[train hyper-parameters: Namespace(batch_size=16, lr=0.001, lrf=0.01)]
[epoch: 1]
train loss:0.0707 test accuracy:0.8121
[epoch: 2]
train loss:0.0287 test accuracy:0.8879
[epoch: 3]
train loss:0.0141 test accuracy:0.9000
[epoch: 4]
train loss:0.0097 test accuracy:0.9242
[epoch: 5]
train loss:0.0061 test accuracy:0.9303
[epoch: 6]
train loss:0.0065 test accuracy:0.9424
[epoch: 7]
train loss:0.0054 test accuracy:0.9485
[epoch: 8]
train loss:0.0029 test accuracy:0.9182
[epoch: 9]
train loss:0.0019 test accuracy:0.9182
[epoch: 10]
train loss:0.0032 test accuracy:0.9394
[epoch: 11]
train loss:0.0044 test accuracy:0.9515
[epoch: 12]
train loss:0.0020 test accuracy:0.9303
[epoch: 13]
train loss:0.0010 test accuracy:0.9545
[epoch: 14]
train loss:0.0007 test accuracy:0.9636
[epoch: 15]
train loss:0.0004 test accuracy:0.9667
[epoch: 16]
train loss:0.0002 test accuracy:0.9667
[epoch: 17]
train loss:0.0001 test accuracy:0.9667
[epoch: 18]
train loss:0.0006 test accuracy:0.9515
[epoch: 19]
train loss:0.0008 test accuracy:0.9606
[epoch: 20]
train loss:0.0004 test accuracy:0.9606
[epoch: 21]
train loss:0.0003 test accuracy:0.9636
[epoch: 22]
train loss:0.0002 test accuracy:0.9606
[epoch: 23]
train loss:0.0001 test accuracy:0.9667
[epoch: 24]
train loss:0.0001 test accuracy:0.9667
