没有合适的资源?快使用搜索试试~ 我知道了~
The planner is scalable to both highway and lower-speed city driving scenarios. We also demonstrate the algorithm through scenario illustrations and on-road test results.
资源推荐
资源详情
资源评论
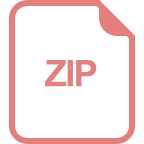
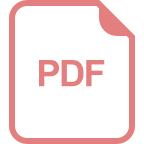
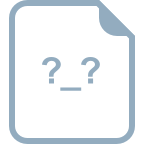
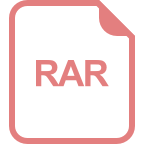
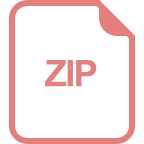
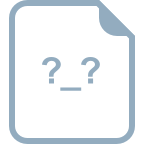
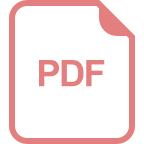
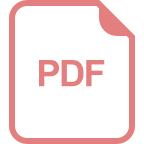
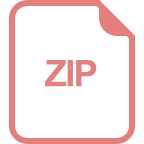
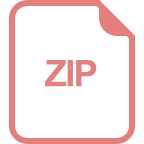
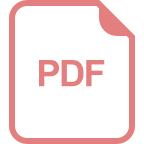
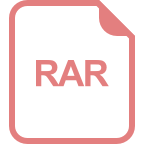
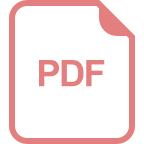
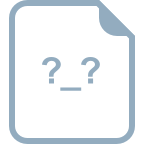
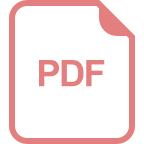
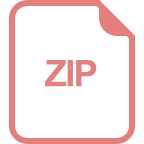
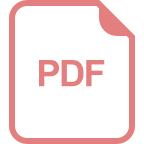
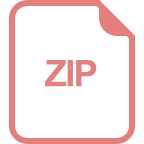
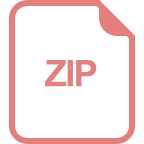
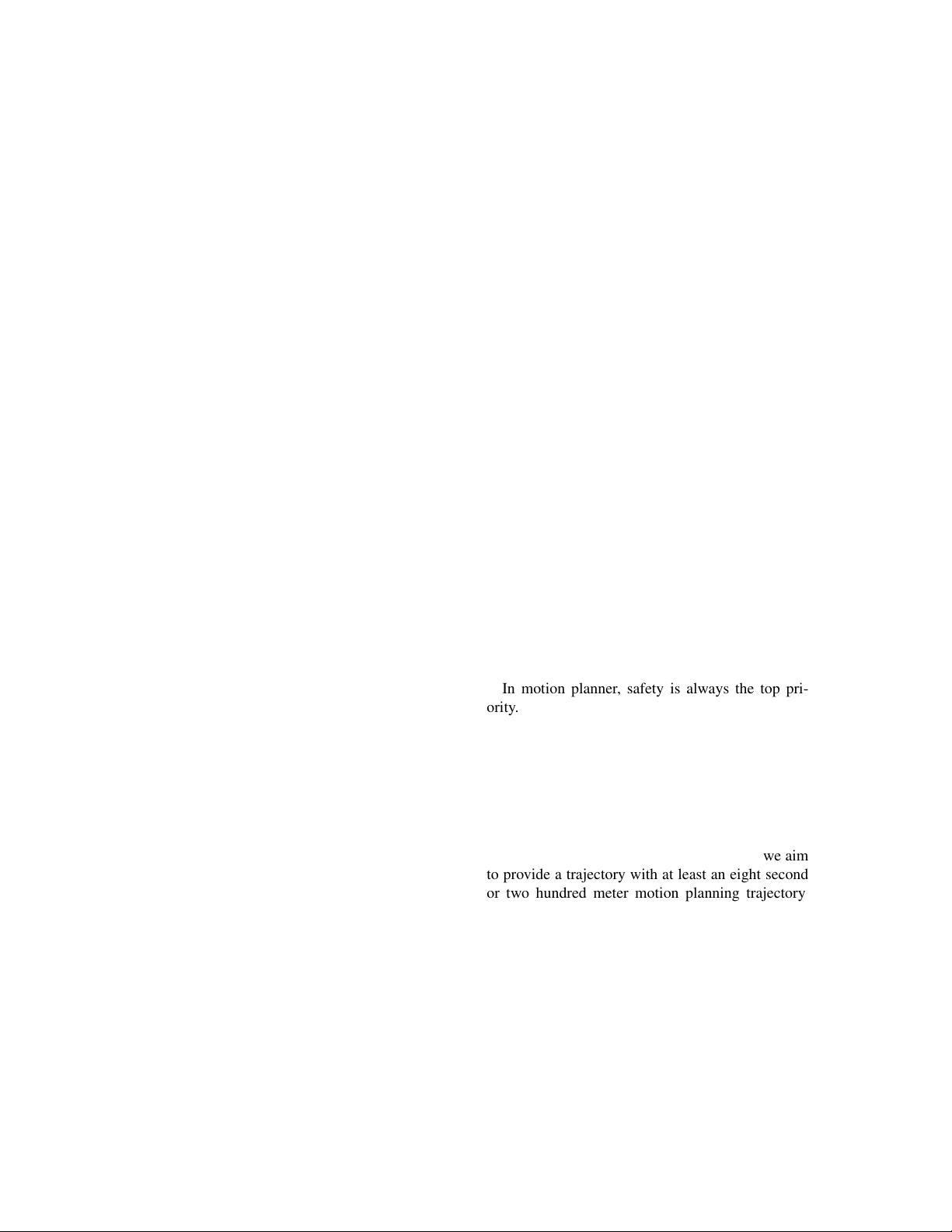
Baidu Apollo EM Motion Planner
Haoyang Fan
1,†
, Fan Zhu
2,†
, Changchun Liu, Liangliang Zhang, Li Zhuang,
Dong Li, Weicheng Zhu, Jiangtao Hu, Hongye Li, Qi Kong
3,∗
Abstract— In this manuscript, we introduce a real-
time motion planning system based on the Baidu
Apollo (open source) autonomous driving platform.
The developed system aims to address the industrial
level-4 motion planning problem while considering
safety, comfort and scalability. The system covers
multilane and single-lane autonomous driving in a
hierarchical manner: (1) The top layer of the system
is a multilane strategy that handles lane-change sce-
narios by comparing lane-level trajectories computed
in parallel. (2) Inside the lane-level trajectory gener-
ator, it iteratively solves path and speed optimization
based on a Frenet frame. (3) For path and speed
optimization, a combination of dynamic programming
and spline-based quadratic programming is proposed
to construct a scalable and easy-to-tune framework
to handle traffic rules, obstacle decisions and smooth-
ness simultaneously. The planner is scalable to both
highway and lower-speed city driving scenarios. We
