没有合适的资源?快使用搜索试试~ 我知道了~
optimization of Conditional Value-at-Risk.pdf
需积分: 30 5 下载量 143 浏览量
2020-10-22
13:34:02
上传
评论
收藏 236KB PDF 举报
温馨提示
A new approach to optimizing or hedging a portfolio of nancial instruments to reduce risk is presented and tested on applications. It focuses on minimizing Conditional Value-at-Risk (CVaR) rather than minimizing Value-at-Risk (VaR)
资源推荐
资源详情
资源评论
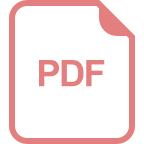
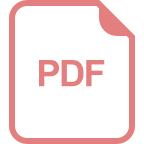
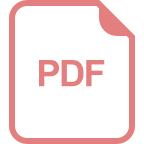
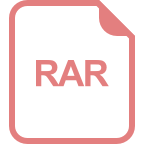
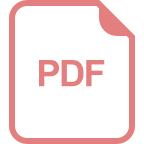
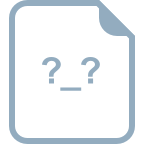
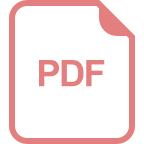
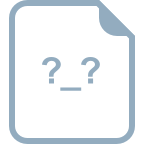
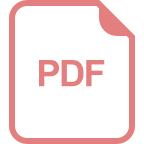
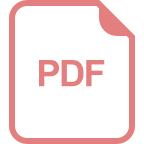
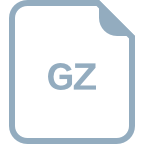
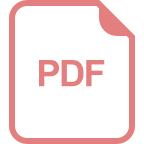
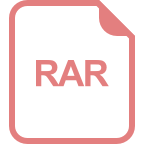
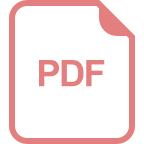
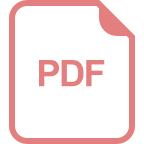
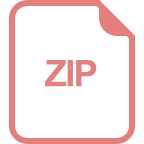
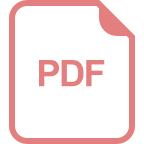
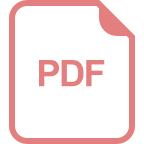
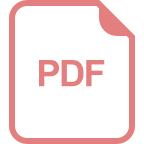
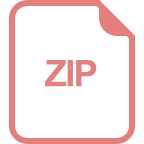
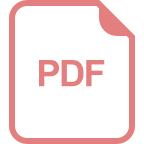
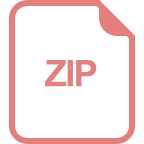
