# SentencePiece Python Wrapper
Python wrapper for SentencePiece. This API will offer the encoding, decoding and training of Sentencepiece.
## Build and Install SentencePiece
For Linux (x64/i686), macOS, and Windows(win32/x64) environment, you can simply use pip command to install SentencePiece python module.
```
% pip install sentencepiece
```
To build and install the Python wrapper from source, try the following commands to build and install wheel package.
```
% git clone https://github.com/google/sentencepiece.git
% cd sentencepiece
% mkdir build
% cd build
% cmake .. -DSPM_ENABLE_SHARED=OFF -DCMAKE_INSTALL_PREFIX=./root
% make install
% cd ../python
% python setup.py bdist_wheel
% pip install dist/sentencepiece*.whl
```
If you don’t have write permission to the global site-packages directory or don’t want to install into it, please try:
```
% python setup.py install --user
```
## Usage
See [this google colab page](https://github.com/google/sentencepiece/blob/master/python/sentencepiece_python_module_example.ipynb) to run sentencepiece interactively.
### Segmentation
```
% python
>>> import sentencepiece as spm
>>> sp = spm.SentencePieceProcessor(model_file='test/test_model.model')
>>> sp.encode('This is a test')
[284, 47, 11, 4, 15, 400]
>>> sp.encode(['This is a test', 'Hello world'], out_type=int)
[[284, 47, 11, 4, 15, 400], [151, 88, 21, 887]]
>>> sp.encode_as_ids(['This is a test', 'Hello world'])
[[284, 47, 11, 4, 15, 400], [151, 88, 21, 887]]
>>> sp.encode('This is a test', out_type=str)
['▁This', '▁is', '▁a', '▁', 't', 'est']
>>> sp.encode(['This is a test', 'Hello world'], out_type=str)
[['▁This', '▁is', '▁a', '▁', 't', 'est'], ['▁He', 'll', 'o', '▁world']]
>>> sp.encode_as_pieces(['This is a test', 'Hello world'])
[['▁This', '▁is', '▁a', '▁', 't', 'est'], ['▁He', 'll', 'o', '▁world']]
>>> proto = sp.encode('This is a test', out_type='immutable_proto')
>>> for n in proto.pieces:
... print('piece="{}" surface="{}" id={} begin={} end={}'.format(n.piece, n.surface, n.id, n.begin, n.end))
...
piece="▁This" surface="This" id=284 begin=0 end=4
piece="▁is" surface=" is" id=47 begin=4 end=7
piece="▁a" surface=" a" id=11 begin=7 end=9
piece="▁" surface=" " id=4 begin=9 end=10
piece="t" surface="t" id=15 begin=10 end=11
piece="est" surface="est" id=400 begin=11 end=14
>>> [[x.id for x in proto.pieces], [x.piece for x in proto.pieces], [x.begin for x in proto.pieces], [x.end for x in proto.pieces]]
[[284, 47, 11, 4, 15, 400], ['▁This', '▁is', '▁a', '▁', 't', 'est'], [0, 4, 7, 9, 10, 11], [4, 7, 9, 10, 11, 14]]
>>> proto2 = sp.encode_as_immutable_proto('This is a test')
>>> proto2 == proto
True
>>> for _ in range(10):
... sp.encode('This is a test', out_type=str, enable_sampling=True, alpha=0.1, nbest_size=-1)
...
