# FeatureSelectionGA
[](https://github.com/kaushalshetty/FeatureSelectionGA/actions)
[](https://pypi.python.org/pypi/feature-selection-ga/)
[](https://featureselectionga.readthedocs.io/en/latest/?badge=latest)
[](https://github.com/psf/black)
### Feature Selection using Genetic Algorithm (DEAP Framework)
Data scientists find it really difficult to choose the right features to get maximum accuracy especially if you are dealing with a lot of features. There are currenlty lots of ways to select the right features. But we will have to struggle if the feature space is really big. Genetic algorithm is one solution which searches for one of the best feature set from other features in order to attain a high accuracy.
#### Installation:
```bash
$ pip install feature-selection-ga
```
#### Documentation:
https://featureselectionga.readthedocs.io/en/latest/
#### Usage:
```python
from sklearn.datasets import make_classification
from sklearn import linear_model
from feature_selection_ga import FeatureSelectionGA, FitnessFunction
X, y = make_classification(n_samples=100, n_features=15, n_classes=3,
n_informative=4, n_redundant=1, n_repeated=2,
random_state=1)
model = linear_model.LogisticRegression(solver='lbfgs', multi_class='auto')
fsga = FeatureSelectionGA(model,X,y, ff_obj = FitnessFunction())
pop = fsga.generate(100)
#print(pop)
```
#### Usage (Advanced):
By default, the FeatureSelectionGA has its own fitness function class. We can also define our own
FitnessFunction class.
```python
class FitnessFunction:
def __init__(self,n_splits = 5,*args,**kwargs):
"""
Parameters
-----------
n_splits :int,
Number of splits for cv
verbose: 0 or 1
"""
self.n_splits = n_splits
def calculate_fitness(self,model,x,y):
pass
```
With this, we can design our own fitness function by defining our calculate fitness!
Consider the following example from [Vieira, Mendoca, Sousa, et al. (2013)](http://www.sciencedirect.com/science/article/pii/S1568494613001361)
`$f(X) = \alpha(1-P) + (1-\alpha) \left(1 - \dfrac{N_f}{N_t}\right)$`
Define the constructor **init** with needed parameters: alpha and N_t.
```python
class FitnessFunction:
def __init__(self,n_total_features,n_splits = 5, alpha=0.01, *args,**kwargs):
"""
Parameters
-----------
n_total_features :int
Total number of features N_t.
n_splits :int, default = 5
Number of splits for cv
alpha :float, default = 0.01
Tradeoff between the classifier performance P and size of
feature subset N_f with respect to the total number of features
N_t.
verbose: 0 or 1
"""
self.n_splits = n_splits
self.alpha = alpha
self.n_total_features = n_total_features
```
Next, we define the fitness function, the name has to be
calculate_fitness:
```python
def calculate_fitness(self,model,x,y):
alpha = self.alpha
total_features = self.n_total_features
cv_set = np.repeat(-1.,x.shape[0])
skf = StratifiedKFold(n_splits = self.n_splits)
for train_index,test_index in skf.split(x,y):
x_train,x_test = x[train_index],x[test_index]
y_train,y_test = y[train_index],y[test_index]
if x_train.shape[0] != y_train.shape[0]:
raise Exception()
model.fit(x_train,y_train)
predicted_y = model.predict(x_test)
cv_set[test_index] = predicted_y
P = accuracy_score(y, cv_set)
fitness = (alpha*(1.0 - P) + (1.0 - alpha)*(1.0 - (x.shape[1])/total_features))
return fitness
```
Example:
You may also see `example2.py`
```python
X, y = make_classification(n_samples=100, n_features=15, n_classes=3,
n_informative=4, n_redundant=1, n_repeated=2,
random_state=1)
# Define the model
model = linear_model.LogisticRegression(solver='lbfgs', multi_class='auto')
# Define the fitness function object
ff = FitnessFunction(n_total_features= X.shape[1], n_splits=3, alpha=0.05)
fsga = FeatureSelectionGA(model,X,y, ff_obj = ff)
pop = fsga.generate(100)
```
Example adopted from [pyswarms](https://pyswarms.readthedocs.io/en/latest/examples/usecases/feature_subset_selection.html)
没有合适的资源?快使用搜索试试~ 我知道了~
使用遗传算法进行特征选择(DEAP 框架)_python_代码_下载
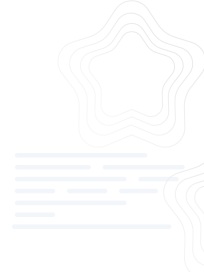
共37个文件
py:10个
md:9个
yml:7个