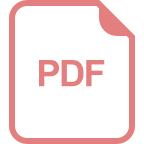
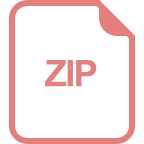
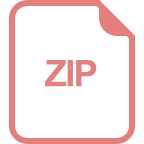
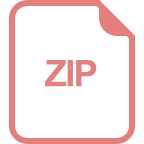
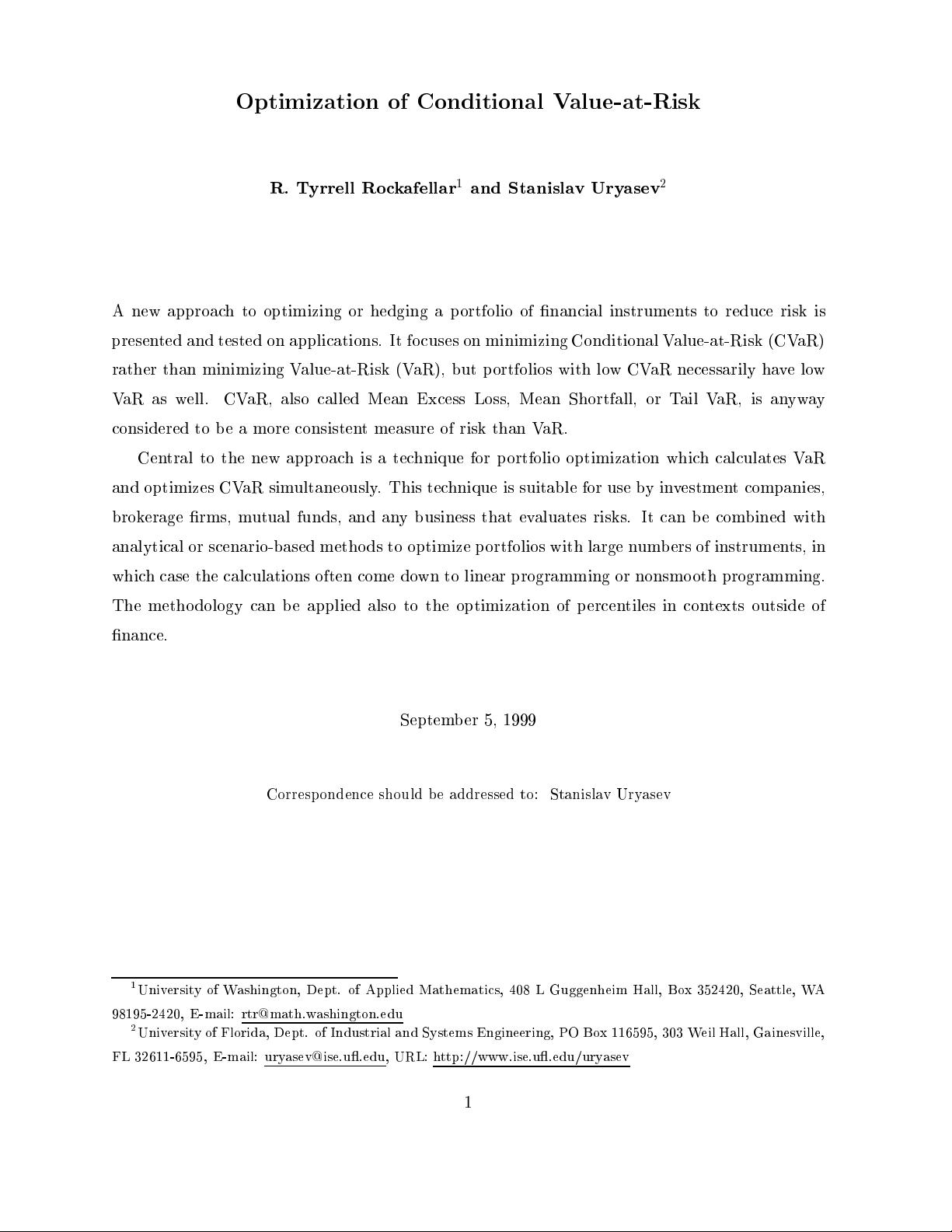
Optimization of Conditional Value-at-Risk
R. Tyrrell Ro ckafellar
1
and Stanislav Uryasev
2
A new approach to optimizing or hedging a p ortfolio of nancial instruments to reduce risk is
presented and tested on applications. It fo cuses on minimizing Conditional Value-at-Risk (CVaR)
rather than minimizing Value-at-Risk (VaR), but portfolios with lowCVaR necessarily havelow
VaR as well. CVaR, also called Mean Excess Loss, Mean Shortfall, or Tail VaR, is anyway
considered to b e a more consistent measure of risk than VaR.
Central to the new approach is a technique for p ortfolio optimization which calculates VaR
and optimizes CVaR simultaneously. This technique is suitable for use byinvestment companies,
brokerage rms, mutual funds, and any business that evaluates risks. It can be combined with
analytical or scenario-based metho ds to optimize p ortfolios with large numb ers of instruments, in
which case the calculations often come down to linear programming or nonsmo oth programming.
The metho dology can be applied also to the optimization of p ercentiles in contexts outside of
nance.