[epoch: 25]
train loss:0.0001 test accuracy:0.9667
[epoch: 26]
train loss:0.0001 test accuracy:0.9667
[epoch: 27]
train loss:0.0000 test accuracy:0.9636
[epoch: 28]
train loss:0.0000 test accuracy:0.9636
[epoch: 29]
train loss:0.0000 test accuracy:0.9636
[epoch: 30]
train loss:0.0000 test accuracy:0.9636
[epoch: 31]
train loss:0.0000 test accuracy:0.9636
[epoch: 32]
train loss:0.0000 test accuracy:0.9606
[epoch: 33]
train loss:0.0000 test accuracy:0.9636
[epoch: 34]
train loss:0.0000 test accuracy:0.9667
[epoch: 35]
train loss:0.0000 test accuracy:0.9667
[epoch: 36]
train loss:0.0000 test accuracy:0.9667
[epoch: 37]
train loss:0.0000 test accuracy:0.9636
[epoch: 38]
train loss:0.0000 test accuracy:0.9636
[epoch: 39]
train loss:0.0000 test accuracy:0.9636
[epoch: 40]
train loss:0.0000 test accuracy:0.9636
[epoch: 41]
train loss:0.0000 test accuracy:0.9636
[epoch: 42]
train loss:0.0000 test accuracy:0.9636
[epoch: 43]
train loss:0.0000 test accuracy:0.9636
[epoch: 44]
train loss:0.0000 test accuracy:0.9636
[epoch: 45]
train loss:0.0000 test accuracy:0.9636
[epoch: 46]
train loss:0.0000 test accuracy:0.9636
[epoch: 47]
train loss:0.0000 test accuracy:0.9636
[epoch: 48]
train loss:0.0000 test accuracy:0.9636
[epoch: 49]
