import numpy as np
import matplotlib.pyplot as plt
import time
from skimage import morphology
import cv2
import os
import random
from scipy import ndimage
'''
A*算法
'''
class Node():
"""A node class for A* Pathfinding"""
def __init__(self, parent=None, position=None):
self.parent = parent
self.position = position
self.g = 0
self.h = 0
self.f = 0
def __eq__(self, other):
return self.position == other.position
def astar(maze, start, end, time_limit=1):
"""
Returns a list of tuples as a path from the given start to the given end in the given maze.
if end point is not drivable: return None, 0
if exceed time limit: return None, 1
if no valid path: return None, 2
"""
astar_start_time = time.time()
if maze[end[0]][end[1]] != 0:
return (None, 0)
# Create start and end node
start_node = Node(None, start)
start_node.g = start_node.h = start_node.f = 0
end_node = Node(None, end)
end_node.g = end_node.h = end_node.f = 0
# Initialize both open and closed list
open_list = []
closed_list = []
# Add the start node
open_list.append(start_node)
# Loop until you find the end
while len(open_list) > 0:
if time.time() - astar_start_time >= time_limit:
return (None, 1)
# Get the current node
current_node = open_list[0]
current_index = 0
for index, item in enumerate(open_list):
if item.f < current_node.f:
current_node = item
current_index = index
# Pop current off open list, add to closed list
open_list.pop(current_index)
closed_list.append(current_node)
# Found the goal
if current_node == end_node:
path = []
current = current_node
while current is not None:
path.append(current.position)
current = current.parent
path = path[::-1] # Return reversed path
if len(path) == 0:
return (None, 2)
else:
return (path, -1)
# Generate children
children = []
for new_position in [(0, -1), (0, 1), (-1, 0), (1, 0), (-1, -1), (-1, 1), (1, -1), (1, 1)]: # Adjacent squares
# Get node position
# node_position = (current_node.position[0] + new_position[0], current_node.position[1] + new_position[1])
node_position = [current_node.position[0] + new_position[0], current_node.position[1] + new_position[1]]
# Make sure within range
if node_position[0] > (len(maze) - 1) or node_position[0] < 0 or node_position[1] > (len(maze[len(maze)-1]) -1) or node_position[1] < 0:
continue
# Make sure walkable terrain
if maze[node_position[0]][node_position[1]] != 0:
continue
# Create new node
new_node = Node(current_node, node_position)
# Append
children.append(new_node)
# Loop through children
for child in children:
# Child is on the closed list
for closed_child in closed_list:
if child == closed_child:
continue
# Create the f, g, and h values
child.g = current_node.g + 1
child.h = ((child.position[0] - end_node.position[0]) ** 2) + ((child.position[1] - end_node.position[1]) ** 2)
child.f = child.g + child.h
# Child is already in the open list
for open_node in open_list:
if child == open_node and child.g > open_node.g:
continue
# Add the child to the open list
open_list.append(child)
'''
根据道路分割结果, 规划行驶路线
'''
def find_path(img, time_limit=1, SHOW_IMAGE=False):
'''
固定图像大小, 便于像素长度和实际长度换算
'''
DIM=(800, 600)
'''
开始计时
'''
start_time = time.time()
if SHOW_IMAGE:
plt.imshow(img)
plt.show()
'''
从网络预测大小放缩到拍摄大小
'''
img = cv2.resize(img, DIM, interpolation=cv2.INTER_NEAREST)
img = cv2.cvtColor(img, cv2.COLOR_GRAY2BGR)
'''
矫正图片
'''
K = np.loadtxt('K_SVGA.csv', dtype=np.float32, delimiter=',')
D = np.loadtxt('D_SVGA.csv', dtype=np.float32, delimiter=',')
nK = K.copy()
nK[0,0]=K[0,0]
nK[1,1]=K[1,1]
map1, map2 = cv2.fisheye.initUndistortRectifyMap(K, D, np.eye(3), nK, DIM, cv2.CV_16SC2)
# print('map:', map1, map2)
def undistort(img):
img += 1
undistorted_img = cv2.remap(img, map1, map2, interpolation=cv2.INTER_NEAREST, borderMode=cv2.BORDER_CONSTANT)
return undistorted_img