Septemb er 5, 1999
Correspondence should b e addressed to: Stanislav Uryasev
1
UniversityofWashington, Dept. of Applied Mathematics, 408 L Guggenheim Hall, Box 352420, Seattle, WA
98195-2420, E-mail: rtr@math.washington.edu
2
University of Florida, Dept. of Industrial and Systems Engineering, PO Box 116595, 303 Weil Hall, Gainesville,
FL 32611-6595, E-mail: uryasev@ise.u.edu
, URL: http://www.ise.u.edu/uryasev
1
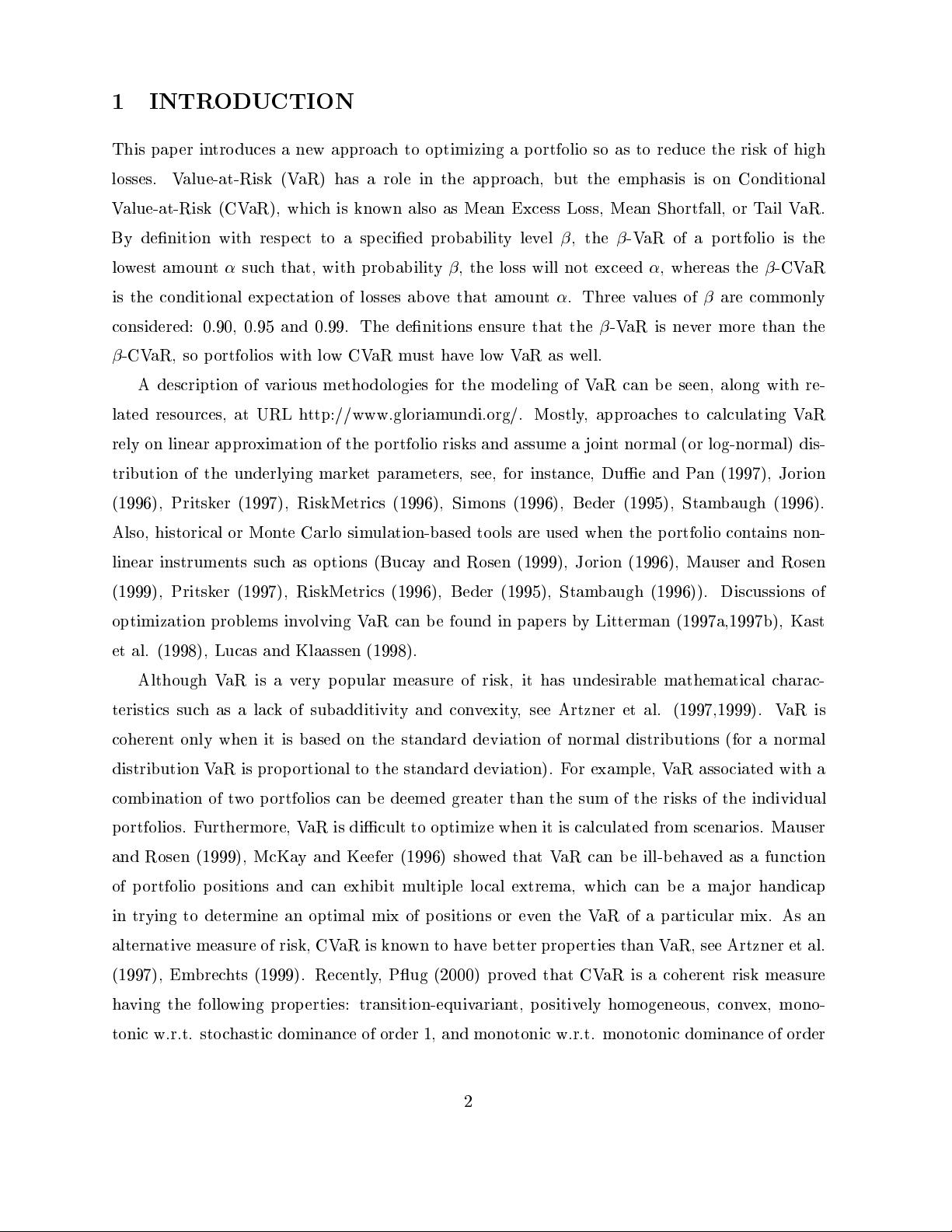
1 INTRODUCTION
This pap er introduces a new approach to optimizing a p ortfolio so as to reduce the risk of high
losses. Value-at-Risk (VaR) has a role in the approach, but the emphasis is on Conditional
Value-at-Risk (CVaR), whichisknown also as Mean Excess Loss, Mean Shortfall, or Tail VaR.
