没有合适的资源?快使用搜索试试~ 我知道了~
资源推荐
资源详情
资源评论
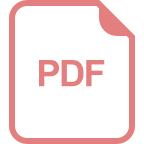
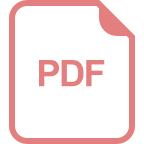
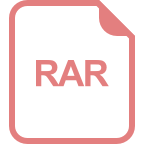
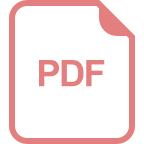
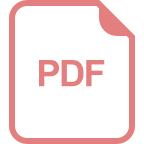
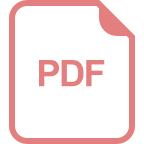
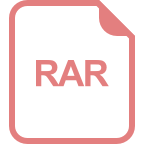
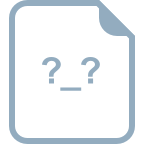
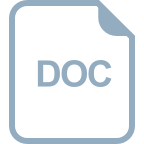
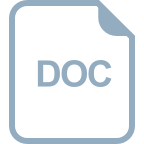
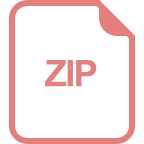
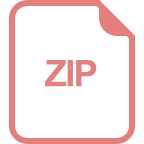
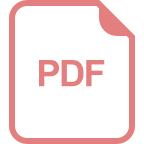
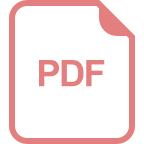
