DeepLearnToolbox
================
A Matlab toolbox for Deep Learning.
Deep Learning is a new subfield of machine learning that focuses on learning deep hierarchical models of data.
It is inspired by the human brain's apparent deep (layered, hierarchical) architecture.
A good overview of the theory of Deep Learning theory is
[Learning Deep Architectures for AI](http://www.iro.umontreal.ca/~bengioy/papers/ftml_book.pdf)
For a more informal introduction, see the following videos by Geoffrey Hinton and Andrew Ng.
* [The Next Generation of Neural Networks](http://www.youtube.com/watch?v=AyzOUbkUf3M) (Hinton, 2007)
* [Recent Developments in Deep Learning](http://www.youtube.com/watch?v=VdIURAu1-aU) (Hinton, 2010)
* [Unsupervised Feature Learning and Deep Learning](http://www.youtube.com/watch?v=ZmNOAtZIgIk) (Ng, 2011)
If you use this toolbox in your research please cite [Prediction as a candidate for learning deep hierarchical models of data](http://www2.imm.dtu.dk/pubdb/views/publication_details.php?id=6284)
```
@MASTERSTHESIS\{IMM2012-06284,
author = "R. B. Palm",
title = "Prediction as a candidate for learning deep hierarchical models of data",
year = "2012",
}
```
Contact: rasmusbergpalm at gmail dot com
Directories included in the toolbox
-----------------------------------
`NN/` - A library for Feedforward Backpropagation Neural Networks
`CNN/` - A library for Convolutional Neural Networks
`DBN/` - A library for Deep Belief Networks
`SAE/` - A library for Stacked Auto-Encoders
`CAE/` - A library for Convolutional Auto-Encoders
`util/` - Utility functions used by the libraries
