# Data-Augmentation-For-Wearable-Sensor-Data
This is a sample code of data augmentation methods for wearable sensor data (time-series data). The example code writen in Jupyter notebook can be found [here](https://github.com/terryum/Data-Augmentation-For-Wearable-Sensor-Data/blob/master/Example_DataAugmentation_TimeseriesData.ipynb) or [here](https://nbviewer.jupyter.org/github/terryum/Data-Augmentation-For-Wearable-Sensor-Data/blob/master/Example_DataAugmentation_TimeseriesData.ipynb). For more details, please refer to the the paper below.
T. T. Um et al., “Data augmentation of wearable sensor data for parkinson’s disease monitoring using convolutional neural networks,” in Proceedings of the 19th ACM International Conference on Multimodal Interaction, ser. ICMI 2017. New York, NY, USA: ACM, 2017, pp. 216–220. [[arXiv]](https://arxiv.org/abs/1706.00527)
## Motivation
Data augmentation is consider as a standard preprocessing in various recognition problems (e.g. image recognition), which gives additional performance improvement by providing more data. Data augmentation can be also interpreted as injecting human's prior knowledge about label-preserving transformation and giving regularization by data. This code provides a simple approach to augment time-series data, e.g., wearable sensor data, by applying various distortions to the data.
## Data augmentation examples
Please see the jupyter notebook file ([here](https://github.com/terryum/Data-Augmentation-For-Wearable-Sensor-Data/blob/master/Example_DataAugmentation_TimeseriesData.ipynb) or [here](https://nbviewer.jupyter.org/github/terryum/Data-Augmentation-For-Wearable-Sensor-Data/blob/master/Example_DataAugmentation_TimeseriesData.ipynb)) for more examples

## Dependency
You need to pre-install numpy, matplotlib, scipy, and transforms3d for running the code. (You can install them using `pip`)
## License
You can freely modify this code for your own purpose. However, please leave the citation information untouched when you redistributed the code to others. If this code helps your research, please cite the paper.
```
@inproceedings{TerryUm_ICMI2017,
author = {Um, Terry T. and Pfister, Franz M. J. and Pichler, Daniel and Endo, Satoshi and Lang, Muriel and Hirche, Sandra and Fietzek, Urban and Kuli\'{c}, Dana},
title = {Data Augmentation of Wearable Sensor Data for Parkinson's Disease Monitoring Using Convolutional Neural Networks},
booktitle = {Proceedings of the 19th ACM International Conference on Multimodal Interaction},
series = {ICMI 2017},
year = {2017},
isbn = {978-1-4503-5543-8},
location = {Glasgow, UK},
pages = {216--220},
numpages = {5},
doi = {10.1145/3136755.3136817},
acmid = {3136817},
publisher = {ACM},
address = {New York, NY, USA},
keywords = {Parkinson\&\#39;s disease, convolutional neural networks, data augmentation, health monitoring, motor state detection, wearable sensor},
}
```

快撑死的鱼
- 粉丝: 2w+
- 资源: 9156
最新资源
- Simulink整车控制器VCU模型,别人看不懂的模型,不能说别人有问题,只能说明建模型的人有问题 好的模型一定是处理恰当,可读性强的 此模型简单易懂,也是经过实车验证的 (其中自动驾驶部分已做
- Web自动化测试-ChroPath插件快速精准定位网页元素的方法与实践
- 经纬度转度分秒 方便快捷
- 分布式驱动汽车稳定性控制 采用纯Simulink模型搭建,包括控制策略和车辆动力学模型 采用分层式直接横摆力矩控制,上层包括模型预测MPC,滑模控制SMC,PID控制,LQR控制 可灵活对四种控
- 使用c++的程序设计案例
- 图幅号求算及度分秒换算
- Altium Designer PCB设计实战技巧汇总 - 提升设计效率与质量
- 基于差动制动的横摆稳定性控制 ~carsim自带驾驶员模型计算车轮转角 ~ 滑膜控制计算车辆附加差动力矩 ~ 按照差动制动分配规则分配力矩 ~ 通过仿真验证设计算法的有效性 特殊,出不 (图一三为不
- 微信小程序实现电影列表
- 快速排序算法原理及其Python实现详解-用于高效数据排序
- (文献+程序)多智能体分布式模型预测控制 编队 队形变 lunwen复现带文档 MATLAB MPC 无人车 无人机编队 无人船无人艇控制 编队控制强化学习 嵌入式应用 simulink仿真验证 PI
- 网页版 Visual Studio Code的使用.pdf
- 字节跳动DeepSeek: 先进的人工智能语言及多模态模型及其广泛应用和发展前景
- Halcon License 1月
- 七自由度车辆动力学模型+dugoff轮胎仿真模型 车身三个自由度+四个车轮滚动自由度 simulink 附带公式说明
- OpenHands: Code Less, Make More
资源上传下载、课程学习等过程中有任何疑问或建议,欢迎提出宝贵意见哦~我们会及时处理!
点击此处反馈


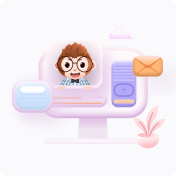