also demonstrate the algorithm through scenario
illustrations and on-road test results.
The system described in this manuscript has been
deployed to dozens of Baidu Apollo autonomous
driving vehicles since Apollo v1.5 was announced in
September 2017. As of May 16th, 2018, the system
has been tested under 3,380 hours and approximately
68,000 kilometers (42,253 miles) of closed-loop au-
tonomous driving under various urban scenarios.
The algorithm described in this manuscript
is available at https://github.com/
ApolloAuto/apollo/tree/master/modules/
planning.
I. INTRODUCTION
Autonomous driving research began in the 1980s
and has significantly grown over the past ten years.
Autonomous driving aims to reduce road fatalities,
†
Authors who contributed equally in this manuscript
∗
Corresponding author for this manuscript
#
www.apollo.auto
1
Haoyang Fan is with Baidu USA LLC,
fanhaoyang01@baidu.com.
2
Fan Zhu is with Baidu USA LLC, fanzhu@baidu.com.
3
Qi Kong is with Baidu USA LLC, kongqi02@baidu.com.
increase traffic efficiency and provide convenient
travel. However, autonomous driving is a chal-
lenging task that requires accurately sensing the
environment, a deep understanding of vehicle inten-
tions and safe driving under different scenarios. To
address these difficulties, we constructed an Apollo
open source autonomous driving platform. The
flexible modularized architecture of the developed
platform supports fully autonomous driving deploy-
ment https://github.com/ApolloAuto/
apollo.
In the figure, the HD map module provides a
high-definition map that can be accessed by every
on-line module. Perception and localization mod-
ules provide the necessary dynamic environment
information, which can be further used to predict
future environment status in the prediction module.
The motion planning module considers all infor-
mation to generate a safe and smooth trajectory to
feed into the vehicle control module.
In motion planner, safety is always the top pri-
ority. We consider autonomous driving safety in,
but not limited to, the following aspects: traffic
regulations, range coverage, cycle time efficiency
and emergency safety. All these aspects are critical.
