# TensorFlow-Slim image classification model library
This directory contains code for training and evaluating several
widely used Convolutional Neural Network (CNN) image classification
models using
[tf_slim](https://github.com/google-research/tf-slim/tree/master/tf_slim).
It contains scripts that allow
you to train models from scratch or fine-tune them from pre-trained network
weights. It also contains code for downloading standard image datasets,
converting them
to TensorFlow's native TFRecord format and reading them in using TF-Slim's
data reading and queueing utilities. You can easily train any model on any of
these datasets, as we demonstrate below. We've also included a
[jupyter notebook](https://github.com/tensorflow/models/blob/master/research/slim/slim_walkthrough.ipynb),
which provides working examples of how to use TF-Slim for image classification.
For developing or modifying your own models, see also the [main TF-Slim page](https://github.com/google-research/tf-slim/tree/master/tf_slim).
## Contacts
Maintainers of TF-slim:
* Sergio Guadarrama, github: [sguada](https://github.com/sguada)
## Citation
"TensorFlow-Slim image classification model library"
N. Silberman and S. Guadarrama, 2016.
https://github.com/tensorflow/models/tree/master/research/slim
## Table of contents
<a href="#Install">Installation and setup</a><br>
<a href='#Data'>Preparing the datasets</a><br>
<a href='#Pretrained'>Using pre-trained models</a><br>
<a href='#Training'>Training from scratch</a><br>
<a href='#Tuning'>Fine tuning to a new task</a><br>
<a href='#Eval'>Evaluating performance</a><br>
<a href='#Export'>Exporting Inference Graph</a><br>
<a href='#Troubleshooting'>Troubleshooting</a><br>
# Installation
<a id='Install'></a>
In this section, we describe the steps required to install the appropriate
prerequisite packages.
## Installing latest version of TF-slim
TF-Slim is available as `tf_slim` package. To test that your
installation is working, execute the following command; it should run without
raising any errors.
```
python -c "import tf_slim as slim; eval = slim.evaluation.evaluate_once"
```
## Installing the TF-slim image models library
To use TF-Slim for image classification, you also have to install
the [TF-Slim image models library](https://github.com/tensorflow/models/tree/master/research/slim),
which is not part of the core TF library.
To do this, check out the
[tensorflow/models](https://github.com/tensorflow/models/) repository as follows:
```bash
cd $HOME/workspace
git clone https://github.com/tensorflow/models/
```
This will put the TF-Slim image models library in `$HOME/workspace/models/research/slim`.
(It will also create a directory called
[models/inception](https://github.com/tensorflow/models/tree/master/research/inception),
which contains an older version of slim; you can safely ignore this.)
To verify that this has worked, execute the following commands; it should run
without raising any errors.
```
cd $HOME/workspace/models/research/slim
python -c "from nets import cifarnet; mynet = cifarnet.cifarnet"
```
# Preparing the datasets
<a id='Data'></a>
As part of this library, we've included scripts to download several popular
image datasets (listed below) and convert them to slim format.
Dataset | Training Set Size | Testing Set Size | Number of Classes | Comments
:------:|:---------------:|:---------------------:|:-----------:|:-----------:
Flowers|2500 | 2500 | 5 | Various sizes (source: Flickr)
[Cifar10](https://www.cs.toronto.edu/~kriz/cifar.html) | 60k| 10k | 10 |32x32 color
[MNIST](http://yann.lecun.com/exdb/mnist/)| 60k | 10k | 10 | 28x28 gray
[ImageNet](http://www.image-net.org/challenges/LSVRC/2012/)|1.2M| 50k | 1000 | Various sizes
VisualWakeWords|82783 | 40504 | 2 | Various sizes (source: MS COCO)
## Downloading and converting to TFRecord format
For each dataset, we'll need to download the raw data and convert it to
TensorFlow's native
[TFRecord](https://www.tensorflow.org/versions/r0.10/api_docs/python/python_io.html#tfrecords-format-details)
format. Each TFRecord contains a
[TF-Example](https://github.com/tensorflow/tensorflow/blob/r0.10/tensorflow/core/example/example.proto)
protocol buffer. Below we demonstrate how to do this for the Flowers dataset.