1.该资源内容由用户上传,如若侵权请联系客服进行举报
2.虚拟产品一经售出概不退款(资源遇到问题,请及时私信上传者)
2.虚拟产品一经售出概不退款(资源遇到问题,请及时私信上传者)
版权申诉

温馨提示
数据科学家发现很难选择正确的特征来获得最大的准确性,尤其是在处理大量特征时。目前有很多方法可以选择正确的功能。但是如果特征空间真的很大,我们将不得不挣扎。遗传算法是一种解决方案,它从其他特征中搜索最佳特征集之一以获得高精度。
资源推荐
资源详情
资源评论
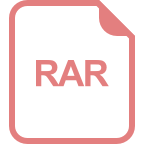
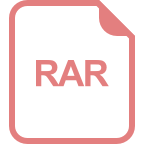
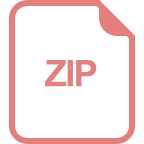
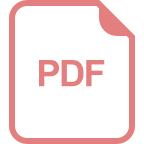
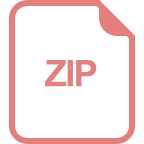
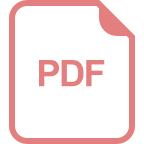
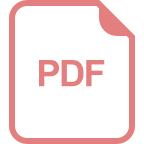
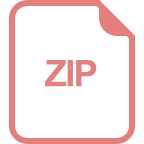
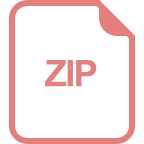
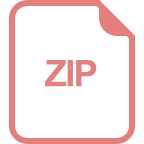
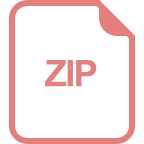
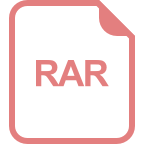
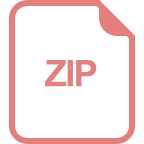
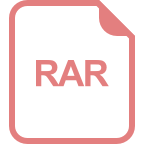
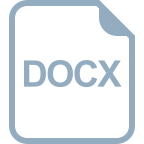
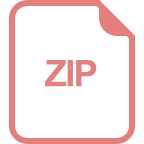
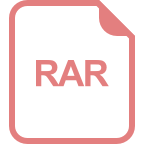
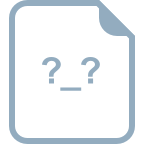
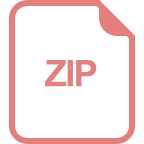
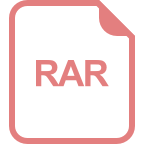
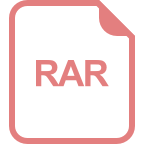
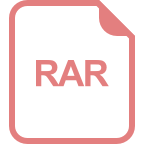
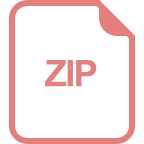
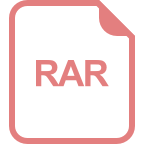
收起资源包目录


