## part 1. Introduction
Implementation of YOLO v3 object detector in Tensorflow (TF-Slim). This repository is inspired by [Paweł Kapica](https://github.com/mystic123). The full details are in [this paper](https://pjreddie.com/media/files/papers/YOLOv3.pdf). In this project we cover several segments as follows:<br>
- [x] [YOLO v3 architecture](https://github.com/YunYang1994/tensorflow-yolov3/blob/master/core/yolov3.py)
- [x] Weights converter (util for exporting loaded COCO weights as TF checkpoint)
- [x] Basic working demo
- [x] Non max suppression on the both `GPU` and `CPU` is supported
- [x] Training pipeline
- [x] Compute COCO mAP
YOLO paper is quick hard to understand, along side that paper. This repo enables you to have a quick understanding of YOLO Algorithmn.
## part 2. Quick start
1. Clone this file
```bashrc
$ git clone https://github.com/YunYang1994/tensorflow-yolov3.git
```
2. You are supposed to install some dependencies before getting out hands with these codes.
```bashrc
$ cd tensorflow-yolov3
$ pip install -r ./docs/requirements.txt
```
3. Exporting loaded COCO weights as TF checkpoint(`yolov3.ckpt`) and frozen graph (`yolov3_gpu_nms.pb`) . If you don't have [yolov3.weights](https://github.com/YunYang1994/tensorflow-yolov3/releases/download/v1.0/yolov3.weights). Download and put it in the dir `./checkpoint`
```bashrc
$ python convert_weight.py --convert --freeze
```
4. Then you will get some `.pb` files in the dir `./checkpoint`, and run the demo script
```bashrc
$ python nms_demo.py
$ python video_demo.py # if use camera, set video_path = 0
```

## part 3. Train on your own dataset
Three files are required as follows:
- `dataset.txt`:
```
xxx/xxx.jpg 18.19 6.32 424.13 421.83 20 323.86 2.65 640.0 421.94 20
xxx/xxx.jpg 55.38 132.63 519.84 380.4 16
# image_path x_min y_min x_max y_max class_id x_min y_min ... class_id
```
- `anchors.txt`
```
0.10,0.13, 0.16,0.30, 0.33,0.23, 0.40,0.61, 0.62,0.45, 0.69,0.59, 0.76,0.60, 0.86,0.68, 0.91,0.76
```
- `class.names`
```
person
bicycle
car
...
toothbrush
```
### 3.1 Train raccoon dataset
To help you understand my training process, I made this training-pipline demo. [raccoon dataset](https://github.com/YunYang1994/raccoon_dataset) has only one class with 200 images (180 for train, 20 for test), I have prepared a shell script in the `./scripts` which enables you to get data and train it !
#### how to train it ?
```
$ sh scripts/make_raccoon_tfrecords.sh
$ python show_input_image.py # show your input image (optional)
$ python kmeans.py # get prior anchors and rescale the values to the range [0,1]
$ python convert_weight.py --convert # get pretrained weights
$ python quick_train.py
$ tensorboard --logdir ./data
```
As you can see in the tensorboard, if your dataset is too small or you train for too long, the model starts to overfit and learn patterns from training data that does not generalize to the test data.
#### how to test and evaluate it ?
```
$ python convert_weight.py -cf ./checkpoint/yolov3.ckpt-2500 -nc 1 -ap ./data/raccoon_anchors.txt --freeze
$ python quick_test.py
$ python evaluate.py
```
if you are still unfamiliar with training pipline, you can join [here](https://github.com/YunYang1994/tensorflow-yolov3/issues/39) to discuss with us.
|raccoon-181.jpg|raccoon-55.jpg|
|---|:---:|
|||
### 3.2 Train other dataset
Download VOC PASCAL trainval and test data
```bashrc
$ wget http://host.robots.ox.ac.uk/pascal/VOC/voc2007/VOCtrainval_06-Nov-2007.tar
$ wget http://host.robots.ox.ac.uk/pascal/VOC/voc2012/VOCtrainval_11-May-2012.tar
$ wget http://host.robots.ox.ac.uk/pascal/VOC/voc2007/VOCtest_06-Nov-2007.tar
```
Download COCO trainval and test data
```
$ wget http://images.cocodataset.org/zips/train2017.zip
$ wget http://images.cocodataset.org/annotations/annotations_trainval2017.zip
$ wget http://images.cocodataset.org/zips/test2017.zip
$ wget http://images.cocodataset.org/annotations/image_info_test2017.zip
```
## part 4. Why it is so magical ?
YOLO stands for You Only Look Once. It's an object detector that uses features learned by a deep convolutional neural network to detect an object. Although we has successfully run these codes, we must understand how YOLO works.