By denition with resp ect to a sp ecied probability level
, the
-VaR of a p ortfolio is the
lowest amount
such that, with probability
, the loss will not exceed
, whereas the
-CVaR
is the conditional exp ectation of losses ab ove that amount
. Three values of
are commonly
considered: 0.90, 0.95 and 0.99. The denitions ensure that the
-VaR is never more than the
-CVaR, so p ortfolios with lowCVaR must havelowVaR as well.
A description of various methodologies for the mo deling of VaR can be seen, along with re-
lated resources, at URL http://www.gloriamundi.org/. Mostly, approaches to calculating VaR
rely on linear approximation of the p ortfolio risks and assume a joint normal (or log-normal) dis-
tribution of the underlying market parameters, see, for instance, Due and Pan (1997), Jorion
(1996), Pritsker (1997), RiskMetrics (1996), Simons (1996), Beder (1995), Stambaugh (1996).
Also, historical or Monte Carlo simulation-based to ols are used when the p ortfolio contains non-
linear instruments such as options (Bucay and Rosen (1999), Jorion (1996), Mauser and Rosen
(1999), Pritsker (1997), RiskMetrics (1996), Beder (1995), Stambaugh (1996)). Discussions of
optimization problems involving VaR can be found in pap ers by Litterman (1997a,1997b), Kast
et al. (1998), Lucas and Klaassen (1998).
Although VaR is a very p opular measure of risk, it has undesirable mathematical charac-
teristics such as a lack of subadditivity and convexity, see Artzner et al. (1997,1999). VaR is
coherent only when it is based on the standard deviation of normal distributions (for a normal
distribution VaR is prop ortional to the standard deviation). For example, VaR asso ciated with a
combination of two portfolios can be deemed greater than the sum of the risks of the individual
p ortfolios. Furthermore, VaR is dicult to optimize when it is calculated from scenarios. Mauser
and Rosen (1999), McKay and Keefer (1996) showed that VaR can b e ill-behaved as a function
of p ortfolio p ositions and can exhibit multiple lo cal extrema, which can be a ma jor handicap
in trying to determine an optimal mix of p ositions or even the VaR of a particular mix. As an
alternative measure of risk, CVaR is known to have b etter prop erties than VaR, see Artzner et al.
(1997), Embrechts (1999). Recently, Pug (2000) proved that CVaR is a coherent risk measure