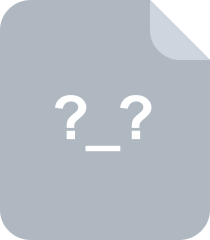

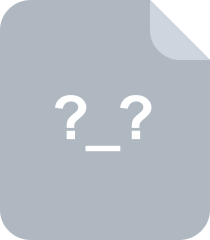
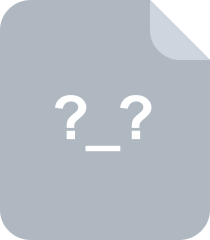
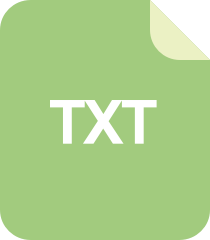
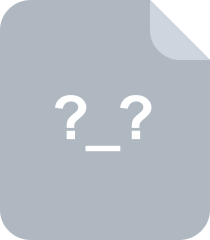

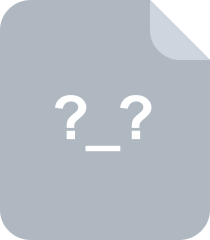
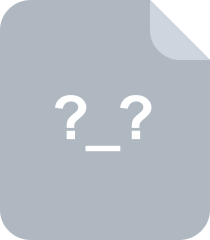
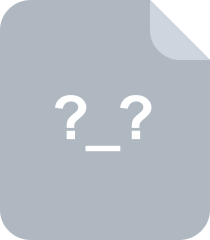
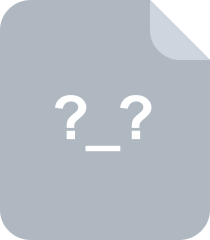
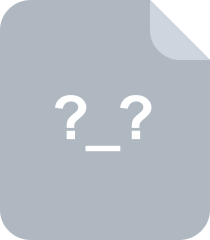
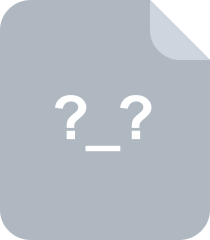

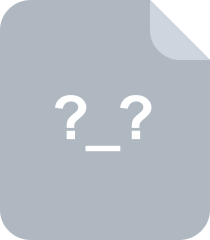
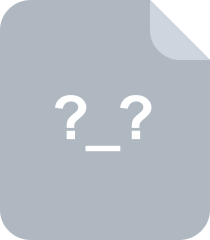

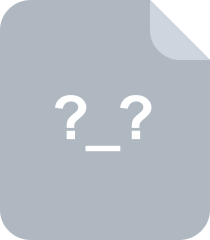
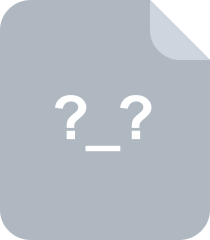
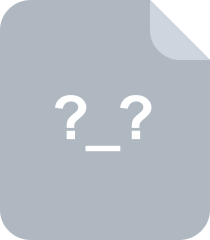
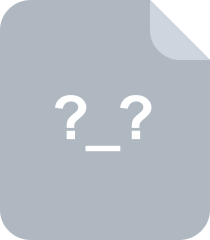

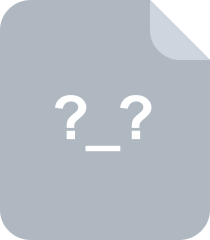
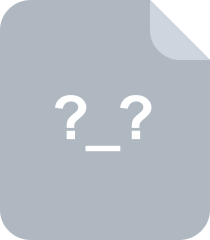
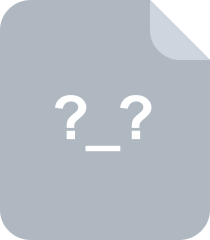
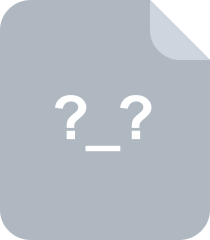
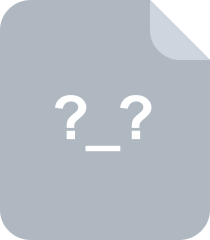