Traffic regulations are designed by governments for
public transportation safety, and such regulations
also apply to autonomous driving vehicles. An
autonomous driving vehicle should follow traffic
regulations at all times. For range coverage, we aim
to provide a trajectory with at least an eight second
or two hundred meter motion planning trajectory.
The reason is to leave enough room to maintain safe
driving within regular autonomous driving vehicle
dynamics. The execution time of the motion plan-
ning algorithm is also important. In the case of an
emergency, the system could react within 100 ms,
compared with a 300 ms reaction time for a normal
arXiv:1807.08048v1 [cs.RO] 20 Jul 2018
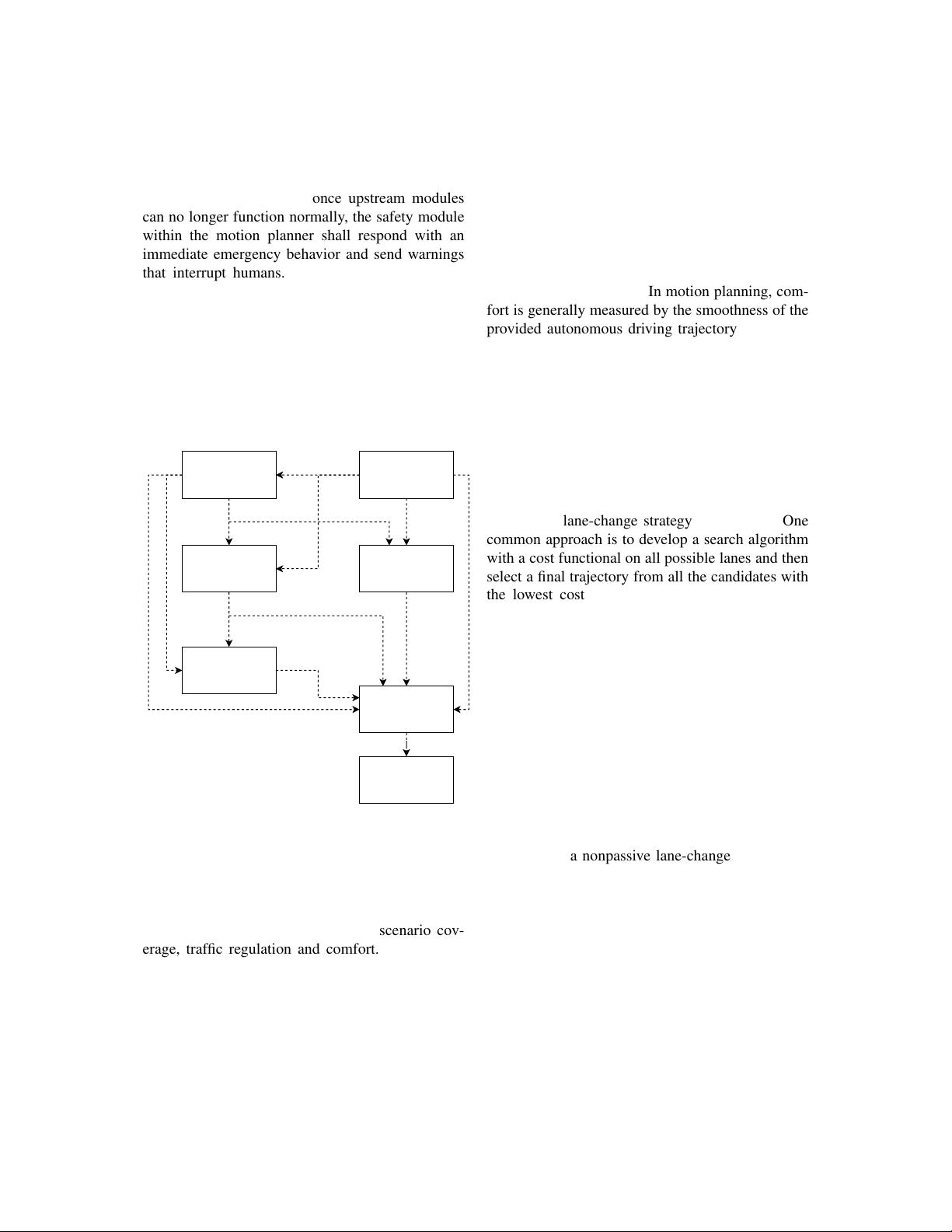
human driver. A safety emergency module is the
last shell for protecting the safety of riders. For
a level-4 motion planner, once upstream modules
can no longer function normally, the safety module
within the motion planner shall respond with an
immediate emergency behavior and send warnings
that interrupt humans. Moreover, the autonomous
driving system shall have the ability to react to
emergencies in a lower-level-like control module.