train loss:0.0000 test accuracy:0.9636
[epoch: 50]
train loss:0.0000 test accuracy:0.9636
没有合适的资源?快使用搜索试试~ 我知道了~
温馨提示
本项目基于VGG16迁移学习的图像分类,可直接运行。 数据集采用其他垃圾六分类数据集(一次性快餐盒、污损塑料、烟蒂、牙签、破碎花盆和碗碟、竹筷),包括990张训练图片和330张预测图片。 网络训练的时候采用cos 学习率自动衰减,训练了50个epoch。模型在测试集最好的表现达到96.5%精度。 如果想要训练自己的数据集,请查看README文件
资源推荐
资源详情
资源评论
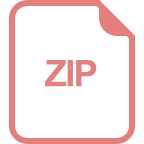
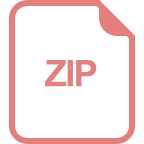
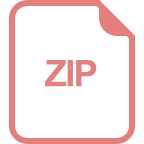
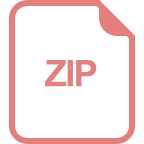
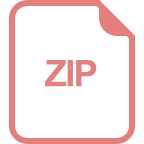
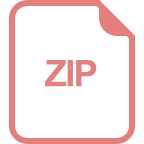
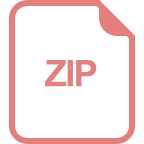
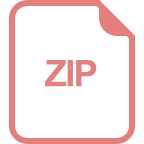
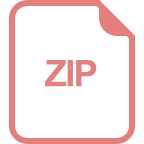
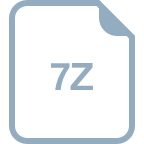
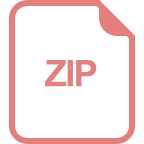
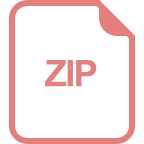
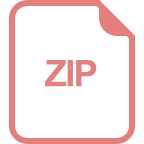
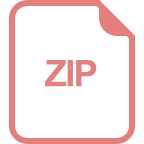
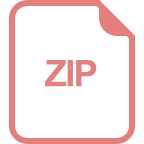
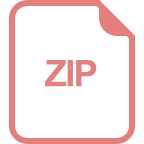
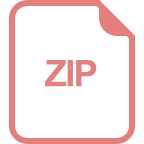
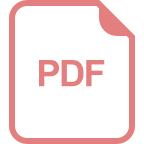
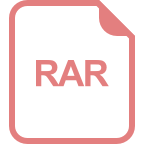
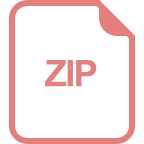
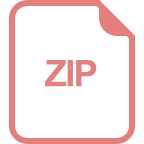
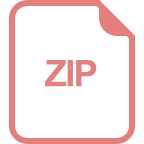
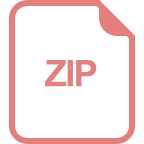
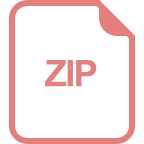