['▁', 'This', '▁', 'is', '▁a', '▁', 't', 'e', 'st']
['▁T', 'h', 'i', 's', '▁is', '▁a', '▁', 'te', 's', 't']
['▁T', 'h', 'is', '▁', 'is', '▁', 'a', '▁', 't', 'est']
['▁', 'This', '▁is', '▁', 'a', '▁', 't', 'e', 'st']
['▁', 'This', '▁', 'is', '▁', 'a', '▁', 't', 'e', 's', 't']
['▁This', '▁is', '▁a', '▁', 'te', 's', 't']
['▁This', '▁is', '▁', 'a', '▁', 't', 'e', 'st']
['▁', 'T', 'h', 'is', '▁', 'is', '▁', 'a', '▁', 'te', 'st']
['▁', 'This', '▁', 'i', 's', '▁a', '▁', 't', 'e', 'st']
['▁This', '▁', 'is', '▁a', '▁', 't', 'est']
>> sp.nbest_encode('This is a test', nbest_size=5, out_type=str)
[['▁This', '▁is', '▁a', '▁', 't', 'est'],
['▁This', '▁is', '▁a', '▁', 'te', 'st'],
['▁This', '▁is', '▁a', '▁', 'te', 's', 't'],
['▁This', '▁is', '▁a', '▁', 't', 'e', 'st'],
['▁This', '▁is', '▁a', '▁', 't', 'es', 't']]
>>> sp.sample_encode_and_score('This is a test', num_samples=5, alpha=0.1, out_type=str, wor=True)
[(['▁This', '▁', 'i', 's', '▁a', '▁', 'te', 's', 't'], -3.043105125427246),
(['▁This', '▁', 'i', 's', '▁a', '▁', 'te', 'st'], -2.8475849628448486),
(['▁', 'This', '▁is', '▁', 'a', '▁', 'te', 'st'], -3.043248176574707),
(['▁', 'This', '▁is', '▁a', '▁', 't', 'e', 'st'], -2.87727689743042),
(['▁', 'This', '▁', 'i', 's', '▁', 'a', '▁', 't', 'est'], -3.6284031867980957)]
>>> sp.decode([284, 47, 11, 4, 15, 400])
'This is a test'
>>> sp.decode([[284, 47, 11, 4, 15, 400], [151, 88, 21, 887]])
['This is a test', 'Hello world']
>>> proto = sp.decode([284, 47, 11, 4, 15, 400], out_type='immutable_proto')
>>> proto.text
'This is a test'
>>> sp.decode(['▁', 'This', '▁', 'is', '▁a', '▁', 't', 'e', 'st'])
'This is a test'
>>> sp.decode([['▁This', '▁is', '▁a', '▁', 't', 'est'], ['▁He', 'll', 'o', '▁world']])
['This is a test', 'Hello world']
>>> sp.get_piece_size()
1000
>>> sp.id_to_piece(2)
'</s>'
>>> sp.id_to_piece([2, 3, 4])
['</s>', '\r', '▁']
>>> sp.piece_to_id('<s>')
1
>>> sp.piece_to_id(['</s>', '\r', '▁'])
[2, 3, 4]
>>> len(sp)
1000
>>> sp['</s>']
2
```
### Model Training
Training is performed by passing parameters of [spm_train](https://github.com/google/sentencepiece#train-sentencepiece-model) to SentencePieceTrainer.train() function.
```
>>> import sentencepiece as spm
>>> spm.SentencePieceTrainer.train(input='test/botchan.txt', model_prefix='m', vocab_size=1000, user_defined_symbols=['foo', 'bar'])
sentencepiece_trainer.cc(73) LOG(INFO) Starts training with :
trainer_spec {
input: test/botchan.txt
.. snip
unigram_model_trainer.cc(500) LOG(INFO) EM sub_iter=1 size=1188 obj=10.2839 num_tokens=32182 num_tokens/piece=27.0892
unigram_model_trainer.cc(500) LOG(INFO) EM sub_iter=0 size=1100 obj=10.4269 num_tokens=33001 num_tokens/piece=30.0009
unigram_model_trainer.cc(500) LOG(INFO) EM sub_iter=1 size=1100 obj=10.4069 num_tokens=33002 num_tokens/piece=30.0018
trainer_interface.cc(595) LOG(INFO) Saving model: m.model
trainer_interface.cc(619) LOG(INFO) Saving vocabs: m.vocab
>>>
```
### Training without local filesystem
Sentencepiece trainer can receive any iterable object to feed training sentences. You can also pass a file object (instance with write() method) to emit the output model to any devices. These features are useful to run sentencepiece on environment that have limited access to the local file system (e.g., Google colab.)