### 4.1 Anchors clustering
The paper suggests to use clustering on bounding box shape to find the good anchor box specialization suited for the data. more details see [here](https://nbviewer.jupyter.org/github/YunYang1994/tensorflow-yolov3/blob/master/docs/Box-Clustering.ipynb)

### 4.2 Architercutre details
In this project, I use the pretrained weights, where we have 80 trained yolo classes (COCO dataset), for recognition. And the class [label](./data/coco.names) is represented as `c` and it's integer from 1 to 80, each number represents the class label accordingly. If `c=3`, then the classified object is a `car`. The image features learned by the deep convolutional layers are passed onto a classifier and regressor which makes the detection prediction.(coordinates of the bounding boxes, the class label.. etc).details also see in the below picture. (thanks [Levio](https://blog.csdn.net/leviopku/article/details/82660381) for your great image!)

### 4.3 Neural network io:
- **input** : [None, 416, 416, 3]
- **output** : confidece of an object being present in the rectangle, list of rectangles position and sizes and classes of the objects begin detected. Each bounding box is represented by 6 numbers `(Rx, Ry, Rw, Rh, Pc, C1..Cn)` as explained above. In this case n=80, which means we have `c` as 80-dimensional vector, and the final size of representing the bounding box is 85.The first number `Pc` is the confidence of an project, The second four number `bx, by, bw, bh` represents the information of bounding boxes. The last 80 number each is the output probability of corresponding-index class.
### 4.4 Filtering with score threshold
The output result may contain several rectangles that are false positives or overlap, if your input image size of `[416, 416, 3]`, you will get `(52X52+26X26+13X13)x3=10647` boxes since YOLO v3 totally uses 9 anchor boxes. (Three for each scale). So It is time to find a way to reduce them. The first attempt to reduce these rectangles is to filter them by score threshold.
**Input arguments**:
- `boxes`: tensor of shape [10647, 4]
- `scores`: tensor of shape `[10647, 80]` containing the detection scores for 80 classes.
- `score_thresh`: float value , then get rid of whose boxes with low score
```
# Step 1: Create a filtering mask based on "box_class_scores" by using "threshold".
score_thresh=0.4
mask = tf.greater_equal(scores, tf.constant(score_thresh))
```
### 4.5 Do non-maximum suppression
Even after yolo filtering by thresholding over, we still have a lot of overlapping boxes. Second approach and filtering is Non-Maximum suppression algorithm.
* Discard all boxes with `Pc <= 0.4`
* While there are any remaining boxes :
* Pick the box with the largest `Pc`
* Output that as a prediction
* Discard any remaining boxes with `IOU>=0.5` with the box output in the previous step
In tensorflow, we can simply implement non maximum suppression algorithm like this. more details see [here](https://github.com/YunYang1994/tensorflow-yolov3/blob/master/core/utils.py)
```
for i in range(num_classes):
tf.image.non_max_suppression(boxes, score[:,i], iou_threshold=0.5)
```
Non-max suppression uses the very important function called **"Intersection over Union"**, or IoU. Here is an exmaple of non maximum suppression algorithm: on input the aglorithm receive 4 overlapping bounding boxes, and the output returns only one

Welecome to discuss with me.
