馃摎 This guide explains how to use **Weights & Biases** (W&B) with YOLOv5 馃殌. UPDATED 29 September 2021.
* [About Weights & Biases](#about-weights-&-biases)
* [First-Time Setup](#first-time-setup)
* [Viewing runs](#viewing-runs)
* [Disabling wandb](#disabling-wandb)
* [Advanced Usage: Dataset Versioning and Evaluation](#advanced-usage)
* [Reports: Share your work with the world!](#reports)
## About Weights & Biases
Think of [W&B](https://wandb.ai/site?utm_campaign=repo_yolo_wandbtutorial) like GitHub for machine learning models. With a few lines of code, save everything you need to debug, compare and reproduce your models 鈥� architecture, hyperparameters, git commits, model weights, GPU usage, and even datasets and predictions.
Used by top researchers including teams at OpenAI, Lyft, Github, and MILA, W&B is part of the new standard of best practices for machine learning. How W&B can help you optimize your machine learning workflows:
* [Debug](https://wandb.ai/wandb/getting-started/reports/Visualize-Debug-Machine-Learning-Models--VmlldzoyNzY5MDk#Free-2) model performance in real time
* [GPU usage](https://wandb.ai/wandb/getting-started/reports/Visualize-Debug-Machine-Learning-Models--VmlldzoyNzY5MDk#System-4) visualized automatically
* [Custom charts](https://wandb.ai/wandb/customizable-charts/reports/Powerful-Custom-Charts-To-Debug-Model-Peformance--VmlldzoyNzY4ODI) for powerful, extensible visualization
* [Share insights](https://wandb.ai/wandb/getting-started/reports/Visualize-Debug-Machine-Learning-Models--VmlldzoyNzY5MDk#Share-8) interactively with collaborators
* [Optimize hyperparameters](https://docs.wandb.com/sweeps) efficiently
* [Track](https://docs.wandb.com/artifacts) datasets, pipelines, and production models
## First-Time Setup
<details open>
<summary> Toggle Details </summary>
When you first train, W&B will prompt you to create a new account and will generate an **API key** for you. If you are an existing user you can retrieve your key from https://wandb.ai/authorize. This key is used to tell W&B where to log your data. You only need to supply your key once, and then it is remembered on the same device.
W&B will create a cloud **project** (default is 'YOLOv5') for your training runs, and each new training run will be provided a unique run **name** within that project as project/name. You can also manually set your project and run name as:
```shell
$ python train.py --project ... --name ...
```
YOLOv5 notebook example: <a href="https://colab.research.google.com/github/ultralytics/yolov5/blob/master/tutorial.ipynb"><img src="https://colab.research.google.com/assets/colab-badge.svg" alt="Open In Colab"></a> <a href="https://www.kaggle.com/ultralytics/yolov5"><img src="https://kaggle.com/static/images/open-in-kaggle.svg" alt="Open In Kaggle"></a>
<img width="960" alt="Screen Shot 2021-09-29 at 10 23 13 PM" src="https://user-images.githubusercontent.com/26833433/135392431-1ab7920a-c49d-450a-b0b0-0c86ec86100e.png">
</details>
## Viewing Runs
<details open>
<summary> Toggle Details </summary>
Run information streams from your environment to the W&B cloud console as you train. This allows you to monitor and even cancel runs in <b>realtime</b> . All important information is logged:
* Training & Validation losses
* Metrics: Precision, Recall, mAP@0.5, mAP@0.5:0.95
* Learning Rate over time
* A bounding box debugging panel, showing the training progress over time
* GPU: Type, **GPU Utilization**, power, temperature, **CUDA memory usage**
* System: Disk I/0, CPU utilization, RAM memory usage
* Your trained model as W&B Artifact
* Environment: OS and Python types, Git repository and state, **training command**
<p align="center"><img width="900" alt="Weights & Biases dashboard" src="https://user-images.githubusercontent.com/26833433/135390767-c28b050f-8455-4004-adb0-3b730386e2b2.png"></p>
</details>
## Disabling wandb
* training after running `wandb disabled` inside that directory creates no wandb run
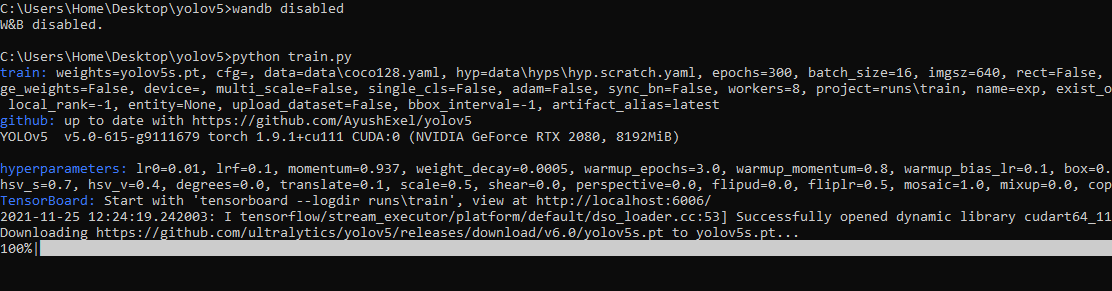
* To enable wandb again, run `wandb online`
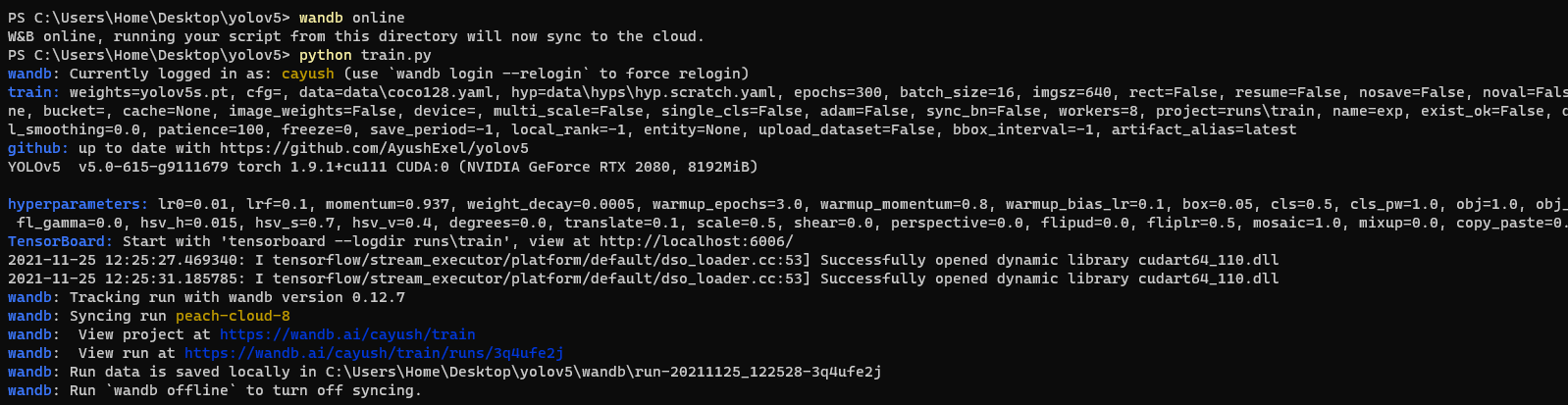
## Advanced Usage
You can leverage W&B artifacts and Tables integration to easily visualize and manage your datasets, models and training evaluations. Here are some quick examples to get you started.