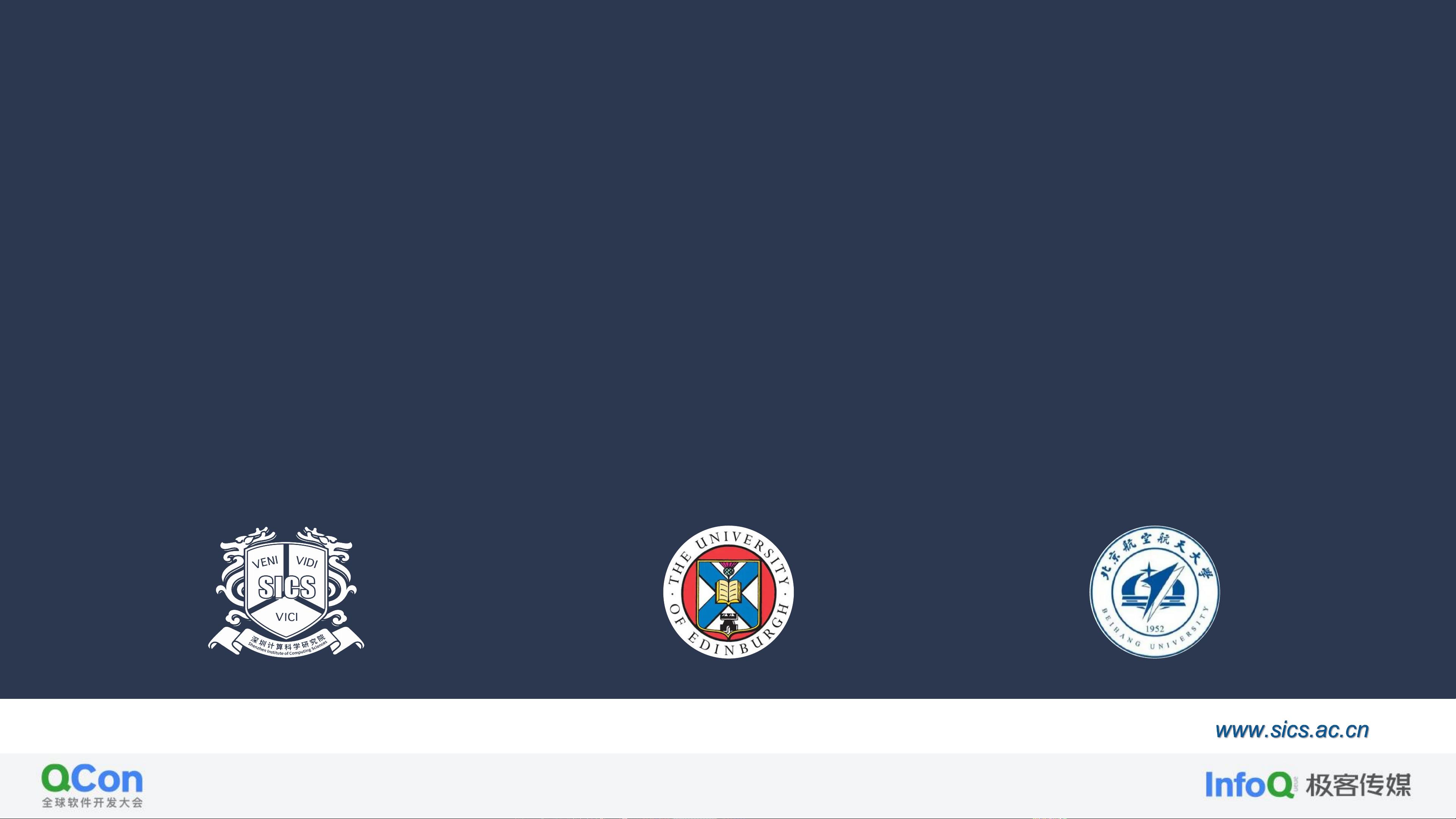
Wenfei Fan
Shenzhen Institute of Computing Sciences
University of Edinburgh
Beihang University
Big Data:
From Theory to Systems
1
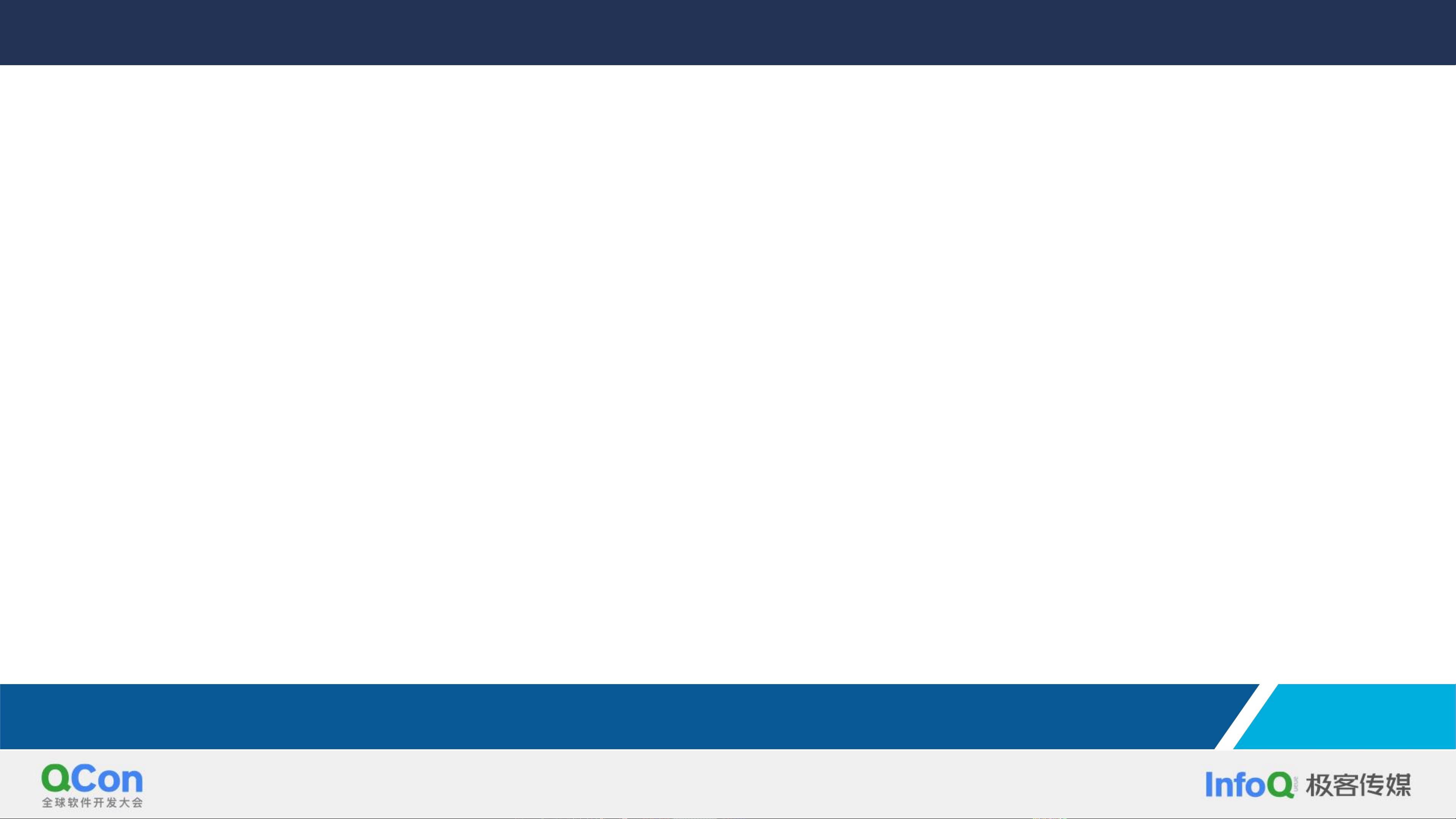
The 5 V’s of Big Data
The study has raised as many questions as it has answered
2
Volume: The size of data grows rapidly and continuously
• China generated 23.9 ZB business data in 2022. It is expected to reach 76.6 ZB in 2027
Velocity: “You cannot afford to make decisions based on yesterday’s data”
• Healthcare, retail, financial services, cyber security, …
Variety: Relational database D, transaction graph G