## part 5. Other Implementations
[- **`YOLO
没有合适的资源?快使用搜索试试~ 我知道了~
tensorflow版本的YOLO v3,在Windows系统下亲测可运行(Python)
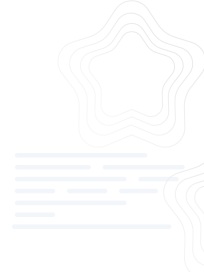
共726个文件
jpg:355个
xml:300个
py:18个


温馨提示
tensorflow版本的YOLO v3,在Windows系统下亲测可运行,代码完整,包含训练测试demo等等,上手简单容易,无需复杂的环境,tensorflow版本的YOLO v3,在Windows系统下亲测可运行,代码完整,包含训练测试demo等等,上手简单容易,无需复杂的环境,tensorflow版本的YOLO v3,在Windows系统下亲测可运行,代码完整,包含训练测试demo等等,上手简单容易,无需复杂的环境,tensorflow版本的YOLO v3,在Windows系统下亲测可运行,代码完整,包含训练测试demo等等,上手简单容易,无需复杂的环境,tensorflow版本的YOLO v3,在Windows系统下亲测可运行,代码完整,包含训练测试demo等等,上手简单容易,无需复杂的环境
资源推荐
资源详情
资源评论
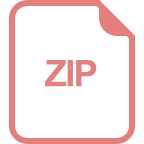
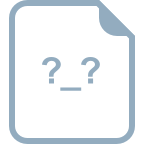
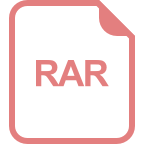
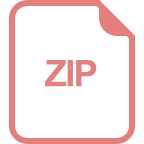
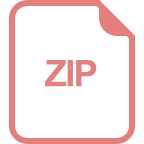
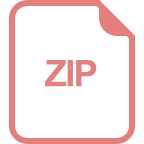
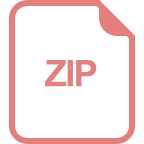
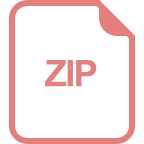
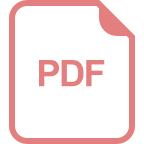
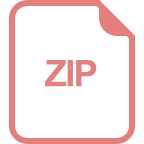
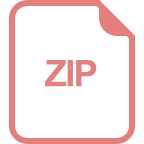
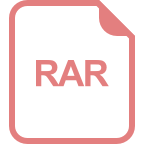
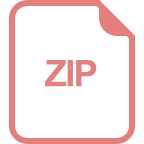
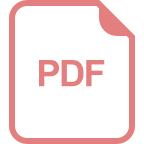