<details open>
<h3> 1: Train and Log Evaluation simultaneousy </h3>
This is an extension of the previous section, but it'll also training after uploading the dataset. <b> This also evaluation Table</b>
Evaluation table compares your predictions and ground truths across the validation set for each epoch. It uses the references to the already uploaded datasets,
so no images will be uploaded from your system more than once.
<details open>
<summary> <b>Usage</b> </summary>
<b>Code</b> <code> $ python train.py --upload_data val</code>
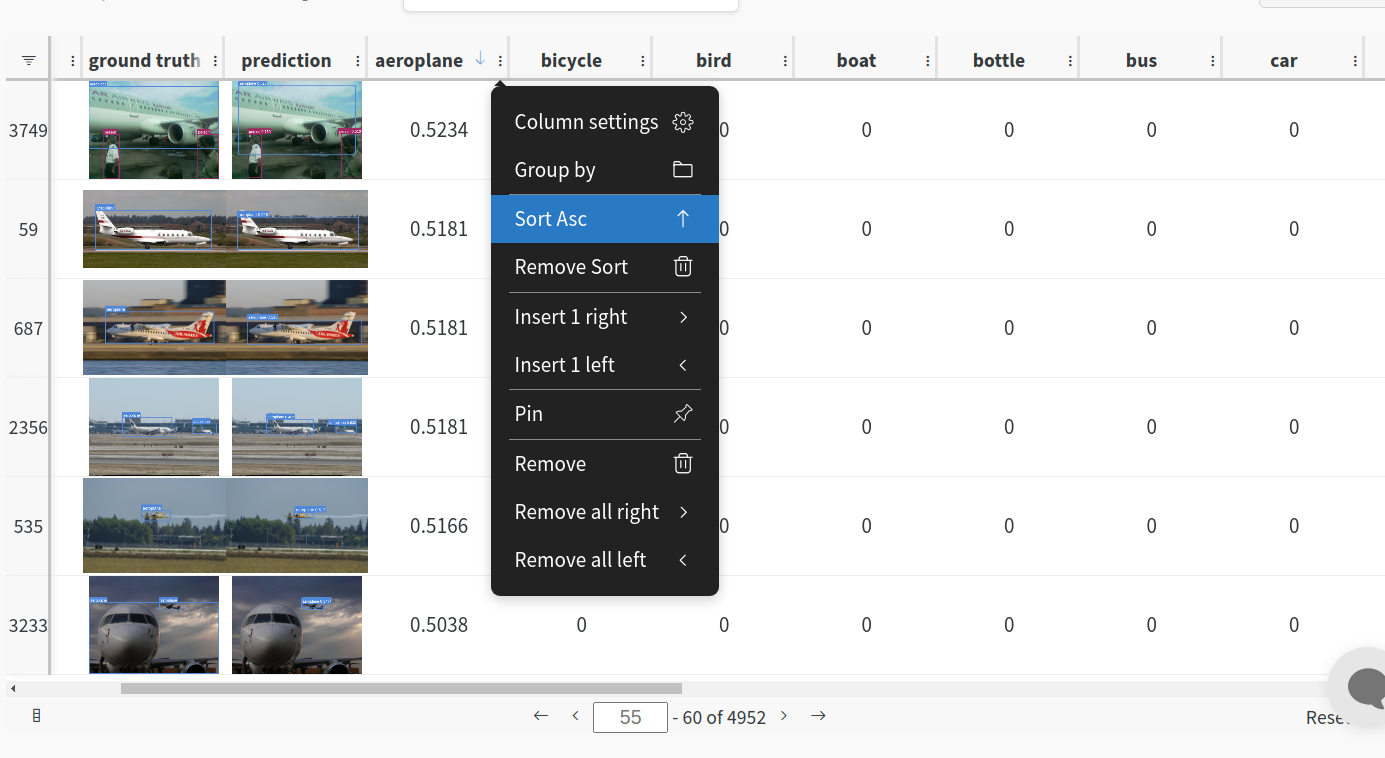
</details>
<h3>2. Visualize and Version Datasets</h3>
Log, visualize, dynamically query, and understand your data with <a href='https://docs.wandb.ai/guides/data-vis/tables'>W&B Tables</a>. You can use the following command to log your dataset as a W&B Table. This will generate a <code>{dataset}_wandb.yaml</code> file which can be used to train from dataset artifact.