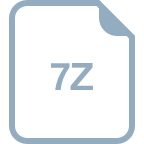
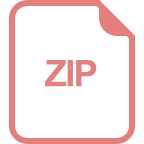
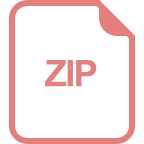
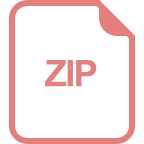
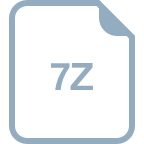
收起资源包目录

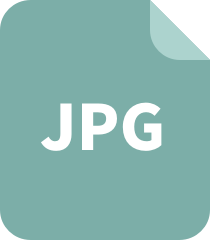
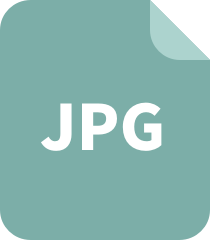
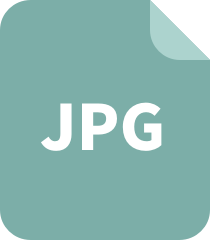
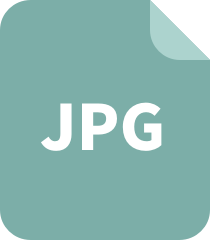
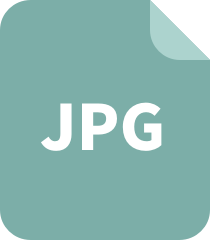
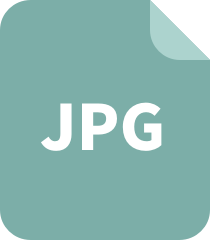
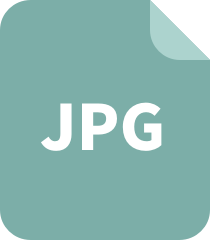
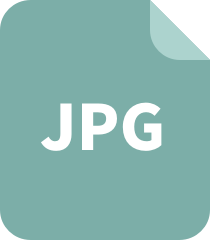
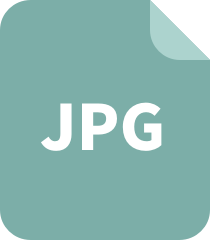
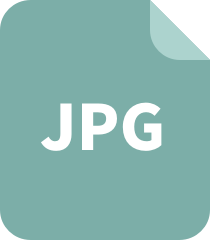
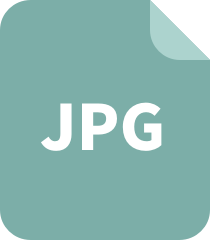
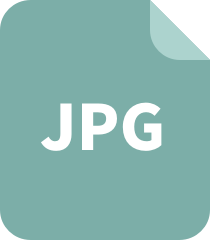
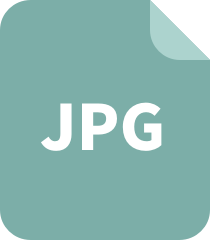
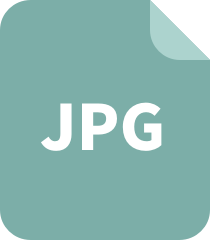
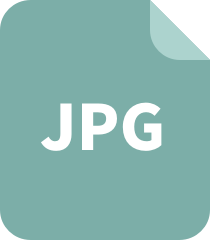
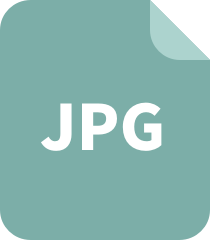
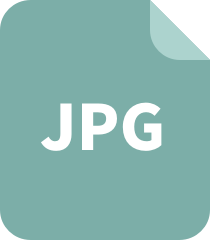
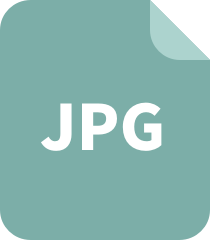
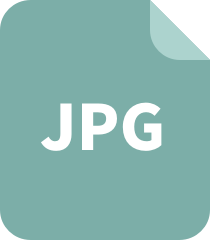
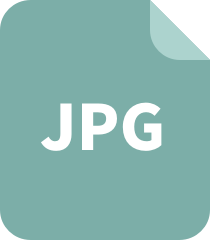
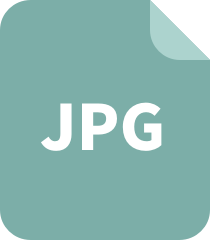
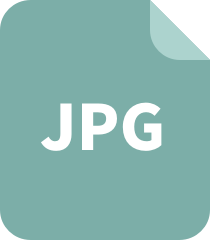
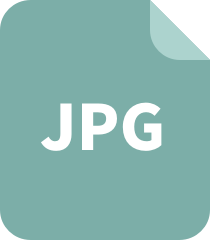
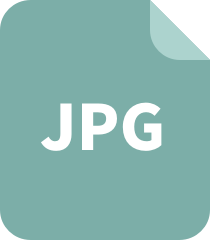
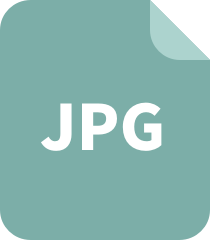
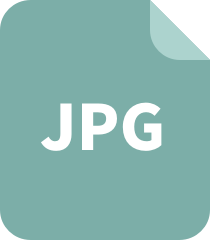
