<div align="center">
<img src="../resources/mmpt-logo.png" width="600"/>
<div> </div>
<div align="center">
<b><font size="5">OpenMMLab website</font></b>
<sup>
<a href="https://openmmlab.com">
<i><font size="4">HOT</font></i>
</a>
</sup>
<b><font size="5">OpenMMLab platform</font></b>
<sup>
<a href="https://platform.openmmlab.com">
<i><font size="4">TRY IT OUT</font></i>
</a>
</sup>
</div>
<div> </div>
[](https://pypi.org/project/mmpretrain)
[](https://mmpretrain.readthedocs.io/en/latest/)
[](https://github.com/open-mmlab/mmpretrain/actions)
[](https://codecov.io/gh/open-mmlab/mmpretrain)
[](https://github.com/open-mmlab/mmpretrain/blob/main/LICENSE)
[](https://github.com/open-mmlab/mmpretrain/issues)
[](https://github.com/open-mmlab/mmpretrain/issues)
[ð Documentation](https://mmpretrain.readthedocs.io/en/latest/) |
[ð ï¸ Installation](https://mmpretrain.readthedocs.io/en/latest/get_started.html#installation) |
[ð Model Zoo](https://mmpretrain.readthedocs.io/en/latest/modelzoo_statistics.html) |
[ð Update News](https://mmpretrain.readthedocs.io/en/latest/notes/changelog.html) |
[ð¤ Reporting Issues](https://github.com/open-mmlab/mmpretrain/issues/new/choose)
<img src="https://user-images.githubusercontent.com/36138628/230307505-4727ad0a-7d71-4069-939d-b499c7e272b7.png" width="400"/>
English | [ç®ä½ä¸æ](/docs/README_zh-CN.mdCN.md)
</div>
</div>
<div align="center">
<a href="https://openmmlab.medium.com/" style="text-decoration:none;">
<img src="https://user-images.githubusercontent.com/25839884/219255827-67c1a27f-f8c5-46a9-811d-5e57448c61d1.png" width="3%" alt="" /></a>
<img src="https://user-images.githubusercontent.com/25839884/218346358-56cc8e2f-a2b8-487f-9088-32480cceabcf.png" width="3%" alt="" />
<a href="https://discord.gg/raweFPmdzG" style="text-decoration:none;">
<img src="https://user-images.githubusercontent.com/25839884/218347213-c080267f-cbb6-443e-8532-8e1ed9a58ea9.png" width="3%" alt="" /></a>
<img src="https://user-images.githubusercontent.com/25839884/218346358-56cc8e2f-a2b8-487f-9088-32480cceabcf.png" width="3%" alt="" />
<a href="https://twitter.com/OpenMMLab" style="text-decoration:none;">
<img src="https://user-images.githubusercontent.com/25839884/218346637-d30c8a0f-3eba-4699-8131-512fb06d46db.png" width="3%" alt="" /></a>
<img src="https://user-images.githubusercontent.com/25839884/218346358-56cc8e2f-a2b8-487f-9088-32480cceabcf.png" width="3%" alt="" />
<a href="https://www.youtube.com/openmmlab" style="text-decoration:none;">
<img src="https://user-images.githubusercontent.com/25839884/218346691-ceb2116a-465a-40af-8424-9f30d2348ca9.png" width="3%" alt="" /></a>
<img src="https://user-images.githubusercontent.com/25839884/218346358-56cc8e2f-a2b8-487f-9088-32480cceabcf.png" width="3%" alt="" />
<a href="https://space.bilibili.com/1293512903" style="text-decoration:none;">
<img src="https://user-images.githubusercontent.com/25839884/219026751-d7d14cce-a7c9-4e82-9942-8375fca65b99.png" width="3%" alt="" /></a>
<img src="https://user-images.githubusercontent.com/25839884/218346358-56cc8e2f-a2b8-487f-9088-32480cceabcf.png" width="3%" alt="" />
<a href="https://www.zhihu.com/people/openmmlab" style="text-decoration:none;">
<img src="https://user-images.githubusercontent.com/25839884/219026120-ba71e48b-6e94-4bd4-b4e9-b7d175b5e362.png" width="3%" alt="" /></a>
</div>
## Introduction
MMPreTrain is an open source pre-training toolbox based on PyTorch. It is a part of the [OpenMMLab](https://openmmlab.com/) project.