Further safety design is beyond this manuscript’s
scope.
Fig. 1 shows the architecture of the Apollo online
modules.
HD Map
Perception
Localization
Routing
Prediction
Motion
Planning
Vehicle
Control
Apollo Realtime Module Pipeline
Fig. 1: On board modules of the Apollo open source
autonomous driving platform
In addition to safety, passengers’ ride experience
is also important. The measurement of ride experi-
ence includes, but is not limited to, scenario cov-
erage, traffic regulation and comfort. For scenario
coverage, the motion planner should not only be
able to handle simple driving scenarios (e.g., stop,
nudge, yield and overtake) but also handle multi-
lane driving, heavy traffic and other complicated
on-road driving scenarios. Planning within traffic
regulations is also important for ride experience. In
addition to being a safety requirement, following
the traffic regulations will also minimize the risk of
accidents and reduce emergency reactions for au-
tonomous driving. The comfort during autonomous
driving is also important. In motion planning, com-
fort is generally measured by the smoothness of the
provided autonomous driving trajectory.
This manuscript presents the Apollo EM planner,
which is based on an EM-type iterative algorithm
([1]). This planner targets safety and ride experi-
ence with a multilane, path-speed iterative, traffic
rule and decision combined design. The intuitions
for this planner are introduced as follows:
A. Multilane Strategy
For level-4 on-road autonomous driving motion
planning, a lane-change strategy is necessary. One
common approach is to develop a search algorithm
with a cost functional on all possible lanes and then
select a final trajectory from all the candidates with
the lowest cost [2], [3]. This approach has some
difficulties. First, the search space is expanded
across multiple lanes, which causes the algorithm
to be computationally expensive. Second, traffic
regulations (e.g., right of the road, traffic lights)
are different across lanes, and it is not easy to
apply traffic regulations under the same frame.
Furthermore, trajectory stability that avoids sudden
changes between cycles should be taken into con-
sideration. It is important to follow consistent on-
road driving behavior to inform other drivers of the
intention of the autonomous driving vehicle.
Typically, a multilane strategy should cover both
nonpassive and passive lane-change scenarios. In
EM planner, a nonpassive lane-change is a request
triggered by the routing module for the purpose
of reaching the final destination. A passive lane
change is defined as an ego car maneuver when
