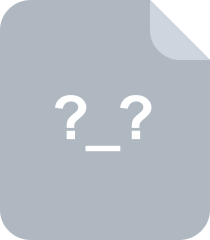
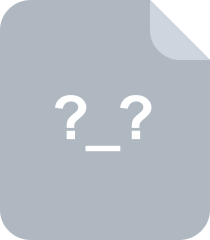
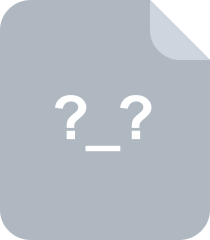
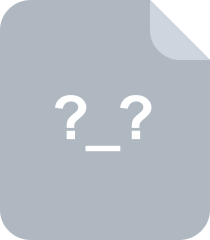
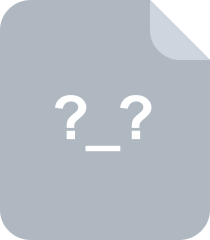
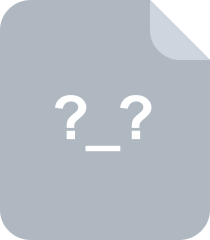
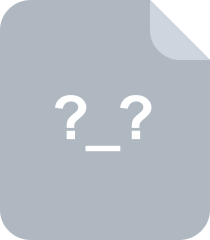
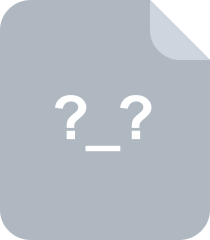
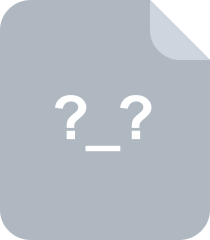
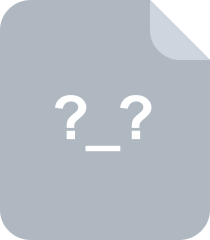
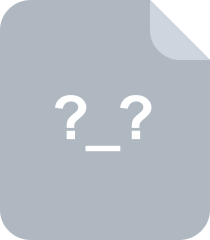
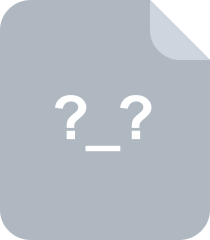
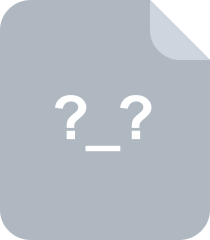
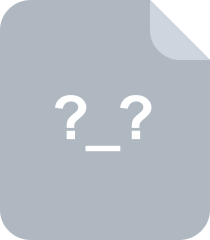
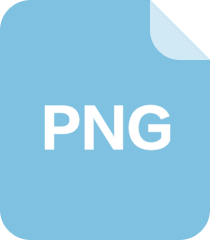
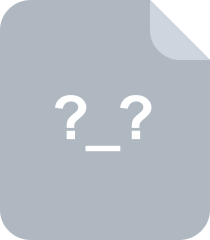
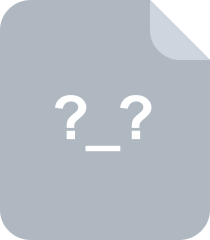
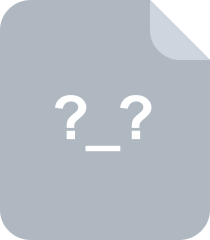
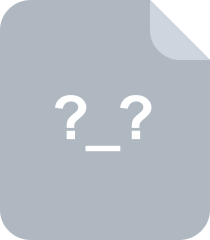
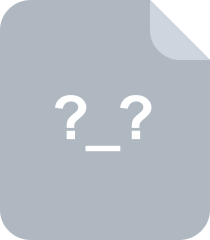
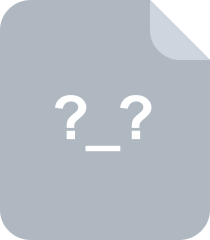
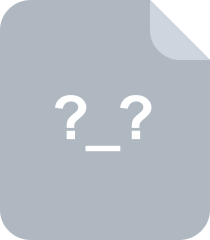