undistorted_img = undistort(img)
undistorted_img = undistorted_img[:, :, 0]
if SHOW_IMAGE:
plt.imshow(undistorted_img)
plt.show()
'''
鸟瞰图
'''
# 1.读取透视变换矩阵
H = np.loadtxt('H_SVGA.csv', dtype=np.float32, delimiter=',')
# 2.执行透视变换
birdview_img = cv2.warpPerspective(undistorted_img, H, (DIM[1], DIM[0]), flags=cv2.INTER_NEAREST)
birdview_img = birdview_img.swapaxes(1, 0)
if SHOW_IMAGE:
plt.imshow(birdview_img)
plt.show()
'''
获取可行驶区域图
实际尺寸与鸟瞰图像素换算比例为 2mm = 1px
车身半宽为 7.5 cm, 即 37.5 px
'''
# 设置形态学操作的核
kernel_1 = cv2.getStructuringElement(cv2.MORPH_ELLIPSE, (3, 3)) #定义结构元素的形状和大小
kernel_2 = cv2.getStructuringElement(cv2.MORPH_ELLIPSE, (39, 39))
kernel_3 = cv2.getStructuringElement(cv2.MORPH_ELLIPSE, (37, 37))
# 获得 grass 标签
# grass = np.zeros(birdview_img.shape, dtype=np.uint8)
# grass[birdview_img == 2] = 2
grass = birdview_img == 2
# 去除 grass 中误识别出的小区域
if SHOW_IMAGE:
plt.subplot(1, 2, 1)
plt.imshow(grass)
grass = morphology.remove_small_objects(grass, min_size=60, connectivity=1)
grass = grass*np.uint8(2)
# print('back datatype:', grass.dtype)
if SHOW_IMAGE:
plt.subplot(1, 2, 2)
plt.imshow(grass)
plt.show()
# 获得背景标签
back = birdview_img == 3
# 去除背景中误识别出的小区域
if SHOW_IMAGE:
plt.subplot(1, 2, 1)
plt.imshow(back)
back = morphology.remove_small_objects(back, min_size=4000, connectivity=1)
back = back*np.uint8(3)
# print('back datatype:', back.dtype)
if SHOW_IMAGE:
plt.subplot(1, 2, 2)
plt.imshow(back)
plt.show()
# 可视化
if SHOW_IMAGE:
plt.subplot(1, 3, 1)
plt.imshow(birdview_img, 'gray')
# 初始化 drivable_img
drivable_img = np.zeros(birdview_img.shape, dtype=np.uint8)
drivable_img[birdview_img == 1] = 1
# 先扩展 grass 部分
dst = cv2.erode(grass, kernel_1)
dst = cv2.dilate(dst, kernel_2)
drivable_img = np.maximum(drivable_img, dst)
if SHOW_IMAGE:
plt.subplot(1, 3, 2)
plt.imshow(drivable_img, 'gray')
# 再扩展 back 部分
dst = cv2.dilate(back, kernel_3)
# dst = cv2.erode(back, kernel_1)
# dst = cv2.dilate(dst, kernel_2)
drivable_img = np.maximum(drivable_img, dst)
if SHOW_IMAGE:
plt.subplot(1, 3, 3)
plt.imshow(drivable_img, 'gray')
plt.show()
(h, w) = drivable_img.shape
maze = np.zeros((h, w), dtype=np.uint8)
maze[drivable_img==1] = 1
# print(cv2.resize(maze, (20, 20), interpolation=cv2.INTER_NEAREST))
没有合适的资源?快使用搜索试试~ 我知道了~
温馨提示
使用python和百度EsayDL实现自动驾驶算法,使用ESP32开发板作为智能车主控芯片的自动驾驶智能车项目.zip嵌入式优质项目,资源经过严格测试可直接运行成功且功能正常的情况才上传,可轻松copy复刻,拿到资料包后可轻松复现出一样的项目。 本人单片机开发经验充足,深耕嵌入式领域,有任何使用问题欢迎随时与我联系,我会及时为你解惑,提供帮助。 【资源内容】:包含完整源码+工程文件+说明,项目具体内容可查看下方的资源详情。 【附带帮助】: 若还需要嵌入式物联网单片机相关领域开发工具、学习资料等,我会提供帮助,提供资料,鼓励学习进步。 【本人专注嵌入式领域】: 有任何使用问题欢迎随时与我联系,我会及时解答,第一时间为你提供帮助,CSDN博客端可私信,为你解惑,欢迎交流。 【建议小白】: 在所有嵌入式开发中硬件部分若不会画PCB/电路,可选择根据引脚定义将其代替为面包板+杜邦线+外设模块的方式,只需轻松简单连线,下载源码烧录进去便可轻松复刻出一样的项目 【适合场景】: 相关项目设计中,皆可应用在项目开发、毕业设计、课程设计、期末/期中/大作业、工程实训、大创等学科竞赛比赛、初期项目立项、学习/练手等方面中 可借鉴此优质项目实现复刻,也可以基于此项目进行扩展来开发出更多功能
资源推荐
资源详情
资源评论
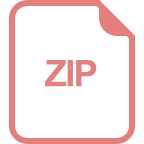
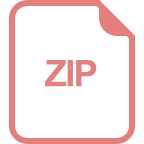
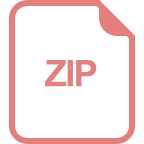
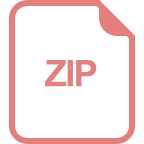
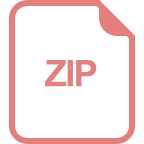
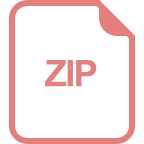
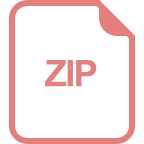
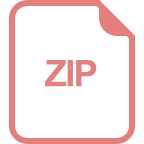
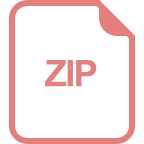
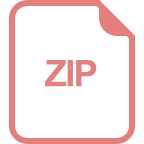
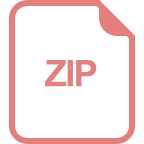
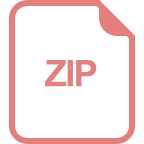
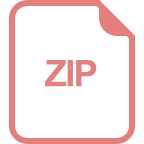
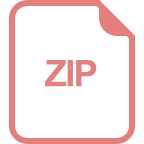
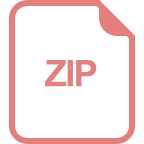
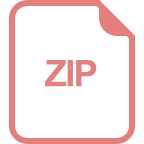
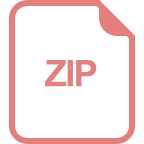
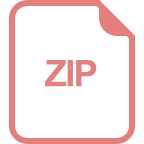
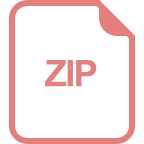
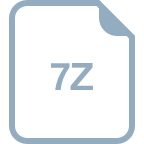
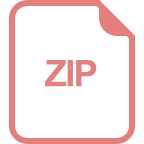
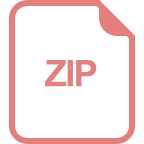
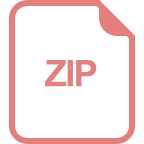
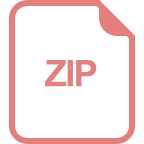
收起资源包目录



