
所罗门的恶魔
- 粉丝: 0
- 资源: 22
最新资源
- 10、安徽省大学生学科和技能竞赛A、B类项目列表(2019年版).xlsx
- 9、教育主管部门公布学科竞赛(2015版)-方喻飞
- C语言-leetcode题解之83-remove-duplicates-from-sorted-list.c
- C语言-leetcode题解之79-word-search.c
- C语言-leetcode题解之78-subsets.c
- C语言-leetcode题解之75-sort-colors.c
- C语言-leetcode题解之74-search-a-2d-matrix.c
- C语言-leetcode题解之73-set-matrix-zeroes.c
- 树莓派物联网智能家居基础教程
- YOLOv5深度学习目标检测基础教程
资源上传下载、课程学习等过程中有任何疑问或建议,欢迎提出宝贵意见哦~我们会及时处理!
点击此处反馈


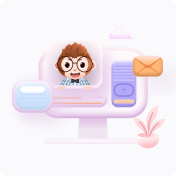