# Deep Learning Papers Reading Roadmap
>If you are a newcomer to the Deep Learning area, the first question you may have is "Which paper should I start reading from?"
>Here is a reading roadmap of Deep Learning papers!
The roadmap is constructed in accordance with the following four guidelines:
- From outline to detail
- From old to state-of-the-art
- from generic to specific areas
- focus on state-of-the-art
You will find many papers that are quite new but really worth reading.
I would continue adding papers to this roadmap.
---------------------------------------
# 1 Deep Learning History and Basics
## 1.0 Book
**[0]** Bengio, Yoshua, Ian J. Goodfellow, and Aaron Courville. "**Deep learning**." An MIT Press book. (2015). [[html]](http://www.deeplearningbook.org/) **(Deep Learning Bible, you can read this book while reading following papers.)** :star::star::star::star::star:
## 1.1 Survey
**[1]** LeCun, Yann, Yoshua Bengio, and Geoffrey Hinton. "**Deep learning**." Nature 521.7553 (2015): 436-444. [[pdf]](http://www.cs.toronto.edu/~hinton/absps/NatureDeepReview.pdf) **(Three Giants' Survey)** :star::star::star::star::star:
## 1.2 Deep Belief Network(DBN)(Milestone of Deep Learning Eve)
**[2]** Hinton, Geoffrey E., Simon Osindero, and Yee-Whye Teh. "**A fast learning algorithm for deep belief nets**." Neural computation 18.7 (2006): 1527-1554. [[pdf]](http://www.cs.toronto.edu/~hinton/absps/ncfast.pdf)**(Deep Learning Eve)** :star::star::star:
**[3]** Hinton, Geoffrey E., and Ruslan R. Salakhutdinov. "**Reducing the dimensionality of data with neural networks**." Science 313.5786 (2006): 504-507. [[pdf]](http://www.cs.toronto.edu/~hinton/science.pdf) **(Milestone, Show the promise of deep learning)** :star::star::star:
## 1.3 ImageNet Evolution(Deep Learning broke out from here)
**[4]** Krizhevsky, Alex, Ilya Sutskever, and Geoffrey E. Hinton. "**Imagenet classification with deep convolutional neural networks**." Advances in neural information processing systems. 2012. [[pdf]](http://papers.nips.cc/paper/4824-imagenet-classification-with-deep-convolutional-neural-networks.pdf) **(AlexNet, Deep Learning Breakthrough)** :star::star::star::star::star:
**[5]** Simonyan, Karen, and Andrew Zisserman. "**Very deep convolutional networks for large-scale image recognition**." arXiv preprint arXiv:1409.1556 (2014). [[pdf]](https://arxiv.org/pdf/1409.1556.pdf) **(VGGNet,Neural Networks become very deep!)** :star::star::star:
**[6]** Szegedy, Christian, et al. "**Going deeper with convolutions**." Proceedings of the IEEE Conference on Computer Vision and Pattern Recognition. 2015. [[pdf]](http://www.cv-foundation.org/openaccess/content_cvpr_2015/papers/Szegedy_Going_Deeper_With_2015_CVPR_paper.pdf) **(GoogLeNet)** :star::star::star:
**[7]** He, Kaiming, et al. "**Deep residual learning for image recognition**." arXiv preprint arXiv:1512.03385 (2015). [[pdf]](https://arxiv.org/pdf/1512.03385.pdf) **(ResNet,Very very deep networks, CVPR best paper)** :star::star::star::star::star:
## 1.4 Speech Recognition Evolution
**[8]** Hinton, Geoffrey, et al. "**Deep neural networks for acoustic modeling in speech recognition: The shared views of four research groups**." IEEE Signal Processing Magazine 29.6 (2012): 82-97. [[pdf]](http://cs224d.stanford.edu/papers/maas_paper.pdf) **(Breakthrough in speech recognition)**:star::star::star::star:
**[9]** Graves, Alex, Abdel-rahman Mohamed, and Geoffrey Hinton. "**Speech recognition with deep recurrent neural networks**." 2013 IEEE international conference on acoustics, speech and signal processing. IEEE, 2013. [[pdf]](http://arxiv.org/pdf/1303.5778.pdf) **(RNN)**:star::star::star:
**[10]** Graves, Alex, and Navdeep Jaitly. "**Towards End-To-End Speech Recognition with Recurrent Neural Networks**." ICML. Vol. 14. 2014. [[pdf]](http://www.jmlr.org/proceedings/papers/v32/graves14.pdf):star::star::star:
**[11]** Sak, Haşim, et al. "**Fast and accurate recurrent neural network acoustic models for speech recognition**." arXiv preprint arXiv:1507.06947 (2015). [[pdf]](http://arxiv.org/pdf/1507.06947) **(Google Speech Recognition System)** :star::star::star:
**[12]** Amodei, Dario, et al. "**Deep speech 2: End-to-end speech recognition in english and mandarin**." arXiv preprint arXiv:1512.02595 (2015). [[pdf]](https://arxiv.org/pdf/1512.02595.pdf) **(Baidu Speech Recognition System)** :star::star::star::star:
**[13]** W. Xiong, J. Droppo, X. Huang, F. Seide, M. Seltzer, A. Stolcke, D. Yu, G. Zweig "**Achieving Human Parity in Conversational Speech Recognition**." arXiv preprint arXiv:1610.05256 (2016). [[pdf]](https://arxiv.org/pdf/1610.05256v1) **(State-of-the-art in speech recognition, Microsoft)** :star::star::star::star:
>After reading above papers, you will have a basic understanding of the Deep Learning history, the basic architectures of Deep Learning model(including CNN, RNN, LSTM) and how deep learning can be applied to image and speech recognition issues. The following papers will take you in-depth understanding of the Deep Learning method, Deep Learning in different areas of application and the frontiers. I suggest that you can choose the following papers based on your interests and research direction.
