# PatchmatchNet (CVPR2021 Oral)
official source code of paper 'PatchmatchNet: Learned Multi-View Patchmatch Stereo'

## Updates
- 13.12.2021: New unified format for training and evaluation datasets, support for arbitrary image sizes
and multi-camera setups, and new names for script parameters.
- 27.09.2021: The code now allows for Torchscript export and includes a pre-trained TorchScript module.
## Introduction
PatchmatchNet is a novel cascade formulation of learning-based Patchmatch which aims at decreasing memory consumption and
computation time for high-resolution multi-view stereo. If you find this project useful for your research, please cite:
```
@misc{wang2020patchmatchnet,
title={PatchmatchNet: Learned Multi-View Patchmatch Stereo},
author={Fangjinhua Wang and Silvano Galliani and Christoph Vogel and Pablo Speciale and Marc Pollefeys},
journal={CVPR},
year={2021}
}
```
## Installation
### Requirements
* python 3.8
* CUDA >= 10.1
```
pip install -r requirements.txt
```
## Reproducing Results
* Download our pre-processed dataset:
[DTU's evaluation set](https://drive.google.com/file/d/1jN8yEQX0a-S22XwUjISM8xSJD39pFLL_/view?usp=sharing),
[Tanks & Temples](https://drive.google.com/file/d/1gAfmeoGNEFl9dL4QcAU4kF0BAyTd-r8Z/view?usp=sharing) and
[ETH3D benchmark](https://polybox.ethz.ch/index.php/s/pmTGWobErOnhEg0). Each dataset is already organized as follows:
```
root_directory
├──scan1 (scene_name1)
├──scan2 (scene_name2)
├── images
│ ├── 00000000.jpg
│ ├── 00000001.jpg
│ └── ...
├── cams
│ ├── 00000000_cam.txt
│ ├── 00000001_cam.txt
│ └── ...
└── pair.txt
```
Note:
- The subfolders for Tanks & Temples and ETH3D will not be named `scanN` but the lists included under
`./lists/eth3d` and `./lists/tanks` will have the correct naming conventions.
- If the folders for images and cameras, and the pair file don't follow the standard naming conventions you can modify
the settings of `MVSDataset` in `datasets/mvs.py` to specify the custom `image_folder`, `cam_folder`, and `pair_path`
- The `MVSDataset` is configured by default for JPEG images. If you're using a different format (e.g., PNG) you can change
the `image_extension` parameter of `MVSDataset` accordingly.
Camera file `cam.txt` stores the camera parameters, which includes extrinsic, intrinsic, minimum depth and maximum depth:
```
extrinsic
E00 E01 E02 E03
E10 E11 E12 E13
E20 E21 E22 E23
E30 E31 E32 E33
intrinsic
K00 K01 K02
K10 K11 K12
K20 K21 K22
DEPTH_MIN DEPTH_MAX
```
`pair.txt ` stores the view selection result. For each reference image, N (10 or more) best source views are stored in the file:
```
TOTAL_IMAGE_NUM
IMAGE_ID0 # index of reference image 0
10 ID0 SCORE0 ID1 SCORE1 ... # 10 best source images for reference image 0
IMAGE_ID1 # index of reference image 1
10 ID0 SCORE0 ID1 SCORE1 ... # 10 best source images for reference image 1
...
```
* In `eval.sh`, set `DTU_TESTING`, `ETH3D_TESTING` or `TANK_TESTING` as the root directory of corresponding dataset
and uncomment the evaluation command for corresponding dataset (default is to evaluate on DTU's evaluation set).
If you want to change the output location (default is same as input one), modify the `--output_folder` parameter.
For Tanks the `--scan_list` can be intermediate or advanced and for ETH3D it can be test or train.
* `CKPT_FILE` is the checkpoint file (our pretrained model is `./checkpoints/params_000007.ckpt`), change it if you want
to use your own model. If you want to use the model from the TorchScript module instead, you can specify the checkpoint
file as `./checkpoints/module_000007.pt` and set the option `--input_type module`.
* Test on GPU by running `sh eval.sh`. The code includes depth map estimation and depth fusion. The outputs are the
point clouds in `ply` format.
* For quantitative evaluation on DTU dataset, download [SampleSet](http://roboimagedata.compute.dtu.dk/?page_id=36) and
[Points](http://roboimagedata.compute.dtu.dk/?page_id=36). Unzip them and place `Points` folder in `SampleSet/MVS Data/`.
The structure looks like:
```
SampleSet
├──MVS Data
└──Points
```
In `evaluations/dtu/BaseEvalMain_web.m`, set `dataPath` as path to `SampleSet/MVS Data/`, `plyPath` as directory that
stores the reconstructed point clouds and `resultsPath` as directory to store the evaluation results. Then run
`evaluations/dtu/BaseEvalMain_web.m` in matlab.
The results look like:
| Acc. (mm) | Comp. (mm) | Overall (mm) |
|-----------|------------|--------------|
| 0.427 | 0.277 | 0.352 |
* For detailed quantitative results on Tanks & Temples and ETH3D, please check the leaderboards
([Tanks & Temples](https://www.tanksandtemples.org/details/1170/), [ETH3D](https://www.eth3d.net/result_details?id=216))
## Evaluation on Custom Dataset
* For evaluation, we support preparing the custom dataset from COLMAP's results. The script `colmap_input.py`
(modified based on the script from [MVSNet](https://github.com/YoYo000/MVSNet)) converts COLMAP's sparse reconstruction
results into the same format as the datasets that we provide. After reconstruction, COLMAP will generate a folder
`COLMAP/dense/`, which contains `COLMAP/dense/images/` and `COLMAP/dense/sparse`. Then you need to run like this:
```
python colmap_input.py --input_folder COLMAP/dense/
```
* The default output location is the same as the input one. If you want to change that, set the `--output_folder` parameter.