```shell
$ DATA_DIR=/tmp/data/flowers
$ python download_and_convert_data.py \
--dataset_name=flowers \
--dataset_dir="${DATA_DIR}"
```
When the script finishes you will find several TFRecord files created:
```shell
$ ls ${DATA_DIR}
flowers_train-00000-of-00005.tfrecord
...
flowers_train-00004-of-00005.tfrecord
flowers_validation-00000-of-00005.tfrecord
...
flowers_validation-00004-of-00005.tfrecord
labels.txt
```
These represent the training and validation data, sharded over 5 files each.
You will also find the `$DATA_DIR/labels.txt` file which contains the mapping
from integer labels to class names.
You can use the same script to create the mnist, cifar10 and visualwakewords
datasets. However, for ImageNet, you have to follow the instructions
[here](https://github.com/tensorflow/models/blob/master/research/inception/README.md#getting-started).
Note that you first have to sign up for an account at image-net.org. Also, the
download can take several hours, and could use up to 500GB.
## Creating a TF-Slim Dataset Descriptor.
Once the TFRecord files have been created, you can easily define a Slim
[Dataset](https://github.com/google-research/tf-slim/master/tf_slim/data/dataset.py),
which stores pointers to the data file, as well as various other pieces of
metadata, such as the class labels, the train/test split, and how to parse the
TFExample protos. We have included the TF-Slim Dataset descriptors
for
[Flowers](https://github.com/tensorflow/models/blob/master/research/slim/datasets/flowers.py),
[Cifar10](https://github.com/tensorflow/models/blob/master/research/slim/datasets/cifar10.py),
[MNIST](https://github.com/tensorflow/models/blob/master/research/slim/datasets/mnist.py),
[ImageNet](https://github.com/tensorflow/models/blob/master/research/slim/datasets/imagenet.py)
and
[VisualWakeWords](https://github.com/tensorflow/models/blob/master/research/slim/datasets/visualwakewords.py),
An example of how to load data using a TF-Slim dataset descriptor using a
TF-Slim
[DatasetDataProvider](https://github.com/google-research/tf-slim/tree/master/tf_slim/data/dataset_data_provider.py)
is found below:
```python
import tensorflow.compat.v1 as tf
import tf_slim as slim
from datasets import flowers
# Selects the 'validation' dataset.
dataset = flowers.get_split('validation', DATA_DIR)
# Creates a TF-Slim DataProvider which reads the dataset in the background
# during both training and testing.
provider = slim.dataset_data_provider.DatasetDataProvider(dataset)
[image, label] = provider.get(['image', 'label'])
```
## An automated script for processing ImageNet data.
Training a model with the ImageNet dataset is a common request. To facilitate
working with the ImageNet dataset, we provide an automated script for
downloading and processing the ImageNet dataset into the native TFRecord
format.
The TFRecord format consists of a set of sharded files where each entry is a serialized `tf.Example` proto. Each `tf.Example` proto contains the ImageNet image (JPEG encoded) as well as metadata such as label and bounding box information.
We provide a single [script](datasets/download_and_convert_imagenet.sh) for
downloading and converting ImageNet data to TFRecord format. Downloading and
preprocessing the data may take several hours (up to half a day) depending on
your network and computer speed. Please be patient.
To begin, you will need to sign up for an account with [ImageNet]
(http://image-net.org) to gain access to the data. Look for the sign up page,
create an account and request an access key to download the data.