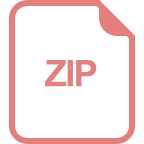
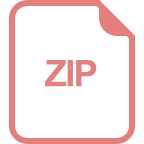
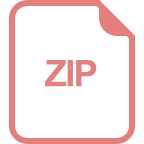
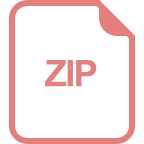
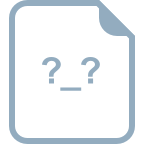
收起资源包目录

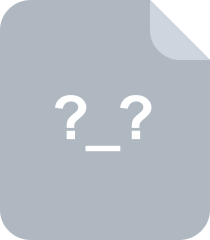
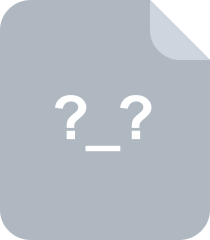
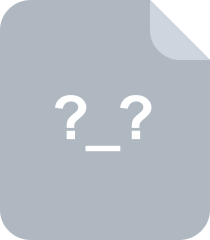
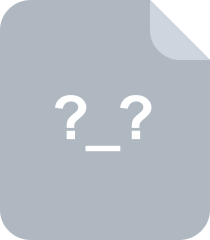
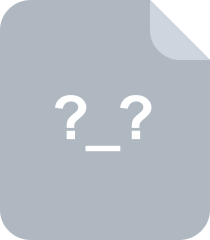
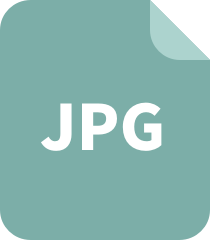
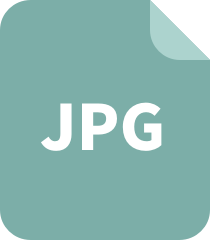
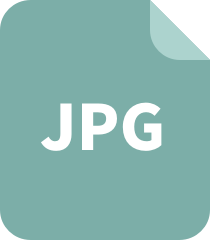
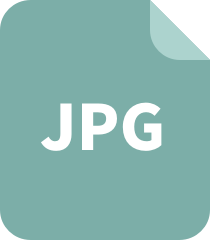
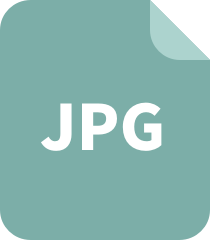
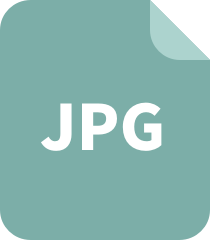
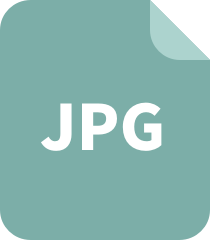
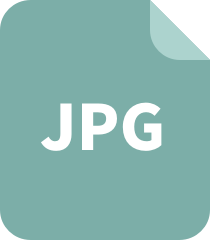
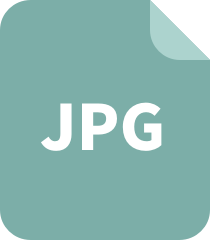
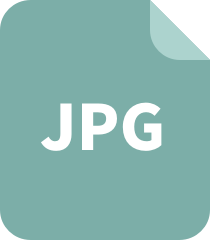
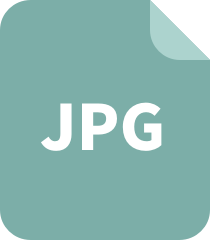
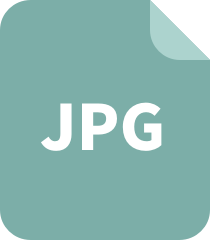
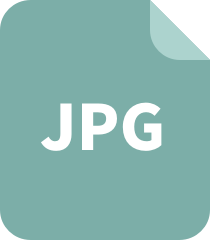
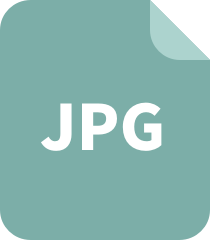
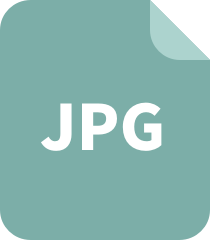
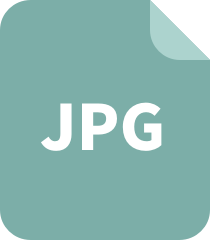
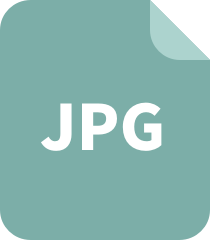