<details>
<summary> <b>Usage</b> </summary>
<b>Code</b> <code> $ python utils/logger/wandb/log_dataset.py --project ... --name ... --data .. </code>
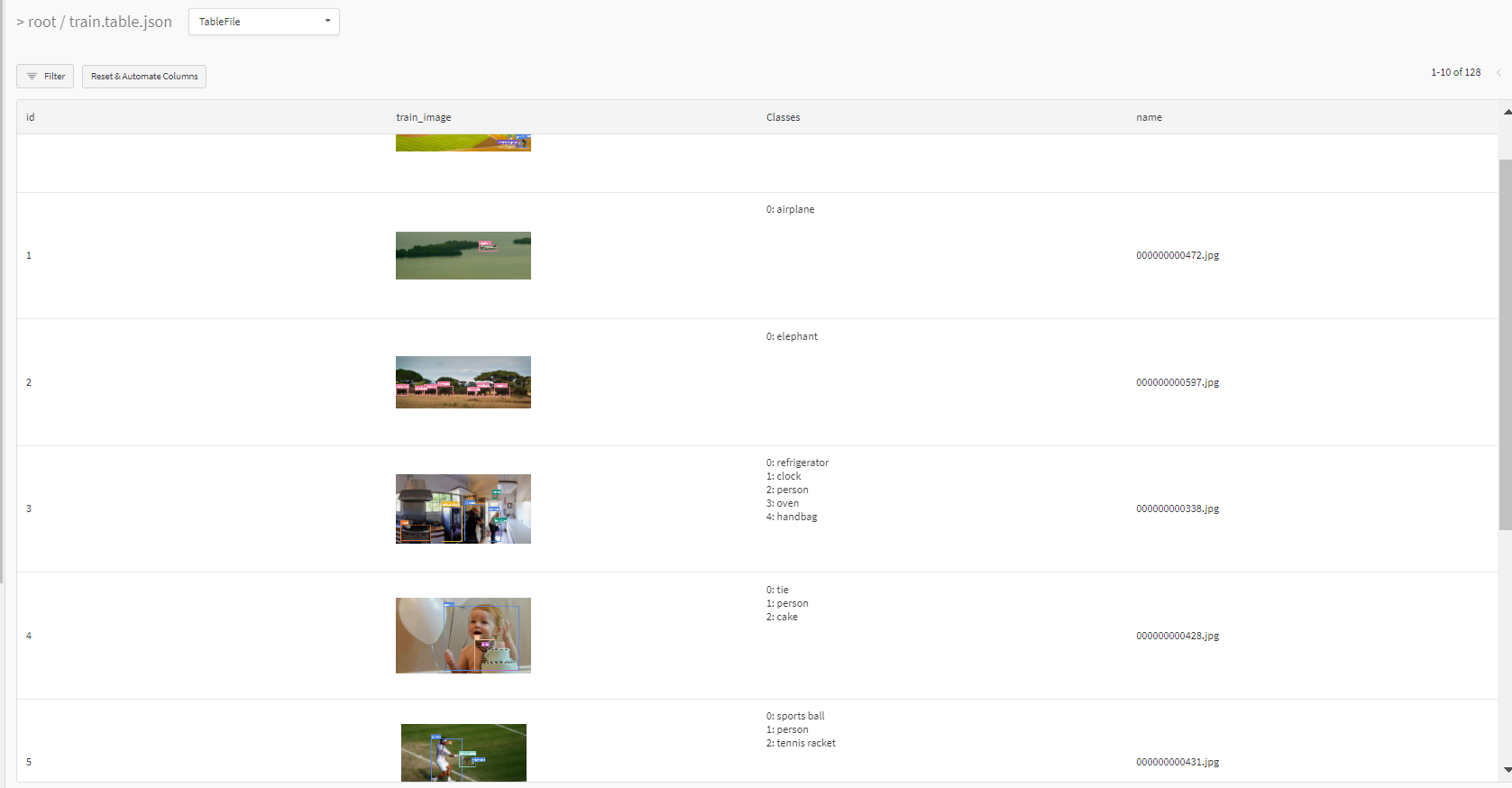
</details>
<h3> 3: Train using dataset artifact </h3>
When you upload a dataset as described in the first section, you get a new config file with an added `_wandb` to its name. This file contains the information that
can be used to train a model directly from the dataset artifact. <b> This also logs evaluation </b>
<details>
<summary> <b>Usage</b> </summary>
<b>Code</b> <code> $ python train.py --data {data}_wandb.yaml </code>
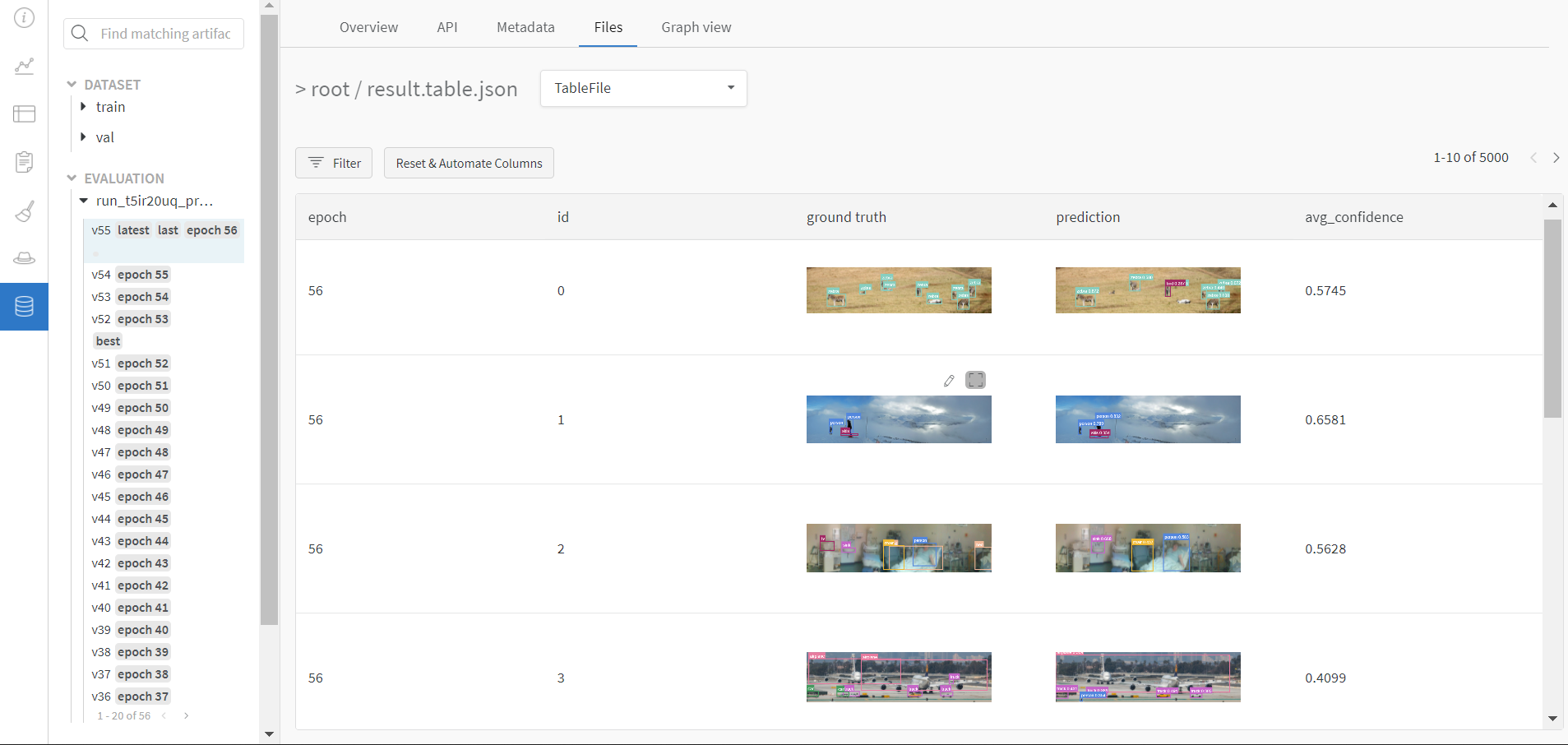
</details>
<h3> 4: Save model checkpoints as artifacts </h3>
To enable saving and versioning checkpoints of your experiment, pass `--save_period n` with the base cammand, where `n` represents checkpoint interval.