After you have `USERNAME` and `PASSWORD`, you are ready to run our script. Make
sure that your hard disk has at least 500 GB of free space for down
没有合适的资源?快使用搜索试试~ 我知道了~
温馨提示
**内容概要:** MobileNetV2模型是一种轻量级的卷积神经网络变体模型,旨在在资源受限的移动设备上实现高效的图像分类和目标识别。MobileNetV2通过使用深度可分离卷积等技术,实现了在保持较高准确性的同时减少模型参数和计算量。 适用人群:MobileNetV2模型适用于需要在移动设备或资源受限环境下进行图像分类、目标检测等任务的研究人员、工程师以及移动应用开发者。其轻量级设计和高效性能使得在移动端部署和使用成为可能。 使用场景及目标:MobileNetV2模型的主要目标是在移动设备上实现高效的图像分类和目标识别。通过减少模型参数和计算量,MobileNetV2能够在资源受限的情况下保持较高的准确性,适用于移动端应用、嵌入式系统等场景。 其他说明:MobileNetV2模型的设计思想是在保持模型轻量级和高效性的同时,尽可能提高图像分类和目标识别的性能。其成功应用在移动设备上,为在资源受限环境下部署深度学习模型提供了重要的解决方案。
资源推荐
资源详情
资源评论
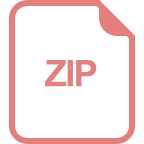
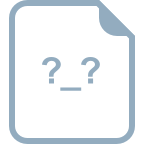
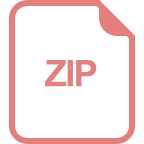
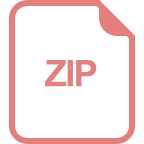
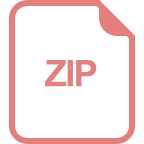
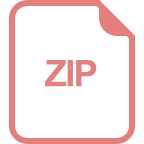
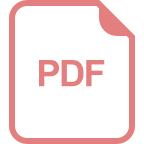
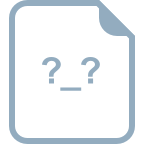
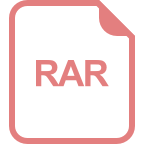
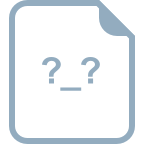
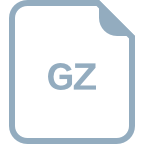
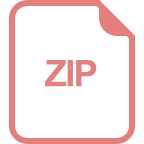
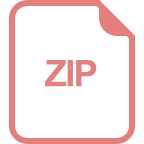
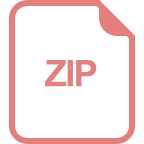