```
import urllib.request
import io
import sentencepiece as spm
# Loads model from URL as iterator and stores the model to BytesIO.
model = io.BytesIO()
with urllib.request.urlopen(
'https://raw.githubusercontent.com/google/sentencepiece/master/data/botchan.txt'
) as response:
spm.SentencePieceTrainer.train(
sentence_iterator=response, model_writer=model, vocab_size=1000)
# Serialize the model as file.
# with open('out.model', 'wb') as f:
# f.write(model.getvalue())
# Directly load the model from serialized model.
sp = spm.SentencePieceProcessor(model_proto=model.getvalue())
print(sp.encode('this is test'))
```
没有合适的资源?快使用搜索试试~ 我知道了~
温馨提示
SentencePiece是一个无监督的文本分词器和去分词器,主要用于 基于神经网络的文本生成系统,其中词汇量 在神经模型训练之前预先确定。SentencePiece实现了子词单元(例如,字节对编码(BPE)[Sennrich et al.])和unigram语言模型[Kudo.]。 随着原始句子的直接训练的扩展。SentencePiece允许我们创建一个纯粹的端到端系统,不依赖于特定于语言的预处理/后处理。
资源推荐
资源详情
资源评论
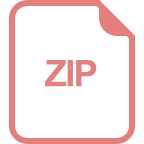
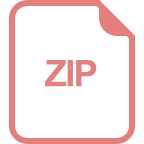
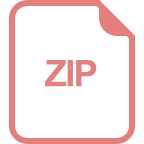
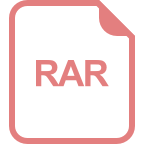
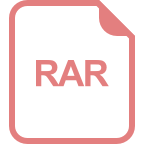
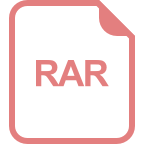
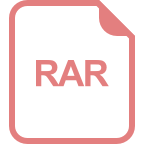