having the following properties: transition-equivariant, positively homogeneous, convex, mono-
tonic w.r.t. sto chastic dominance of order 1, and monotonic w.r.t. monotonic dominance of order
2
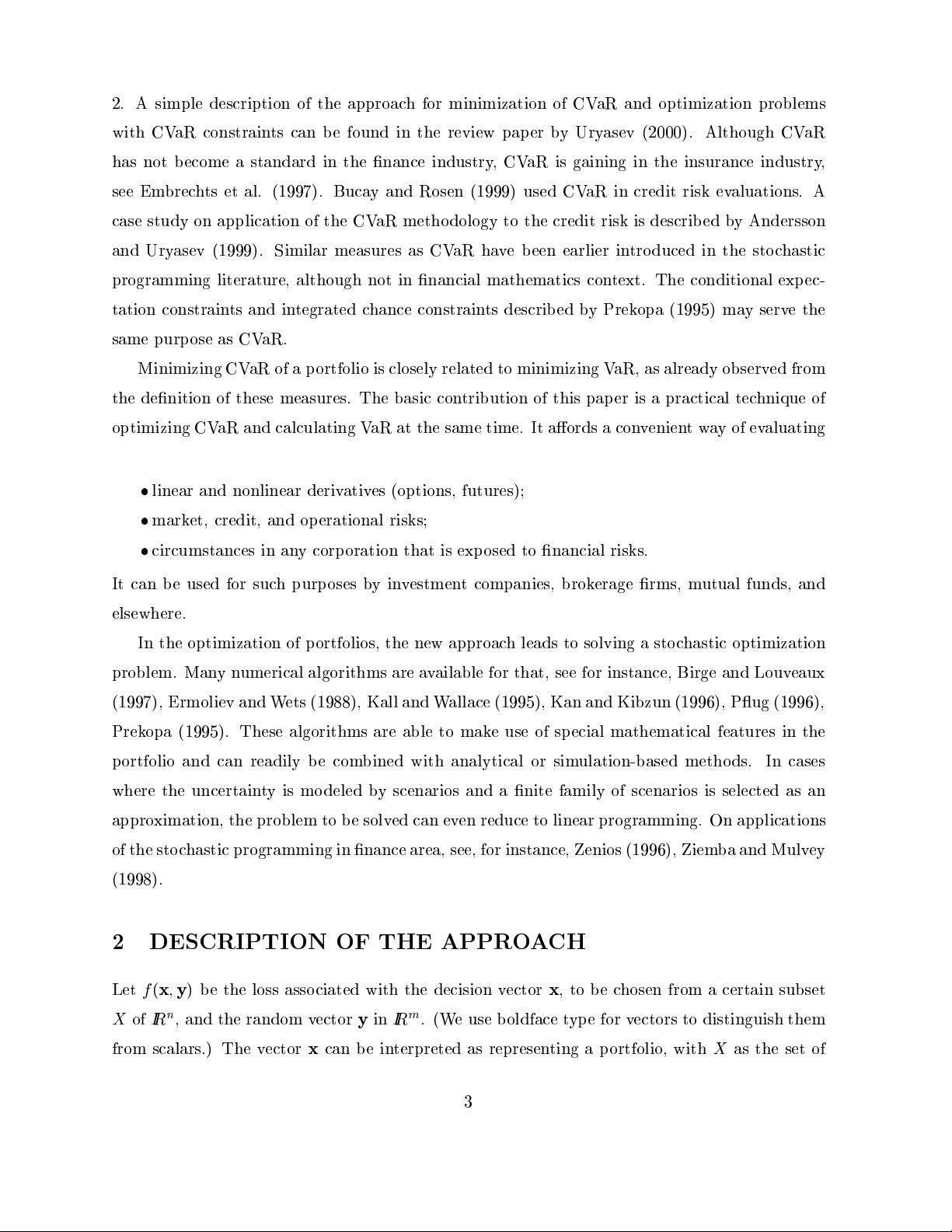
2. A simple description of the approach for minimization of CVaR and optimization problems
with CVaR constraints can be found in the review pap er by Uryasev (2000). Although CVaR
has not b ecome a standard in the nance industry, CVaR is gaining in the insurance industry,
see Embrechts et al. (1997). Bucay and Rosen (1999) used CVaR in credit risk evaluations. A
case study on application of the CVaR metho dology to the credit risk is describ ed by Andersson
and Uryasev (1999). Similar measures as CVaR have b een earlier introduced in the sto chastic
programming literature, although not in nancial mathematics context. The conditional exp ec-
tation constraints and integrated chance constraints describ ed by Prekopa (1995) may serve the
same purp ose as CVaR.
Minimizing CVaR of a p ortfolio is closely related to minimizing VaR, as already observed from
the denition of these measures. The basic contribution of this pap er is a practical technique of
optimizing CVaR and calculating VaR at the same time. It aords a convenientwayofevaluating
linear and nonlinear derivatives (options, futures);
market, credit, and op erational risks;
circumstances in any corp oration that is exp osed to nancial risks.
It can be used for such purp oses by investment companies, brokerage rms, mutual funds, and
elsewhere.
In the optimization of p ortfolios, the new approach leads to solving a sto chastic optimization
problem. Manynumerical algorithms are available for that, see for instance, Birge and Louveaux
(1997), Ermoliev and Wets (1988), Kall and Wallace (1995), Kan and Kibzun (1996), Pug (1996),
Prekopa (1995). These algorithms are able to make use of sp ecial mathematical features in the
p ortfolio and can readily be combined with analytical or simulation-based metho ds. In cases
where the uncertainty is modeled by scenarios and a nite family of scenarios is selected as an
approximation, the problem to b e solved can even reduce to linear programming. On applications
of the sto chastic programming in nance area, see, for instance, Zenios (1996), Ziemba and Mulvey
(1998).
