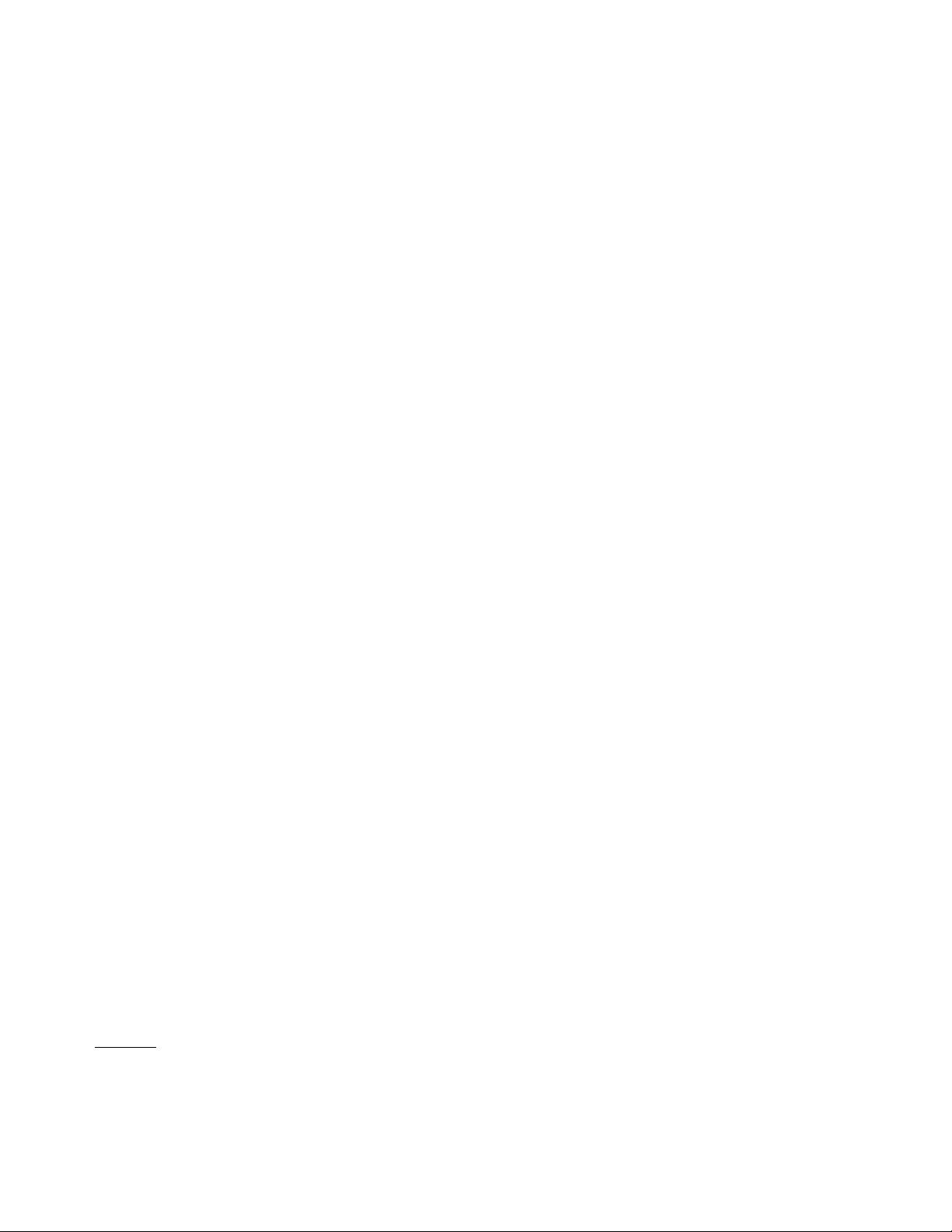
Structural joint inversion of time‐lapse crosshole ERT
and GPR traveltime data
Joseph Doetsch,
1
Niklas Linde,
2
and Andrew Binley
3
Received 13 September 2010; revised 21 October 2010; accepted 26 October 2010; published 21 December 2010.
[1]Time‐lapse geophysical monitoring and inversion are
valuable tools in h ydroge ology for monitoring changes in
the subsurface due to natural and forced (tracer) dynamics.