`data/` - Data used by the examples
`tests/` - unit tests to verify toolbox is working
For references on each library check REFS.md
Setup
-----
1. Download.
2. addpath(genpath('DeepLearnToolbox'));
Everything is work in progress
------------------------------
Example: Deep Belief Network
---------------------
```matlab
function test_example_DBN
load mnist_uint8;
train_x = double(train_x) / 255;
test_x = double(test_x) / 255;
train_y = double(train_y);
test_y = double(test_y);
%% ex1 train a 100 hidden unit RBM and visualize its weights
rng(0);
dbn.sizes = [100];
opts.numepochs = 1;
opts.batchsize = 100;
opts.momentum = 0;
opts.alpha = 1;
dbn = dbnsetup(dbn, train_x, opts);
dbn = dbntrain(dbn, train_x, opts);
figure; visualize(dbn.rbm{1}.W'); % Visualize the RBM weights
%% ex2 train a 100-100 hidden unit DBN and use its weights to initialize a NN
rng(0);
%train dbn
dbn.sizes = [100 100];
opts.numepochs = 1;
opts.batchsize = 100;
opts.momentum = 0;
opts.alpha = 1;
dbn = dbnsetup(dbn, train_x, opts);
dbn = dbntrain(dbn, train_x, opts);
%unfold dbn to nn
nn = dbnunfoldtonn(dbn, 10);
nn.activation_function = 'sigm';
%train nn
opts.numepochs = 1;
opts.batchsize = 100;
nn = nntrain(nn, train_x, train_y, opts);
[er, bad] = nntest(nn, test_x, test_y);
assert(er < 0.10, 'Too big error');
```
Example: Stacked Auto-Encoders
---------------------
```matlab
function test_example_SAE
load mnist_uint8;
train_x = double(train_x)/255;
test_x = double(test_x)/255;
train_y = double(train_y);
test_y = double(test_y);
%% ex1 train a 100 hidden unit SDAE and use it to initialize a FFNN
% Setup and train a stacked denoising autoencoder (SDAE)
