import os
import numpy as np
import math
def sigmoid(x):
"""
Compute the sigmoid of x
Arguments:
x -- A scalar or numpy array of any size
Return:
s -- sigmoid(x)
"""
# write your code here
sig = 1 / (1 + np.exp(-x))
return sig
def softmax(x):
"""Calculates the softmax for the input x.
Argument:
x -- A numpy matrix of shape (n,)
Returns:
s -- A numpy matrix equal to the softmax of x, of shape (n,)
"""
# write your code here
x = np.exp(x) / np.sum(np.exp(x), axis=0, keepdims=True)
return x
def cross_entropy_loss(target, prediction):
"""
Compute the cross entropy loss between target and prediction
Arguments:
target -- the real label, a scalar or numpy array size = (n,)
prediction -- the output of model, a scalar or numpy array, size=(n, c)
Return:
mean loss -- cross_entropy_loss(target, prediction)
"""
# write your code here
delta = 1e-6
return -np.sum(prediction * np.log(target + delta))
def forward(w, b, x):
"""
Arguments:
w -- weights, a numpy array of size (m, 1)
b -- bias, a scalar
x -- data of size (n, m)
Return:
prediction
"""
## write your code here
prediction = sigmoid(x @ w + b)
# print(prediction.shape)
return prediction
def backward(x, target, prediction):
"""
Arguments:
x -- data of size (n, m)
target -- data of size (n, num_class)
prediction -- data of size (n, num_class)
Return:
dw, db
"""
delta = target - prediction
db = delta
dw = sigmoid(x.T) @ delta
return dw, db
# don't edit
if __name__ == '__main__':
## three samples
x = np.array([[12, 3, 7, 4], [3, 10, 4, 9], [9, 6, 2, 0]])
target = np.array([0, 1, 2])
num_class = 3
## learning rate of the gradient descent update rule
learning_rate = 0.001
## one-hot label
target = np.eye(num_class)[target]
n, m = x.shape
w = np.zeros([m, num_class])
b = 0
# three iterations of forward and backward
for i in range(3):
prediction = forward(w, b, x)
loss = cross_entropy_loss(target, softmax(prediction))
dw, db = backward(x, target, prediction)
# update w and b
w = w - learning_rate * dw
b = b - learning_rate * db
print("iter = {}, w = {}, b = {}, loss= {}".format(i, w, b, loss))

timerring
- 粉丝: 17w+
- 资源: 61
最新资源
- 年底三大运营商白嫖话费教程.mp4
- Python入门考试试题集-覆盖语法、函数、数据处理与应用实例
- 智慧校园之家长子-JAVA-基于springBoot智慧校园之家长子系统设计与实现
- 农行领10亓数币红包0亓撸汽水.mp4
- 暖石运营掌握更专业的技能360课时个人发展.mp4
- 爬虫网课资源站做自己资源站无限变现.mp4
- 爬网课资源站发布到自己网站无限变现.mp4
- 拼多多日销千单训练营第31期微付费带流玩法.mp4
- 苹果企业证书 目前可用.mp4
- 基于springboot+vue3+uniapp的点餐小程序源码+数据库+文档说明
- Web开发领域中的WebSocket协议简介及其应用实例
- 大学生科创项目-JAVA-大学生科创项目在线管理系统的设计与实现(毕业论文+开题)
- 基于springboot+vue3+uniapp的点餐小程序源代码+数据库+文档说明(高分毕设)
- 在线互动学习-JAVA-基于springboot在线互动学习网站设计(毕业论文+开题报告)
- main.c.docx
- 全球收音机MyRadio v1.1.99.1024解锁VIP版.mp4
资源上传下载、课程学习等过程中有任何疑问或建议,欢迎提出宝贵意见哦~我们会及时处理!
点击此处反馈


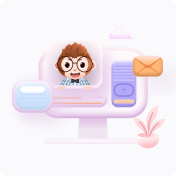
评论0