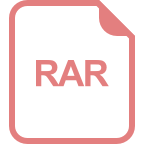
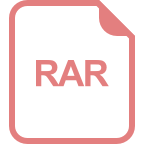
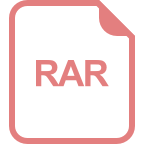
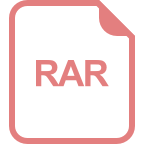
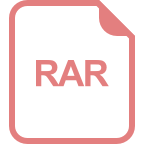
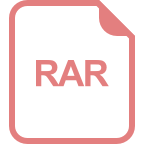
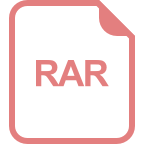
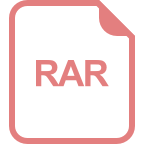
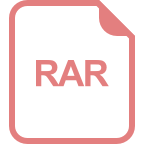
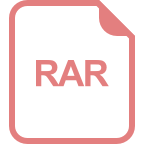
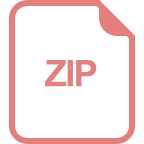
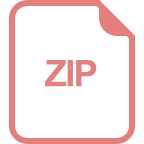
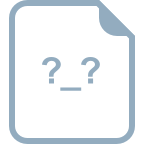
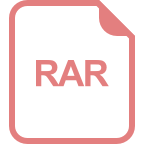
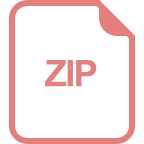
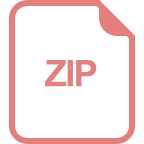
收起资源包目录

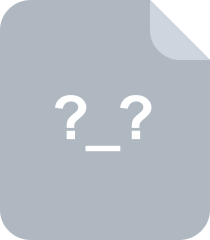
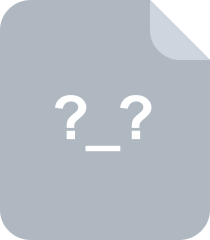
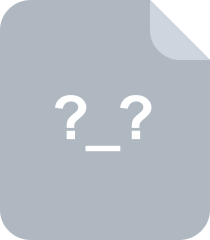
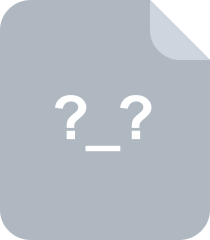
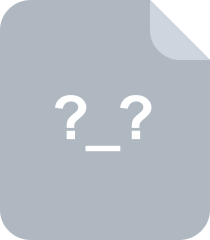
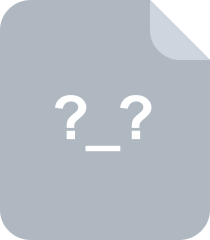
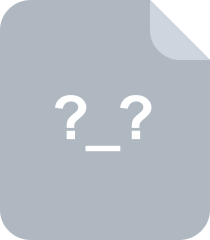
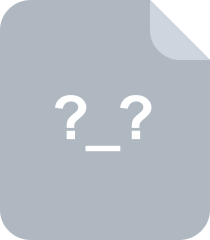
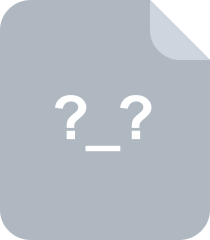
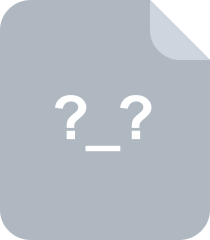
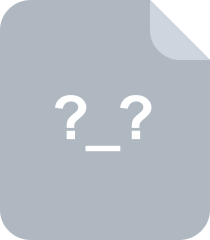
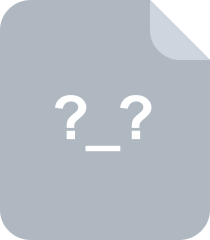
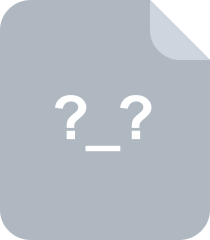
