# MXNet-im2rec_tutorial
**this simple tutorial will introduce how to use im2rec for mx.image.ImageIter and ImageDetIter and how to use im2rec for COCO DataSet**
ok let's start but prepare your im2rec first
https://github.com/apache/incubator-mxnet/blob/master/tools/im2rec.py
## im2rec ##
**step 1. you should make a .lst file**
run in terminal
```
python im2rec.py --list testlst Dataset
--list => make .lst file
you should set True when your folder didnt have any .lst file
test => name of your .lst name what you want
DataSet => your image dataset folder
check your folder path correct
```
**use .lst for imageIter**
```python
data_iter = mx.image.ImageIter(
batch_size=4,
data_shape=(3,816, 1232),
label_width=1,
path_imglist='test.lst',
path_root='DataSet')
for data in data_iter:
d = data.data[0]
break
img =nd.transpose(d,(0,2,3,1))
print(img.shape)
io.imshow(img[0].asnumpy().astype(np.uint8))
io.show()
```
**step 2. make .rec file**
```
python im2rec.py testrec Dataset
testrec => name of your .rec name what you want
Dataset => your image dataset folder
```
**use .rec for imageiter**
```python
train_iter = mx.image.ImageIter(
batch_size=32,
data_shape=(3, 816, 1232),
path_imgrec='data.rec',
path_imgidx='data.idx', #help shuffle performance
shuffle=True,
aug_list=[mx.image.HorizontalFlipAug(0.5)]
)
train_iter.reset()
for batch in train_iter:
x = batch.data[0]
break
img =nd.transpose(x,(0,2,3,1))
print(img.shape)
io.imshow(img[0].asnumpy().astype(np.uint8))
io.show()
```
**other feature**
```python
'--list'
If this is set im2rec will create image list(s) by traversing root folder
and output to .lst
Otherwise im2rec will read .lst and create a database at .rec
'--exts', default=['.jpeg', '.jpg', '.png']
list of acceptable image extensions.
'--train-ratio', default=1.0
Ratio of images to use for training.
'--test-ratio', default=0,
Ratio of images to use for testing.
'--no-shuffle', dest='shuffle', action='store_false',
If this is passed,im2rec will not randomize the image order in <prefix>.lst'
'--resize', type=int, default=0,
resize the shorter edge of image to the newsize, original images will
be packed by default'
'--center-crop',
specify whether to crop the center image to make it rectangular.
'--quality', default=95,
JPEG quality for encoding, 1-100; or PNG compression for encoding, 1-9
'--num-thread',default=1,
number of thread to use for encoding. order of images will be different
'--color', default=1, choices=[-1, 0, 1],
specify the color mode of the loaded image.
1: Loads a color image. Any transparency of image will be neglected.
It is the default flag
0: Loads image in grayscale mode.
-1:Loads image as such including alpha channel.
```
# ImageDetIter #
## make own .lst for detection ##
**A B [extra header] [(object0), (object1), ... (objectN)]**
1. you should write the .lst with your image information
Where A is the width of header (2 + length of extra header), B is the width of each object. Extra header is optional and used for inserting helper information such as (width, height). Each object is usually 5 or 6 numbers describing the object properties, for example: **[id, xmin, ymin, xmax, ymax, difficulty]** Putting all together, we have a `lst` file for object detection:
ref: https://mxnet.incubator.apache.org/api/python/image/image.html
```python
with open('dataset.lst', 'w+') as f:
for i in range(3):
f.write(
str(i) + '\t' +
# idx
str(4) + '\t' + str(5) + '\t' +
# width of header and width of each object.
str(256) + '\t' + str(256) + '\t' +
# (width, height)
str(1) + '\t' +
# class
str((i / 10)) + '\t' + str((i / 10)) + '\t' + str(((i + 3) / 10)) + '\t' +str(((i + 3) / 10)) + '\t' +
# xmin, ymin, xmax, ymax
str(i) + '.jpg\n'
)
```
you will see like this format in .lst
```
idx A B [extra header] [(object0), (object1), ... (objectN)]
0 4 5 256 256 1 0.1 0.1 0.3 0.3 0.jpg
1 4 5 256 256 1 1.1 1.1 1.3 1.3 1.jpg
2 4 5 256 256 1 2.1 2.1 2.3 2.3 2.jpg
```
**must **'\t'** between data information**
## make own dataset .rec for ImageDetIter ##
```
python im2rec.py --pack-label dataset.lst Dataset
--pack-label => when you have label
dataset.lst => name for .rec type for your self in .lst
Dataset => DataSet image folder
```
```python
import mxnet as mx