You can also log both the dataset and model checkpoints simultaneously. If not passed, only the final model will be logged
<details>
<summary> <b>Usage</b> </summary>
<b>Code</b> <code> $ python train.py --save_period 1 </code>
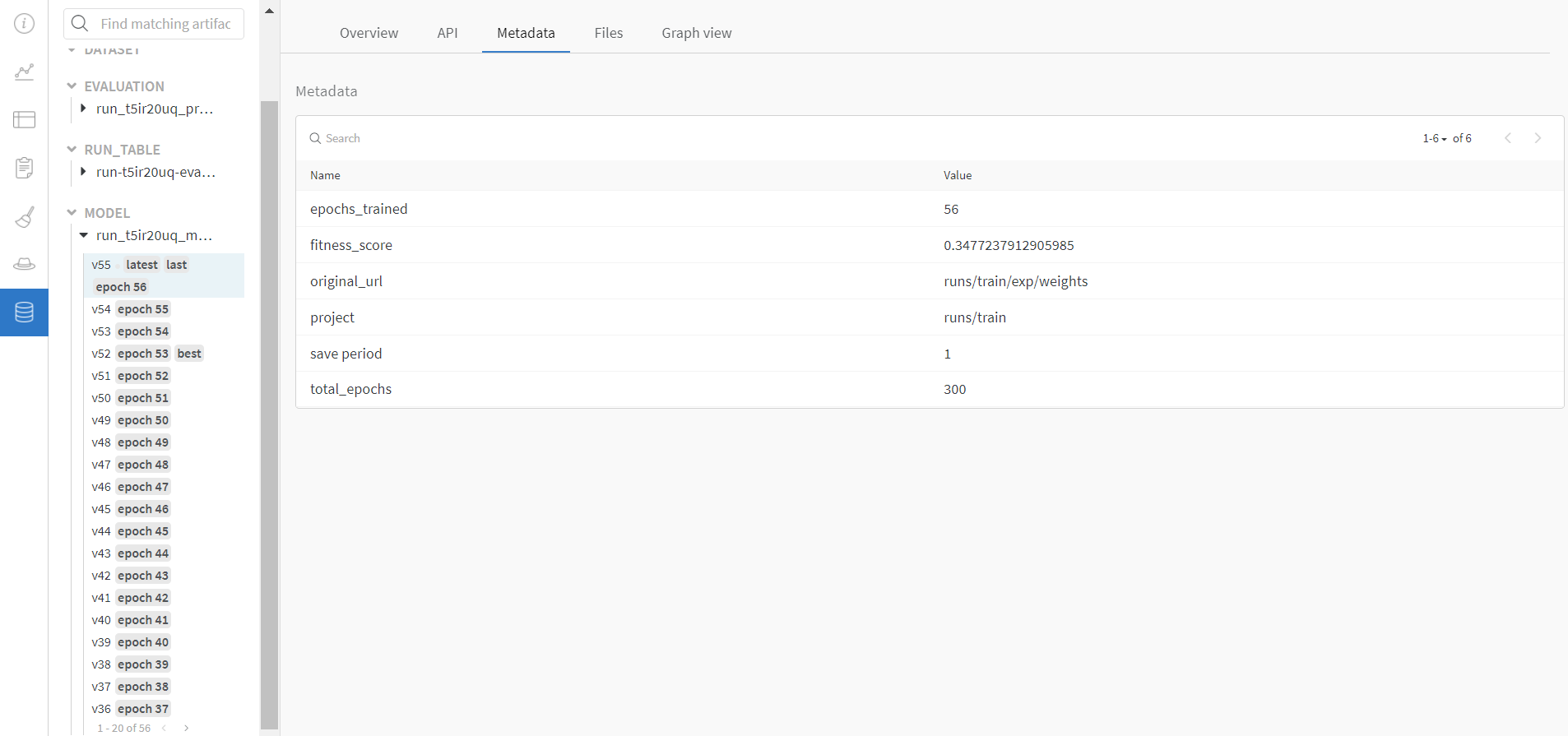
</details>
</details>
<h3> 5: Resume runs from checkpoint artifacts. </h3>
Any run can be resumed using artifacts if the <code>--resume</code> argument starts with聽<code>wandb-artifact://</code>聽prefix followed by the run path, i.e,聽<code>wandb-artifact://username/project/runid </code>. This doesn't require the model checkpoint to be present on the local system.