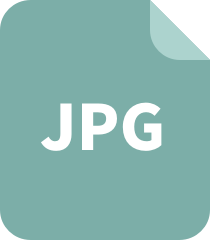
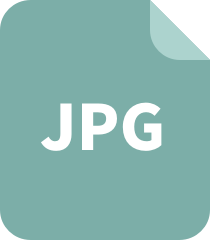
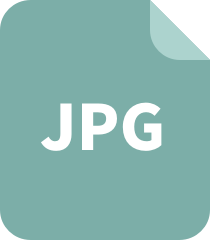
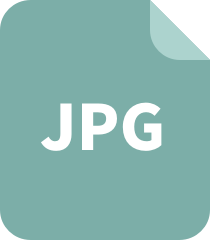
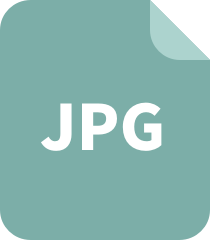
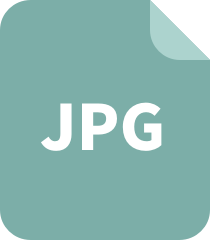
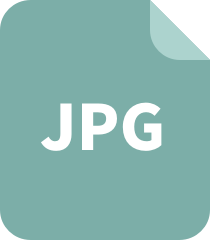
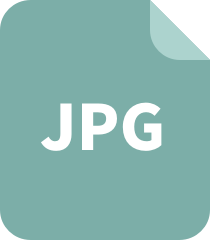
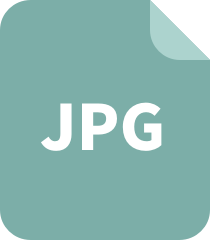
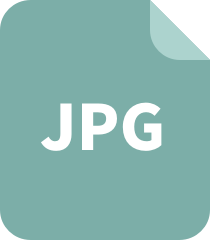
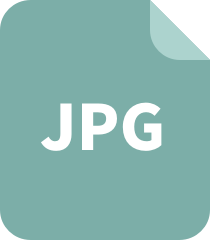
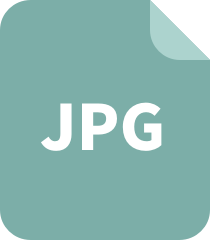
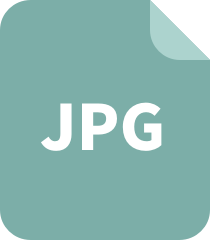
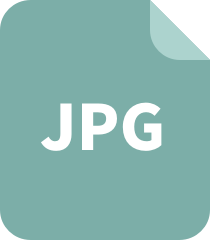
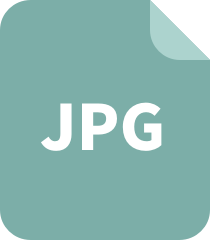
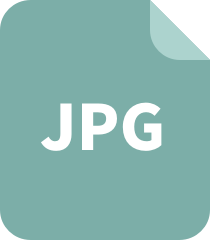
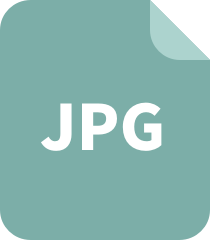
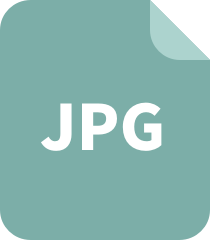
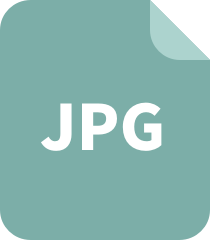
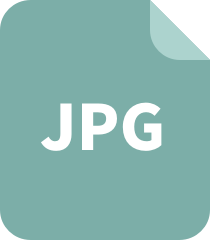
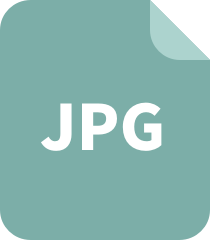
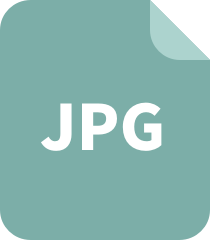
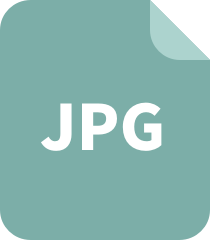
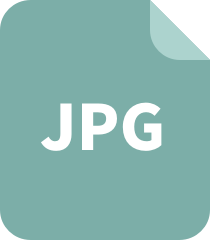
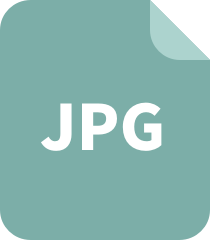
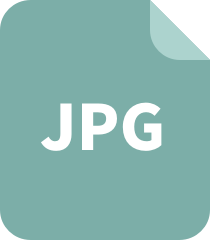
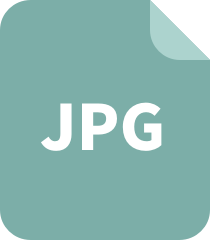
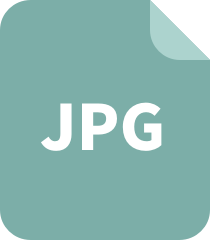
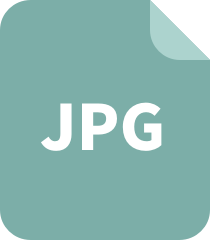
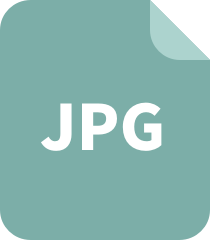
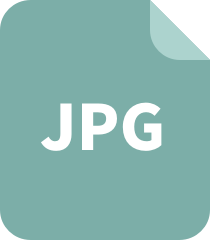