rng(0);
sae = saesetup([784 100]);
sae.ae{1}.activation_function = 'sigm';
sae.ae{1}.learningRate = 1;
sae.ae{1}.inputZeroMaskedFraction = 0.5;
opts.numepochs = 1;
opts.batchsize = 100;
sae = saetrain(sae, train_x, opts);
visualize(sae.ae{1}.W{1}(:,2:end)')
% Use the SDAE to initialize a FFNN
nn = nnsetup([784 100 10]);
nn.activation_function = 'sigm';
nn.learningRate = 1;
nn.W{1} = sae.ae{1}.W{1};
% Train the FFNN
opts.numepochs = 1;
opts.batchsize = 100;
nn = nntrain(nn, train_x, train_y, opts);
[er, bad] = nntest(nn, test_x, test_y);
assert(er < 0.16, 'Too big error');
%% ex2 train a 100-100 hidden unit SDAE and use it to initialize a FFNN
% Setup and train a stacked denoising autoencoder (SDAE)
rng(0);
sae = saesetup([784 100 100]);
sae.ae{1}.activation_function = 'sigm';
sae.ae{1}.learningRate = 1;
sae.ae{1}.inputZeroMaskedFraction = 0.5;
sae.ae{2}.activation_function = 'sigm';
sae.ae{2}.learningRate = 1;
sae.ae{2}.inputZeroMaskedFraction = 0.5;
opts.numepochs = 1;
opts.batchsize = 100;
sae = saetrain(sae, train_x, opts);
visualize(sae.ae{1}.W{1}(:,2:end)')
% Use the SDAE to initialize a FFNN
nn = nnsetup([784 100 100 10]);
nn.activation_function = 'sigm';
nn.learningRate = 1;
%add pretrained weights
nn.W{1} = sae.ae{1}.W{1};
nn.W{2} = sae.ae{2}.W{1};
% Train the FFNN
opts.numepochs = 1;
opts.batchsize = 100;
nn = nntrain(nn, train_x, train_y, opts);
[er, bad] = nntest(nn, test_x, test_y);
assert(er < 0.1, 'Too big error');
```
Example: Convolutional Neural Nets
---------------------
```matlab
function test_example_CNN
load mnist_uint8;
train_x = double(reshape(train_x',28,28,60000))/255;
