=============
durable_rules
=============
durable_rules is a polyglot micro-framework for real-time, consistent and scalable coordination of events. With durable_rules you can track and analyze information about things that happen (events) by combining data from multiple sources to infer more complicated circumstances.
A full forward chaining implementation (A.K.A. Rete) is used to evaluate facts and events in real time. A simple meta-linguistic abstraction lets you define simple and complex rulesets as well as control flow structures such as flowcharts, statecharts, nested statecharts and time driven flows.
The durable_rules core engine is implemented in C, which enables fast rule evaluation as well as muti-language support.
durable_rules can be scaled out by offloading state to a data store out of process such as Redis. State offloading is extensible, so you can integrate the data store of your choice.
In durable_rules V2, less is more: The Rete tree is fully evaluated in C. Thus, the framework is 5x to 10x faster (depending on the scenario) and does not require Redis. The programming model for posting events, asserting and retracting facts is synchronous and does not prescribe any web framework.
**Getting Started**
durable_rules is simple: to define a rule, all you need to do is describe the event or fact pattern to match (antecedent) and the action to take (consequent).
To install the framework do: `pip install durable_rules`
::
from durable.lang import *
with ruleset('test'):
# antecedent
@when_all(m.subject == 'World')
def say_hello(c):
# consequent
print ('Hello {0}'.format(c.m.subject))
post('test', { 'subject': 'World' })
**Forward Inference**
durable_rules super-power is the foward-chaining evaluation of rules. In other words, the repeated application of logical modus ponens(https://en.wikipedia.org/wiki/Modus_ponens) to a set of facts or observed events to derive a conclusion. The example below shows a set of rules applied to a small knowledge base (set of facts).
::
from durable.lang import *
with ruleset('animal'):
@when_all(c.first << (m.predicate == 'eats') & (m.object == 'flies'),
(m.predicate == 'lives') & (m.object == 'water') & (m.subject == c.first.subject))
def frog(c):
c.assert_fact({ 'subject': c.first.subject, 'predicate': 'is', 'object': 'frog' })
@when_all(c.first << (m.predicate == 'eats') & (m.object == 'flies'),
(m.predicate == 'lives') & (m.object == 'land') & (m.subject == c.first.subject))
def chameleon(c):
c.assert_fact({ 'subject': c.first.subject, 'predicate': 'is', 'object': 'chameleon' })
@when_all((m.predicate == 'eats') & (m.object == 'worms'))
def bird(c):
c.assert_fact({ 'subject': c.m.subject, 'predicate': 'is', 'object': 'bird' })
@when_all((m.predicate == 'is') & (m.object == 'frog'))
def green(c):
c.assert_fact({ 'subject': c.m.subject, 'predicate': 'is', 'object': 'green' })
@when_all((m.predicate == 'is') & (m.object == 'chameleon'))
def grey(c):
c.assert_fact({ 'subject': c.m.subject, 'predicate': 'is', 'object': 'grey' })
@when_all((m.predicate == 'is') & (m.object == 'bird'))
def black(c):
c.assert_fact({ 'subject': c.m.subject, 'predicate': 'is', 'object': 'black' })
@when_all(+m.subject)
def output(c):
print('Fact: {0} {1} {2}'.format(c.m.subject, c.m.predicate, c.m.object))
assert_fact('animal', { 'subject': 'Kermit', 'predicate': 'eats', 'object': 'flies' })
assert_fact('animal', { 'subject': 'Kermit', 'predicate': 'lives', 'object': 'water' })
assert_fact('animal', { 'subject': 'Greedy', 'predicate': 'eats', 'object': 'flies' })
assert_fact('animal', { 'subject': 'Greedy', 'predicate': 'lives', 'object': 'land' })
assert_fact('animal', { 'subject': 'Tweety', 'predicate': 'eats', 'object': 'worms' })