The `main` branch works with **PyTorch 1.8+**.
### Major features
- Various backbones and pretrained models
- Rich training strategies (supervised learning, self-supervised learning, multi-modality learning etc.)
- Bag of training tricks
- Large-scale training configs
- High efficiency and extensibility
- Powerful toolkits for model analysis and experiments
- Various out-of-box inference tasks.
- Image Classification
- Image Caption
- Visual Question Answering
- Visual Grounding
- Retrieval (Image-To-Image, Text-To-Image, Image-To-Text)
https://github.com/open-mmlab/mmpretrain/assets/26739999/e4dcd3a2-f895-4d1b-a351-fbc74a04e904
## What's new
ð v1.0.0rc8 was released in 22/05/2023
- Support multiple **multi-modal** algorithms and inferencers. You can explore these features by the [gradio demo](https://github.com/open-mmlab/mmpretrain/tree/main/projects/gradio_demo)!
- Add EVA-02, Dino-V2, ViT-SAM and GLIP backbones.
- Register torchvision transforms into MMPretrain, you can now easily integrate torchvision's data augmentations in MMPretrain. See [the doc](https://mmpretrain.readthedocs.io/en/latest/api/data_process.html#torchvision-transforms)
ð v1.0.0rc7 was released in 07/04/2023
- Integrated Self-supervised learning algorithms from **MMSelfSup**, such as **MAE**, **BEiT**, etc.
- Support **RIFormer**, a simple but effective vision backbone by removing token mixer.
- Add t-SNE visualization.
- Refactor dataset pipeline visualization.
Update of previous versions
- Support **LeViT**, **XCiT**, **ViG**, **ConvNeXt-V2**, **EVA**, **RevViT**, **EfficientnetV2**, **CLIP**, **TinyViT** and **MixMIM** backbones.
- Reproduce the training accuracy of **ConvNeXt** and **RepVGG**.
- Support confusion matrix calculation and plot.
- Support **multi-task** training and testing.
- Support Test-time Augmentation.
- Upgrade API to get pre-defined models of MMPreTrain.
- Refactor BEiT backbone and support v1/v2 inference.
This release introduced a brand new and flexible training & test engine, but it's still in progress. Welcome
to try according to [the documentation](https://mmpretrain.readthedocs.io/en/latest/).
And there are some BC-breaking changes. Please check [the migration tutorial](https://mmpretrain.readthedocs.io/en/latest/migration.html).
Please refer to [changelog](https://mmpretrain.readthedocs.io/en/latest/notes/changelog.html) for more details and other release history.
## Installation
Below are quick steps for installation:
```shell
conda create -n open-mmlab python=3.8 pytorch==1.10.1 torchvision==0.11.2 cudatoolkit=11.3 -c pytorch -y
conda activate open-mmlab
pip install openmim
git clone https://github.com/open-mmlab/mmpretrain.git
cd mmpretrain
mim install -e .
```
Please refer to [installation documentation](https://mmpretrain.readthedocs.io/en/latest/get_started.html) for more detailed installation and dataset preparation.
For multi-modality models support, please install the extra dependencies by:
```shell
mim install -e ".[multimodal]"
```
## User Guides
We provided a series of tutorials about the basic usage of MMPreTrain for new users:
- [Learn about Configs](https://mmpretrain.readthedocs.io/en/latest/user_guides/config.html)
- [Prepare Dataset](https://mmpretrain.readthedocs.io/en/latest/user_guides/dataset_prepare.html)
- [Inference with existing models](https://mmpretrain.readthedocs.io/en/latest/user_guides/inference.html)
- [Train](https://mmpretrain.readthedocs.io/en/latest/user_guides/train.html)
- [Test](https://mmpretrain.readthedocs.io/en/latest/user_guides/test.html)
- [Downstream tasks](https://mmpretrain.readthedocs.io/en/latest/user_guides/downstream.html)
For more information, please refer to [our documentation](https://mmpretrain.readthedocs.io/en/latest/).