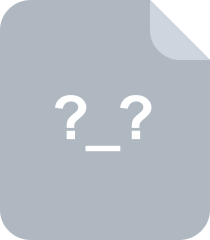
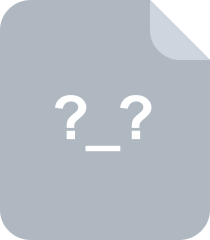
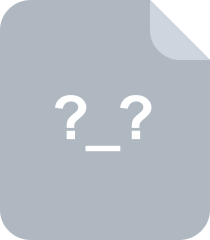
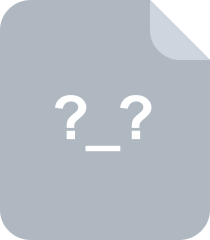
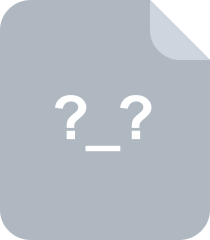
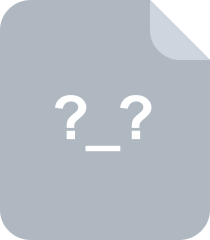
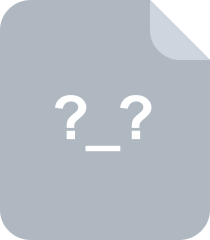
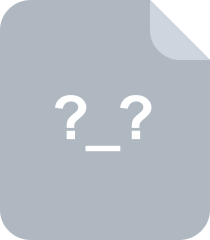
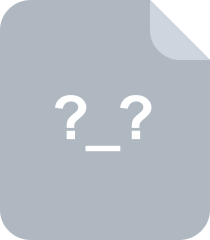
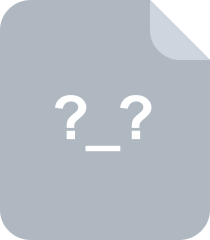
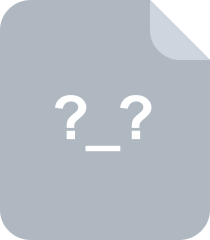
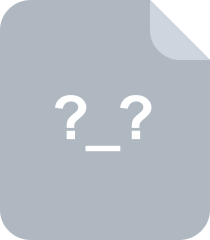
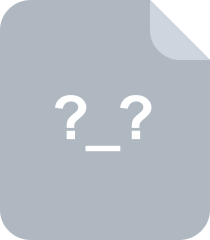
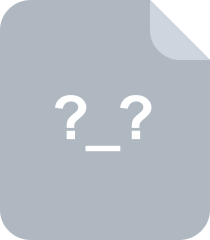
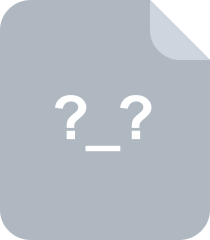
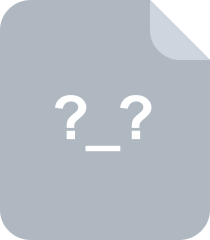
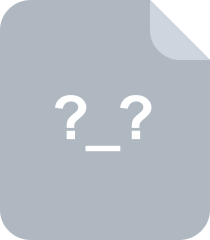
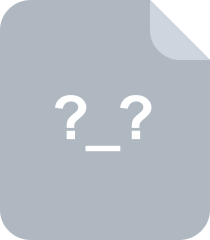
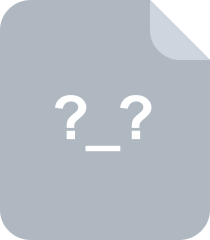
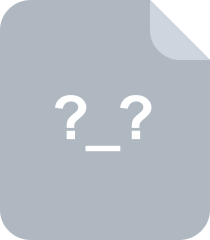
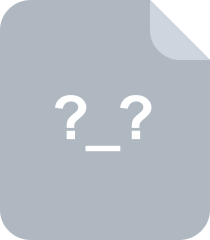
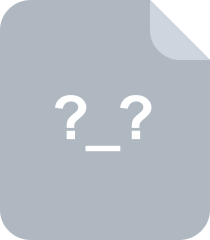