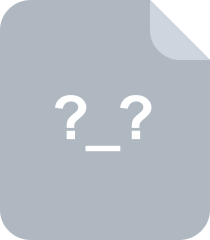
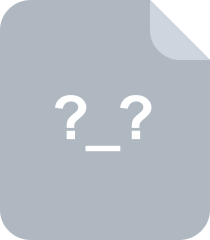
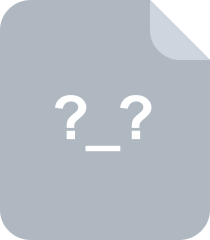
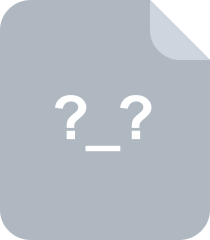
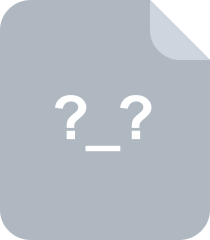
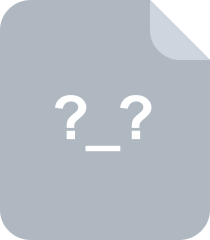
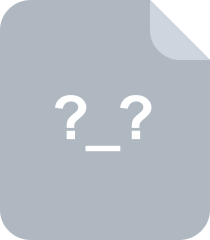
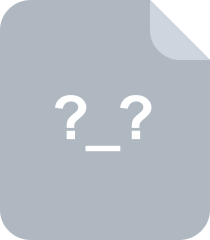
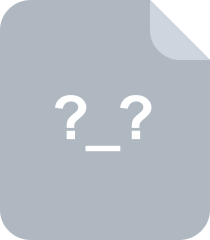
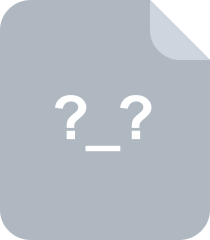
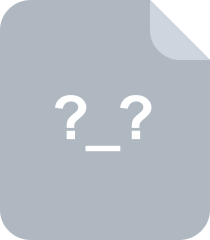
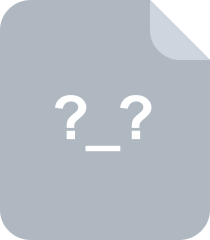
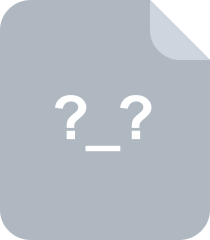
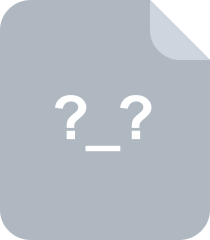
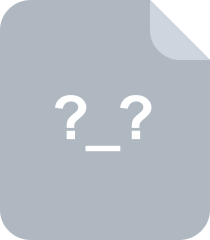
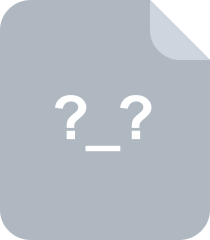
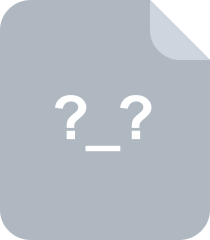
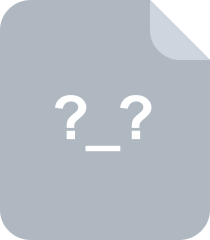
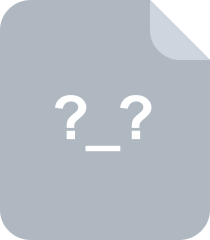
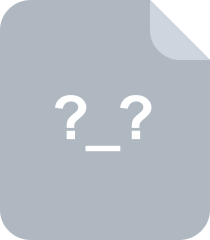
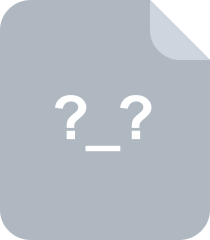
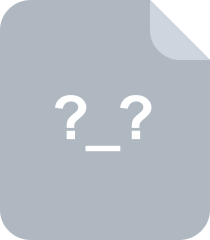
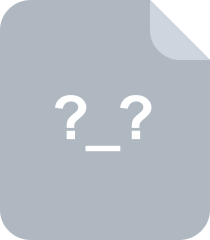
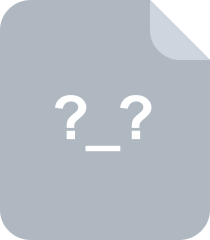
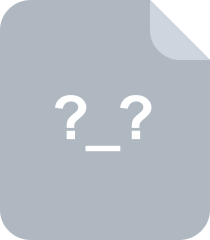
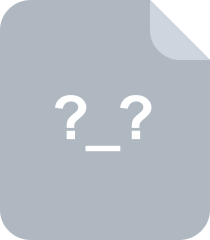
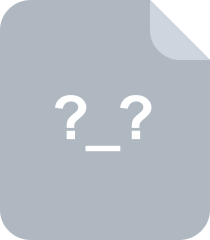
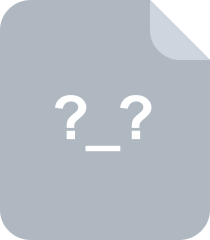
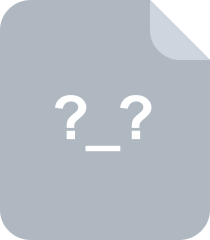