## Model zoo
Resul

白话Learning
- 粉丝: 4737
- 资源: 3119
最新资源
- 基于51单片机的密码锁 门禁系统仿真设计 实现功能: 1、密码6位,初始密码123456 2、按下按键,带有“滴”按键提示音 3、输入密码后,密码由“数字”变为“*”号 4、其余功能见下图使用说明
- 基于51单片机的串口控制系统仿真设计 实现功能: 1、 将温湿度传感器(DHT11)采集到的数据实时显示在lcd上,并通过串口显示 2、可通过串口远程控制继电器和LED的开关 PS:通过串口助手发送命
- 基于51单片机的波形发生器系统仿真设计 其它仿真设计也可加好友 实现功能: 1、通过按键切波形 可输出正弦波 方波 三角波 锯齿波 组合波(正弦波+三角波+锯齿波) 梯形波 2、切波形时,数码管显示
- 三菱自动机、自动卖机 GX Work2程序和GT Designer3程序 功能: 1、可以买5种产 2、投大于等于价格时对应的才可以 3、选择的后自动扣 4、按 币键自动金额自动清零 00
- abaqus粗糙表面随机分布建模,随机粗糙表面,高斯分布,Step通用格式
- 西门子1200和多台smart PN 通讯案例 网上西门子1200和200smart pn通讯例程都是一台smart从站,都没有讲多台从站时的配置和编程方法 本案例展示了一台1200PLC和
- 基于8086 微机原理的计算器系统仿真设计 实现功能: 1、实现加减乘除运算,并通过四位一体数码管显示 2、清零功能 包含仿真+源码 仿真软件:Proteus8.9 编程软件:Masm for Win
- 西门子S7-1500暖通空调制药厂洁净空调PLC程序案例,硬件采用西门子1500CPU+ET200SP接口IO模块,HMI采用西门子触摸屏 具体为制药厂BMS(洁净空调自控系统)医药洁净室程序,程
- 变压器温度检测系统温度报警器 1.kealc编程 2.protues仿真 3.绘图AD 要求该系统能够实时检测变压器顶层油温和绕组温度,温度超限时报警,并能实时显示当前温度值 顶层油温度规定限值:对
- 威纶通触摸屏与两台台达变频器modbus rtu通讯程序 触摸屏为mt6103ip,变频器为VFD-M,用的在线模拟,真实触摸屏只需修改com口
- 三相级联H桥逆变器仿真模型,七电平,十一电平逆变器,采用载波移相或者载波层叠的控制方法,可以提供参考文献
- 单相pfc升压斩波电路仿真,交流电源经过不控整流再经过boost升压,输出直流400v 电压闭环pi控制,含功率因数测量部分
- 单相交交变频电路仿真,负载为阻感负载,文件中附带理论说明 仿真为自己搭建,不懂得地方可以咨询讲解,便于自学和理解交交变频电路的原理 仿真中包含输出电压的傅立叶分析,可以改变负载 默认发matl
- 酒精浓度检测器 可带报告,带 proteus仿真,带keil源程序 1、根据所设计目的设置可调节的酒精浓度检测器,并通过硬件软件系统将检测的酒精浓度反应到LCD显示屏上; 2、可通过按键实现报警浓
- 钢铁厂电除尘控制系统上位机画面+博途plc程序+触摸屏画面的完整项目文件,附带eplan图纸,实际运行的项目,wincc7.5版本,博途V16,都采用结构化编程,是学习wincc画面组态和博途编程及触
- 基于51单片机的智能家居控制系统仿真设计 环境监测 实现功能: 1、通过按键可设置温湿度数据的阈值上下限,设置烟雾浓度的阈值上限 2、将温湿度传感器(DHT11)的数据实时显示在LCD上 当温湿度数
资源上传下载、课程学习等过程中有任何疑问或建议,欢迎提出宝贵意见哦~我们会及时处理!
点击此处反馈


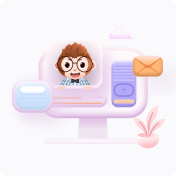