test_x = double(reshape(test_x',28,28,10000))/255;
train_y = double(train_y');
test_y = double(test_y');
%% ex1 Train a 6c-2s-12c-2s Convolutional neural network
%will run 1 epoch in about 200 second and get around 11% error.
%With 100 epochs you'll get around 1.2% error
rng(0)
cnn.layers = {
struct('type', 'i') %input layer
struct('type', 'c', 'outputmaps', 6, 'kernelsize', 5) %convolution layer
struct('type', 's', 'scale', 2) %sub sampling layer
struct('type', 'c', 'outputmaps', 12, 'kernelsize', 5) %convolution layer
struct('type', 's', 'scale', 2) %subsampling layer
};
cnn = cnnsetup(cnn, train_x, train_y);
opts.alpha = 1;
opts.batchsize = 50;
opts.numepochs = 1;
cnn = cnntrain(cnn, train_x, train_y, opts);
[er, bad] = cnntest(cnn, test_x, test_y);
%plot mean squared error
figure; plot(cnn.rL);
assert(er<0.12, 'Too big error');
```
Example: Neural Networks
---------------------
```matlab
function test_example_NN
load mnist_uint8;
train_x = double(train_x) / 255;
test_x = double(test_x) / 255;
train_y = double(train_y);
test_y = double(test_y);
% normalize
[train_x, mu, sigma] = zscore(train_x);
test_x = normalize(test_x, mu, sigma);
%% ex1 vanilla neural net
rng(0);
nn = nnsetup([784 100 10]);
opts.numepochs = 1; % Number of full sweeps through data
opts.batchsize = 100; % Take a mean gradient step over this many samples
[nn, L] = nntrain(nn, train_x, train_y, opts);
[er, bad] = nntest(nn, test_x, test_y);
assert(er < 0.08, 'Too big error');
% Make an artificial one and verify that we can predict it
x = zeros(1,28,28);
x(:, 14:15, 6:22) = 1;
x = reshape(x,1,28^2);
