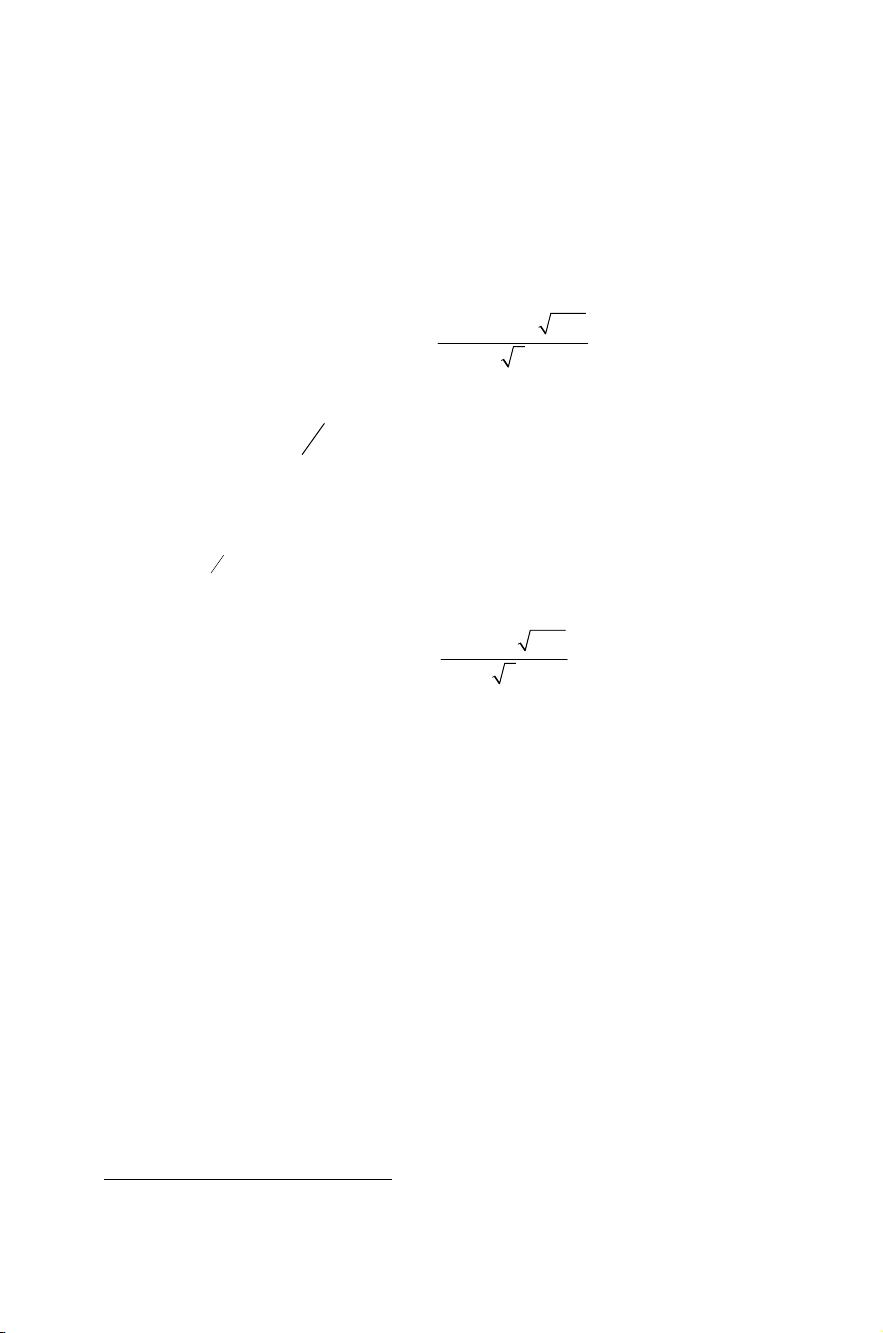
82 4. Issues and Intuition in Path Analysis
service
*
may be forgiven for asking the question, “Why is yet one more test neces-
sary?” However, there are three new issues in clinical trial monitoring that the clas-
sic hypothesis testing procedures do not directly address. They are (1) repeated test-
ing of data, (2) variability, and (3) the notion of dependency.
4.3.1 Repeated Testing
We have established that one of the most important issues in generalizing results
from a sample to the population is the effect of sampling variability. One notewor-
thy way in which sampling error can mislead an investigator is by the construction
(through the random aggregation of subjects in a sample) of a result that can appear
to be clinically relevant but at the same time is not representative of the population.
The two types of sampling errors that clinical researchers measure are the type I or
alpha error rate and the type II or beta error rate, as discussed in Chapter One. The
medical and regulatory communities are comfortable with drawing conclusions
from concordantly executed studies
†
when these rates are kept at acceptably low
levels.
However, these rates can grow to unacceptably high levels when statistical
testing is carried out repeatedly in the same research effort. The monitoring of a
clinical study during the course of its follow-up period is a clear example of this
phenomenon. Other illustrations are multiple endpoint evaluation, subgroup ana-
lyzes, and the evaluation of different contrasts between the arms of a clinical trial
with more than two treatment groups. Difficulties with these analyzes have been
well elucidated in the literature [2,3,4]. The particular problems induced in the in-
terim monitoring setting have also been elaborated [5].
The principal difficulty with multiple analyzes is that the overall false
positive error rate or alpha error rate increases with the number of tests that are exe-
cuted. Thus, although each test provides the same level of protection, the integrity
of the overall process degrades. This is easily demonstrated. Consider an example
of a clinical study that assesses the ability of a therapy to reduce the fatal stroke rate.
At the conclusion of the research, the investigator plans to construct a test statistic
that will produce a type I error rate of 0.05. However, the investigator intends to
have the study monitored every year until the five-year study has concluded. At
each monitoring point, he is looking for an early demonstration of the same effect
that he hopes will be demonstrated at the conclusion of the five-year study. There-
fore, a treatment effect finding resulting in a p-value 0.05 for any of the five ana-
lyzes would be sufficient for him.
At first appearance, this collection of tests may appear to offer substantial
protection against the occurrence of an alpha error or false positive results. After all,
this 0.05 level of protection was satisfactory for drawing conclusions at the end of
the research effort. If it will be adequate when applied at the end of the study, why
wouldn’t it afford adequate protection during the interim monitoring times?
*
T-tests, chi-square tests, tests of equality of proportions, life table analyzes, and Bayes pro-
cedures are but a few of the many types of test statistics brought to bear in the evaluation of
clinical research data.
†
A concordantly executed study is one that is follows its prospectively written protocol.
评论0
最新资源