#2 Deep Learning Method
## 2.1 Model
**[14]** Hinton, Geoffrey E., et al. "**Improving neural networks by preventing co-adaptation of feature detectors**." arXiv preprint arXiv:1207.0580 (2012). [[pdf]](https://arxiv.org/pdf/1207.0580.pdf) **(Dropout)** :star::star::star:
**[15]** Srivastava, Nitish, et al. "**Dropout: a simple way to prevent neural networks from overfitting**." Journal of Machine Learning Research 15.1 (2014): 1929-1958. [[pdf]](https://www.cs.toronto.edu/~hinton/absps/JMLRdropout.pdf) :star::star::star:
**[16]** Ioffe, Sergey, and Christian Szegedy. "**Batch normalization: Accelerating deep network training by reducing internal covariate shift**." arXiv preprint arXiv:1502.03167 (2015). [[pdf]](http://arxiv.org/pdf/1502.03167) **(An outstanding Work in 2015)** :star::star::star::star:
**[17]** Ba, Jimmy Lei, Jamie Ryan Kiros, and Geoffrey E. Hinton. "**Layer normalization**." arXiv preprint arXiv:1607.06450 (2016). [[pdf]](https://arxiv.org/pdf/1607.06450.pdf?utm_source=sciontist.com&utm_medium=refer&utm_campaign=promote) **(Update of Batch Normalization)** :star::star::star::star:
**[18]** Courbariaux, Matthieu, et al. "**Binarized Neural Networks: Training Neural Networks with Weights and Activations Constrained to+ 1 or−1**." [[pdf]](https://pdfs.semanticscholar.org/f832/b16cb367802609d91d400085eb87d630212a.pdf) **(New Model,Fast)** :star::star::star:
**[19]** Jaderberg, Max, et al. "**Decoupled neural interfaces using synthetic gradients**." arXiv preprint arXiv:1608.05343 (2016). [[pdf]](https://arxiv.org/pdf/1608.05343) **(Innovation of Training Method,Amazing Work)** :star::star::star::star::star:
**[20]** Chen, Tianqi, Ian Goodfellow, and Jonathon Shlens. "Net2net: Accelerating learning via knowledge transfer." arXiv preprint arXiv:1511.05641 (2015). [[pdf]](https://arxiv.org/abs/1511.05641) **(Modify previously trained network to reduce training epochs)** :star::star::star:
**[21]** Wei, Tao, et al. "Network Morphism." arXiv preprint arXiv:1603.01670 (2016). [[pdf]](https://arxiv.org/abs/1603.01670) **(Modify previously trained network to reduce training epochs)** :star::star::star:
## 2.2 Optimization
**[22]** Sutskever, Ilya, et al. "**On the importance of initialization and momentum in deep learning**." ICML (3) 28 (2013): 