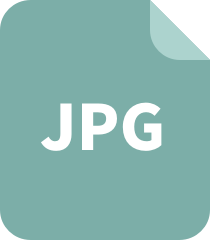
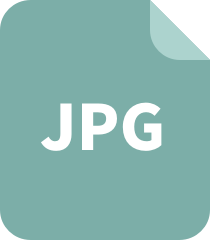
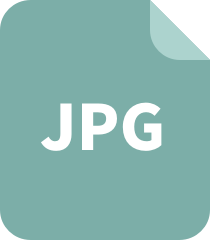
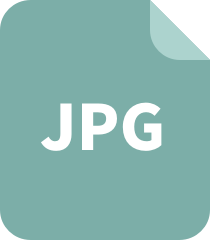
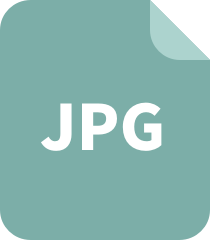
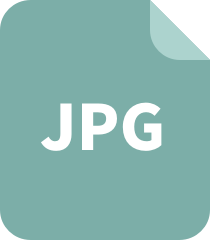
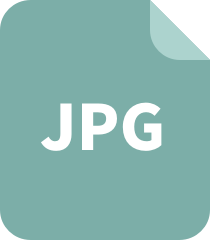
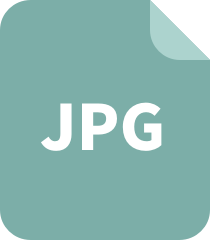
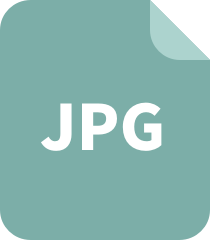
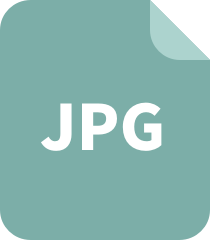
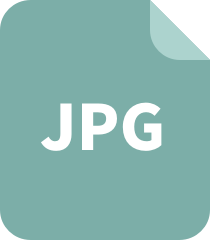
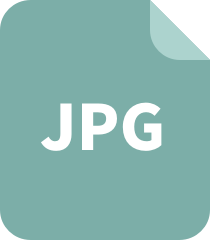
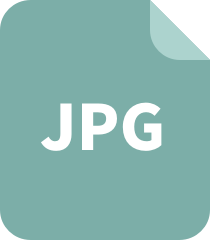
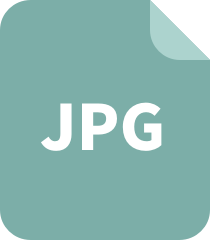
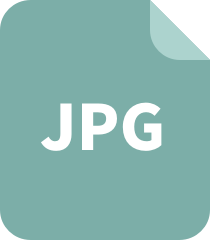
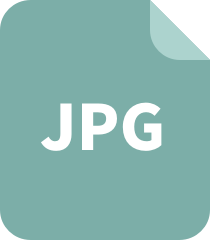
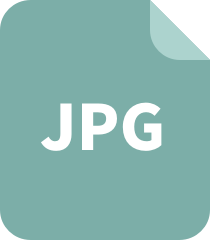
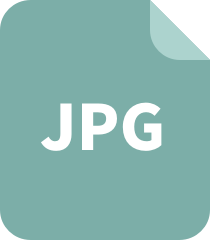
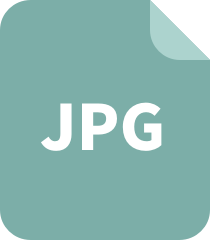
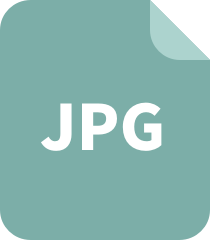
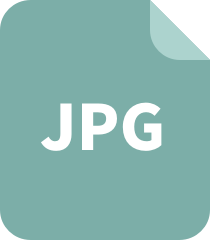
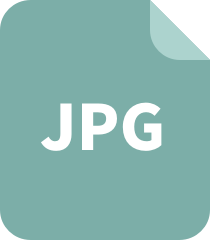
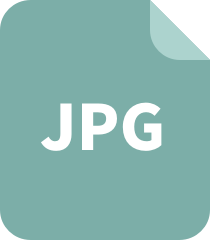
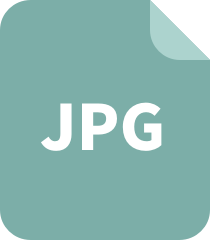
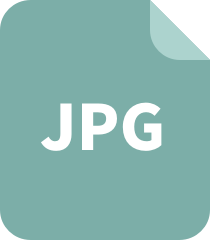
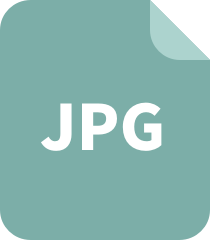
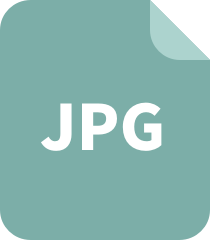
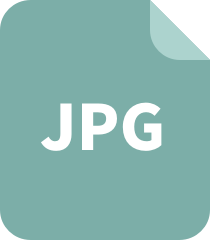