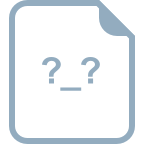
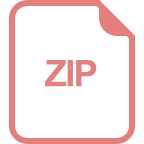
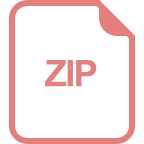
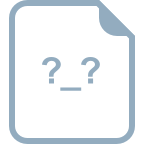
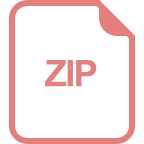
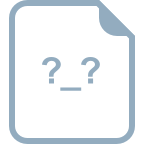
收起资源包目录

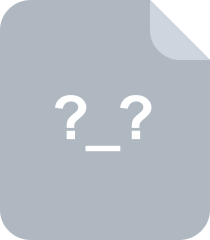
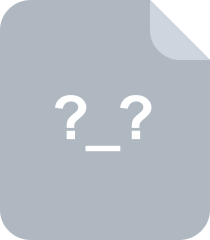
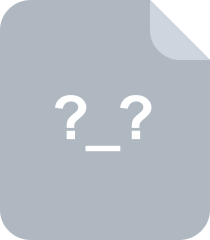
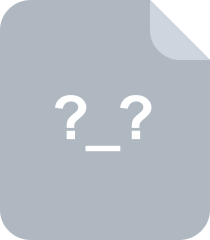
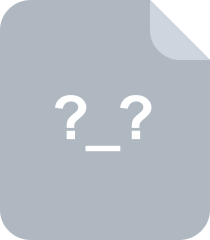
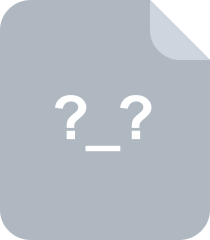
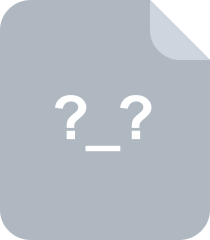
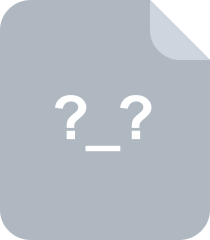
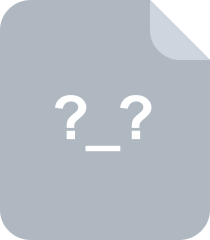
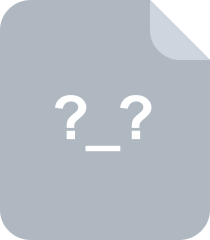
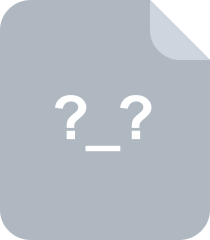
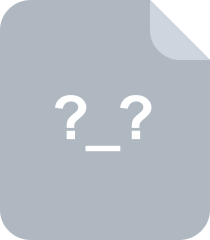
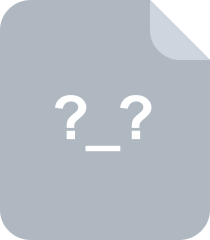
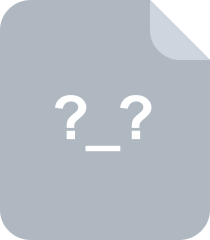
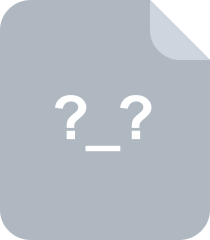
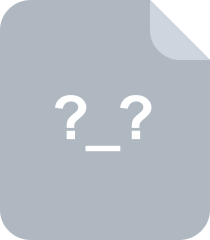
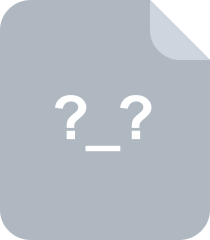
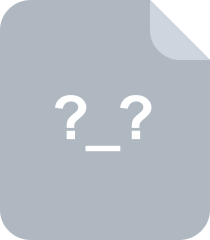
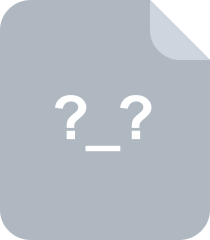
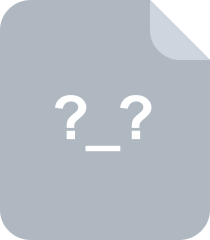
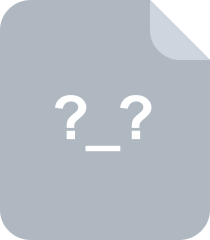
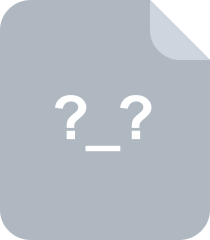