1139-1147. [[pdf]](http://www.jmlr.org/proceedings/papers/v28/sutskever13.pdf) **(Momentum optimizer)** :star::star:
**[23]** Kingma, Diederik, and Jimmy Ba. "**Adam: A method for stochastic optimization**." arXiv preprint arXiv:1412.6980 (2014). [[pdf]](http://arxiv.org/pdf/1412.6980) **(Maybe used most often currently)** :star::star::star:
**[24]** Andrychowicz, Marcin, et al. "**Learning to learn by gradient descent by gradi
没有合适的资源?快使用搜索试试~ 我知道了~
资源推荐
资源详情
资源评论
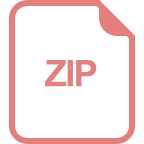
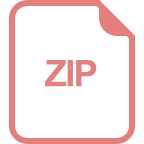
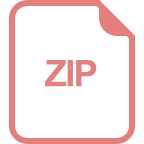
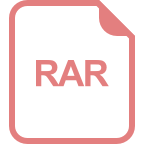
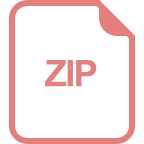
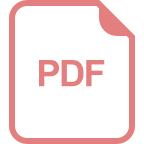
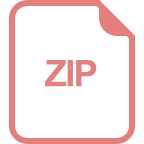
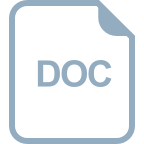
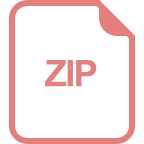
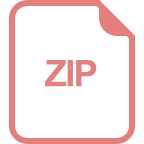
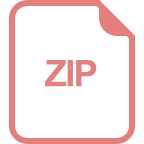
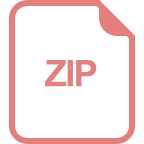
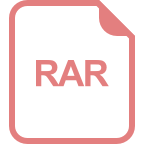
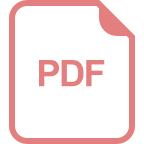
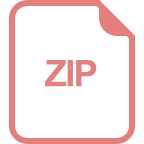
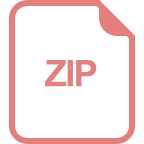
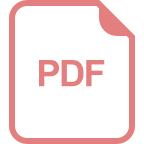
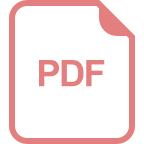
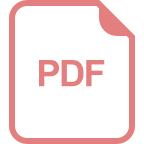
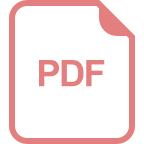
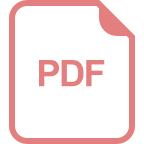
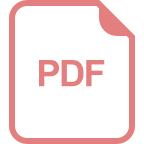
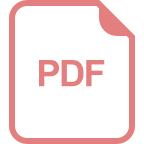
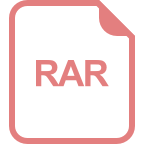
收起资源包目录


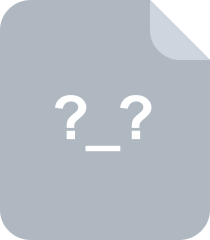
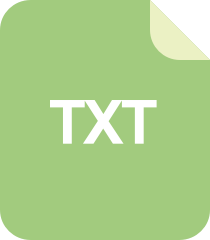
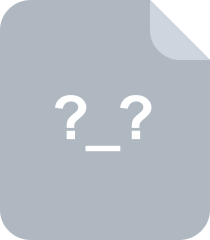
共 3 条
- 1
资源评论
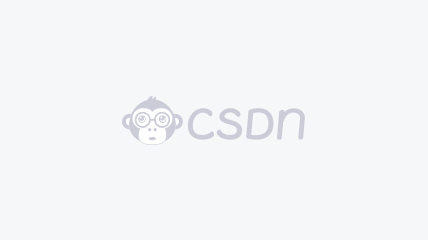
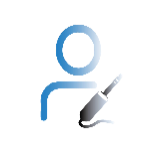
好家伙VCC
- 粉丝: 1910
- 资源: 9086
上传资源 快速赚钱
我的内容管理 展开
我的资源 快来上传第一个资源
我的收益
登录查看自己的收益我的积分 登录查看自己的积分
我的C币 登录后查看C币余额
我的收藏
我的下载
下载帮助

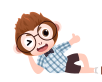
最新资源
- PyOpenGL-3.1.8-cp313-cp313-win32.whl
- PyOpenGL-3.1.8-cp313-cp313-win-amd64.whl
- PyOpenGL-3.1.8-cp312-cp312-win32.whl
- PyOpenGL-3.1.8-cp312-cp312-win-arm64.whl
- PyOpenGL-3.1.8-cp312-cp312-win-amd64.whl
- PyOpenGL-3.1.8-cp311-cp311-win32.whl
- PyOpenGL-3.1.8-cp311-cp311-win-arm64.whl
- PyOpenGL-3.1.8-cp311-cp311-win-amd64.whl
- PyOpenGL-3.1.8-cp310-cp310-win32.whl
- 湿度温度传感器HDC1080的Verilog仿真模块实现与测试
资源上传下载、课程学习等过程中有任何疑问或建议,欢迎提出宝贵意见哦~我们会及时处理!
点击此处反馈


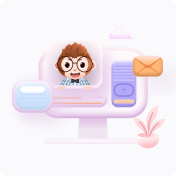
安全验证
文档复制为VIP权益,开通VIP直接复制