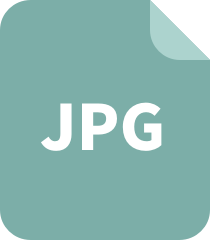
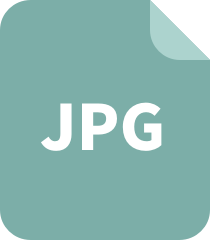
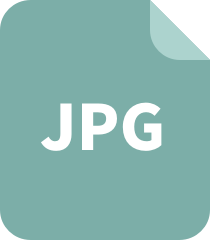
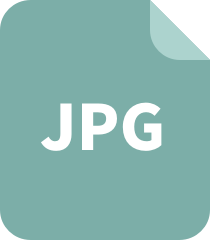
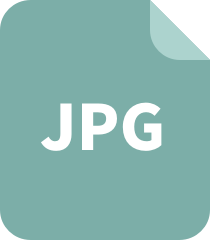
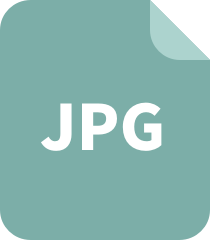
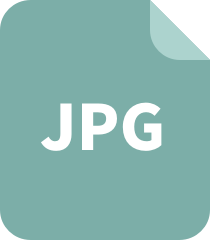
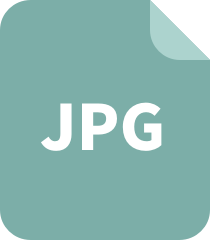
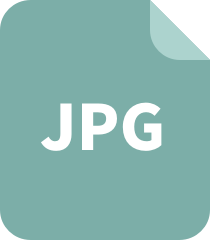
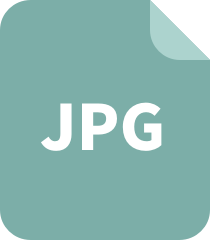
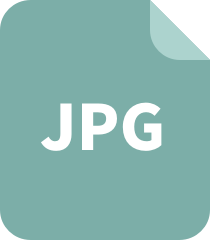
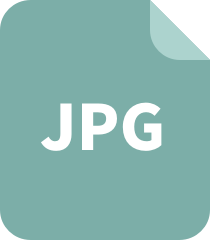
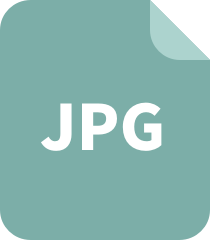
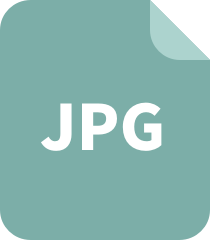
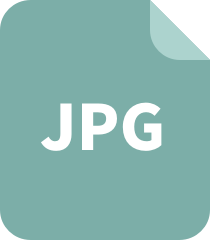
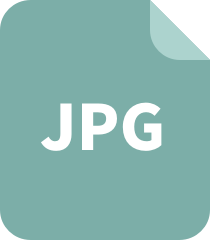
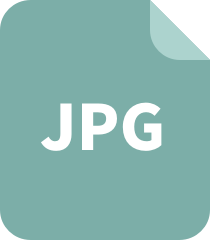
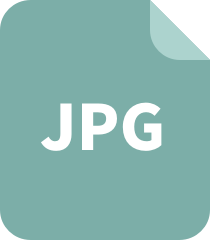
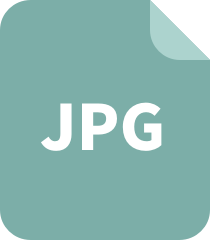
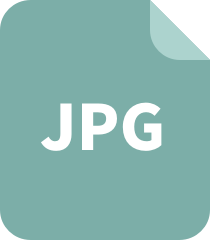
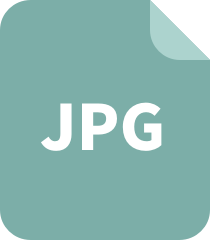
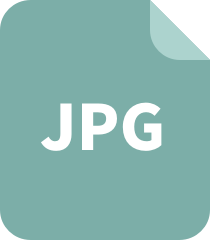
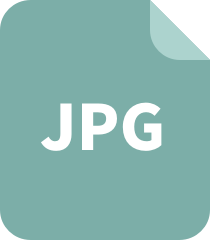
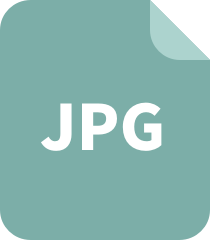
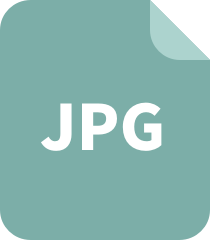
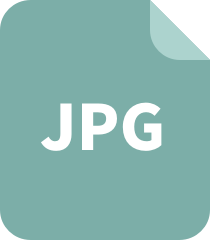
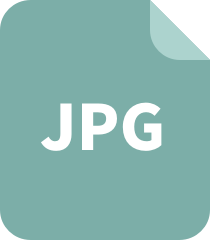
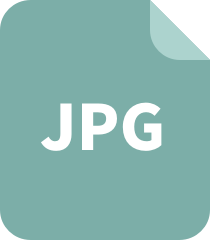
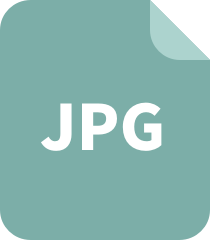
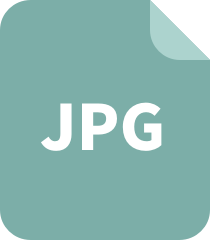
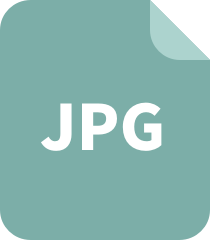