the default lane is blocked by the dynamic en-
vironment. In both passive and nonpassive lane
changes, we aim to deliver a safe and smooth lane-
change strategy with a high success rate. Thus,
we propose a parallel framework to handle both
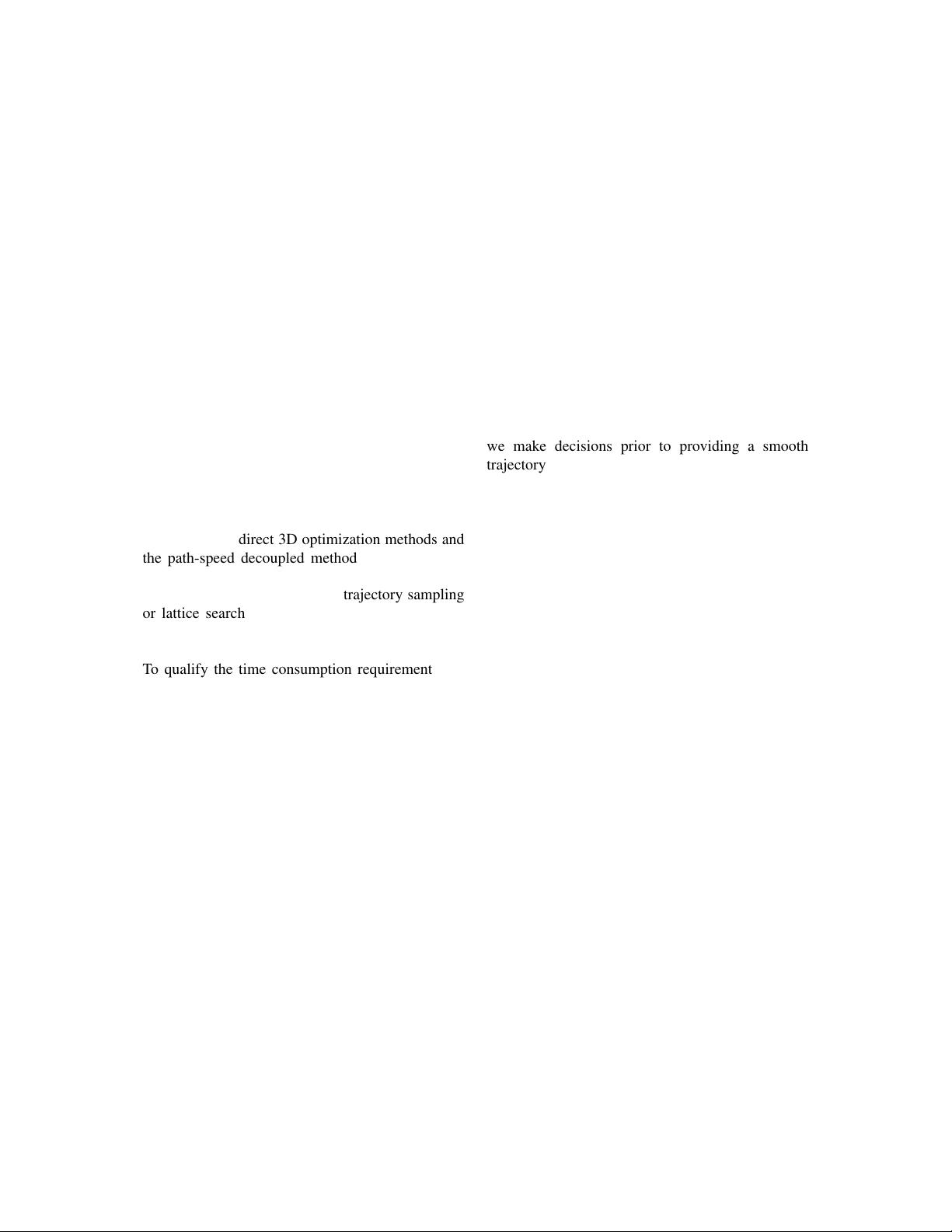
passive and nonpassive lane changes. For candidate
lanes, all obstacles and environment information are
projected on lane-based Frenet frames. Then, the
traffic regulations are bound with the given lane-
level strategy. Under this framework, each candi-
date lane will generate a best-possible trajectory
based on the lane-level optimizer. Finally, a cross-
lane trajectory decider will determine which lane to
choose based on both the cost functional and safety
rules.
B. Path-Speed Iterative Algorithm
In lane-level motion planning, optimality and
time consumption are both important. Thus, many
autonomous driving motion planning algorithms
are developed in Frenet frames with time (SLT)
to reduce the planning dimension with the help
of a reference line. Finding the optimal trajectory
in a Frenet frame is essentially a 3D constrained
optimization problem. There are typically two types
of approaches: direct 3D optimization methods and
the path-speed decoupled method. Direct methods
(e.g., [4] and [5]) attempt to find the optimal tra-
jectory within SLT using either trajectory sampling
or lattice search. These approaches are limited by
their search complexity, which increases as both
the spatial and temporal search resolutions increase.
To qualify the time consumption requirement, one
has to compromise with increasing the search grid
size or sampling resolution. Thus, the generated
trajectory is suboptimal. Conversely, the path-speed
decoupled approach optimizes path and speed sep-
arately. Path optimization typically considers static
obstacles. Then, the speed profile is created based
on the generated path [6]. It is possible that the
path-speed approach is not optimal with the ap-
pearance of dynamic obstacles. However, since
the path and speed are decoupled, this approach
achieves more flexibility in both path and speed
optimization.
EM planner optimizes path and speed iteratively.
The speed profile from the last cycle is used to
estimate interactions with oncoming and low-speed
dynamic obstacles in the path optimizer. Then, the
generated path is sent to the speed optimizer to
evaluate an optimal speed profile. For high-speed
dynamic obstacles, EM planner prefers a lane-
change maneuver rather than nudging for safety
reasons. Thus, the iterative strategy of EM planner
can help to address dynamic obstacles under the
path-speed decoupled framework.