<details>
<summary> <b>Usage</b> </summary>
<b>Code</b> <code> $ python train.py --resume wandb-artifact://{run_path} </code>
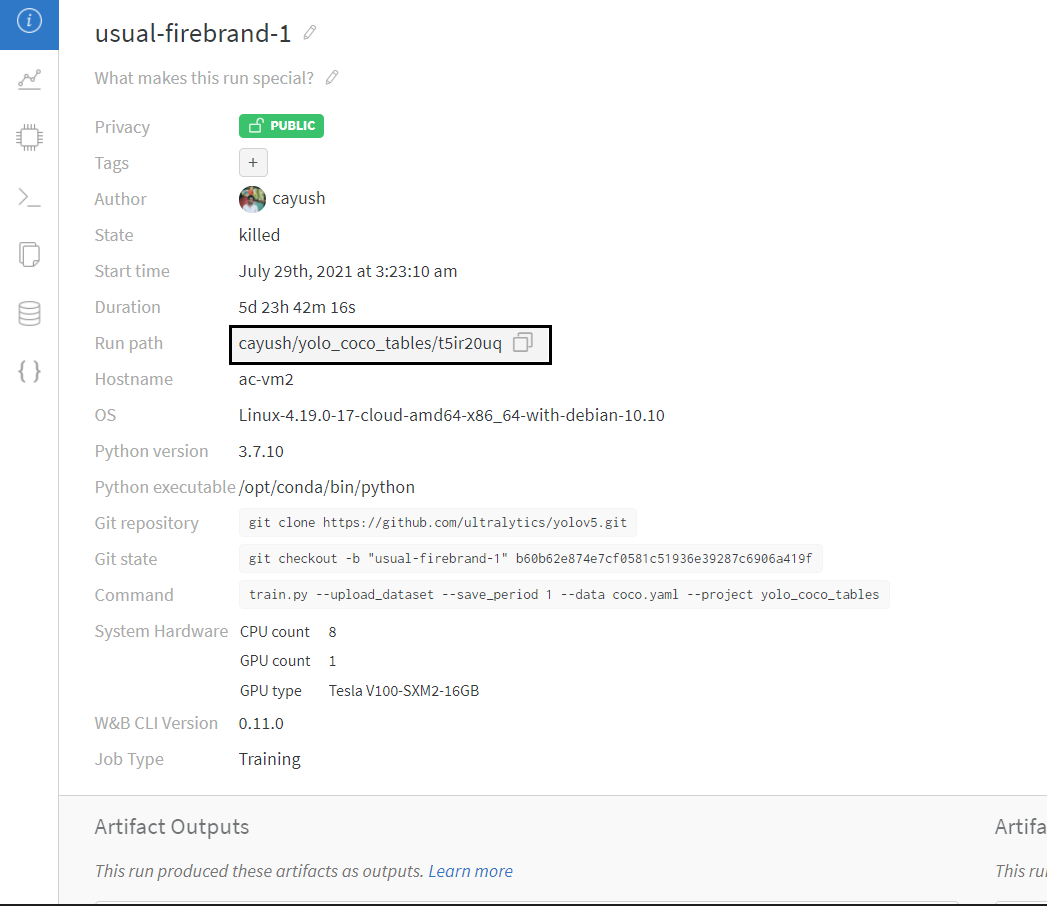
</details>
<h3> 6: Resume runs from dataset artifact & checkpoint artifacts. </h3>
<b> Local dataset or model checkpoints are not required. This can be used to resume runs directly on a different device </b>
The syntax is same as the previous section, but you'll need to lof both the dataset and model checkpoints as artifacts, i.e, set bot <code>--upload_dataset<
没有合适的资源?快使用搜索试试~ 我知道了~
温馨提示
本文件已对YOLOV5的代码进行全中文注释,帮助小伙伴们解决代码看不懂的问题,注释不易切用且珍惜,白嫖的话可以直接看,本项目配套https://blog.csdn.net/qq_39237205/category_11911202.html进行讲解,需要更多详情的可以关注栏目,YOLOv5 是在 YOLOv4 出来之后没多久就横空出世了。目前 YOLOv5 发布了新的版本,6.0版本。在这里,YOLOv5 也在5.0基础上集成了更多特性,同时也对模型做了微调,并且优化了模型大小,减少了模型的参数量。那么这样,就更加适合移动端了。【UTF-8编码】
资源推荐
资源详情
资源评论
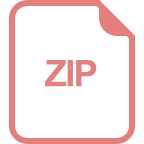
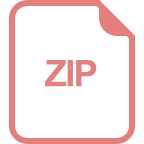
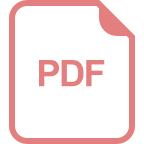
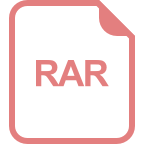
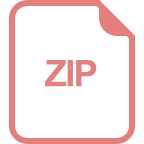
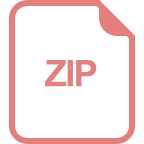
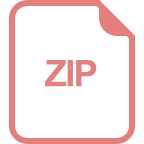
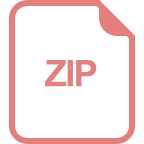
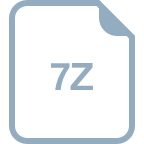
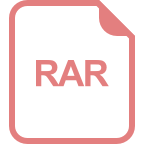
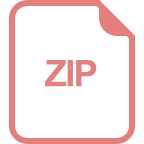
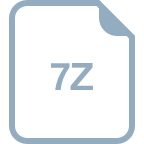
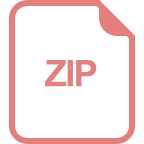
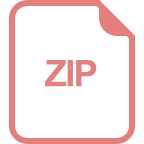
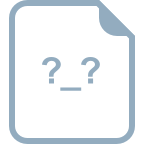
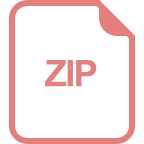
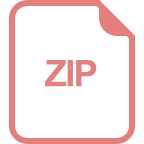
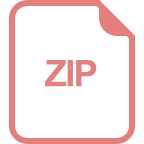
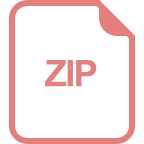
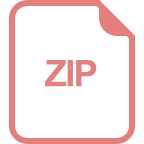
收起资源包目录

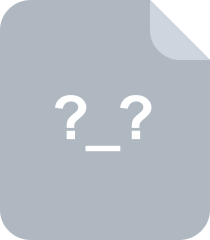
