# 单变量负荷预测
from __future__ import print_function
import pandas as pd
import numpy as np
import matplotlib.pyplot as plt
from keras.layers.core import Dense, Activation, Dropout
from keras.layers.recurrent import LSTM
from keras.models import Sequential
from keras.models import load_model
import pywt
#print(pywt.families,pywt.wavelist('coif'))
import statistics
import math
from keras.optimizers import SGD
from keras import backend as K
# define a function to convert a vector of time series into a 2D matrix 定义将时间序列向量转换为二维矩阵的函数
def convertSeriesToMatrix(vectorSeries, sequence_length):
matrix=[]
for i in range(len(vectorSeries)-sequence_length+1):
matrix.append(vectorSeries[i:i+sequence_length])
return matrix
def dwt(a):
[ca, cd] = pywt.dwt(a,'haar')
return ca,cd
def idwt(ca,cd):
ori = pywt.idwt(ca,cd,'haar')
return ori
def generateData(sample, outputnum):
a = np.array(sample)
mu = np.mean(a)
#sigma_2 = np.var(a) / 2
sigma_2 = np.var(a) / 24 #方差
result = np.random.normal(loc = mu, scale = np.sqrt(sigma_2), size = outputnum) #正态分布
#result = np.random.logistic(loc=mu, scale=np.sqrt(sigma_2), size=outputnum) #Logistic分布
# result = np.random.laplace(loc=mu, scale=np.sqrt(sigma_2), size=outputnum) #拉普拉斯/双指数分布
print('mu = %f\tsigma^2 = %f'%(mu,sigma_2))
return mu,sigma_2,result
def drawResult(mu,sigma_2,result):
plt.figure(figsize=(10,8),dpi=80)
count, bins, ignored = plt.hist(result, 30, normed=True)
plt.plot(bins, 1/(np.sqrt(2 * np.pi * sigma_2)) *np.exp( - (bins - mu)**2 / (2 * sigma_2) ),linewidth=2, color='r')
def dataset(matrix_load,train_row):
# shift all data by mean 去均值
matrix_load = np.array(matrix_load)
#shifted_value = matrix_load.mean()
#matrix_load -= shifted_value
print("Data shape: ", matrix_load.shape)
# 划分数据集
train_set = matrix_load[:train_row, :]
# random seed
np.random.seed(1234)
# shuffle the training set (but do not shuffle the test set)
np.random.shuffle(train_set)
# the training set
X_train = train_set[:, :-1]
y_train = train_set[:, -1]
# the test set
X_test = matrix_load[train_row:, :-1]
y_test = matrix_load[train_row:, -1]
# the input to LSTM layer needs to have the shape of (number of samples, the dimension of each element) 输入(样本数量,每个元素维数)形式
X_train = np.reshape(X_train, (X_train.shape[0], X_train.shape[1], 1))
X_test = np.reshape(X_test, (X_test.shape[0], X_test.shape[1], 1))
print(np.shape(X_train), np.shape(X_test))
return X_train,y_train,X_test,y_test
def root_mean_squared_error(actual, pred):
return K.sqrt(K.mean(K.square(pred - actual), axis=-1))
def train_ca_cd(type,X_train,y_train,X_test,y_test):
# build the model 序贯模型
model = Sequential()
# layer 1: LSTM
model.add(LSTM( input_dim=1, output_dim=150, return_sequences=True))
model.add(Dropout(0.2))
# layer 2: LSTM
model.add(LSTM(output_dim=200, return_sequences=False))
model.add(Dropout(0.2))
# layer 3: dense
# linear activation: a(x) = x
model.add(Dense(output_dim=1, activation='linear'))
# show model
model.summary()
# compile the model
# sgd = SGD(lr=0.01, decay=1e-6, momentum=0.9, nesterov=True)
model.compile(loss='mse', optimizer="rmsprop")
# train the model
model.fit(X_train, y_train, batch_size=512, nb_epoch=100, validation_split=0.05, verbose=2)
# save model
model.save('../model/dwt_lstm_'+type+'.h5')
# evaluate the result
#test_mse = model.evaluate(X_test, y_test, verbose=1)
#print('\nThe MSE of %s on the test data set is %.3f over %d test samples.' % (type,test_mse, len(y_test)))
return model
# load raw data 加载原始数据
df_raw = pd.read_csv('../data/ENTSO-E/load.csv', header=0, usecols=[0,1])
# numpy array
df_raw_array = df_raw.values
# daily load 加载日负载数据
list_hourly_load = [df_raw_array[i,1]/1000 for i in range(1, len(df_raw))]
print ("Data shape of list_hourly_load: ", np.shape(list_hourly_load))
# 异常值处理
k = 0
for j in range(0, len(list_hourly_load)):
if(abs(list_hourly_load[j]-list_hourly_load[j-1])>2 and abs(list_hourly_load[j]-list_hourly_load[j+1])>2):
k = k + 1
list_hourly_load[j] = (list_hourly_load[j - 1] + list_hourly_load[j + 1]) / 2 + list_hourly_load[j - 24] - list_hourly_load[j - 24 - 1] / 2
sum = 0
num = 0
for t in range(1,8):
if(j - 24*t >= 0):
num = num + 1
sum = sum + list_hourly_load[j - 24*t]
if(j + 24*t < len(list_hourly_load)):
num = num + 1
sum = sum + list_hourly_load[j + 24*t]
sum = sum / num
if(abs(list_hourly_load[j] - sum)>3):
k = k + 1
if(list_hourly_load[j] > sum): list_hourly_load[j] = sum + 3
else: list_hourly_load[j] = sum - 3
print(k)
# 去均值
list_hourly_load = np.array(list_hourly_load)
shifted_value = list_hourly_load.mean()
list_hourly_load -= shifted_value
# 小波变换
a2 , d2 , d1 = pywt.wavedec(list_hourly_load, 'db4', mode = 'sym', level = 2)
a2 = a2 / 10
shifted_value_a2 = a2.mean()
a2 -= shifted_value_a2
# lhl = pywt.waverec([a2, d2, d1], 'db4')
# print(np.shape(a2),np.shape(d2),np.shape(d1),np.shape(lhl))
print(a2[0],d2[0],d1[0])
print('DWT finish.')