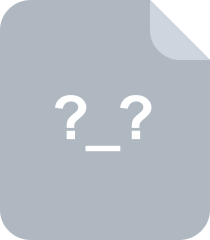
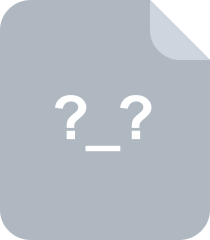
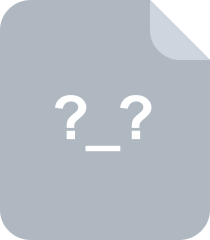
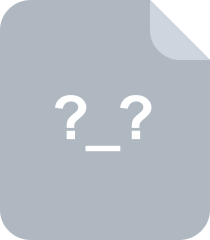
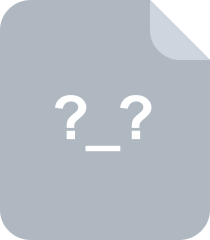
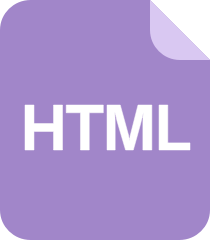
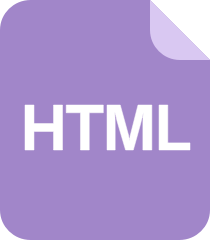
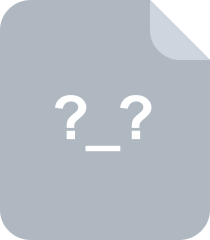
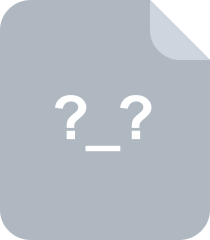
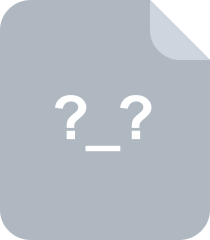
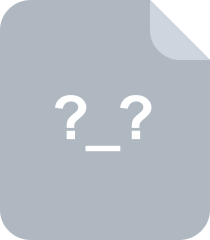
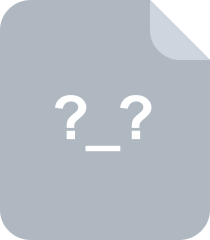
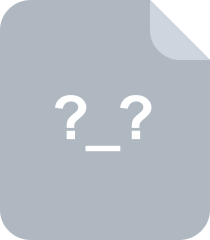
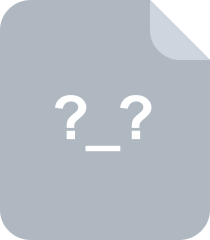
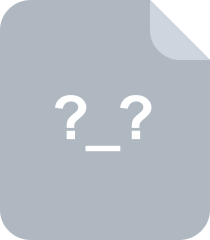
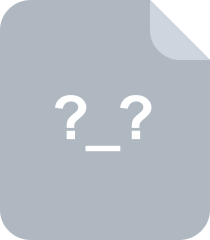
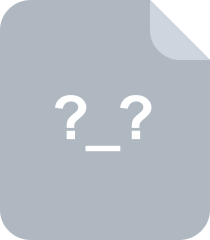
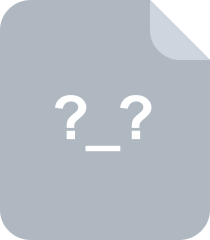
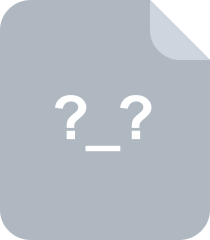
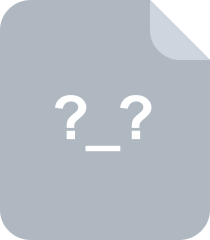
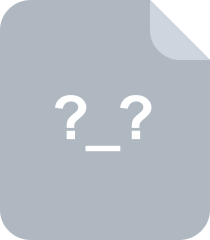
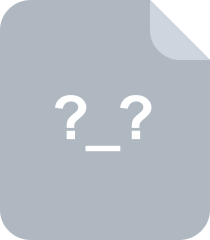
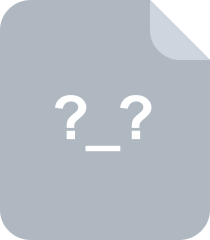
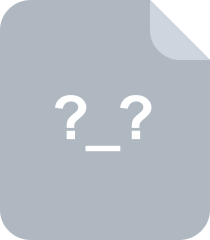
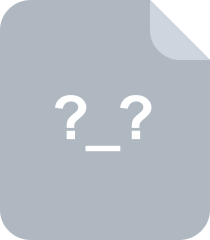
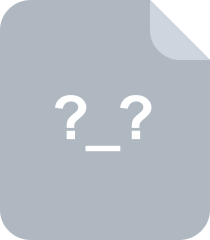
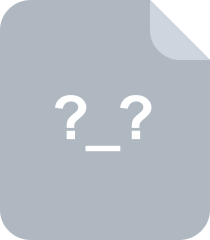
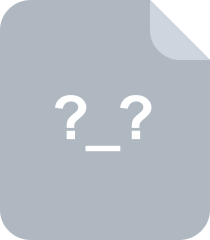
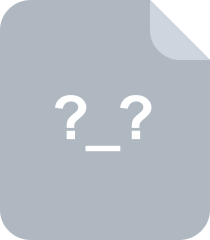