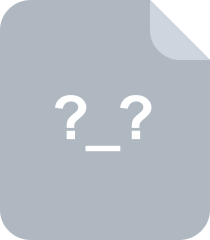
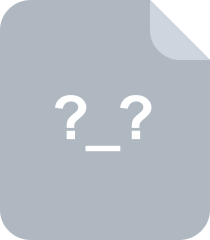
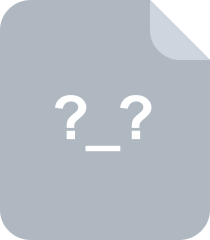
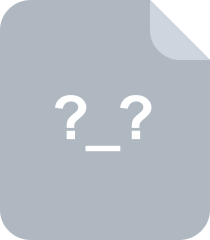
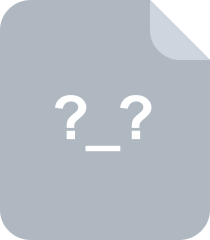
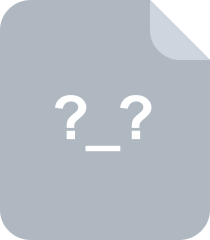
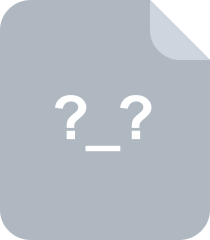
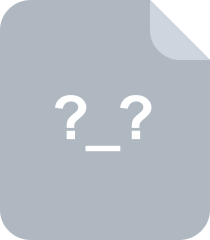
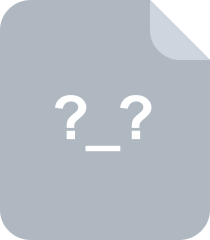
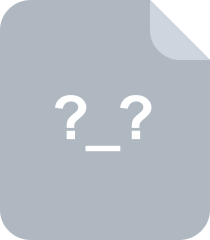
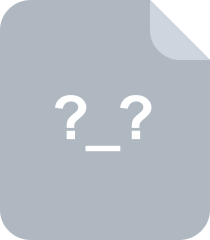
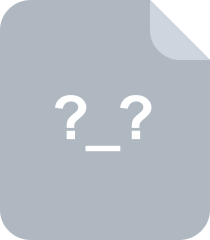
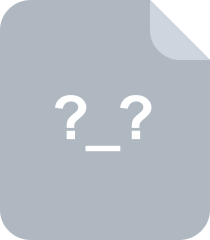
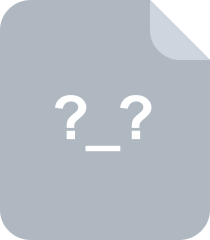
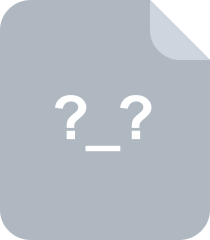
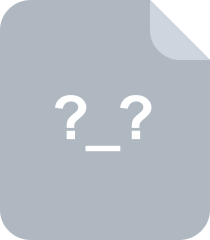
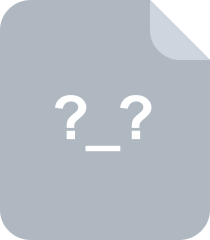
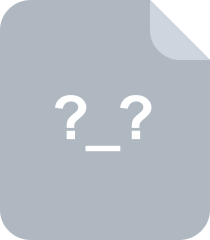
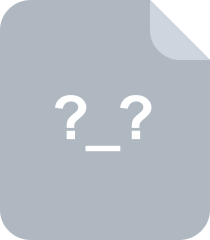
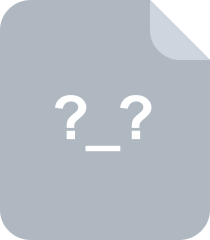
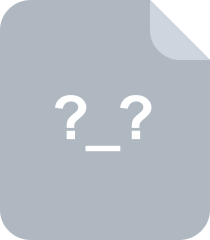
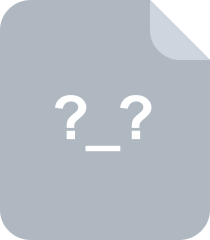
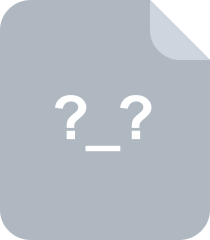
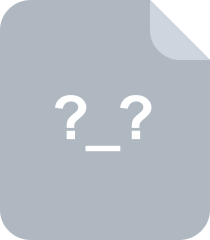
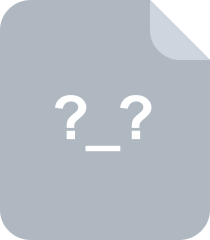
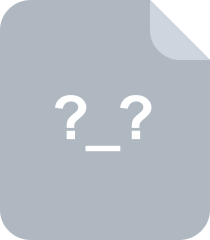
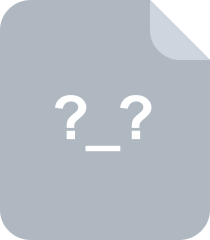
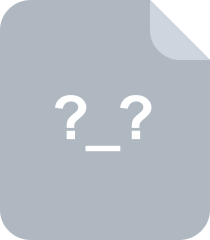