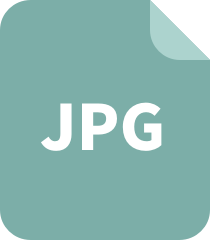
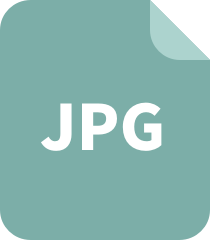
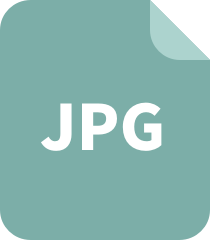
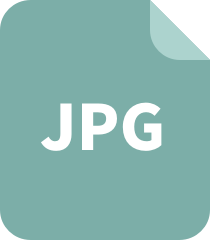
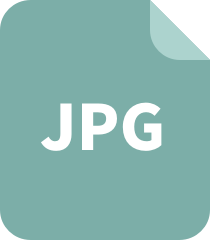
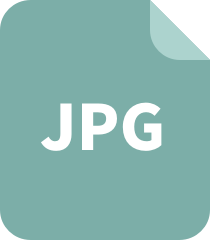
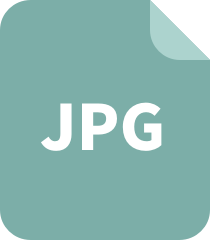
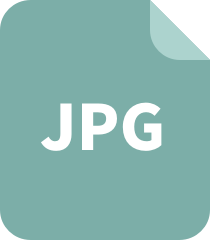
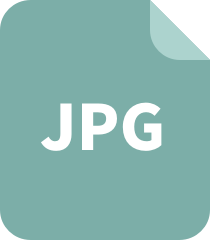
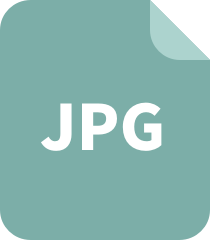
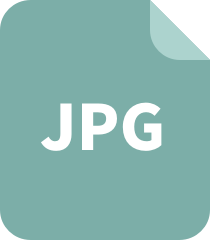
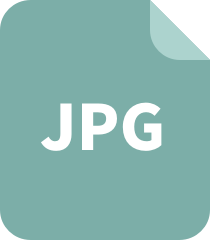
共 1342 条
- 1
- 2
- 3
- 4
- 5
- 6
- 14
资源评论
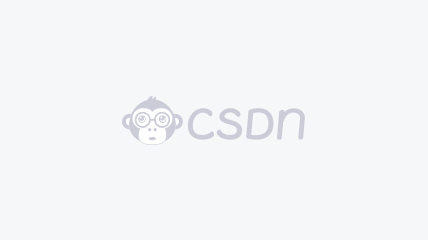

Ai医学图像分割
- 粉丝: 2w+
- 资源: 2128

下载权益

C知道特权

VIP文章

课程特权

开通VIP
上传资源 快速赚钱
我的内容管理 展开
我的资源 快来上传第一个资源
我的收益
登录查看自己的收益我的积分 登录查看自己的积分
我的C币 登录后查看C币余额
我的收藏
我的下载
下载帮助

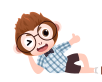
最新资源
- GitBook 教授 Javascript 编程基础知识.zip
- Generation.org 开发的 JAVA 模块练习.zip
- FastDFS Java 客户端 SDK.zip
- etcd java 客户端.zip
- Esercizi di informatica!执行计划,metti alla prova!.zip
- Eloquent JavaScript 翻译 - 2ª edição .zip
- Eclipse Paho Java MQTT 客户端库 Paho 是一个 Eclipse IoT 项目 .zip
- disconf 的 Java 应用程序.zip
- cloud.google.com 上使用的 Java 和 Kotlin 代码示例.zip
- 未命名3(3).cpp
资源上传下载、课程学习等过程中有任何疑问或建议,欢迎提出宝贵意见哦~我们会及时处理!
点击此处反馈


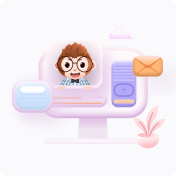
安全验证
文档复制为VIP权益,开通VIP直接复制