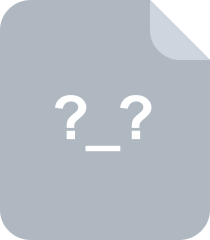
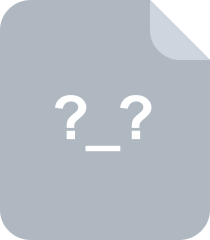
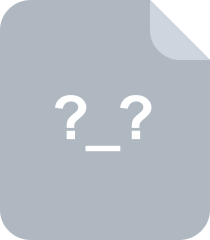
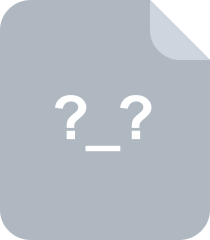
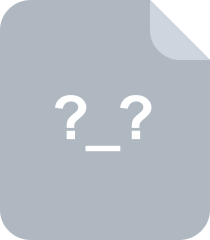
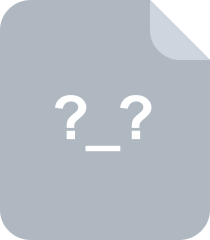
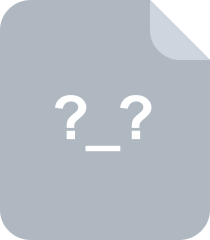
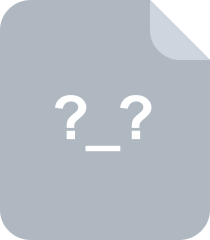
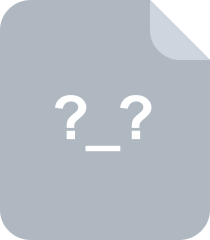
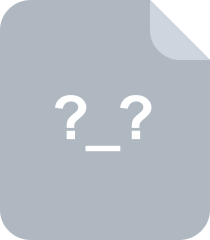
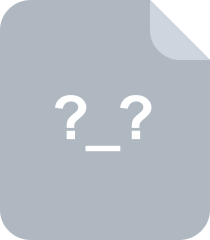
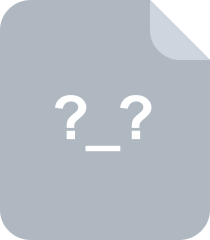
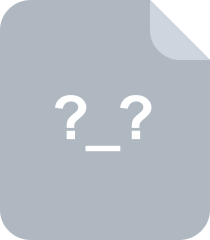
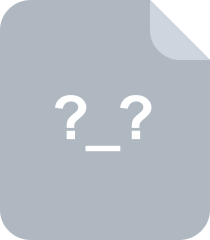
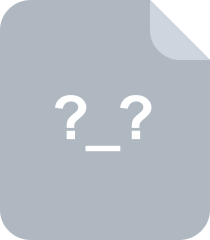
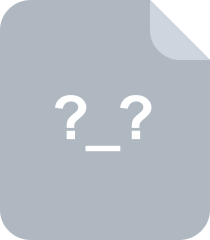
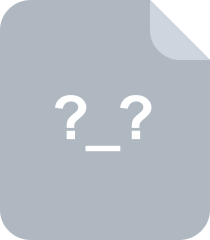
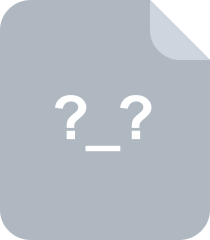
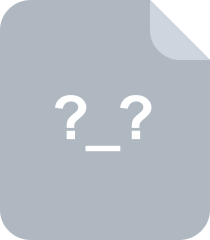
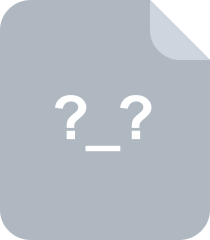
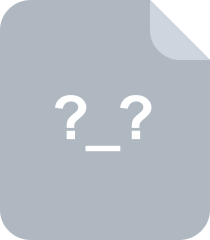
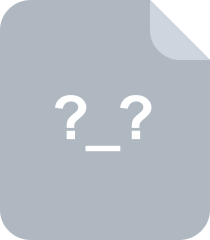
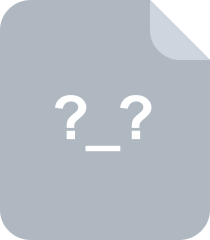
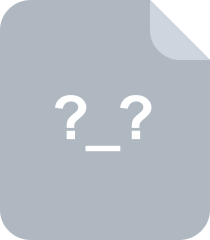
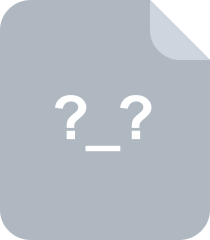
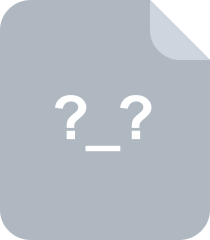
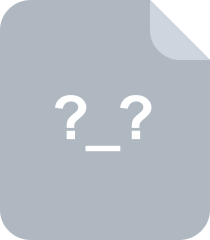
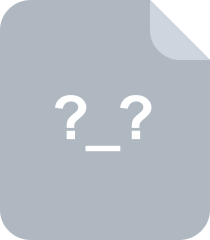
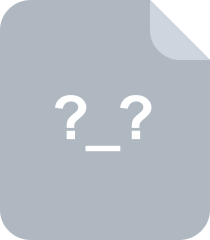