However, the resulting models may suffer from insufficient
resolution, which leads to underestimated variability and
poor mass recovery. Stru ctural joint inversion using cross‐
gradient constraints can provide higher‐resoluti on models
compared with individual inversions and we present the
first application to time‐lapse data. The results from a
synthetic and field vadose z one water tracer injection
experiment show that joint 3‐D time‐la pse inversion of
crosshole electrical resistance tomography (ERT) and
ground penetrating radar (GPR) traveltime data significantly
improve the imaged characteristics of the point injected
plume, such as lateral spreading and center o f mass, as well
as the overall consistency between models. The joint
inversionmethodappearstoworkwellforcaseswhenone
hydrological state varia ble (in this case moisture content)
controls the time‐lapse response of both geophysical
methods.
Citation: Doetsch, J., N. Linde, and A. Binley (2010),
Structural joint inversion of time‐lapse crosshole ERT and
GPR traveltime data, Geophys. Res. Lett., 37, L24404,
doi:10.1029/2010GL045482.
1. Introduction
[2] Time‐lapse geophysical monitoring and inversion are
valuable tools in a wide range of application areas, such as
hydrogeology, seismology, volcanology, landslide studies,
and reservoir management. By inverting for temporal chan-
ges in geophysical properties it is possible to focus on
resolving changes in state variables, such as water content and
pore water salinity. The quality and resolution of time‐lapse
inversion results may also improve compared with static
inversions as modeling and observational errors are generally
smaller [e.g., LaBrecque and Yang, 2001]. Time‐lapse
inversion results are, unfortunately, also resolution‐limited,
leading to models that might be physically implausible or the
resolved scales might be larger than those of interest [e.g.,
Day‐Lewis et al., 2005]. Well known problems include the
difficulty of recovering the injected mass of tracer or water
from time‐lapse inversion results [e.g., Binley et al., 2002a]
and significant smearing in the horizontal directions
[Singha and Gorelick, 2005] that reduce the value of geo-
physical time‐lapse models in quantitative flow and trans-
port studies.
[
3] Structural joint inversions of geophysical data acquired
under static field conditions provide geometrically similar
models and improve model resolution compared with indi-
vidual inversions [e.g., Gallardo and Meju, 2004; Linde
et al., 2008]. We focus here, for the first time, on the
applicability of a structural joint inversion approach to time‐
lapse data. Structure is imposed by penalizing deviations
from cases when the gradients ‐ for different geophysical
properties ‐ of the total model updates from background
models point in the same or opposite directions. These
background models are obtained by inversion of the data
acquired prior to any perturbation. We investigate the merits
of this cross‐gradient‐constrained joint inversion using a
synthetic and field vadose zone water‐injection experiment,
both of which employ time‐lapse crosshole electrical resis-
tivity tomography (ERT) data and first‐arrival ground‐pen-
etrating radar (GPR) traveltimes. Under these conditions, the
time‐lapse changes in both data types are related solely to
variations in moisture content.
2. Methods
2.1. Time‐Lapse Inversion Strategy
[
4] The first step of our time‐lapse inversion strategy is to
obtain background (and initial) models of the logarithm of
electrical resistivity (m
e,0
) and radar slowness (m
r,0
)by
inverting data sets acquired prior to any perturbations. The
data are inverted following an Occam’s type inversion by
penalizing differences from a homogeneous model as defined
by an exponential covariance model [Linde et al., 2006]. We
then use a difference inversion approach to invert the time‐
lapse data [e.g., LaBrecque and Yang, 2001] in which we, in a
similar manner, penalize deviations from m
e,0
and m
r,0
.
[
5] For the ERT inversions, we use an error model con-
sisting of a systematic contribution e
es
that is the same for
all time‐lapse steps, and a random observational error e
e,p
(p =0,1,2,… P, where P is the number of time steps) that is
different for each time‐lapse data set [e.g., LaBrecque and
Yang, 2001] but assumed to stem from the same zero‐mean
Gaussian distribution. The observed data at time 0 are thus
d
obs
e;0
¼ g m
e;0
þ e
es
þ e
e;0
; ð1Þ
with the forward response g(m
e,0
). The main contribution to
the background residual
r
e;0
¼ d
obs
e;0
g m
e;0
¼ e
es
þ e
e;0
ð2Þ
is the systematic error e
es
, which is a combination of mod-
eling errors and systematic measurement errors due to
ground coupling problems or geometrical errors. It is largely
1
Institute of Geophysics, ETH Zurich, Zurich, Switzerland.
2
Institute of Geophysics, University of Laus anne, Lausanne ,
Switzerland.
3
Lancaster Environment Centre, Lancaster University, Lancaster,
UK.
Copyright 2010 by the American Geophysical Union.
0094‐8276/10/2010GL045482
GEOPHYSICAL RESEARCH LETTERS, VOL. 37, L24404, doi:10.1029/2010GL045482, 2010
L24404 1of6