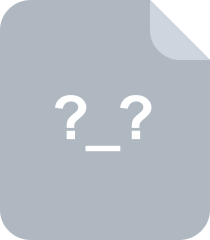
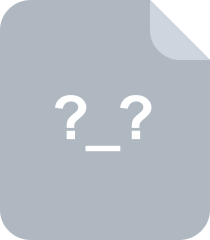
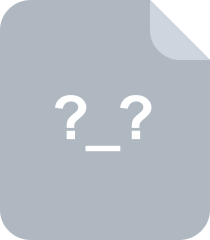
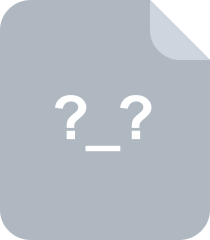
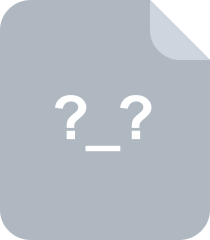
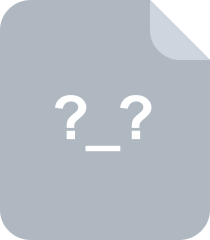
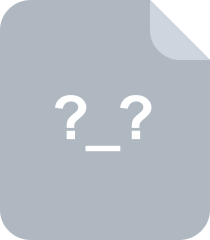
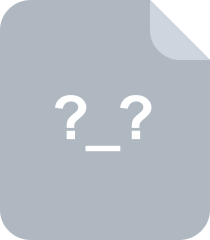
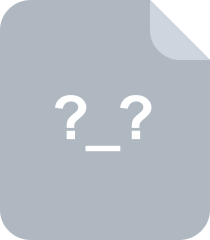
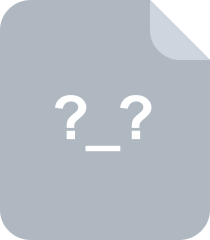
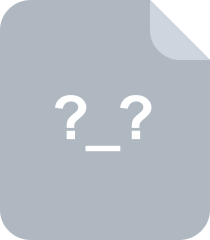
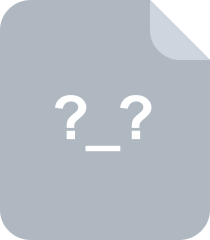
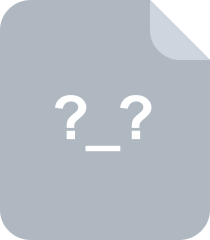
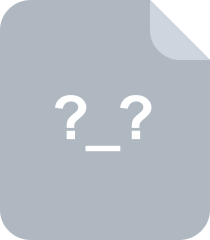
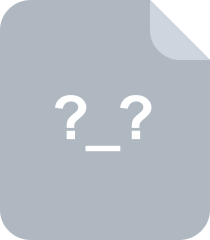
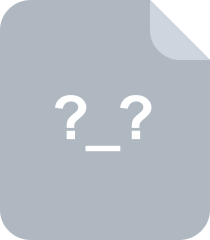
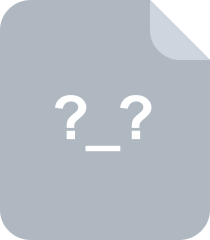
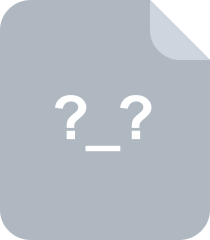
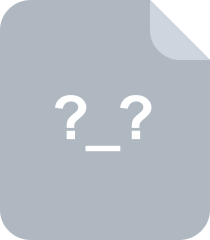
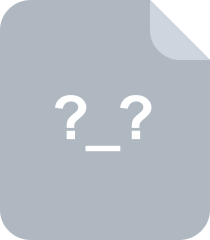
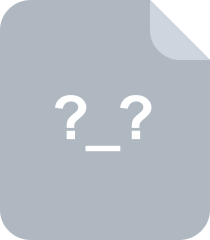
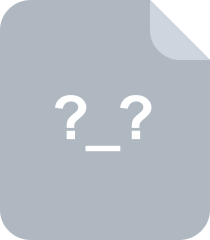
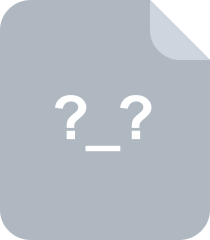
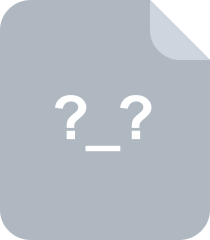
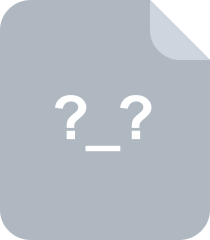
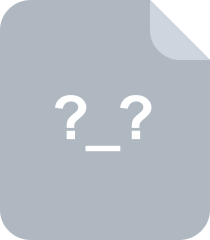
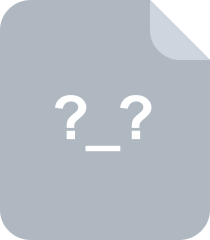
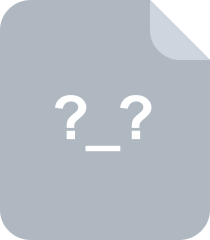
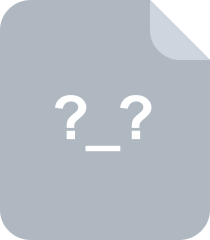