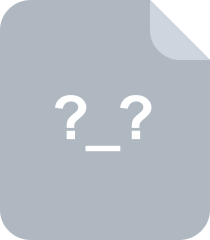
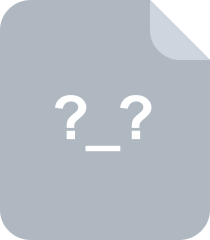
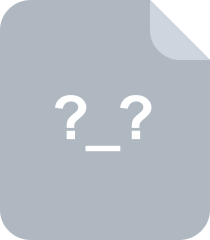
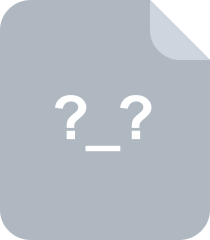
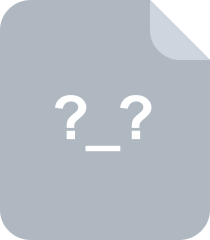
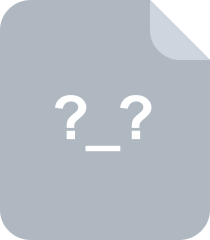
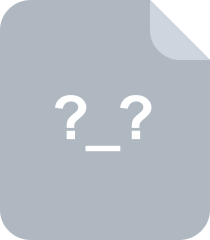
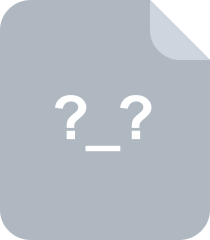
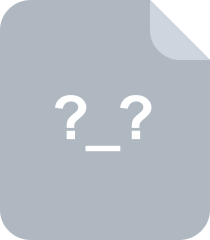
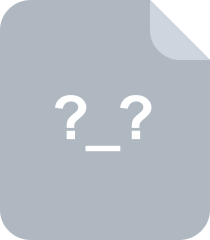
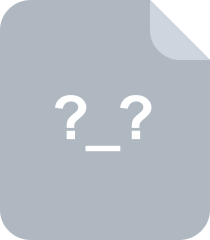
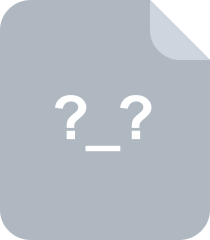
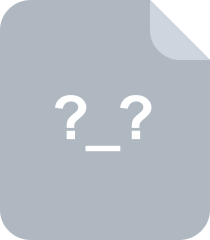
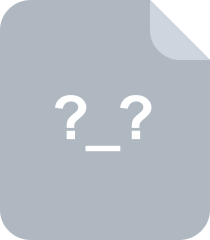
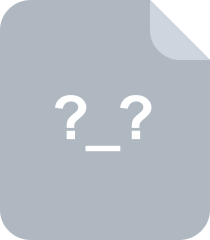
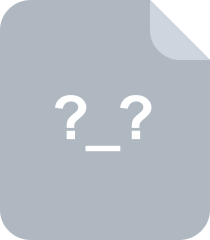
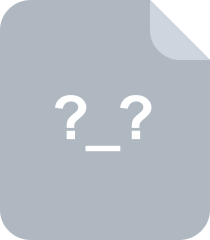
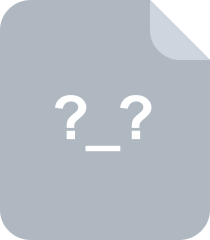
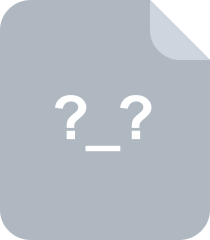
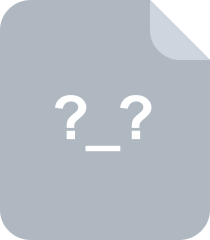
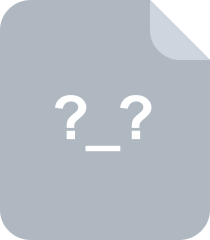
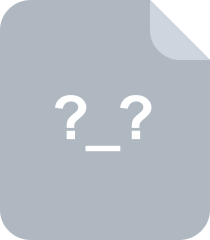
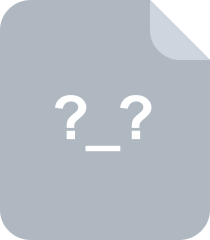
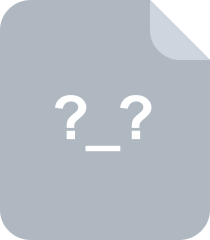
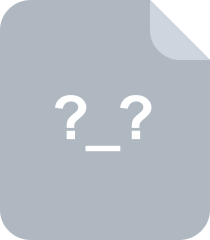
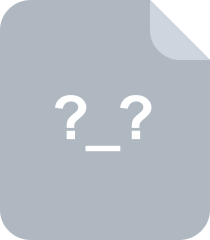
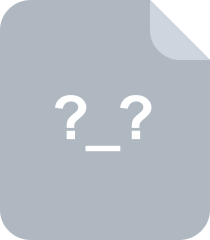
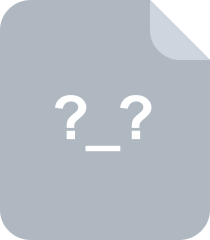
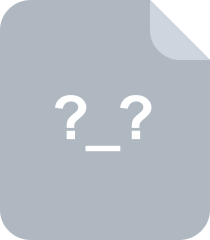
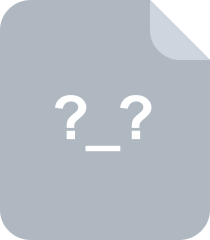
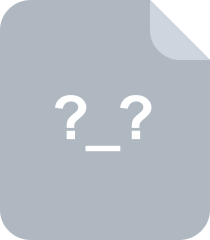
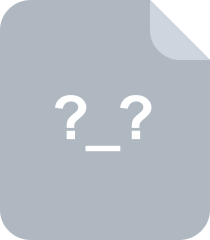
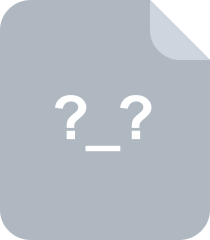
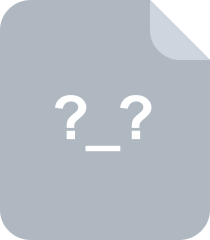
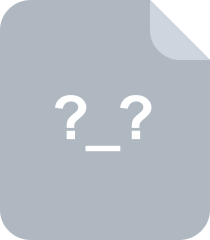
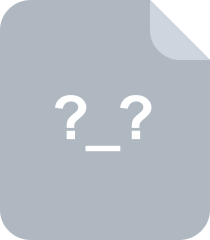
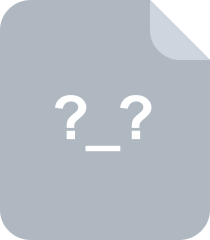
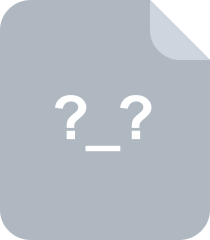
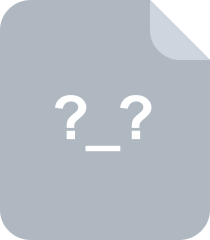
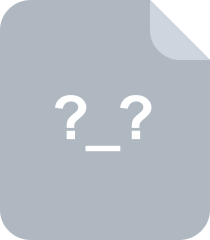
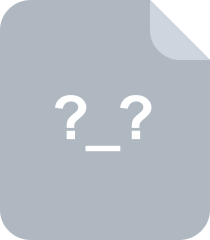