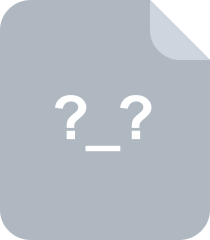
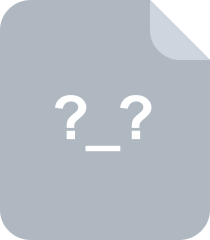
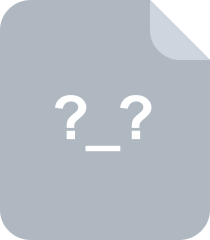
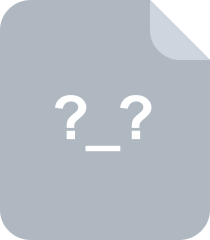

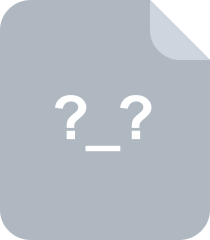
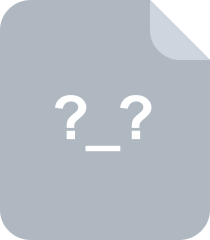
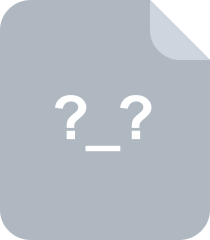
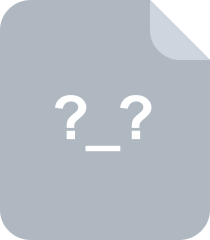
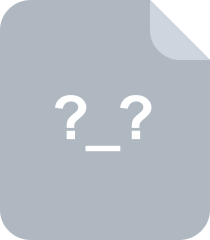
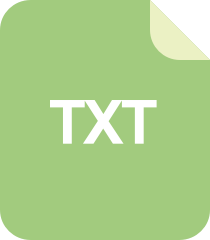
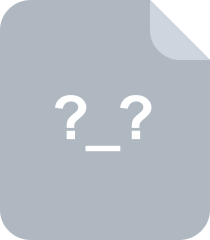
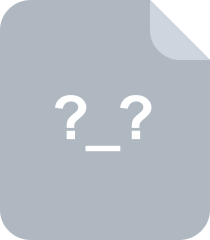
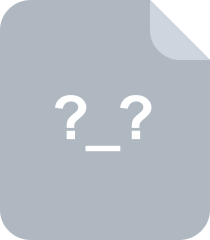
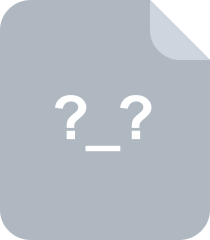
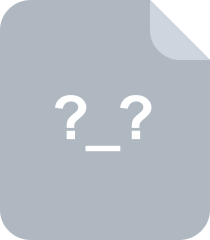
共 37 条
- 1

快撑死的鱼
- 粉丝: 1w+
- 资源: 9149

下载权益

C知道特权

VIP文章

课程特权

开通VIP
上传资源 快速赚钱
我的内容管理 展开
我的资源 快来上传第一个资源
我的收益
登录查看自己的收益我的积分 登录查看自己的积分
我的C币 登录后查看C币余额
我的收藏
我的下载
下载帮助

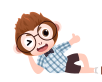
最新资源
- (源码)基于JavaWeb的学生管理系统.zip
- (源码)基于Android的VR应用转换系统.zip
- (源码)基于NetCore3.1和Vue的系统管理平台.zip
- (源码)基于Arduino的蓝牙控制LED系统.zip
- SwitchResX 4.6.4 自定义分辨率 黑苹果神器
- (源码)基于Spring Boot和MyBatis的大文件分片上传系统.zip
- (源码)基于Spring Boot和MyBatis的后台管理系统.zip
- (源码)基于JDBC的Java学生管理系统.zip
- (源码)基于Arduino的教室电力节能管理系统.zip
- (源码)基于Python语言的注释格式处理系统.zip
资源上传下载、课程学习等过程中有任何疑问或建议,欢迎提出宝贵意见哦~我们会及时处理!
点击此处反馈


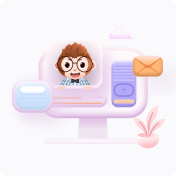
安全验证
文档复制为VIP权益,开通VIP直接复制

- 1
- 2
- 3
- 4
前往页