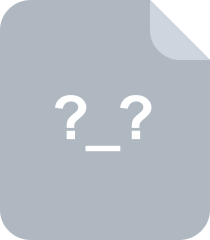
共 239 条
- 1
- 2
- 3
资源评论
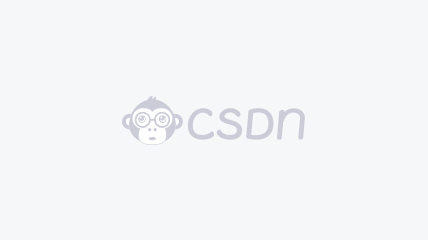

Java程序员-张凯
- 粉丝: 1w+
- 资源: 7530
上传资源 快速赚钱
我的内容管理 展开
我的资源 快来上传第一个资源
我的收益
登录查看自己的收益我的积分 登录查看自己的积分
我的C币 登录后查看C币余额
我的收藏
我的下载
下载帮助

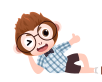
最新资源
- 基于java+springboot+vue+mysql的水果蔬菜商城 源码+数据库+论文(高分毕业设计).zip
- 基于java+springboot+vue+mysql的网上购物商城 源码+数据库+论文(高分毕业设计).zip
- 基于java+springboot+vue+mysql的校园朋友圈系统 源码+数据库+论文(高分毕业设计).zip
- 深度学习驱动的油气开发工程技术
- 基于java+springboot+vue+mysql的无可购物网站 源码+数据库+论文(高分毕业设计).zip
- sasl-0.3.1-cp37-cp37m-win-amd64.whl
- 蛇年的祝福前奏,以及JAVA贪吃蛇的一个项目讲解
- 包含fontawesome-pro依赖库的node库,版本对应v18.20.4
- 深度学习在岩土工程中应用与实践=-前沿技术与创新实践(2025)
- boost1.72+win10+vs2022+64位动态编译+debug版本
- 静态无功补偿器(SVC)仿真模型 采用静态无功补偿器(SVC)对一个500kv, 3000mva的系统进行电压调节 (1)当系统电压较低时,SVC产生无功功率(SVC电容性) (2)当系统电压较高
- 数字孪生行业发展和数字孪生发展研究报告.pdf
- Huang晶体塑性umat耦合Johnson-Cook损伤模型,实现晶体材料弹塑性损伤模拟分析
- Commander One PRO for Mac v.3.12
- 自动化码头AGV无冲突动态路径规划
- 基于simulink的三自由度汽车操纵模型,模型全套可运行 自由度:侧向-侧倾-横摆 带数据参数与详细公式文档 基于二自由度模型的成熟理论,采用SAE坐标系建立三自由度汽车操纵模型 该模型能够反映
资源上传下载、课程学习等过程中有任何疑问或建议,欢迎提出宝贵意见哦~我们会及时处理!
点击此处反馈


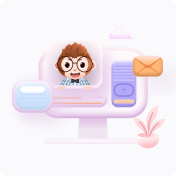
安全验证
文档复制为VIP权益,开通VIP直接复制