figure; visualize(x');
predicted = nnpredict(nn,x)-1;
assert(predicted == 1);
%% ex2 neural net with L2 weight decay
rng(0);
nn = nnsetup([784 100 10]);
nn.weightPenaltyL2 = 1e-4; % L2 weight decay
opts.numepochs = 1; % Number of full sweeps through data
opts.batchsize = 100; % Take a mean gradient step over this many samples
nn = nntrain(nn, train_x, train_y, opts);
[er, bad] = nntest(nn, test_x, test_y);
assert(er < 0.1, 'Too big error');
%% ex3 neural net with dropout
rng(0);
nn = nnsetup([784 100 10]);
nn.dropoutFraction = 0.5; % Dropout fraction
opts.numepochs = 1; % Number of full sweeps through data
opts.batchsize = 100; % Take a mean gradient step over this many samples
nn = nntrain(nn, train_x, train_y, opts);
[er, bad] = nntest(nn, test_x, test_y);
assert(er < 0.1, 'Too big error');
%% ex4 neural net with sigmoid activation function
rng(0);
nn = nnsetup([784 100 10]);
nn.activation_function = 'sigm'; % Sigmoid activation function
nn.learningRate = 1; % Sigm require a lower learning rate
opts.numepochs = 1; % Number of full sweeps through data
opts.batchsize = 100; % Take a mean gradient step over this
没有合适的资源?快使用搜索试试~ 我知道了~
资源推荐
资源详情
资源评论
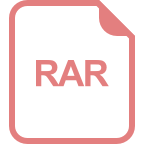
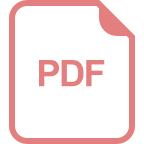
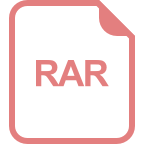
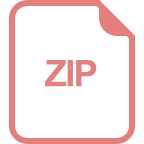
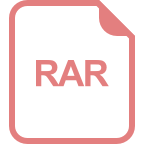
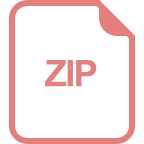
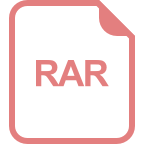
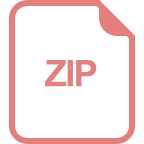
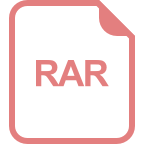
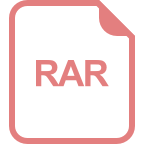
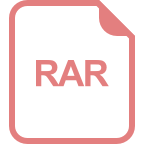
收起资源包目录