2 DESCRIPTION OF THE APPROACH
Let
f
(
x
;
y
) be the loss associated with the decision vector
x
,tobechosen from a certain subset
X
of
IR
n
, and the random vector
y
in
IR
m
. (We use b oldface type for vectors to distinguish them
from scalars.) The vector
x
can be interpreted as representing a p ortfolio, with
X
as the set of
3
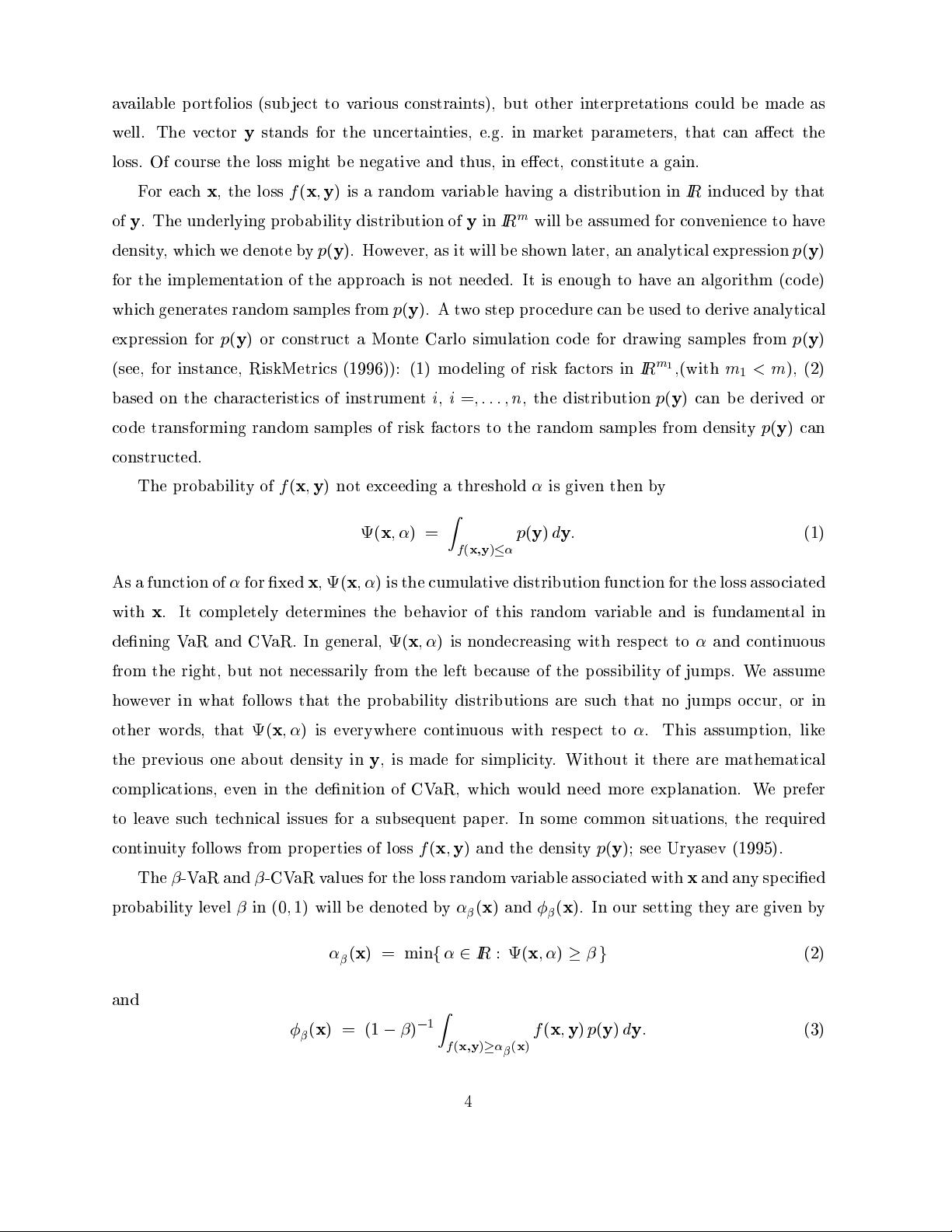
available portfolios (sub ject to various constraints), but other interpretations could be made as
well. The vector
y
stands for the uncertainties, e.g. in market parameters, that can aect the
loss. Of course the loss might b e negative and thus, in eect, constitute a gain.