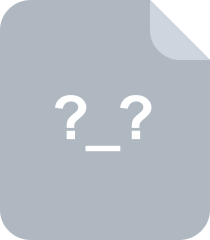
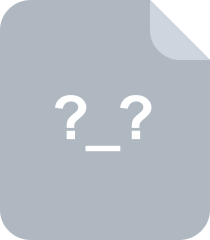
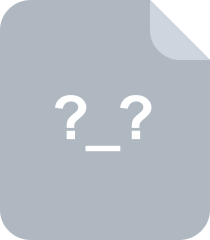
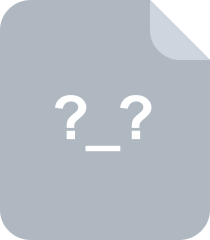
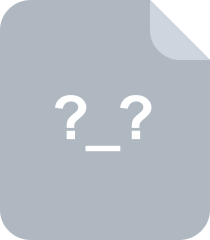
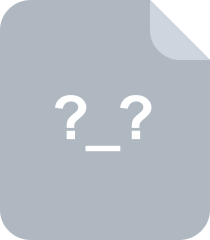
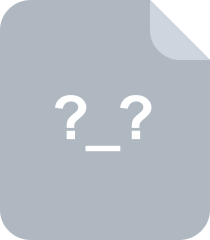
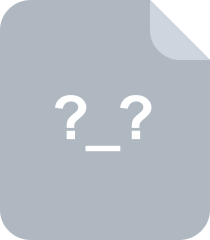
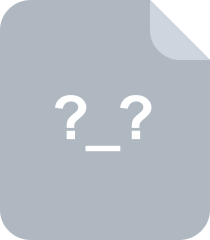
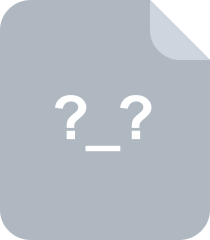
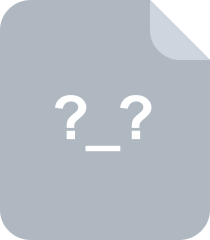
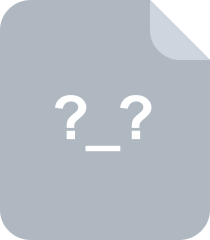
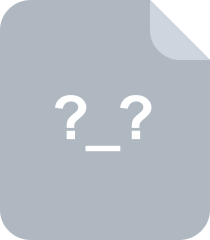
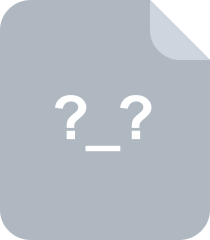
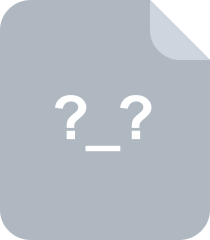
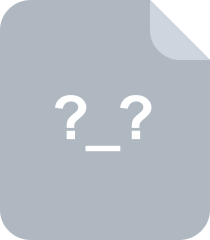
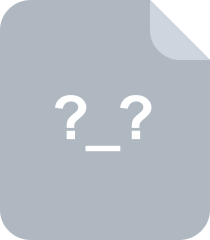
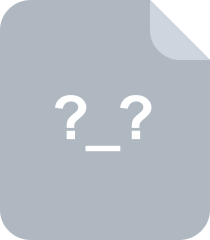
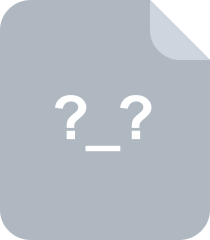
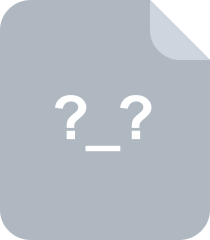
共 2000 条
- 1
- 2
- 3
- 4
- 5
- 6
- 20
资源评论
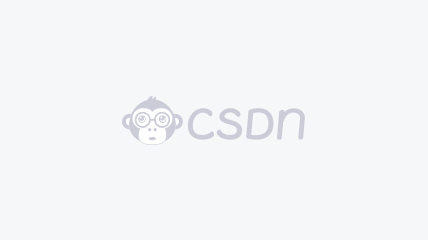

Glaube07
- 粉丝: 1
- 资源: 6
上传资源 快速赚钱
我的内容管理 展开
我的资源 快来上传第一个资源
我的收益
登录查看自己的收益我的积分 登录查看自己的积分
我的C币 登录后查看C币余额
我的收藏
我的下载
下载帮助

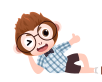
最新资源
资源上传下载、课程学习等过程中有任何疑问或建议,欢迎提出宝贵意见哦~我们会及时处理!
点击此处反馈


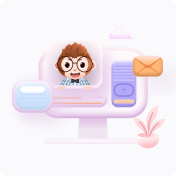
安全验证
文档复制为VIP权益,开通VIP直接复制