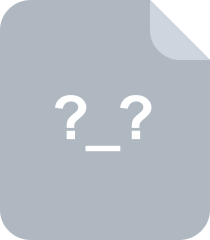
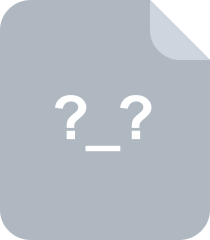
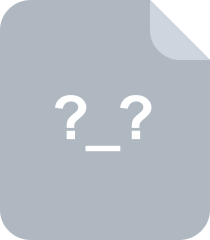
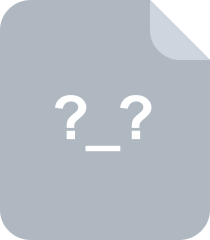
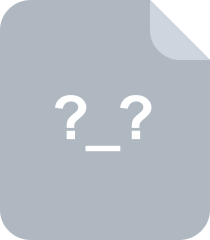
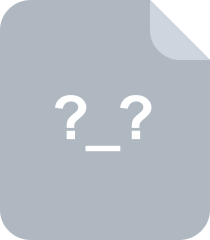
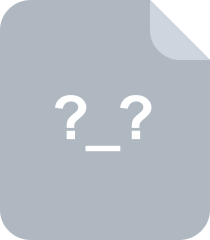
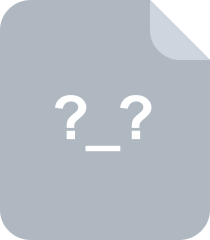
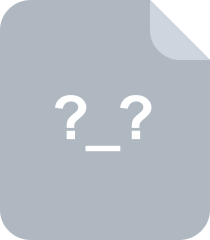
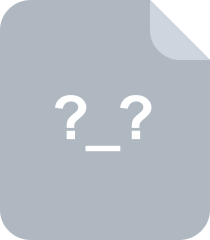
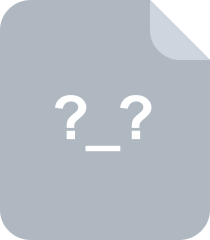
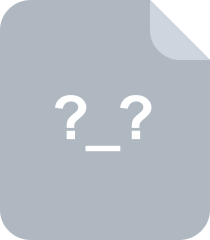
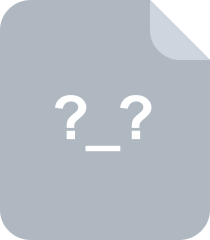
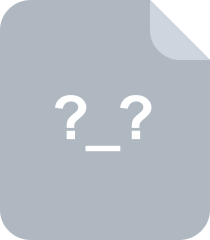
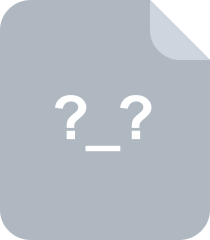
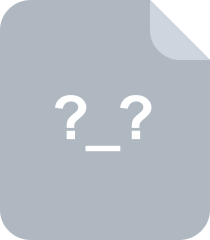
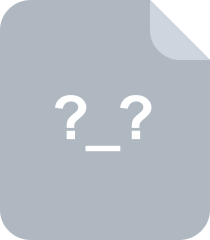
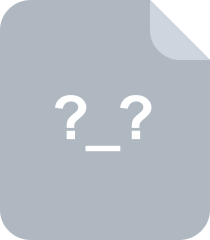
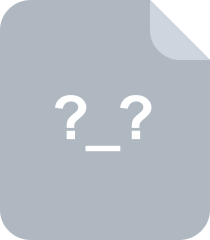
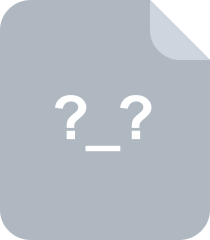
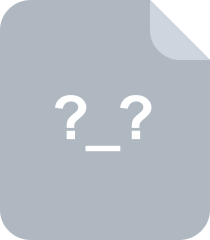
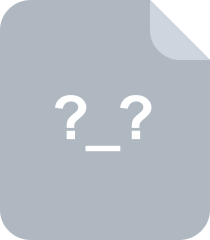
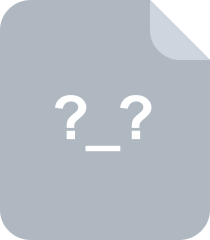
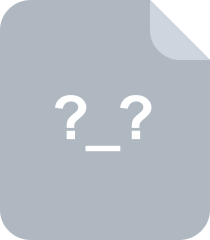
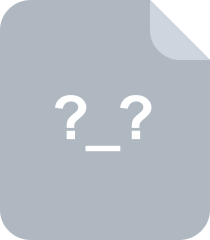
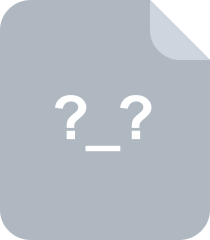
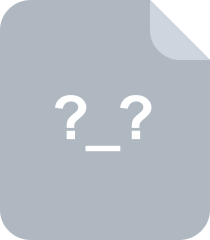
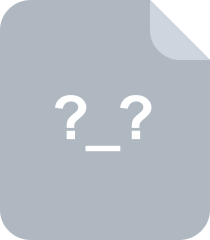
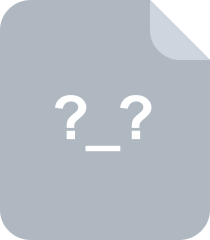
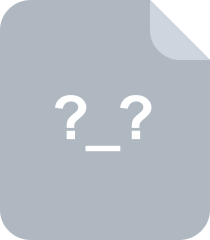
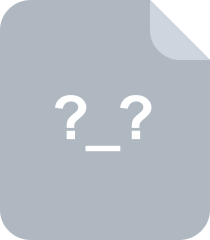
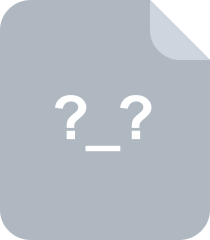
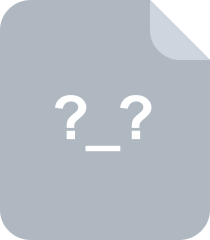
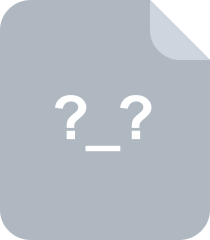
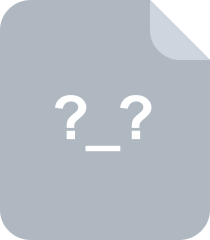
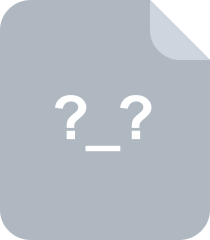
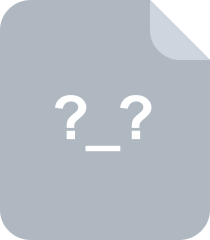
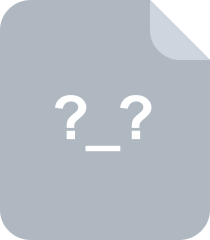
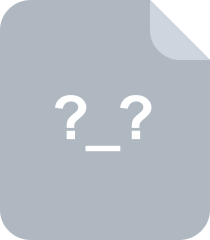
