from tqdm import tqdm
import pycrfsuite
from crf_tagger._fileresource import FileResource
from crf_tagger.trainer import LinePerIterationTrainer
from crf_tagger.compat import BaseEstimator
class CRF(BaseEstimator):
"""
python-crfsuite wrapper with interface siimlar to scikit-learn.
It allows to use a familiar fit/predict interface and scikit-learn
model selection utilities (cross-validation, hyperparameter optimization).
Unlike pycrfsuite.Trainer / pycrfsuite.Tagger this object is picklable;
on-disk files are managed automatically.
Parameters
----------
algorithm : str, optional (default='lbfgs')
Training algorithm. Allowed values:
* ``'lbfgs'`` - Gradient descent using the L-BFGS method
* ``'l2sgd'`` - Stochastic Gradient Descent with L2 regularization term
* ``'ap'`` - Averaged Perceptron
* ``'pa'`` - Passive Aggressive (PA)
* ``'arow'`` - Adaptive Regularization Of Weight Vector (AROW)
min_freq : float, optional (default=0)
Cut-off threshold for occurrence
frequency of a feature. CRFsuite will ignore features whose
frequencies of occurrences in the training data are no greater
than `min_freq`. The default is no cut-off.
all_possible_states : bool, optional (default=False)
Specify whether CRFsuite generates state features that do not even
occur in the training data (i.e., negative state features).
When True, CRFsuite generates state features that associate all of
possible combinations between attributes and labels.
Suppose that the numbers of attributes and labels are A and L
respectively, this function will generate (A * L) features.
Enabling this function may improve the labeling accuracy because
the CRF model can learn the condition where an item is not predicted
to its reference label. However, this function may also increase
the number of features and slow down the training process
drastically. This function is disabled by default.
all_possible_transitions : bool, optional (default=False)
Specify whether CRFsuite generates transition features that
do not even occur in the training data (i.e., negative transition
features). When True, CRFsuite generates transition features that
associate all of possible label pairs. Suppose that the number
of labels in the training data is L, this function will
generate (L * L) transition features.
This function is disabled by default.
c1 : float, optional (default=0)
The coefficient for L1 regularization.
If a non-zero value is specified, CRFsuite switches to the
Orthant-Wise Limited-memory Quasi-Newton (OWL-QN) method.
The default value is zero (no L1 regularization).
Supported training algorithms: lbfgs
c2 : float, optional (default=1.0)
The coefficient for L2 regularization.
Supported training algorithms: l2sgd, lbfgs
max_iterations : int, optional (default=None)
The maximum number of iterations for optimization algorithms.
Default value depends on training algorithm:
* lbfgs - unlimited;
* l2sgd - 1000;
* ap - 100;
* pa - 100;
* arow - 100.
num_memories : int, optional (default=6)
The number of limited memories for approximating the inverse hessian
matrix.
Supported training algorithms: lbfgs
epsilon : float, optional (default=1e-5)
The epsilon parameter that determines the condition of convergence.
Supported training algorithms: ap, arow, lbfgs, pa
period : int, optional (default=10)
The duration of iterations to test the stopping criterion.
Supported training algorithms: l2sgd, lbfgs
delta : float, optional (default=1e-5)
The threshold for the stopping criterion; an iteration stops
when the improvement of the log likelihood over the last
`period` iterations is no greater than this threshold.
Supported training algorithms: l2sgd, lbfgs
linesearch : str, optional (default='MoreThuente')
The line search algorithm used in L-BFGS updates. Allowed values:
* ``'MoreThuente'`` - More and Thuente's method;
* ``'Backtracking'`` - backtracking method with regular Wolfe condition;
* ``'StrongBacktracking'`` - backtracking method with strong Wolfe
condition.
Supported training algorithms: lbfgs
max_linesearch : int, optional (default=20)
The maximum number of trials for the line search algorithm.
Supported training algorithms: lbfgs
calibration_eta : float, optional (default=0.1)
The initial value of learning rate (eta) used for calibration.
Supported training algorithms: l2sgd
calibration_rate : float, optional (default=2.0)
The rate of increase/decrease of learning rate for calibration.
Supported training algorithms: l2sgd
calibration_samples : int, optional (default=1000)
The number of instances used for calibration.
The calibration routine randomly chooses instances no larger
than `calibration_samples`.