• Can we write a query across D and G in SQL?
Veracity: The most challenging issue among the 5V’s
• Real-life data is dirty: semantic inconsistencies, duplicates, stale data, missing links
Value : Killer APPs?
• What practical value can we get out of big data?
Big Data: Volume, Variety, Velocity, Veracity, Value
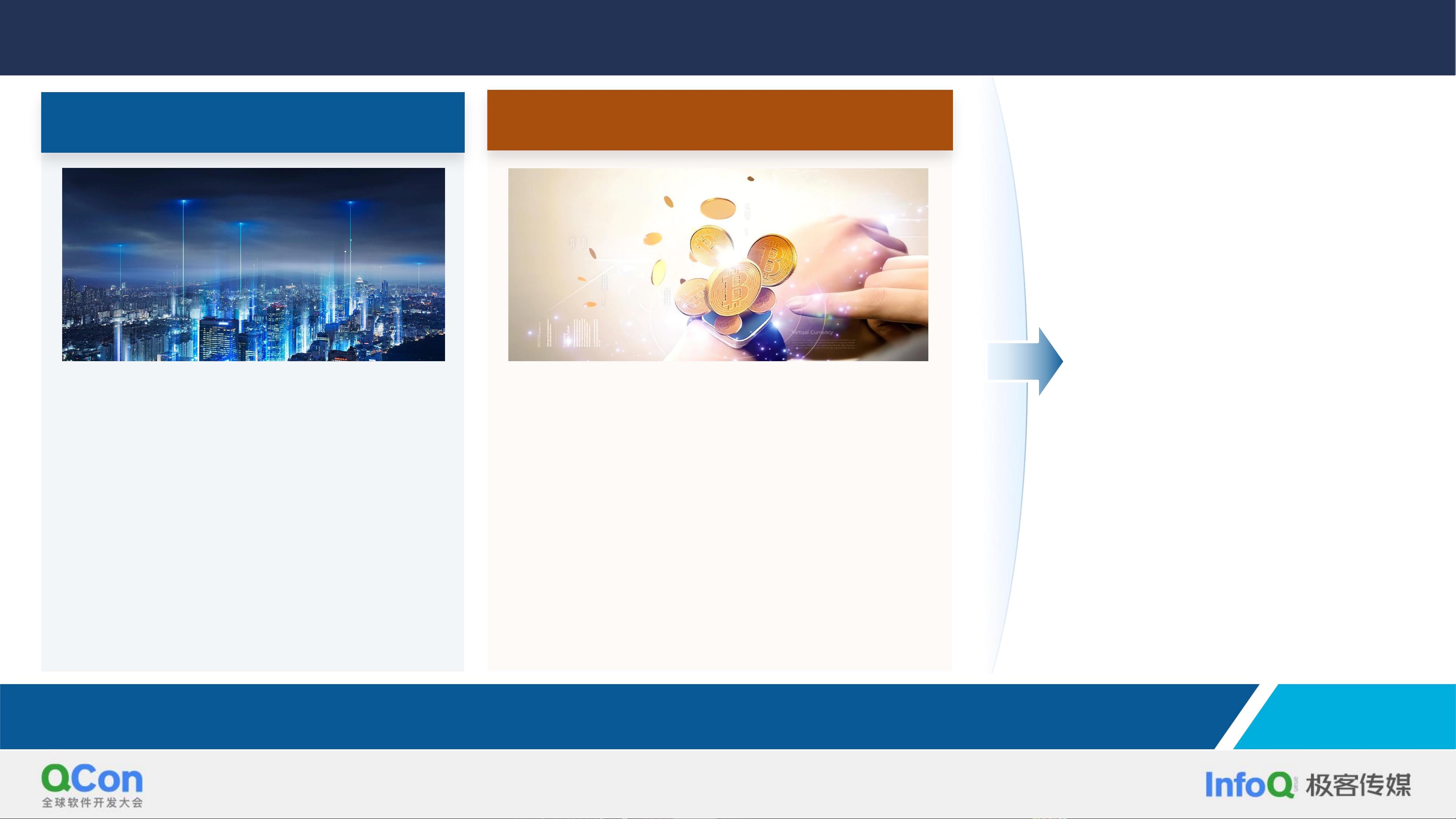
The challenges introduced by digital economy
Digital Currency
• Heterogeneous queries on big
data across different models
• Real-time transaction
processing with consistency
and reliability requirements
• Data-driven fraud detection
and intelligent analysis
Challenges:
How to query big data