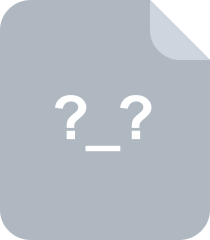

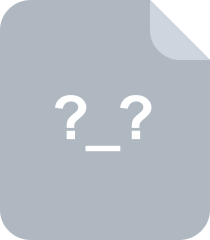
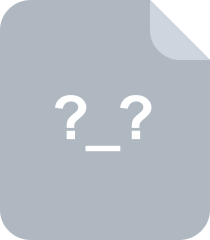
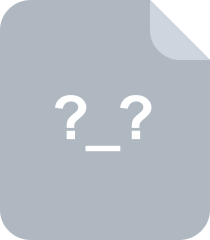

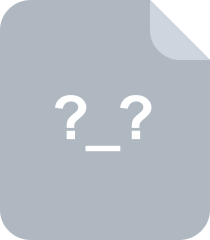
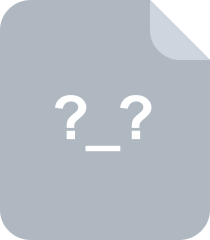
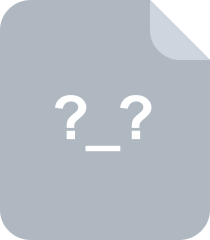

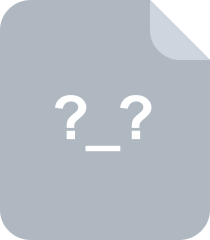
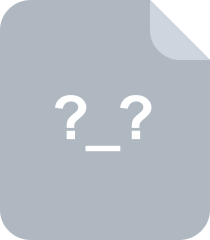
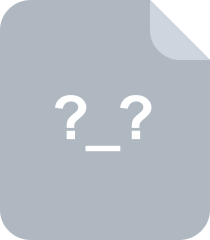
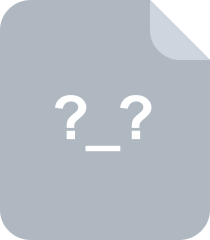