Supported training algorithms: l2sgd
calibration_candidates : int, optional (default=10)
The number of candidates of learning rate.
The calibration routine terminates after finding
`calibration_samples` candidates of learning rates
that can increase log-likelihood.
Supported training algorithms: l2sgd
calibration_max_trials : int, optional (default=20)
The maximum number of trials of learning rates for calibration.
The calibration routine terminates after trying
`calibration_max_trials` candidate values of learning rates.
Supported training algorithms: l2sgd
pa_type : int, optional (default=1)
The strategy for updating feature weights. Allowed values:
* 0 - PA without slack variables;
* 1 - PA type I;
* 2 - PA type II.
Supported training algorithms: pa
c : float, optional (default=1)
Aggressiveness parameter (used only for PA-I and PA-II).
This parameter controls the influence of the slack term on the
objective function.
Supported training algorithms: pa
error_sensitive : bool, optional (default=True)
If this parameter is True, the optimization routine includes
into the objective function the square root of the number of
incorrect labels predicted by the model.
Supported training algorithms: pa
averaging : bool, optional (default=True)
If this parameter is True, the optimization routine computes
the average of feature weights at all updates in the training
process (similarly to Averaged Perceptron).
Supported training algorithms: pa
variance : float, optional (default=1)
The initial variance of every feature weight.
The algorithm initialize a vector of feature weights as
a multivariate Gaussian distribution with mean 0
and variance `variance`.
Supported training algorithms: arow
gamma : float, optional (default=1)
The tradeoff between loss function and changes of feature weights.
Supported training algorithms: arow
verbose : bool, optional (default=False)
Enable trainer verbose mode.
model_filename : str, optional (default=None)
A path to an existing CRFSuite model.
This parameter allows to load and use existing crfsuite models.
By default, model files are created automatically and saved
in temporary locations; the preferred way to save/load CRF models
is to use pickle (or its alternatives like joblib).
"""
def __init__(
self,
algorithm=None,

挣扎的蓝藻
- 粉丝: 14w+
- 资源: 15万+
最新资源
- springboot基于协同过滤算法的黔醉酒业白酒销售系统_p091v--论文-springboot毕业项目,适合计算机毕-设、实训项目、大作业学习.zip
- 基于CGAN网络的二维码图像生成技术研究与实现
- 431大神PHP基于MVC三层酒店预订管理系统毕业课程源码设计
- springboot基于Spark的共享单车数据存储系统的设计与实现_71220--论文-springboot毕业项目,适合计算机毕-设、实训项目、大作业学习.zip
- springboot基于hive旅游数据的分析与应用_4x1c2--论文-springboot毕业项目,适合计算机毕-设、实训项目、大作业学习.zip
- springboot基于Web的铁路订票管理系统_w8iq4-springboot毕业项目,适合计算机毕-设、实训项目、大作业学习.zip
- 计算机毕业设计Java实现的智能二维码门禁管理系统源码+论文资料
- springboot档案管理系统_8xpgg--论文-springboot毕业项目,适合计算机毕-设、实训项目、大作业学习.zip
- nash-m-02结果.7z
- springboot+vue办公管理系统-springboot毕业项目,适合计算机毕-设、实训项目、大作业学习.zip
- springcloud房产销售平台-springboot毕业项目,适合计算机毕-设、实训项目、大作业学习.zip
- Java高校党支部党务管理系统-springboot毕业项目,适合计算机毕-设、实训项目、大作业学习.zip
- 基于龙芯的智能识别系统设计3:Python-Opencv边缘检测,输出坐标像素点
- 永磁同步电机非线性磁链观测器-示例代码 介绍: 1.该算法是对传统三段式启动方法(定位-强拖-闭环)的全新升级,可以实现零速闭环直接启动 相比三段式启动,代码大大简化 2.对于轻载启动,如风机,水
- 2025-01-07 11-24-25 的屏幕截图.png
- springboot大学生活动社团管理系统(完整运行版本)-springboot毕业项目,适合计算机毕-设、实训项目、大作业学习.zip
资源上传下载、课程学习等过程中有任何疑问或建议,欢迎提出宝贵意见哦~我们会及时处理!
点击此处反馈


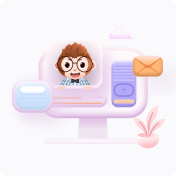