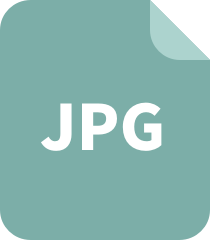
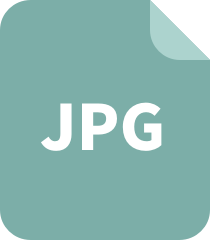
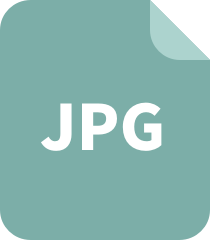
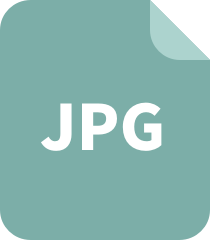
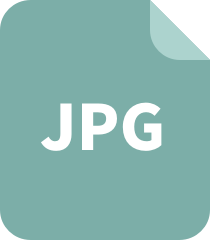
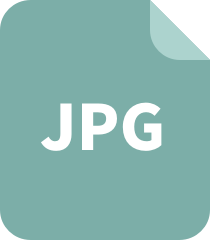
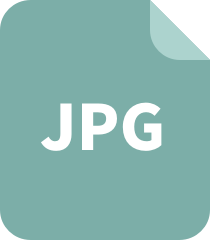
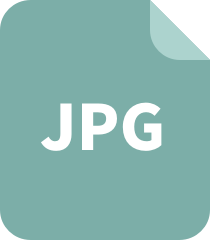
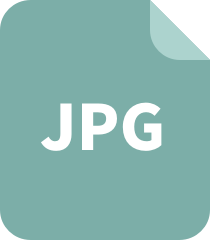
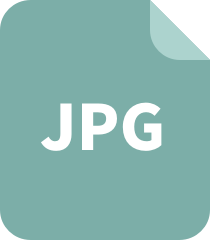
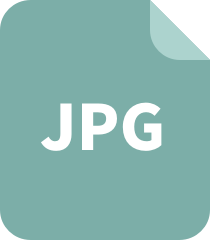
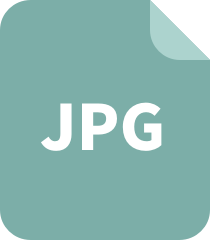
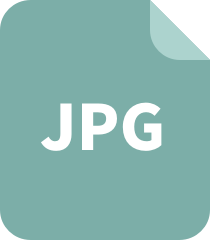
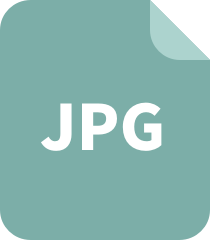
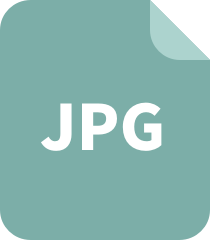
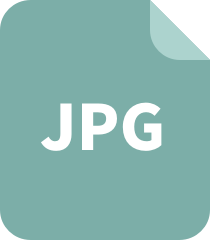
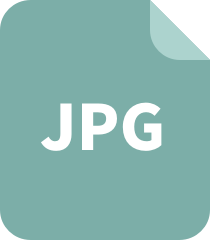
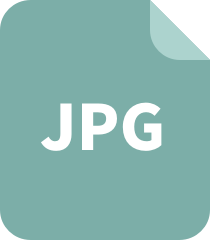
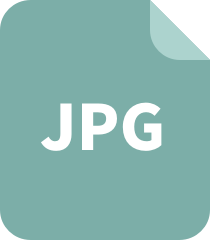
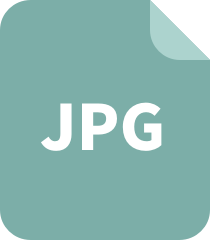
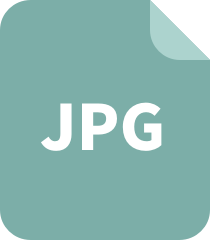
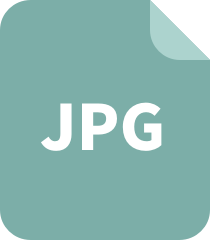
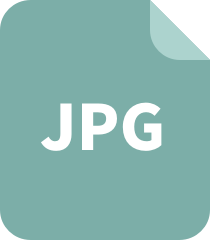
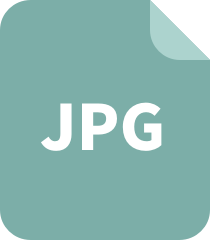
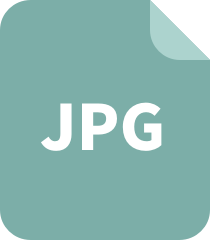
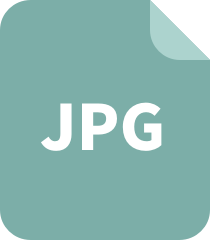
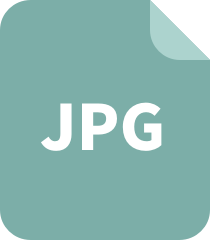