print(np.shape(a2),np.shape(d2),np.shape(d1))
# the length of the sequnce for predicting the future value
sequence_length = 25
# convert the vector to a 2D matrix
a2_matrix_load = convertSeriesToMatrix(a2, sequence_length)
d2_matrix_load = convertSeriesToMatrix(d2, sequence_length)
d1_matrix_load = convertSeriesToMatrix(d1, sequence_length)
# split dataset: 90% for training and 10% for testing 切分数据集
# train_row = int(round(0.9 * matrix_load.shape[0]))
train_row_2 = len(a2_matrix_load) - 1001
train_row_1 = len(d1_matrix_load) - 1995
print('train:', train_row_2 , train_row_1, 'test:', 1001, 1995)
y_test_true = list_hourly_load[-166 * 24:]
time_test = [df_raw_array[i, 0] for i in range(len(df_raw) - 166 * 24, len(df_raw))]
# dataset
a2_X_train,a2_y_train,a2_X_test,a2_y_test = dataset(a2_matrix_load,train_row_2)
d2_X_train,d2_y_train,d2_X_test,d2_y_test = dataset(d2_matrix_load,train_row_2)
d1_X_train,d1_y_train,d1_X_test,d1_y_test = dataset(d1_matrix_load,train_row_1)
# train
a2_model = train_ca_cd('a2',a2_X_train,a2_y_train,a2_X_test,a2_y_test)
d2_model = train_ca_cd('d2',d2_X_train,d2_y_train,d2_X_test,d2_y_test)
d1_model = train_ca_cd('d1',d1_X_train,d1_y_train,d1_X_test,d1_y_test)
# load model
#from keras.models import load_model
# a2_model = load_model('dwt_lstm_a2_50.h5')
# d2_model = load_model('dwt_lstm_d2_50.h5')
# d1_model = load_model('dwt_lstm_d1_50.h5')
# evaluate the result
a2_test_mse = a2_model.evaluate(a2_X_test, a2_y_test, verbose=2)
print('\nThe MSE of %s on the test data set is %.3f over %d test samples.' % ('a2',a2_test_mse, len(a2_y_test)))
d2_test_mse = d2_model.evaluate(d2_X_test, d2_y_test, verbose=2)
print('\nThe MSE of %s on the test data set is %.3f over %d test samples.' % ('d2',d2_test_mse, len(d2_y_test)))
d1_test_mse = d1_model.evaluate(d1_X_test, d1_y_test, verbose=2)
print('\nThe MSE of %s on the test data set is %.3f over %d test samples.' % ('d1',d1_test_mse, len(d1_y_test)))
# get the predicted values
a2_pred = a2_model.predict(a2_X_test)[:,0]
a2_pred = (a2_pred + shifted_value_a2) * 10
print('Lowpass coefficient estimation finish.')
# mu, sigma_2, cd_pred = generateData(cd[0:train_row], outputnum=len(cd)-24-train_row)
d2_pred = d2_model.predict(d2_X_test)[:,0]
d1_pred = d1_model.predict(d1_X_test)[:,0]
print('Highpass coefficient estimation finish.')
# print(np.shape(ca_pred),np.shape(cd_pred))
# predicted_values = idwt((ca_pred + shifted_value_ca)* 10, cd_pred)
print(np.shape(a2_pred),np.shape(d2_pred),np.shape(d1_pred))
predicted_values = pywt.waver

share_data
- 粉丝: 1047
- 资源: 107
最新资源
- 激光打标止挡升降一体化流水线体sw17全套技术资料100%好用.zip
- 圣诞老人插画,请收藏之
- 发动机最优油耗曲线绘制MATLAB程序
- IMG_20241227_135342.jpg
- IMG_20241227_135318.jpg
- 夹持输送激光打标设备sw18可编辑全套技术资料100%好用.zip
- 基于web的农业信息管理系统设计与实现
- python demo 数据库联动
- Windows 10系统NH82580EB以太网控制器网络冗余设置教程
- 采用拉丁超立方采样的电力系统概率潮流计算 (自适应核密度估计,自适应带宽核密度估计) 拉丁超立方采样属于分层采样,是一种有效的用采样值反映随机变量的整体分布的方法 其目的是要保证所有的采样区域都能够
- pyth 展示简单页面 展示注册页面 比如 注册信息,用户名,密码,邮件,等信息
- 基于MATLAB和机器学习(向量机)的裂缝检测(识别)系统程序,带GUI界面,对裂缝主要参数(长度,宽度,面积)进行计算,已经训练好分类器,包含裂缝图像训练集和测试集,可以完美运行(带注释),结果可保
- 二分香农(范诺编码)-MATLAB实现
- visual basic 6.0教程、visual basic从入门到精通、visual basic从入门到实践【教程+VB简版】
- html+css+js网页设计 美食 美拾9个页面
- pdf便携文档编辑软件
资源上传下载、课程学习等过程中有任何疑问或建议,欢迎提出宝贵意见哦~我们会及时处理!
点击此处反馈


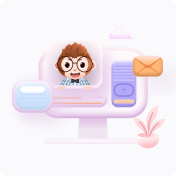
评论0