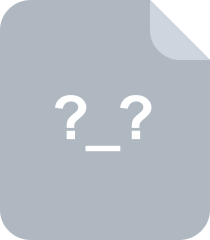
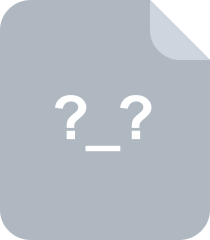
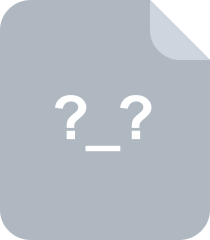
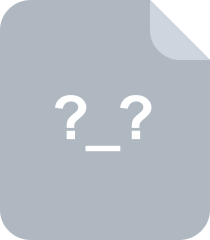
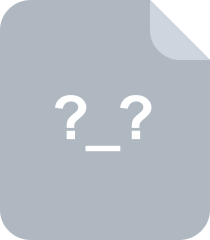
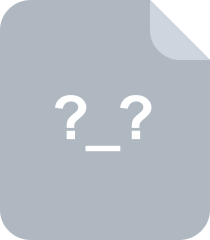
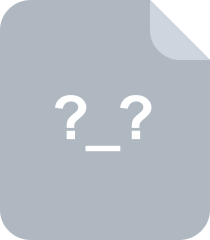
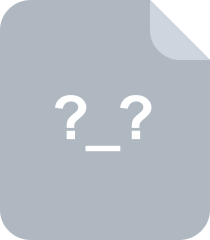
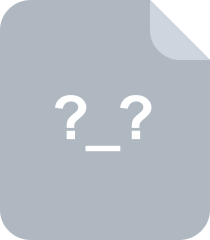
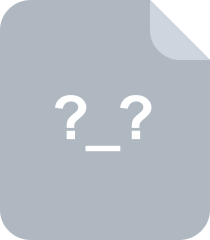
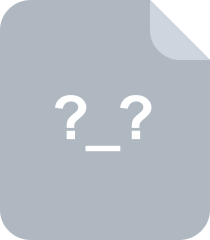
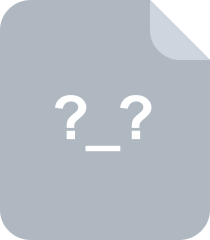
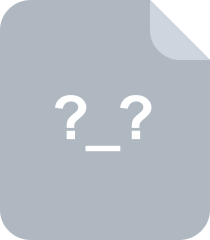
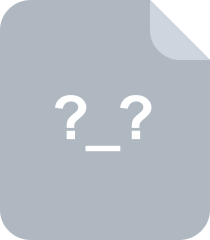
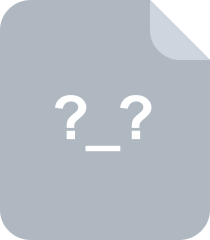
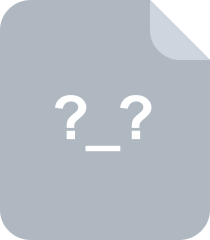
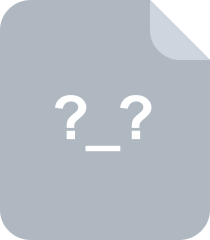
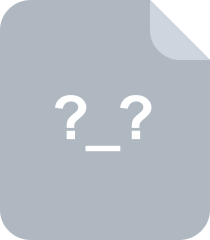
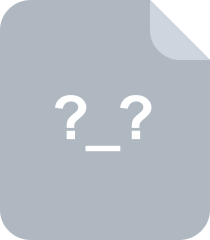
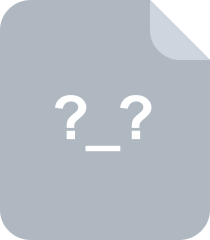
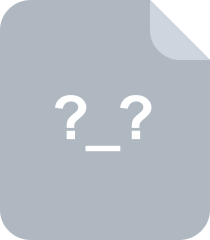
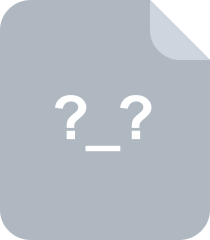