**Pattern Matching**
durable_rules provides string pattern matching. Expressions are compiled down to a DFA, guaranteeing linear execution time in the order of single digit nano seconds per character (note: backtracking expressions are not supported).
::
from durable.lang import *
with ruleset('test'):
@when_all(m.subject.matches('3[47][0-9]{13}'))
def amex(c):
print ('Amex detected {0}'.format(c.m.subject))
@when_all(m.subject.matches('4[0-9]{12}([0-9]{3})?'))
def visa(c):
print ('Visa detected {0}'.format(c.m.subject))
@when_all(m.subject.matches('(5[1-5][0-9]{2}|222[1-9]|22[3-9][0-9]|2[3-6][0-9]{2}|2720)[0-9]{12}'))
def mastercard(c):
print ('Mastercard detected {0}'.format(c.m.subject))
assert_fact('test', { 'subject': '375678956789765' })
assert_fact('test', { 'subject': '4345634566789888' })
assert_fact('test', { 'subject': '2228345634567898' })
**Business Rules and Miss Manners**
durable_rules can also be used to solve traditional Production Business Rules problems. This example is an industry benchmark. Miss Manners has decided to throw a party. She wants to seat her guests such that adjacent people are of opposite sex and share at least one hobby.
Note how the benchmark flow structure is defined using a statechart to improve code readability without sacrificing performance nor altering the combinatorics required by the benchmark. For 128 guests, 438 facts, the execution time is 600 ms.
https://github.com/jruizgit/rules/blob/master/testpy/manners.py
IMac, 4GHz i7, 32GB 1600MHz DDR3, 1.12 TB Fusion Drive
**Image recognition and Waltzdb**
Waltzdb is a constraint propagation problem for image recognition: given a set of lines in a 2D space, the system needs to interpret the 3D depth of the image. The first part of the algorithm consists of identifying four types of junctions, then labeling the junctions following Huffman-Clowes notation. Pairs of adjacent junctions constraint each other’s edge labeling. So, after choosing the labeling for an initial junction, the second part of the algorithm iterates through the graph, propagating the labeling constraints by removing inconsistent labels.
In this case too, the benchmark flow structure is defined using a statechart to improve code readability. The benchmark requirements are not altered. The execution time, for the case of 4 regions 654 ms.
https://github.com/jruizgit/rules/blob/master/testrb/waltzdb.rb
IMac, 4GHz i7, 32GB 1600MHz DDR3, 1.12 TB Fusion Drive
**Reference Manual:**
https://github.com/jruizgit/rules/blob/master/docs/py/reference.md
没有合适的资源?快使用搜索试试~ 我知道了~
温馨提示
资源分类:Python库 所属语言:Python 资源全名:durable_rules-2.0.14.tar.gz 资源来源:官方 安装方法:https://lanzao.blog.csdn.net/article/details/101784059
资源推荐
资源详情
资源评论
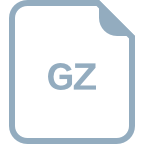
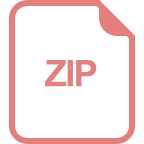
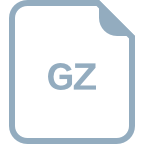
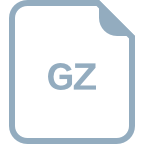
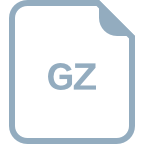
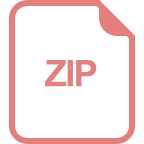
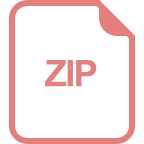
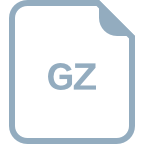
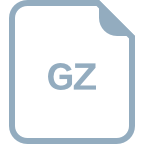
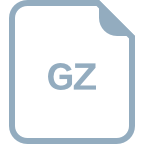
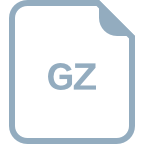
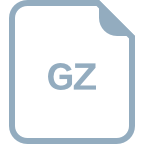
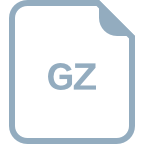
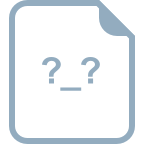
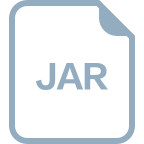
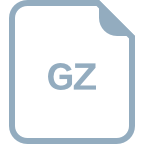
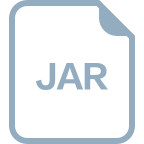
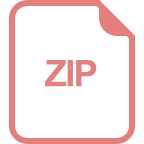
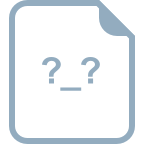
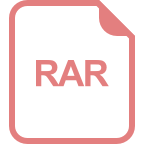
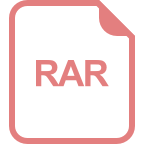
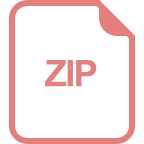
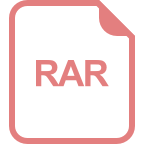
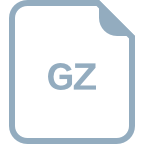
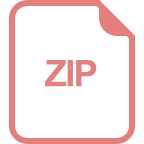
收起资源包目录