with limited resources?
Volume
How to answer queries
across heterogeneous
data models? Variety
How to query dynamic
data in response to
updates? Velocity
How to clean dirty
data? Veracity
What is benefit of big
data analytics? Value
Smart City
• Fusion of data from various
models (historical BIM/CIM;
and newly collected data)
• Massive data from
unreliable data sources
• Real-time analysis in
response to updates
The need for both theory and systems for big data analytics
3
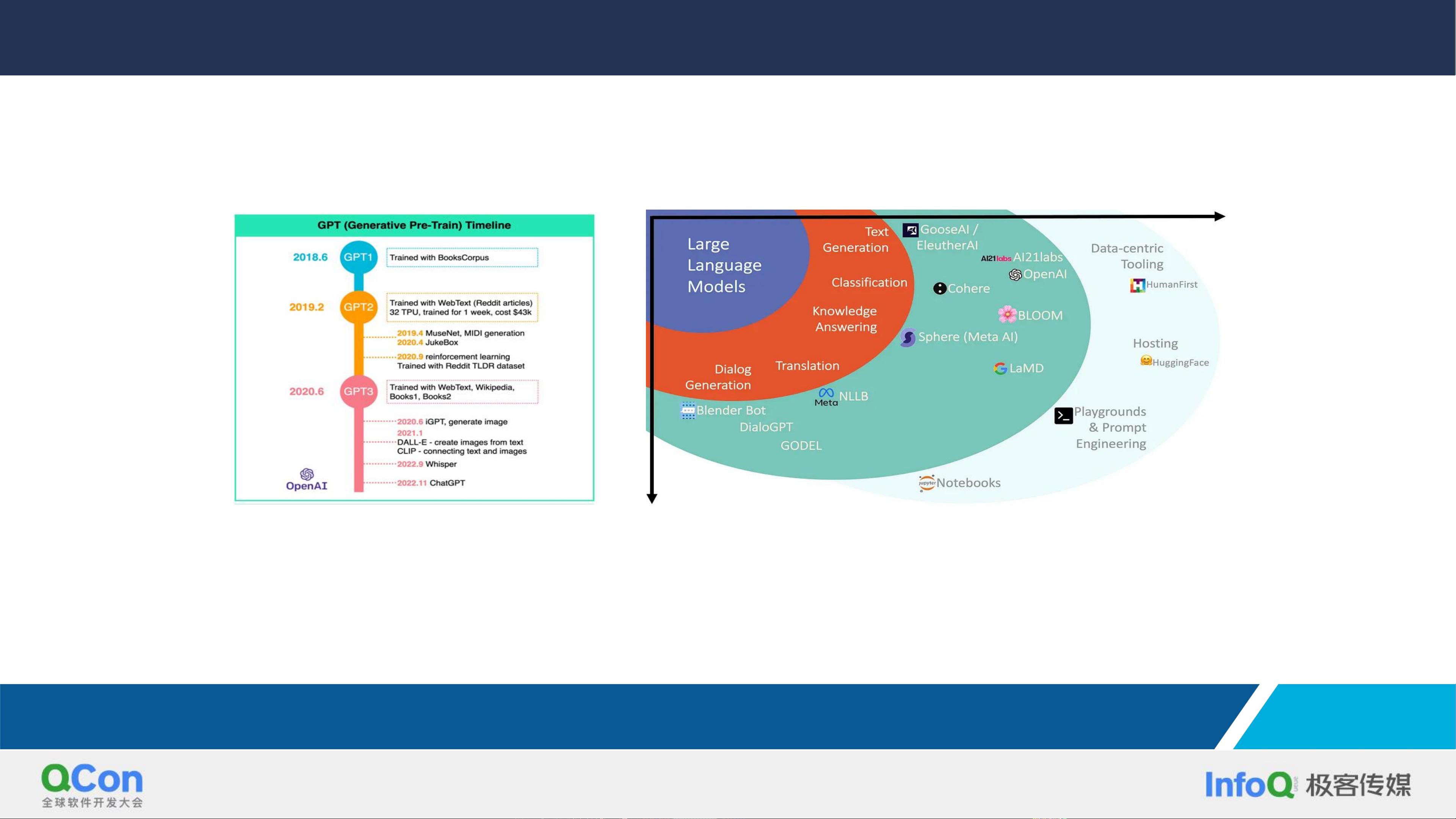
The challenges introduced by AIGC
• ChatGPT has led to a large number of AIGC startups