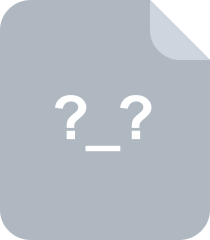
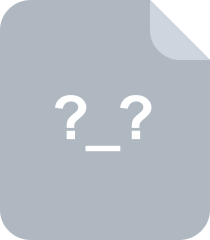
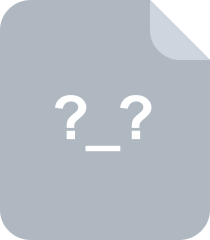
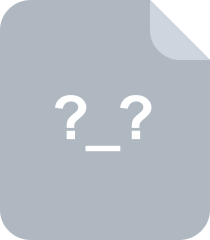
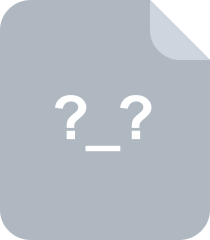
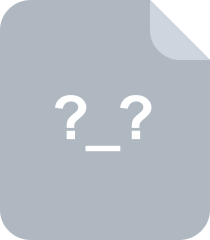
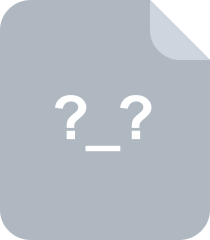
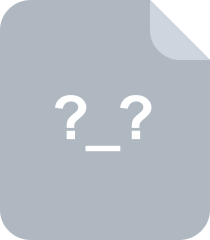
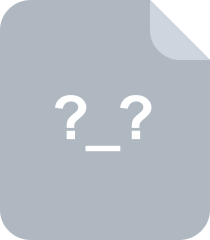
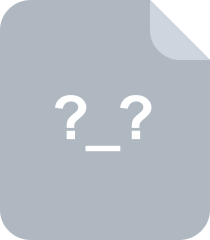
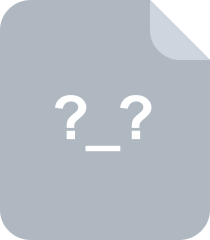
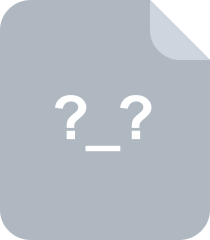
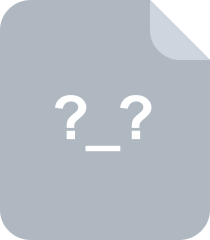
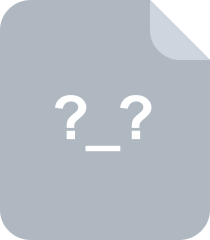
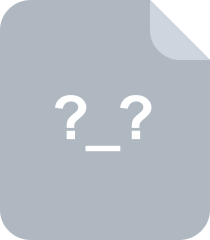
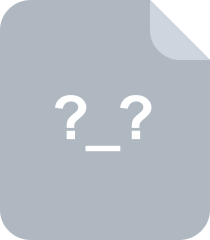
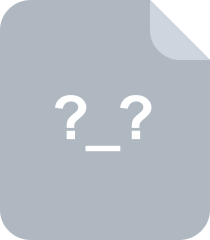
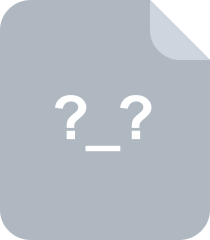
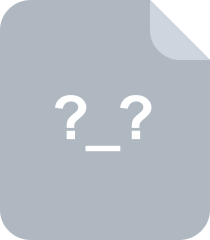
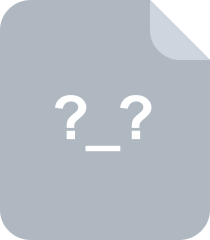
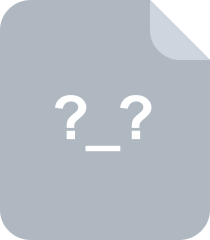
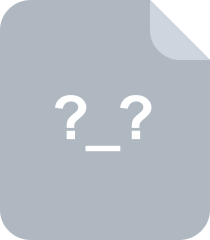