C. Decisions and Traffic Regulations
In EM planner, decisions and traffic regulations
are two different constraints. Traffic regulations are
a non-negotiable hard constraint, whereas obstacle
yield, overtake, and nudge decisions are negotiable
based on different scenarios. For the decision-
making module, some planners directly apply nu-
merical optimization [7], [5] to make decisions
and plans simultaneously. In Apollo EM planner,
we make decisions prior to providing a smooth
trajectory. The decision process is designed to make
on-road intentions clear and reduce the search space
for finding the optimal trajectory. Many decision-
included planners attempt to generate vehicle states
as the ego car decision. These approaches can be
further divided into hand-tuning decisions ([8], [9],
[3]) and model-based decisions ([10], [11]). The
advantage of hand-tuning decision is its tunability.
However, scalability is its limitation. In some cases,
scenarios can go beyond the hand-tuning decision
rule’s description. Conversely, the model-based de-
cision approaches generally discretize the ego car
status into finite driving statuses and use data-driven
methods to tune the model. In particular, some
papers, such as [12] and [13], propose a unified
framework to handle decisions and obstacle pre-
diction simultaneously. The consideration of multi-
agent interactions will benefit both prediction and
decision-making processes.
Targeting level-4 autonomous driving, a decision
module shall include both scalability and feasibil-
ity. Scalability is the scenario expression ability
(i.e., the autonomous driving cases that can be
explained). When considering dozens of obstacles,
the decision behavior is difficult to be accurately
described by a finite set of ego car states. For
feasibility, we mean that the generated decision
shall include a feasible region in which the ego
car can maneuver within dynamic limitations. How-
ever, both hand-tuning and model-based decisions
剩余14页未读,继续阅读
资源评论
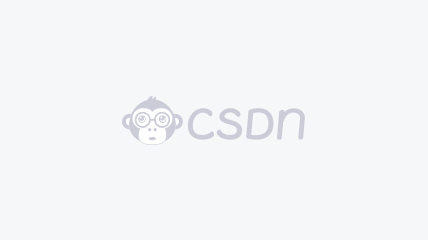

qq_32364337
- 粉丝: 0
- 资源: 2
上传资源 快速赚钱
我的内容管理 展开
我的资源 快来上传第一个资源
我的收益
登录查看自己的收益我的积分 登录查看自己的积分
我的C币 登录后查看C币余额
我的收藏
我的下载
下载帮助

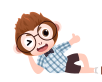
最新资源
- 音乐网站(JSP+SERVLET).rar
- 抢购软件:快速复制信息
- oracle错误代码和信息速查手册chm版最新版本
- MATLAB【逆变器二次调频模型】 微电网分布式电源逆变器DROOP控制二次调频模型,加入二次控制实现二次调频控制,及二次调压控制,程序可实现上图功能,工况有所改变 需要matlab2021A版
- 基于python的网页自动化工具项目全套技术资料100%好用.zip
- Oracle数据库命令速查手册doc版最新版本
- 程序名称:转向设计计算程序 开发平台:基于matlab平台 计算内容:阿克曼转角,转弯半径,转向阻力矩,回正力矩,转向主参数,转向传动比,力矩波动,转向梯形,EPS匹配,HPS匹配,齿轮齿条传动比,循
- 基于二阶自抗扰ADRC的轨迹跟踪控制,对车辆的不确定性和外界干扰具有一定抗干扰性,基于carsim和simulink仿真 跟踪轨迹为双移线,效果良好,有对应复现资料,是学习自抗扰技术快速入门很好的资料
- TianleSoftwareOracle学习手册中文pdf格式最新版本
- MATLAB代码:基于分布式ADMM算法的考虑碳排放交易的电力系统优化调度研究 关键词:分布式调度 ADMM算法 交替方向乘子法 碳排放 最优潮流 仿真平台:MATLAB+CPLEX GUROBI
- Oracle安装配置使用WORD文档doc格式最新版本
- 西门子840D HMI ADVANCED FOR PC 也可用于810D,840DSL中文版 1、软件可安装到台式机或笔记本上,可以连接到机床的NCU进行NC与PLC的数据备份与恢复,备份和恢复的数
- OraclePLSQL简单安装指南WORD文档doc格式最新版本
- 网页数据采集软件项目全套技术资料100%好用.zip
- Oracle高级SQL培训与讲解WORD文档doc格式最新版本
- 超智能体写的人工智能深度学习pdf
资源上传下载、课程学习等过程中有任何疑问或建议,欢迎提出宝贵意见哦~我们会及时处理!
点击此处反馈


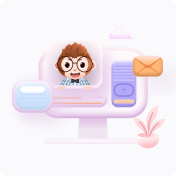
安全验证
文档复制为VIP权益,开通VIP直接复制