import mxnet.ndarray as nd
from skimage import io
import numpy as np
train_iter = mx.image.ImageDetIter(
batch_size=3,
data_shape=(3, 256, 256),
path_imgrec='dataset.rec',
path_imgidx='dataset.idx',
shuffle=True,
rand_crop=1,
min_object_covered=0.95,
max_attempts=200
) # you can aug your data in ImageDetIter
train_iter.reset()
for batch in train_iter:
x = batch.data[0]
y = batch.label[0]
break
print(y[0])
img =nd.transpose(x,(0,2,3,1))
print(img.shape)
io.imshow(img[0].asnumpy().astype(np.uint8))
io.show()
```
# How to use im2rec on COCO DataSet #
**1. download cococ dataset first!!!**
**2. make own .json or use COCO.json**
**3. .json => .lst**
**4. .lst => .rec**
**5. .rec for ImageDetIter**
## how to load COCO DataSet from .json ##
```python
import json
with open('annotations/instances_train2017.json', 'r') as f:
DataSets = json.load(f)
print(DataSets['annotations'][0])
>>{'segmentation': [[239.97, 260.24, 222.04, 270.49, 199.84, 253.41, 213.5, 227.79, 259.62, 200.46, 274.13, 202.17, 277.55, 210.71, 249.37, 253.41, 237.41, 264.51, 242.54, 261.95, 228.87, 271.34]],
'area': 2765.1486500000005,
'iscrowd': 0,
'image_id': 558840,
'bbox': [199.84, 200.46, 77.71, 70.88],
'category_id': 58, 'id': 156}
```
**as you see, these annotations about image 558840 , boundingbox,class.... in detail you can check coco website**
## COCO.json => own.json ##
**make own dataset (class:bird) from COCO dataset**
```python
import json
from mxnet import image
from skimage import io
import os
## load COCO annotations
with open('annotations/instances_train2017.json', 'r') as f:
DataSets = json.load(f)
print(DataSets['annotations'][0])
## save class and own dataset .json
jsonName = 'ownset.json'
directory = 'ownSet/'
data = {}
data['DataSet] = []
with open(jsonName, 'w') as outfile:
if not os.path.exists(directory):
os.makedirs(directory)
for DataSet in DataSets['annotations']:
box = DataSet['bbox']
default_name = "000000000000"
img_id = str(DataSet['image_id'])
img_name = default_name[:len(default_name) - len(img_id)] + str(img_id) + '.jpg'
coco_name = 'train2017/' + img_name
if DataSet['category_id'] == 16: #bird
with open(coco_name, 'rb') as f:
img = image.imdecode(f.read())
height = img.shape[0]
width = img.shape[1]
box[0] = box[0]/width #normalize
box[2] = box[2]/width
box[1] = box[1]/height
box[3] = box[3]/height
io.imsave(directory + img_name, img.asnumpy())
data['DataSet'].append({
'img_name': img_name,
'height': height,
'width': width,
'bbox': box,
'class':DataSet['category_id']
})
json.dump(data, outfile)
print('save ok')
with open(jsonName, 'r') as f:
Sets = json.load(f)
print(Sets['DataSet'][0])
```
>{'img_name': '000000202273.jpg', 'height': 640, 'width
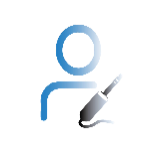
好家伙VCC
- 粉丝: 2827
- 资源: 9136
最新资源
- 基于Spring Boot和SSM框架的ERP进销存管理系统源码:单据流转、精细化的物流信息管理,前端细节设计,权限管理,透明背景与关联单据优化,基于Spring Boot与SSM框架的ERP进销存管
- 燃料电池与超级电容复合能量管理策略:Simulink仿真模型研究及其在混合储能系统中的应用,燃料电池与超级电容复合能量管理策略:Simulink仿真模型研究及其在混合储能系统中的应用,燃料电池电池超级
- 西门子PLC1500控制Fanuc机器人汽车焊装生产线自动化:Profinet通讯与多种模块集成应用,西门子PLC1500大型程序掌控汽车焊装生产线:涵盖Fanuc机器人、触摸屏、Profinet通讯
- 基于MATLAB的综合能源系统优化调度:结合需求响应与碳交易机制研究,综合能源系统优化调度:Matlab程序制定与碳交易机制下的综合需求响应应用,matlab程序制定,综合能源系统优化调度,综合需求响
- 电力市场出清程序:基于IEEE 14节点输电阻塞分析的机组与节点边际电价求解,利用拉格朗日乘子计算最优发电成本,采用MATLAB linprog函数实现,具备广泛参考价值 ,电力市场出清程序:基于IE
- 永磁同步电机双矢量模型预测控制仿真研究:MATLAB下的PMSM控制策略探索,永磁同步电机双矢量模型预测控制仿真研究:基于MATLAB平台的PMSM控制策略探索,永磁同步电机双矢量模型预测控制仿真PM
- 【毕业设计】Python的Django-html开放领域事件抽取系统源码(完整前后端+mysql+说明文档+LW+PPT).zip
- 【毕业设计】Python的Django-html旅游城市关键词分析系统源码(完整前后端+mysql+说明文档+LW+PPT).zip
- 【毕业设计】Python的Django-html旅游景点方面级别情感分析语料库与模型源码(完整前后端+mysql+说明文档+LW+PPT).zip
- LabVIEW利用DLL接口操作结构体指针:获取嵌套指针与混合类型数据处理策略,LabVIEW利用DLL获取结构体指针内嵌套指针元素及多种类型数据的实践方法,LabVIEW通过dll获取结构体指针中的
- 【毕业设计】Python的Django-html某大学学生影响力分析系统源码(完整前后端+mysql+说明文档+LW+PPT).zip
- 【毕业设计】Python的Django-html棉花数据平台建设与可视化系统源码(完整前后端+mysql+说明文档+LW+PPT).zip
- 【毕业设计】Python的Django-html小波变换的数字水印研究系统源码(完整前后端+mysql+说明文档+LW+PPT).zip
- 【毕业设计】Python的Django-html语音识别的智能垃圾分类系统源码(完整前后端+mysql+说明文档+LW+PPT).zip
- 基于蒙特卡洛模拟的分布式电源(风光)概率潮流Matlab仿真研究:IEEE 33节点系统的分析与应用(包括牛拉法潮流计算与电压特性前后对比),基于蒙特卡洛方法的分布式电源(风光)概率潮流计算Matla
- 【毕业设计】Python的Django-html知识图谱的百科知识问答平台源码(完整前后端+mysql+说明文档+LW+PPT).zip
资源上传下载、课程学习等过程中有任何疑问或建议,欢迎提出宝贵意见哦~我们会及时处理!
点击此处反馈


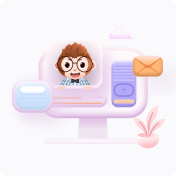