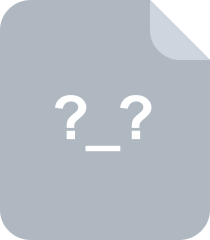
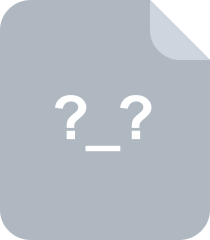
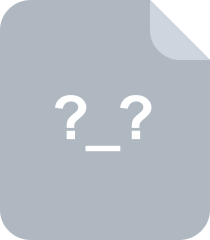
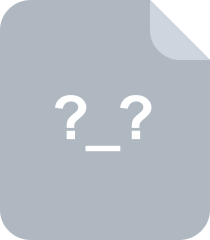
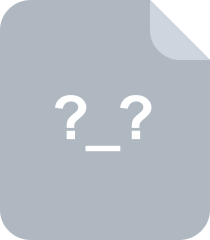
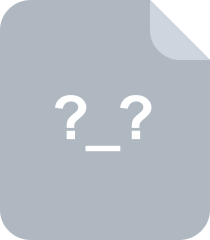
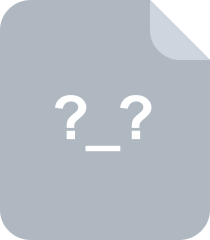
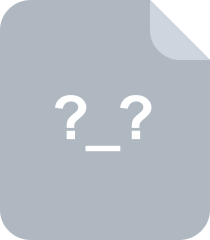
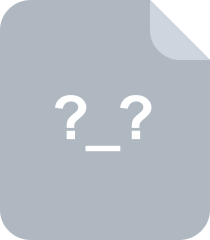
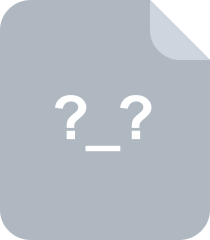
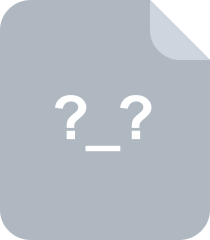
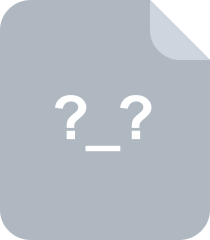
共 108 条
- 1
- 2
资源评论
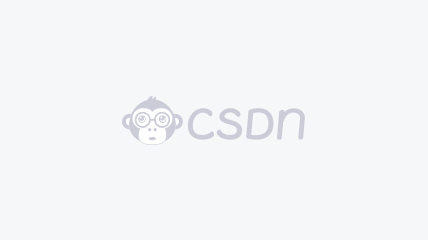
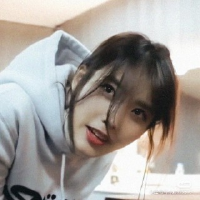
生瓜蛋子
- 粉丝: 3910
- 资源: 7441
上传资源 快速赚钱
我的内容管理 展开
我的资源 快来上传第一个资源
我的收益
登录查看自己的收益我的积分 登录查看自己的积分
我的C币 登录后查看C币余额
我的收藏
我的下载
下载帮助

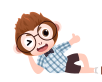
最新资源
资源上传下载、课程学习等过程中有任何疑问或建议,欢迎提出宝贵意见哦~我们会及时处理!
点击此处反馈


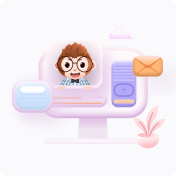
安全验证
文档复制为VIP权益,开通VIP直接复制