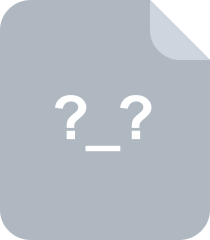
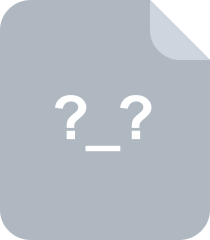


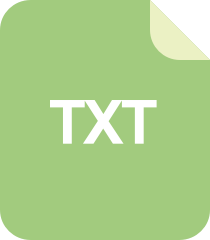


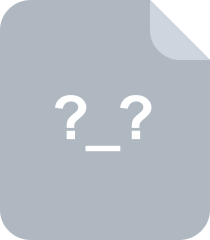
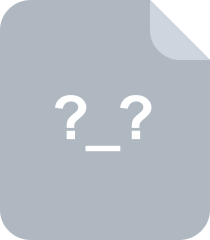
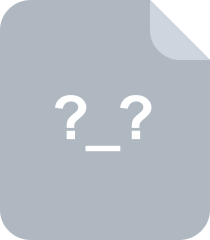

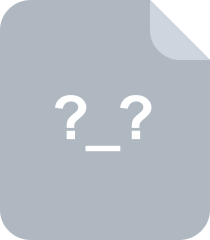
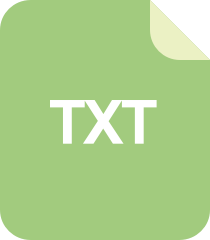
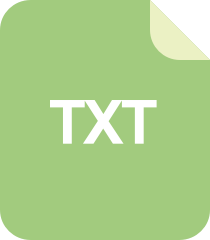
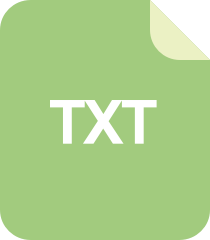
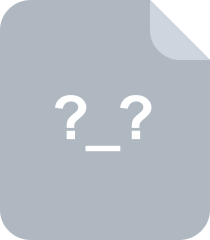


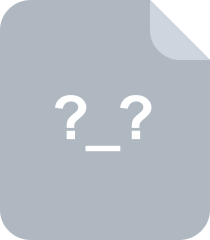
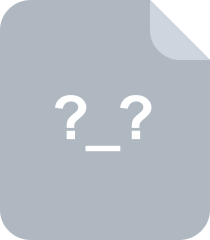
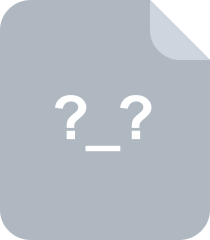
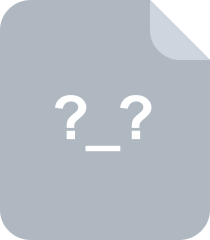
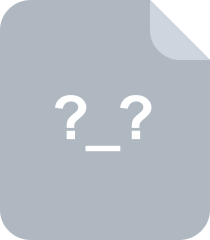
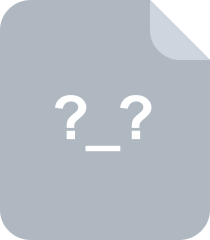
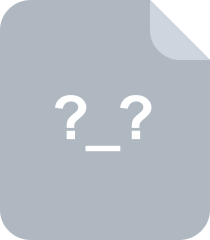
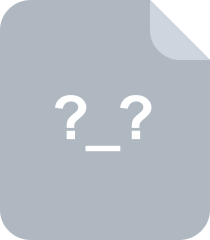
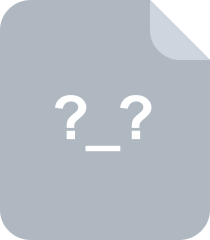
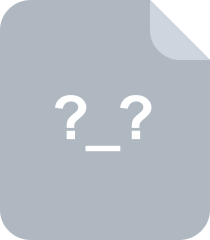

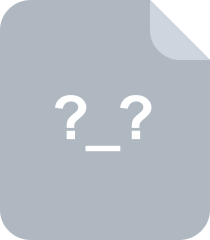
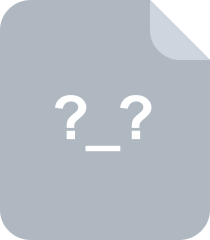
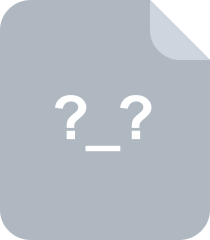
共 24 条
- 1
资源评论
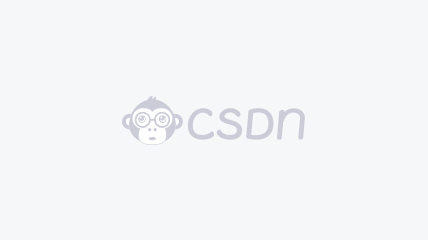

挣扎的蓝藻
- 粉丝: 14w+
- 资源: 15万+
上传资源 快速赚钱
我的内容管理 展开
我的资源 快来上传第一个资源
我的收益
登录查看自己的收益我的积分 登录查看自己的积分
我的C币 登录后查看C币余额
我的收藏
我的下载
下载帮助

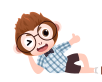
最新资源
资源上传下载、课程学习等过程中有任何疑问或建议,欢迎提出宝贵意见哦~我们会及时处理!
点击此处反馈


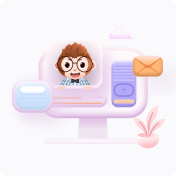
安全验证
文档复制为VIP权益,开通VIP直接复制
