# Augmenting genetic algorithms with deep neural networks for exploring the chemical space
This repository contains code for the paper: [Augmenting genetic algorithms with deep neural networks for exploring the chemical space](https://arxiv.org/abs/1909.11655).
A video summary of the paper can be found here: https://www.youtube.com/watch?v=9VilhlEXm9w&t=16s
Here is a visualization of molecular progress:
<img align="center" src="./readme_docs/mol_view.gif"/>
## Prerequisites
For cloning the repository, please have a look at the Branch Navigator section.
Before running the code, please ensure you have the following:
- [SELFIES (any version)](https://github.com/aspuru-guzik-group/selfies) -
The code was run with v0.1.1 (which is the fastest), however, the code is compatible with any version.
- [RDKit](https://www.rdkit.org/docs/Install.html)
- [tensorboardX](https://pypi.org/project/tensorboardX/)
- [Pytorch v0.4.1](https://pytorch.org/)
- [Python 3.0 or up](https://www.python.org/download/releases/3.0/)
- [numpy](https://pypi.org/project/numpy/)
Please note: that the Synthetic Accesability calculater (i.e. directory SAS_calculator) comes from - [ https://github.com/EricTing/SAscore]( https://github.com/EricTing/SAscore).
## How to run the code? :
We highly recommend using the following version for running your experiments.
```
python ./core_GA.py
```
The following settings can be customized (found at the end of the file 'core_GA.py'):
- num_generations: Number of generations to run the GA
- generation_size: Molecular population size encountered in each generation
- starting_selfies: Initial population of molecules
- max_molecules_len: Length of the largest molecule string
- disc_epochs_per_generation: Number of epochs of training the discriminator neural network
- disc_enc_type: Type of molecular encoding shown to the discriminator
- disc_layers : Discriminator architecture
- training_start_gen: generation after which discriminator training begins
- device: Device the discriminator is trained on
- properties_calc_ls: Property evaluations to be completed for each molecule of the GA
- num_processors: Number of cpu cores to parallelize calculations over
- beta: Value of parameter beta
- impose_time_adapted_pen: Boolean variable to indicated use of a time-adapted discriminator penalty
## How are the results saved? :
All the results are savents in the 'results' directory. Our results are saved as (Note: 'i' is the run iteration):
1. images_generation_0_i:
Images of the top 100 molecules of each generation. Below each molecule are the Fitness, logP, SA, ring penalty and discriminator scores
2. results_0_i:
Each sub-directory is named by the generation. The smile strings (ordered by fitness) and corresponding molecular properties are provided as text
files: 'smiles_ordered.txt', 'logP_ordered.txt', 'sas_ordered.txt', 'ringP_ordered.txt', 'discrP_ordered.txt'.
Outside the sub-directories is the information about the best molecules of a generation.
3. saved_models_0_i:
The trained discriminators after each generation. Please Note: We did not make use of the discriminator predictions in the Fitness for this experiment (beta is set to 0).
## Branch Navigator:
The code for this repository is arranged based on the experiments of the paper. Particularly:
The code for the paper (arranged by experiment) can be found in the [paper_results branch](https://github.com/akshat998/GA/tree/paper_results). The experiments are arranged as follows:
- [Experiment 4.1: ](https://github.com/akshat998/GA/tree/paper_results/4.1) Unconstrained optimization and comparison with other generative models
- [Experiment 4.2: ](https://github.com/akshat998/GA/tree/paper_results/4.2) Long term experiment with a time-dependent adaptive penalty
- [Experiment 4.3: ](https://github.com/akshat998/GA/tree/paper_results/4.3) Analysis of molecule classes explored by the GA
- [Experiment 4.4: ](https://github.com/akshat998/GA/tree/paper_results/4.4) Constrained optimization
- [Experiment 4.5: ](https://github.com/akshat998/GA/tree/paper_results/4.5) Simultaneous logP and QED optimization
- [Experiment 4.6: ](https://github.com/akshat998/GA/tree/paper_results/4.6) Modification of the hyperparameter beta
Instructions on running the experiments of the paper are provided in the above links. Please note that the code has been parallelized based on the number of CPU cores for quick property evaluations.
To run the code quickly, we recommend the following command:
```
git clone -b master --single-branch https://github.com/aspuru-guzik-group/GA.git --depth 1
```
This contains the raw GA code, without any results from the paper. Above is very quick for cloning, and has a small file size.
Due to the large size of the repository, we have created a seperate branch that contains outputs from all the eperiment. For this option, please run (note: this is a 4GB branch, and needs 20mins of cloning time):
```
git clone --single-branch --branch paper_results https://github.com/akshat998/GA.git
```
## Questions, problems?
Make a github issue ����. Please be as clear and descriptive as possible. Please feel free to reach
out in person: (akshat[DOT]nigam[AT]mail[DOT]utoronto[DOT]ca & pascal[DOT]friederich[AT]kit[DOT]edu)
## License
[Apache License 2.0](https://choosealicense.com/licenses/apache-2.0/)

快撑死的鱼
- 粉丝: 2w+
- 资源: 9156
最新资源
- 最新算法北方苍鹰(NGO)与其他算法进行对比 2、NGO算法是2022年新出的算法 3、用几种算法跑测试函数进行对比 4、十分详细的 5、NGO算法主要与ssa、woa、pso、gwo等算法对比 ma
- 三相电压源型逆变器闭环控制仿真模型,孤岛运行 采用电压外环,电流内环的双PI控制,LCL滤波器 在对称负载和不对称负载的情况下,三相输出电压均可保持稳定 运行环境为matlab simulink
- 直流电机双闭环控制,有关直流电机控制系统仿真均
- 五相永磁同步电机矢量控制,滞环控制,弱磁控制,五相永磁同步电机Svpwm双闭环控制
- fpga实现双线性插值缩放代码及资料
- 基于matlab医学图像处理
- 非隔离双向DC DC变器 buck-boost变器仿真 输入侧为直流电压源,输出侧接蓄电池 模型采用电压外环电流内环的双闭环控制方式 正向运行时电压源给电池恒流恒压充电,反向运行时电池放电维持直流侧电
- fpga图像缩放代码及相关资料
- HX711称重,stm32c8t6内核 esp8266阿里云服务器,app上显示重量 OLED 屏幕显示 (只代码)
- 单相全桥逆变器SPWM控制模型 双极性SPWM和单极性SPWM都有 运行环境为matlab simulink
- 二极管中点钳位型三电平整流器(NPC型整流器)MATLAB Simulink仿真 电压电流双闭环控制
- FPGA实现VGA转HDMI功能的IP,配详细的接口和使用说明
- -输电线路故障行波仿真举例, -仿真由3电源和4段分布参数构成环网作为输电线路故障行波仿真平台
- 西门子S7-1200与Factory IO联合仿真程序,6x9立体仓库、双立体仓库,可实现对物的: 自动连续存功能,自动连续取功能,指定位置存功能,指定位置取功能,满仓,空仓,指定仓库有无物报警等功能
- comsol光子晶体光纤有效折射率,模式色散,有效模式面积计算
- 云计算、边缘计算-云边协同系统模型 线形搜索算法寻找最优路径 多线程并行提升系统性能 Matlab实现
资源上传下载、课程学习等过程中有任何疑问或建议,欢迎提出宝贵意见哦~我们会及时处理!
点击此处反馈


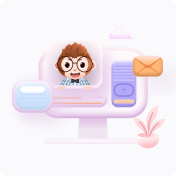