"""Implementation of GradientBoostingRegressor in sklearn using the
boston dataset which is very popular for regression problem to
predict house price.
"""
import matplotlib.pyplot as plt
import pandas as pd
from sklearn.datasets import load_boston
from sklearn.ensemble import GradientBoostingRegressor
from sklearn.metrics import mean_squared_error, r2_score
from sklearn.model_selection import train_test_split
def main():
# loading the dataset from the sklearn
df = load_boston()
print(df.keys())
# now let construct a data frame
df_boston = pd.DataFrame(df.data, columns=df.feature_names)
# let add the target to the dataframe
df_boston["Price"] = df.target
# print the first five rows using the head function
print(df_boston.head())
# Summary statistics
print(df_boston.describe().T)
# Feature selection
x = df_boston.iloc[:, :-1]
y = df_boston.iloc[:, -1] # target variable
# split the data with 75% train and 25% test sets.
x_train, x_test, y_train, y_test = train_test_split(
x, y, random_state=0, test_size=0.25
)
model = GradientBoostingRegressor(
n_estimators=500, max_depth=5, min_samples_split=4, learning_rate=0.01
)
# training the model
model.fit(x_train, y_train)
# to see how good the model fit the data
training_score = model.score(x_train, y_train).round(3)
test_score = model.score(x_test, y_test).round(3)
print("Training score of GradientBoosting is :", training_score)
print("The test score of GradientBoosting is :", test_score)
# Let us evaluation the model by finding the errors
y_pred = model.predict(x_test)
# The mean squared error
print(f"Mean squared error: {mean_squared_error(y_test, y_pred):.2f}")
# Explained variance score: 1 is perfect prediction
print(f"Test Variance score: {r2_score(y_test, y_pred):.2f}")
# So let's run the model against the test data
fig, ax = plt.subplots()
ax.scatter(y_test, y_pred, edgecolors=(0, 0, 0))
ax.plot([y_test.min(), y_test.max()], [y_test.min(), y_test.max()], "k--", lw=4)
ax.set_xlabel("Actual")
ax.set_ylabel("Predicted")
ax.set_title("Truth vs Predicted")
# this show function will display the plotting
plt.show()
if __name__ == "__main__":
main()

流华追梦
- 粉丝: 1w+
- 资源: 3850
最新资源
- SQL语言详细教程:从基础到高级全面解析及实际应用
- 仓库管理系统源代码全套技术资料.zip
- 计算机二级考试详细试题整理及备考建议
- 全国大学生电子设计竞赛(电赛)历年试题及备考指南
- zigbee CC2530网关+4节点无线通讯实现温湿度、光敏、LED、继电器等传感节点数据的采集上传,网关通过ESP8266上传远程服务器及下发控制.zip
- 云餐厅APP项目源代码全套技术资料.zip
- vscode 翻译插件开发,选中要翻译的单词,使用快捷键Ctrl+Shift+T查看翻译
- mrdoc-alpine0.9.2
- ACMNOICSP比赛经验分享:从知识储备到团队协作的全面指南
- 云餐厅项目源代码全套技术资料.zip
- 基于STM32的数字闹钟系统的仿真和程序
- 混合信号设计中DEF文件创建流程
- 美国大学生数学建模竞赛(美赛)详细教程:从组队到赛后总结全攻略
- 病媒生物孳生地调查和治理工作方案.docx
- 保姆的工作标准.docx
- 病媒生物防制指南.docx
资源上传下载、课程学习等过程中有任何疑问或建议,欢迎提出宝贵意见哦~我们会及时处理!
点击此处反馈


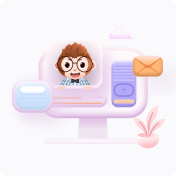