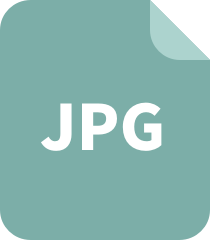
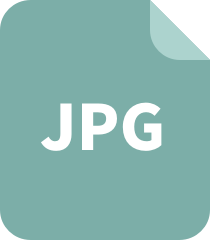
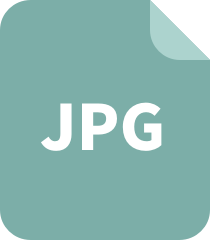
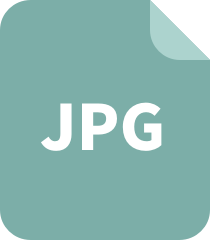
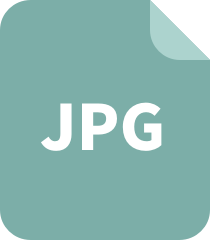
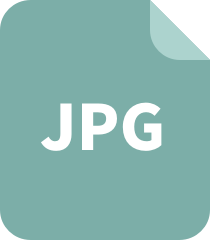
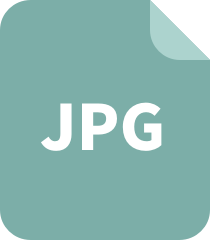
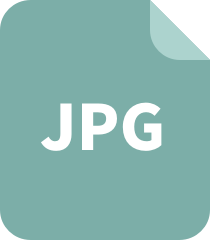
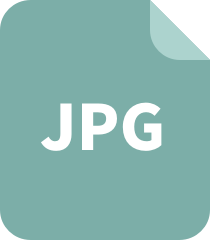
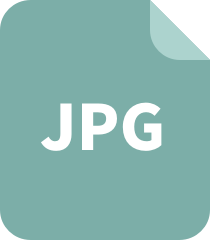
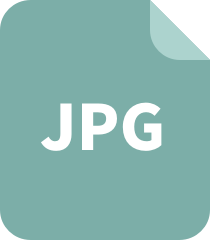
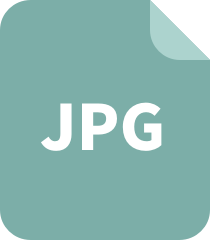
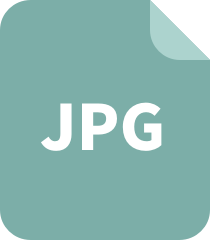
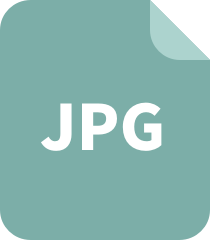
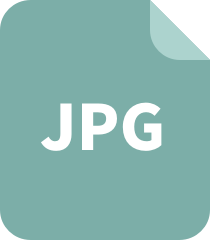
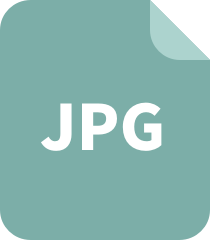
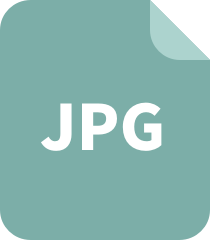
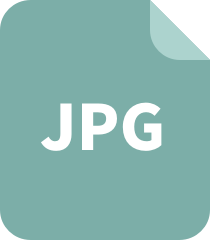
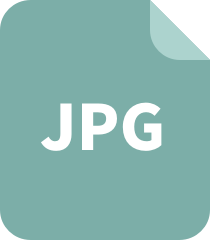
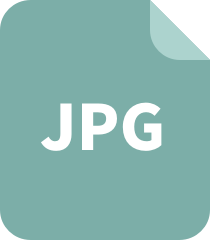
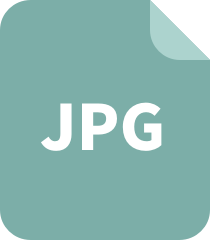
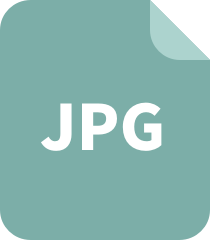
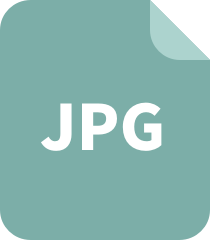
共 726 条
- 1
- 2
- 3
- 4
- 5
- 6
- 8
资源评论
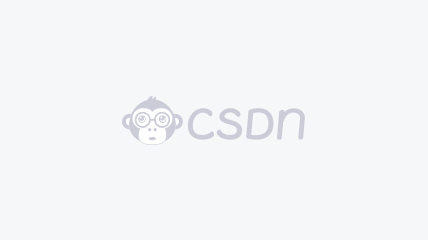
- 有梦想的人睡不着觉2021-10-13您好,您的这个tensorflow是1版本还是2版本啊
- 天马行空‘2020-03-14您好我电脑是CPU版本能够运行吗?sky___fly2020-05-15可以,我做毕设的时候就是CPU跑的