For each
x
, the loss
f
(
x
;
y
) is a random variable having a distribution in
IR
induced by that
of
y
. The underlying probability distribution of
y
in
IR
m
will b e assumed for convenience to have
density, whichwe denote by
p
(
y
). However, as it will b e shown later, an analytical expression
p
(
y
)
for the implementation of the approach is not needed. It is enough to have an algorithm (co de)
which generates random samples from
p
(
y
). Atwo step pro cedure can b e used to derive analytical
expression for
p
(
y
) or construct a Monte Carlo simulation code for drawing samples from
p
(
y
)
(see, for instance, RiskMetrics (1996)): (1) mo deling of risk factors in
IR
m
1
,(with
m
1
<m
), (2)
based on the characteristics of instrument
i
,
i
=
;:::;n
, the distribution
p
(
y
) can be derived or
co de transforming random samples of risk factors to the random samples from density
p
(
y
) can
constructed.
The probabilityof
f
(
x
;
y
) not exceeding a threshold
is given then by
(
x
;
) =
Z
f
(
x
;
y
)
p
(
y
)
d
y
:
(1)
As a function of
for xed
x
,(
x
;
) is the cumulative distribution function for the loss asso ciated
with
x
. It completely determines the b ehavior of this random variable and is fundamental in
dening VaR and CVaR. In general, (
x
;
) is nondecreasing with resp ect to
and continuous
from the right, but not necessarily from the left b ecause of the p ossibility of jumps. We assume
however in what follows that the probability distributions are such that no jumps o ccur, or in
other words, that (
x
;
) is everywhere continuous with resp ect to
. This assumption, like
the previous one about density in
y
, is made for simplicity. Without it there are mathematical
complications, even in the denition of CVaR, which would need more explanation. We prefer
to leave such technical issues for a subsequent paper. In some common situations, the required
continuity follows from prop erties of loss
f
(
x
;
y
) and the density
p
(
y
); see Uryasev (1995).
The
-VaR and
-CVaR values for the loss random variable asso ciated with
x
and any sp ecied
probability level
in (0
;
1) will b e denoted by
(
x
) and
(
x
). In our setting they are given by
(
x
) = min
f
2
IR
: (
x
;
)
g
(2)
and
(
x
) = (1
,
)
,
1
Z
f
(
x
;
y
)
(
x
)
f
(
x
;
y
)
p
(
y
)
d
y
:
(3)
4
剩余25页未读,继续阅读
资源评论
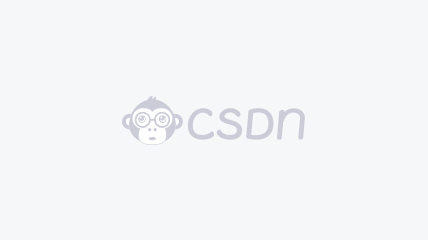

Quant0xff
- 粉丝: 1w+
- 资源: 459
上传资源 快速赚钱
我的内容管理 展开
我的资源 快来上传第一个资源
我的收益
登录查看自己的收益我的积分 登录查看自己的积分
我的C币 登录后查看C币余额
我的收藏
我的下载
下载帮助

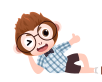
最新资源
资源上传下载、课程学习等过程中有任何疑问或建议,欢迎提出宝贵意见哦~我们会及时处理!
点击此处反馈


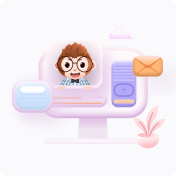
安全验证
文档复制为VIP权益,开通VIP直接复制