• 73% startups in China focus on application domains, and 14% on LLMs.
• Most LLMs are developed via fine-tuning of open-source pre-trained models.
To make practical use of AIGC
The next step: LLMs for specific application domains. But
Where can we get high-quality data in a specific domain for LLM training?
How can we make LLMs accurate, fair and robust?
Can we interprete ML predictions after all?
4
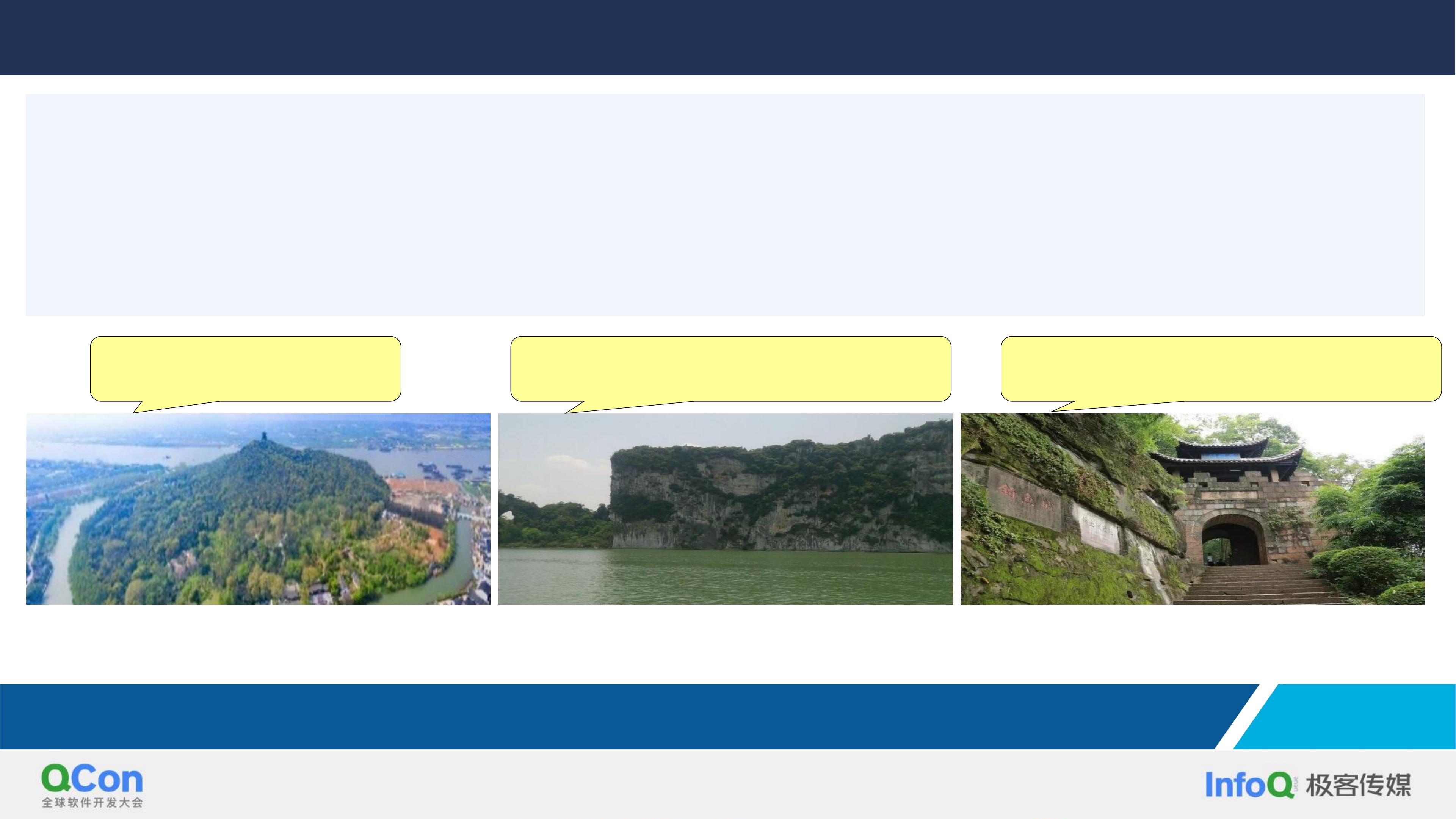
Shenzhen Institute of Computing Sciences
• 500+ people, 87% are experienced engineers
• 3 systems and 5 products since 2019
• 95+ papers in TODS, VLDBJ, SIGMOD, VLDB, ICDE, etc;