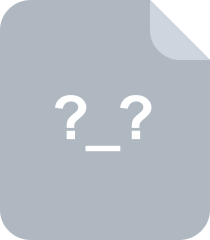
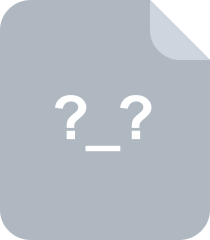
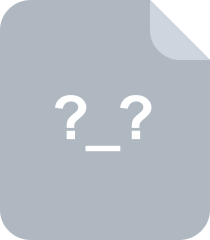
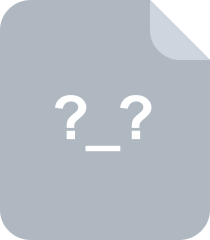
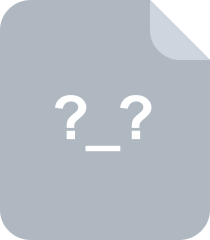
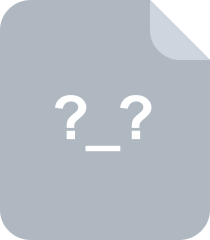
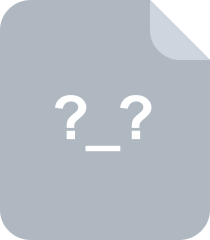
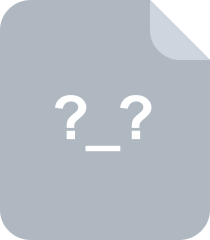
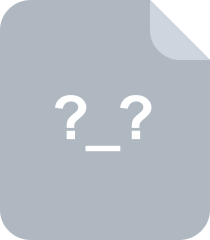
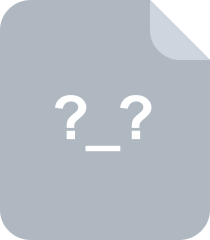
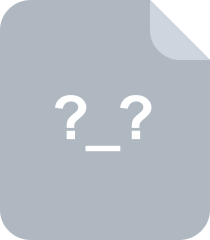
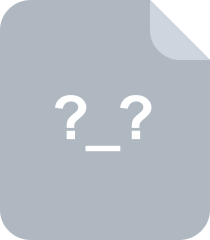
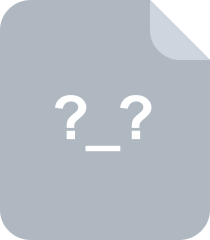
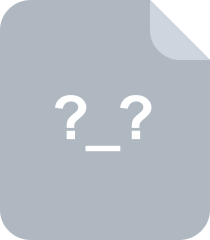
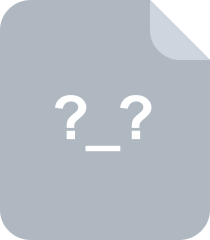
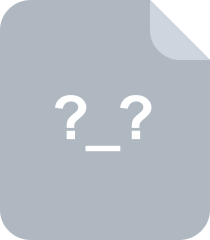
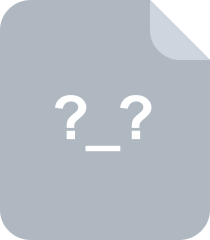
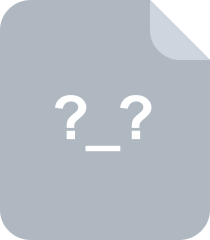
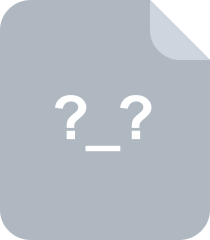
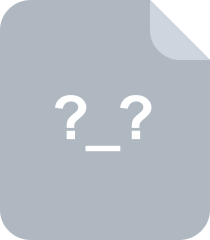
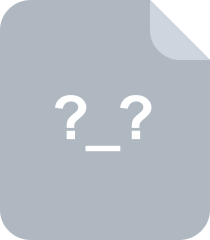
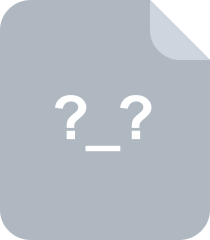
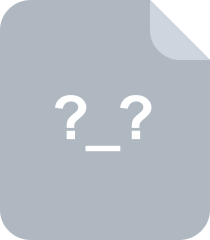
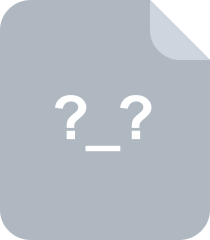
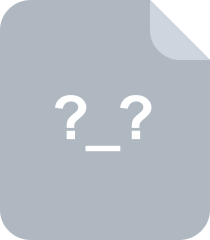
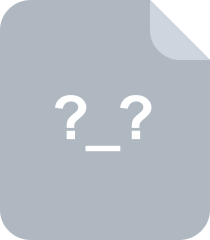
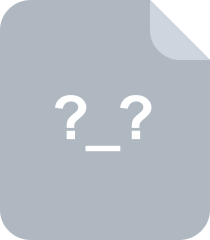
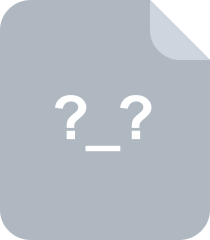
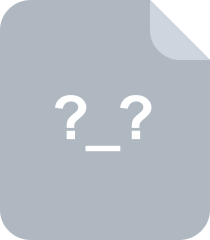
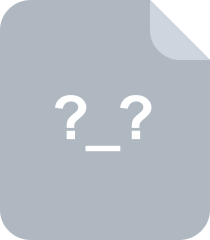
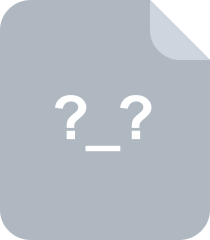
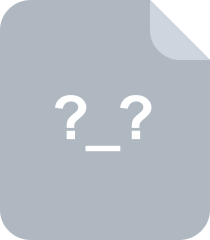
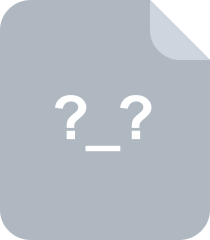
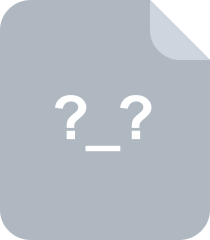
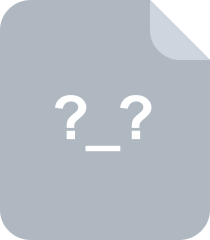
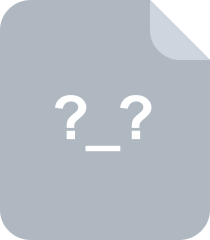
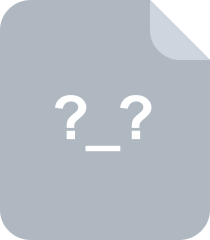
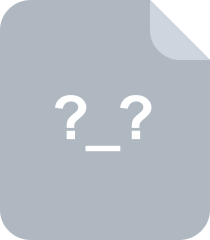
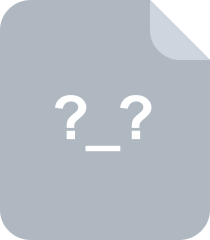
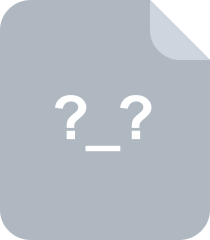
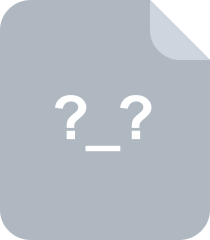
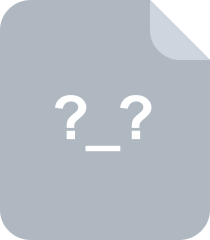