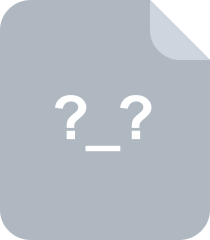
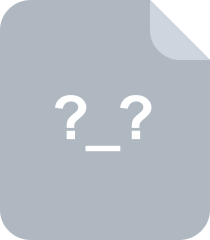
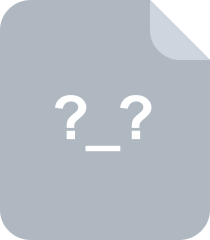
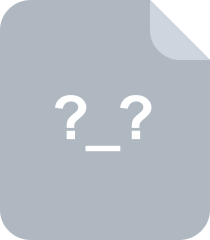
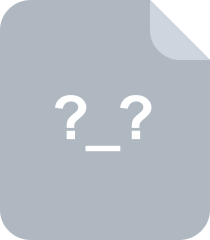
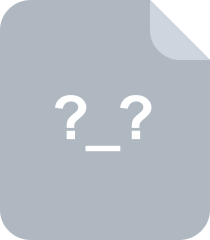
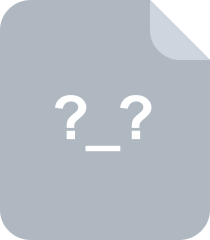
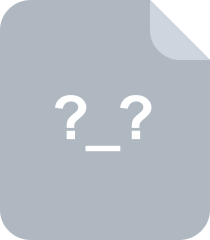
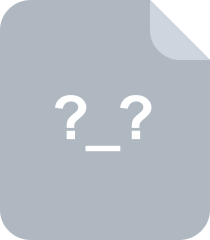
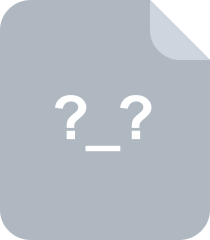
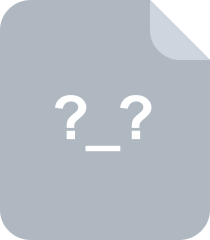
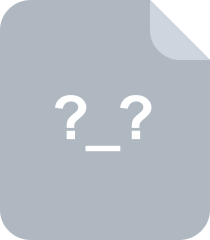
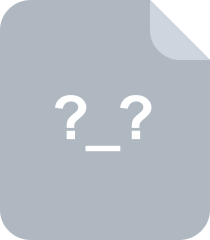
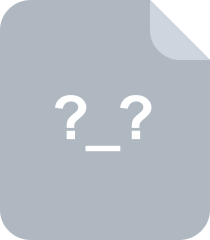
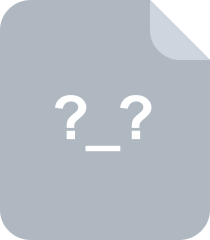
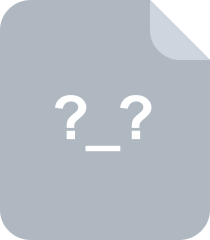
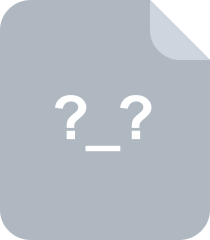
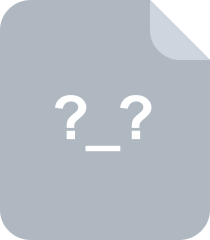
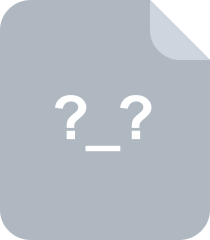
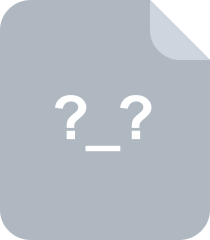
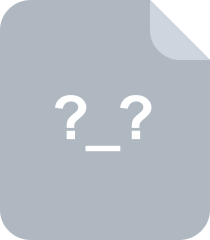
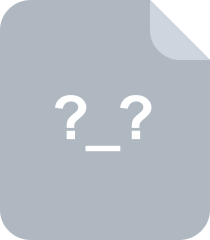
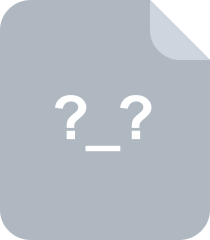
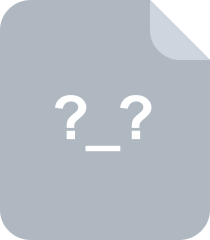
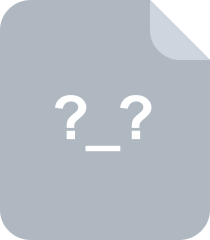
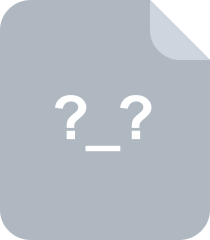
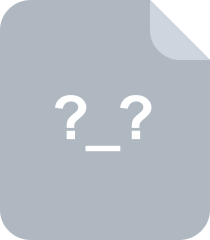
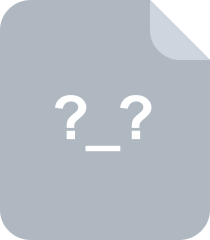
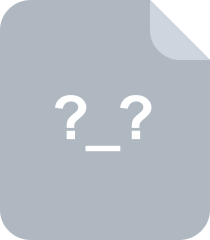
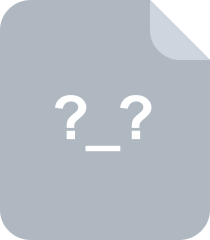
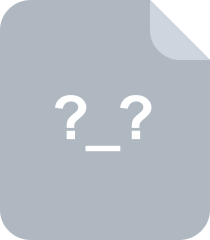
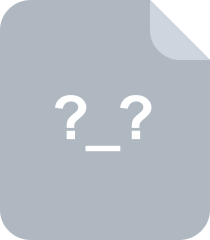
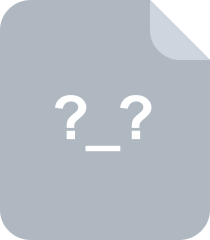
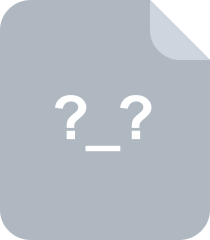
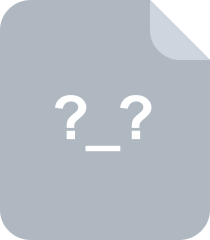
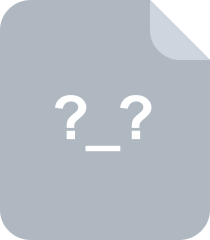
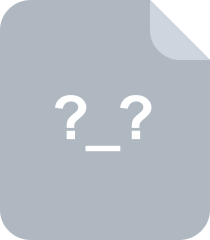
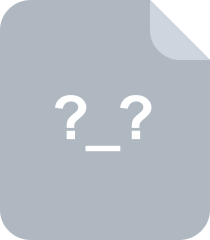
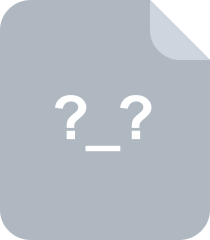
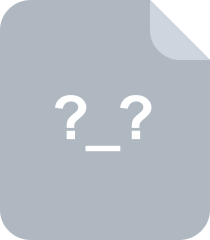