60% of the techniques proposed in the papers have been implemented in the systems
The systems developed at SICS
Rock: Data quality Yashan DB: HTAP DBMS Fishing Fort: Graph analytics
Products: MedHunter, Mirror, Dream Creak, Lemmon Grass, Dasan Pass
An end-to-end solution to big data management
5
剩余34页未读,继续阅读
资源评论
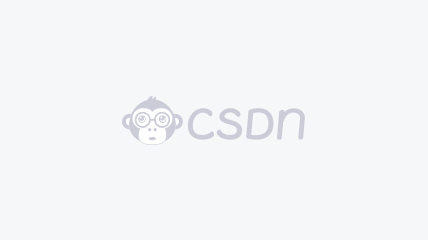

passionSnail
- 粉丝: 460
- 资源: 7531
上传资源 快速赚钱
我的内容管理 展开
我的资源 快来上传第一个资源
我的收益
登录查看自己的收益我的积分 登录查看自己的积分
我的C币 登录后查看C币余额
我的收藏
我的下载
下载帮助

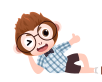
最新资源
资源上传下载、课程学习等过程中有任何疑问或建议,欢迎提出宝贵意见哦~我们会及时处理!
点击此处反馈


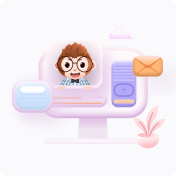
安全验证
文档复制为VIP权益,开通VIP直接复制
