# BoseHubbardGutzwiller
[](https://doi.org/10.5281/zenodo.846904)
[](https://travis-ci.org/tcompa/BoseHubbardGutzwiller)
## What is this?
This is a simple python/cython code implementing the homogeneous Gutzwiller
variational wave function for the [Bose-Hubbard
model](https://en.wikipedia.org/wiki/Bose%E2%80%93Hubbard_model). The search
for the optimal wave-function parameters is performed through [Simulated
Annealing](https://en.wikipedia.org/wiki/Simulated_annealing), a Monte Carlo
method for stochastic optimization.
The program was developed to produce the Gutzwiller phase diagram reported in:
*Density-dependent hopping for ultracold atoms immersed in a
Bose-Einstein-condensate vortex lattice* [[arXiv:1711.10234
cond-mat.quant-gas](https://arxiv.org/abs/1711.10234)], by R. H. Chaviguri, T.
Comparin, M. Di Liberto, and M. A. Caracanhas.
If you use this code in a scientific project, please cite [the corresponding
Zenodo entry](https://zenodo.org/record/1067968):
```
@misc{tommaso_comparin_2017_1067968,
author = {Tommaso Comparin},
title = {tcompa/BoseHubbardGutzwiller v1.0.2},
year = 2017,
doi = {10.5281/zenodo.1067968},
url = {https://doi.org/10.5281/zenodo.1067968}
}
```
## How to use it?
This code requires the [numpy](http://www.numpy.org/) and
[cython](http://cython.org/) libraries (plus the
[future](https://pypi.python.org/pypi/future) library, if you need to run the
tests), and it is working on python 2.7, 3.4, 3.5 and 3.6 (elementary tests are
available in the `tests` folder, and they are performed at each commit - see
the current status on https://travis-ci.org/tcompa/BoseHubbardGutzwiller).
Before being imported in a python script, the module
`lib_gutzwiller_simulated_annealing.pyx` has to be compiled through the command
$ python setup_cython.py build_ext --inplace
After this step, it can be imported in ordinary python scripts.
Have a look at the two example files:
+ In `example_1.py`, a single simulated-annealing run is performed, and the
energy and density are computed (using the optimized Gutzwiller coefficients).
+ In `example_2.py`, a scan of different J values is performed, showing the
transition from a Mott insulator (integer density) to a superfluid.
## Notes
1. The user should play around with values of the simulated-annealing
parameters. For instance a large value of the cooling rate might increase the
chance of hitting local minima. An additional check consists in comparing the
outcome of several independent runs (each one starting with a different initial
condition for the Gutzwiller coefficients).
2. If necessary, the code can easily be optimized further. An example of a
possible change is to use the gsl random-number generator (see <a
href="http://pyinsci.blogspot.it/2010/12/efficcient-mcmc-in-python.html">here</a>),
which is not implemented in this version to avoid an additional dependency.
## License
MIT License
Copyright (c) 2017 Tommaso Comparin
Permission is hereby granted, free of charge, to any person obtaining a copy
of this software and associated documentation files (the "Software"), to deal
in the Software without restriction, including without limitation the rights
to use, copy, modify, merge, publish, distribute, sublicense, and/or sell
copies of the Software, and to permit persons to whom the Software is
furnished to do so, subject to the following conditions:
The above copyright notice and this permission notice shall be included in all
copies or substantial portions of the Software.
THE SOFTWARE IS PROVIDED "AS IS", WITHOUT WARRANTY OF ANY KIND, EXPRESS OR
IMPLIED, INCLUDING BUT NOT LIMITED TO THE WARRANTIES OF MERCHANTABILITY,
FITNESS FOR A PARTICULAR PURPOSE AND NONINFRINGEMENT. IN NO EVENT SHALL THE
AUTHORS OR COPYRIGHT HOLDERS BE LIABLE FOR ANY CLAIM, DAMAGES OR OTHER
LIABILITY, WHETHER IN AN ACTION OF CONTRACT, TORT OR OTHERWISE, ARISING FROM,
OUT OF OR IN CONNECTION WITH THE SOFTWARE OR THE USE OR OTHER DEALINGS IN THE
SOFTWARE.

快撑死的鱼
- 粉丝: 2w+
- 资源: 9157
最新资源
- 华盈恒信—金德精密—金德实业心理特征测评量表答题卡.doc
- 华盈恒信—金德精密—金德实业管理人员心理特征分析报告(发布版).ppt
- 华盈恒信—西洋肥业心理特征测评量表答题卡(1).doc
- 华盈恒信—金德精密—金德实业心理特征测评评价标准(1).doc
- 基于FPGA设计的数字时钟课程设计源码+文档说明(高分项目)
- 机械设计四轴定位装置sw18可编辑全套设计资料100%好用.zip
- 交流能力测评.doc
- 03.阿里巴巴20XX校招软件笔试题经典(含答案).doc
- 04.百度校招笔试题.doc
- 11.外企面试问题大全.doc
- 08.面谈构成表.doc
- 14.校园招聘面试小组讨论题目.doc.doc
- Java项目:校园周边美食探索(java+SpringBoot+Mybaits+Vue+elementui+mysql)
- 关于市场部拓展员面试的十大问题.doc
- 市场部经理面试技巧大全.docx
- 市场营销人员结构化面试题目.docx
资源上传下载、课程学习等过程中有任何疑问或建议,欢迎提出宝贵意见哦~我们会及时处理!
点击此处反馈


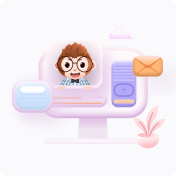