- 是孙大宝啊2019-10-25您好 您发布的tensorflow版本的YOLO v3,在Windows系统下亲测可运行(Python)这一文章 我下载下来之后无法运行啊 麻烦问一下需要怎么配置?sky___fly2019-11-12是不是没有权重文件? 你看一下报错原因,复制到百度里应该就有答案
- water_932019-07-19代码不错,可以使用

sky___fly
- 粉丝: 23
- 资源: 3
上传资源 快速赚钱
我的内容管理 展开
我的资源 快来上传第一个资源
我的收益
登录查看自己的收益我的积分 登录查看自己的积分
我的C币 登录后查看C币余额
我的收藏
我的下载
下载帮助

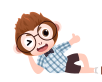
最新资源
- 基于Springboot的网上商城购物系统实现源码+数据库+文档(高分期末大作业)
- (25638822)图书馆管理系统(Servlet+Java+Jsp+Mysql)
- (22559438)基于stm32、0.96寸OLED实现的贪吃蛇小游戏(详细源码注释)
- 机械设计LOGO检测机彩盒CCD检测设备sw18可编辑非常好的设计图纸100%好用.zip
- 基于Pyotrch开发的深度学习物体分类系统(图形化界面)高分项目源码
- Java毕设-基于Springboot的网上商城购物系统实现源码+数据库+文档
- intrinsics.h
- (173873224)05 AUTOSAR行业汽车工程师资料
- 基于S7-200 PLC和组态王大小球大小分拣
- (179461246)MATLAB代码:电-气-热综合能源系统耦合优化调度 关键词:综合能源系统 优化调度 电气热耦合 仿真平台:MATLAB Y
- Kinect v2 Examples with MS-SDK 2.23
- (177300606)软件工程:概要设计说明书
- (177196812)VBA实现合并相同单元格
- (174331414)VBA实现格式相同的excel文件汇总合并
- 封装 axios 拦截器实现用户无感刷新 access-token
- 燃料电池仿真模型燃料电池仿真模型,本模型基于Cruise软件和 Simulink软件共同搭建完成,并基于实际项目搭建,本资料包包含所有源文件
资源上传下载、课程学习等过程中有任何疑问或建议,欢迎提出宝贵意见哦~我们会及时处理!
点击此处反馈


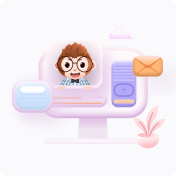
安全验证
文档复制为VIP权益,开通VIP直接复制