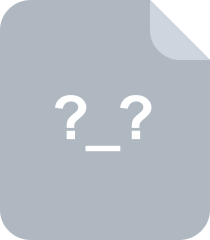
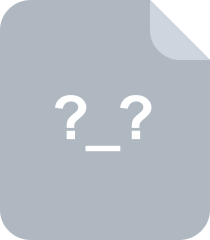
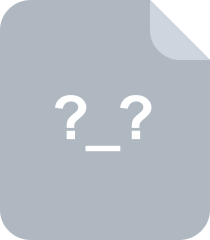
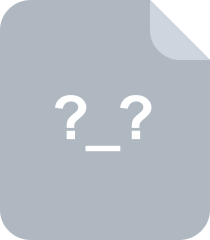
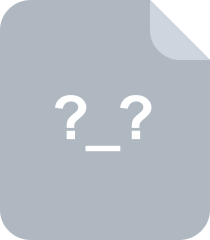
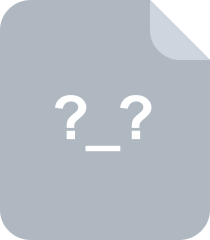
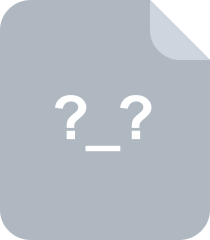
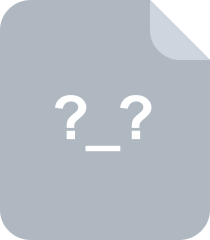
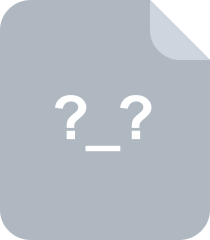
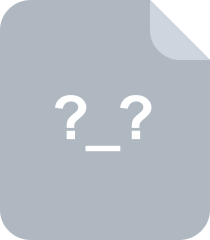
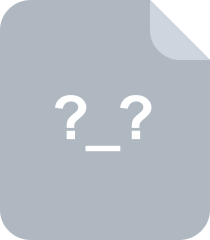
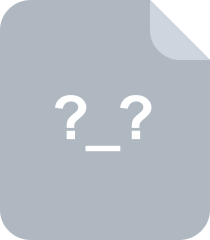
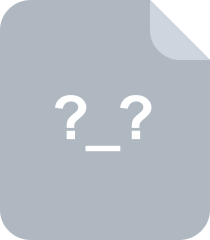
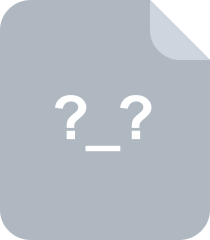
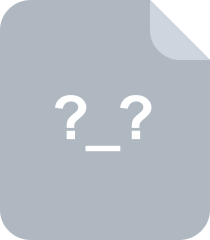
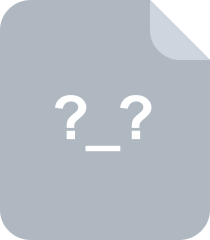
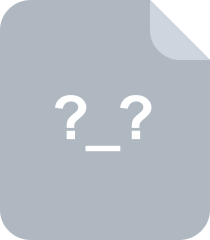
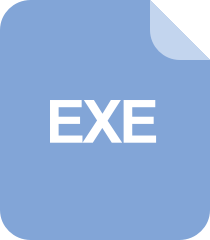
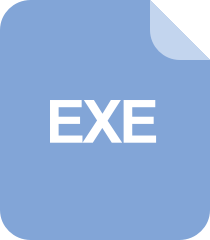
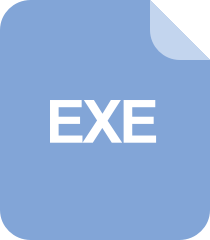
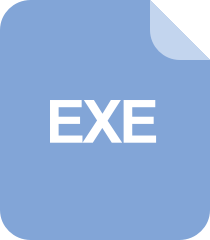
共 578 条
- 1
- 2
- 3
- 4
- 5
- 6
资源评论
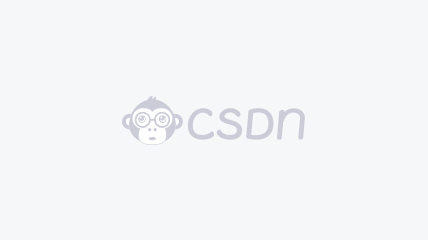

MADMAXS
- 粉丝: 4
- 资源: 10
上传资源 快速赚钱
我的内容管理 展开
我的资源 快来上传第一个资源
我的收益
登录查看自己的收益我的积分 登录查看自己的积分
我的C币 登录后查看C币余额
我的收藏
我的下载
下载帮助

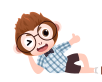
最新资源
- 本资源库是关于“Java Collection Framework API”的参考资料,是 Java 开发社区的重要贡献,旨在提供有关 Java 语言学院 API 的实践示例和递归教育关系 .zip
- 插件: e2eFood.dll
- 打造最强的Java安全研究与安全开发面试题库,帮助师傅们找到满意的工作.zip
- (源码)基于Spark的实时用户行为分析系统.zip
- (源码)基于Spring Boot和Vue的个人博客后台管理系统.zip
- 将流行的 ruby faker gem 引入 Java.zip
- (源码)基于C#和ArcGIS Engine的房屋管理系统.zip
- (源码)基于C语言的Haribote操作系统项目.zip
- (源码)基于Spring Boot框架的秒杀系统.zip
- (源码)基于Qt框架的待办事项管理系统.zip
资源上传下载、课程学习等过程中有任何疑问或建议,欢迎提出宝贵意见哦~我们会及时处理!
点击此处反馈


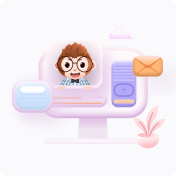
安全验证
文档复制为VIP权益,开通VIP直接复制