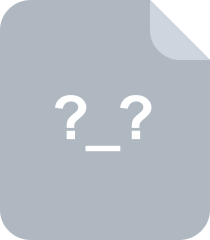
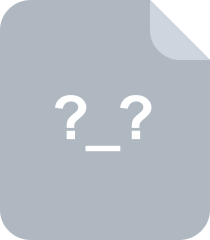
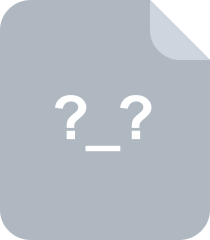
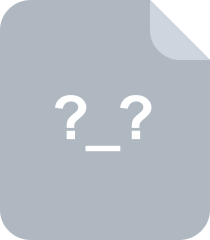


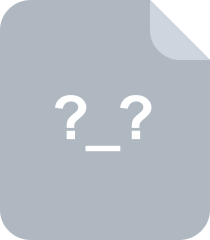
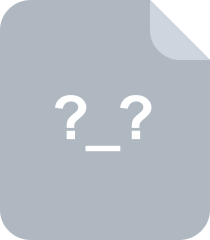

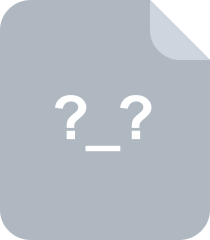
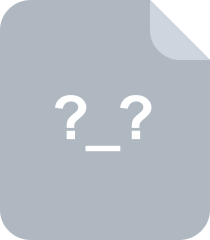
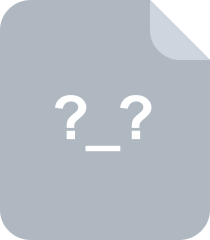

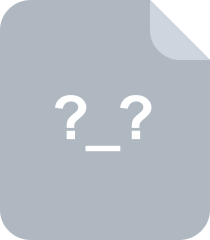

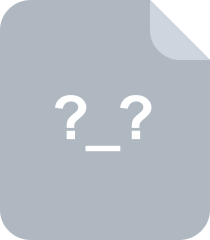

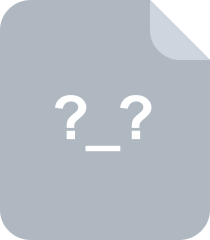
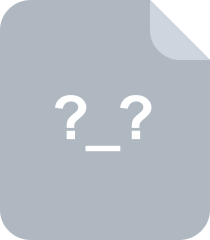
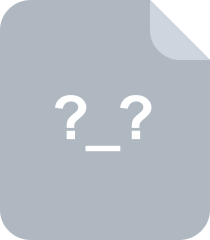
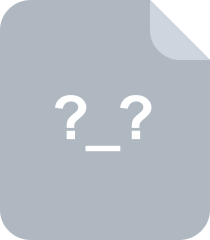
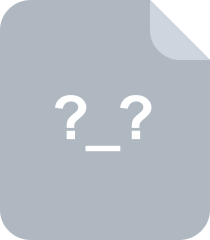

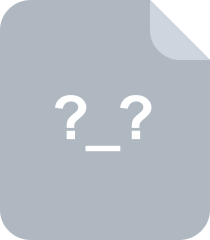

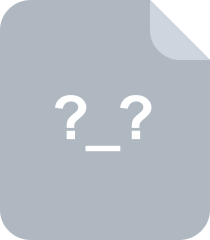
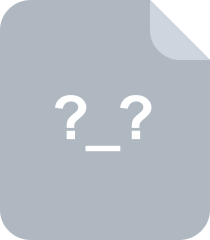
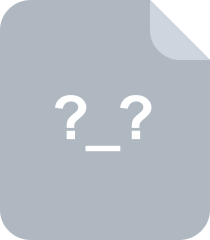
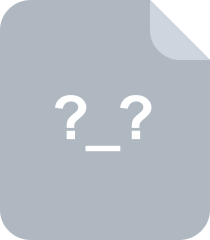
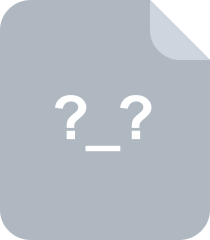
共 33 条
- 1
资源评论
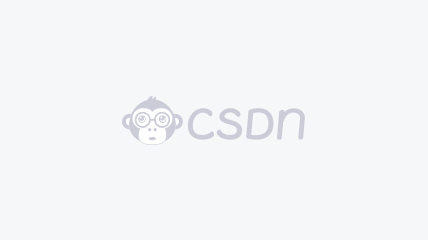

阿齐Archie
- 粉丝: 1w+
- 资源: 2303
上传资源 快速赚钱
我的内容管理 展开
我的资源 快来上传第一个资源
我的收益
登录查看自己的收益我的积分 登录查看自己的积分
我的C币 登录后查看C币余额
我的收藏
我的下载
下载帮助

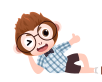
安全验证
文档复制为VIP权益,开通VIP直接复制