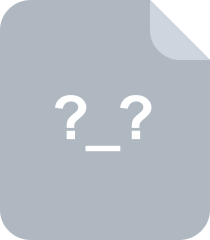
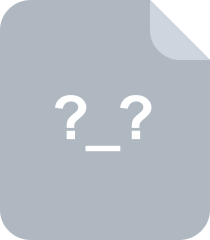
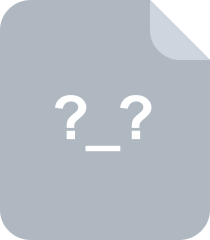
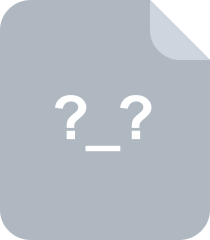
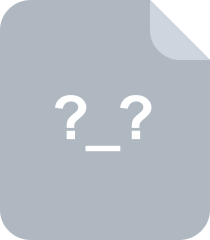
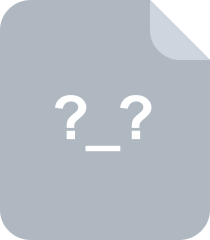
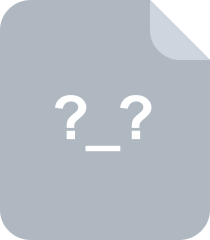
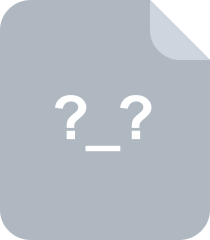
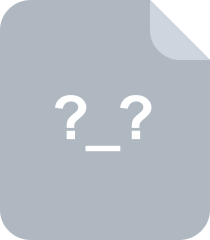
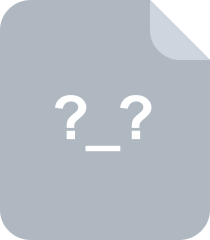
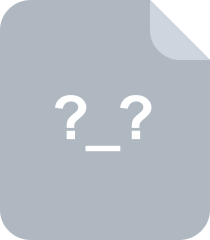
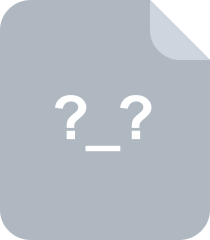
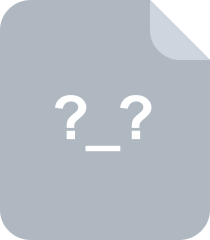
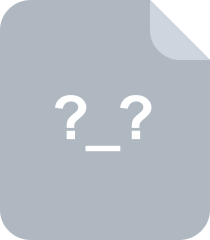
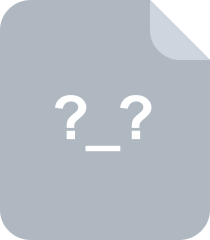
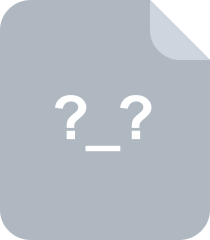
共 2000 条
- 1
- 2
- 3
- 4
- 5
- 6
- 20

布尔大学士
- 粉丝: 9w+
- 资源: 14

下载权益

C知道特权

VIP文章

课程特权

开通VIP
上传资源 快速赚钱
我的内容管理 展开
我的资源 快来上传第一个资源
我的收益
登录查看自己的收益我的积分 登录查看自己的积分
我的C币 登录后查看C币余额
我的收藏
我的下载
下载帮助

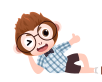
最新资源
- NSArgumentNullException如何解决.md
- VueError解决办法.md
- buvid、did参数生成算法
- tiny-cuda-cnn.zip
- 关于月度总结的PPT模板
- 手表品牌与型号数据集,手表型号数据
- 基于Java实现(IDEA)的贪吃蛇游戏-源码+jar文件+项目报告
- 数字按键3.2考试代码
- 颜色拾取器 for Windows
- 台球检测40-YOLO(v5至v11)、COCO、CreateML、Paligemma、TFRecord、VOC数据集合集.rar
- # 基于MATLAB的导航科学计算库
- Qt源码ModbusTCP 主机客户端通信程序 基于QT5 QWidget, 实现ModbusTCP 主机客户端通信,支持以下功能: 1、支持断线重连 2、通过INI文件配置自定义服务器I
- tesseract ocr 训练相关的环境部署包,包括jdk-8u331-windows-x64.exe、jTessBoxEditorFX-2.6.0.zip 等
- 好用的Linux终端管理工具,支持自定义多行脚本命令,密码保存、断链续接,SFTP等功能
- 大学毕业设计写作与答辩指南:选题、研究方法及PPT制作
- 小偏差线性化模型,航空发动机线性化,非线性系统线性化,求解线性系统具体参数,最小二乘拟合 MATLAB Simulink 航空发动机,非线性,线性,非线性系统,线性系统,最小二乘,拟合,小偏差,系统辨
资源上传下载、课程学习等过程中有任何疑问或建议,欢迎提出宝贵意见哦~我们会及时处理!
点击此处反馈


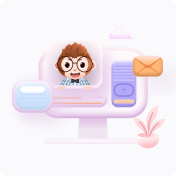
安全验证
文档复制为VIP权益,开通VIP直接复制

- 1
- 2
- 3
- 4
- 5
- 6
前往页