* The default behavior of the converter will find all possible related images for each source image. If you want to constrain
the max number of related images set the `--num_src_images` parameter.
* In `eval.sh`, set `CUSTOM_TESTING` as the root directory of the dataset, set `--output_folder` as the directory to store
the reconstructed point clouds (default is same as input directory), set `--image_max_dim` to an appropriate size (this
is determined by the available GPU memory and the desired processing speed) or use the native size by removing the
parameter, and uncomment the evaluation command. Test on GPU by running `sh eval.sh`.
## Training
Download pre-processed [DTU's training set](https://polybox.ethz.ch/index.php/s/ugDdJQIuZTk4S35). The dataset is already
organized as follows:
```
root_directory
├── Cameras_1
│ ├── train
│ │ ├── 00000000_cam.txt
│ │ ├── 00000000_cam.txt
│ │ └── ...
│ └── pair.txt
├── Depths_raw
│ ├── scan1
│ │ ├── depth_map_0000.pfm
│ │ ├── depth_visual_0000.png
│ │ ├── depth_map_0001.pfm
│ │ ├── depth_visual_0001.png
│ │ └── ...
│ ├── scan2
│ └── ...
└── Rectified
├── scan1_train
│ ├── rect_001_0_r5000.png
│ ├── rect_001_1_r5000.png
│ ├── ...
│ ├── rect_001_6_r5000.png
│ ├── rect_002_0_r5000.png
│ ├── rect_002_1_r5000.png
│ ├── ...
│ ├── rect_002_6_r5000.png
│ └── ...
├── scan2_train
└── ...
```
To use this dataset directly look into the [Legacy Training](#legacy-training) section below. For the current version of training the
dataset needs to be converted to a format compatible with `MVSDataset` in `./datasets/mvs.py` using the script
`convert_dtu_dataset.py` as follows:
```
python convert_dtu_dataset.py --input_folder <original_dataset> --output_folder <converted_dataset> --scan_list ./lists/dtu/all.txt
```
The converted dataset will now be in a f
没有合适的资源?快使用搜索试试~ 我知道了~
温馨提示
深度学习三维重建 PatchMatchNet——CVPR-2021(源码、原文、注释、译文、批注) 深度学习三维重建 PatchMatchNet——CVPR-2021(源码、原文、注释、译文、批注) 深度学习三维重建 PatchMatchNet——CVPR-2021(源码、原文、注释、译文、批注) 深度学习三维重建 PatchMatchNet——CVPR-2021(源码、原文、注释、译文、批注) 深度学习三维重建 PatchMatchNet——CVPR-2021(源码、原文、注释、译文、批注) 深度学习三维重建 PatchMatchNet——CVPR-2021(源码、原文、注释、译文、批注) 深度学习三维重建 PatchMatchNet——CVPR-2021(源码、原文、注释、译文、批注) 深度学习三维重建 PatchMatchNet——CVPR-2021(源码、原文、注释、译文、批注) 深度学习三维重建 PatchMatchNet——CVPR-2021(源码、原文、注释、译文、批注) 深度学习三维重建 PatchMatchNet——CVPR-2021(源码、原文、注释、译文、批注)
资源推荐
资源详情
资源评论
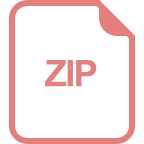
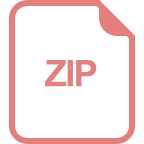
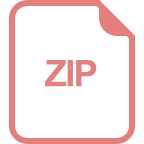
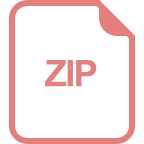
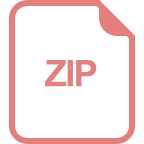
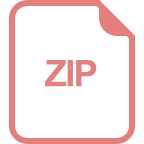
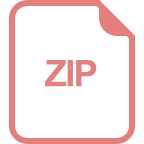
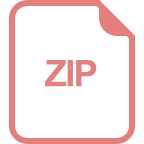
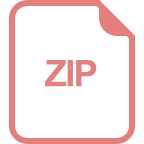
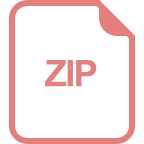
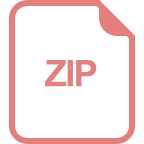
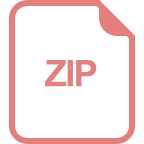
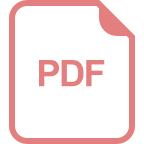
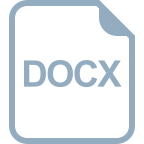
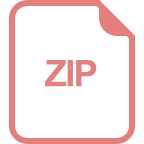
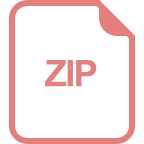
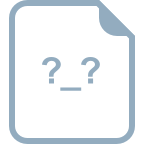
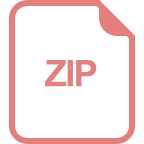
收起资源包目录

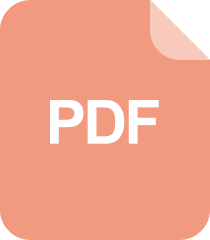
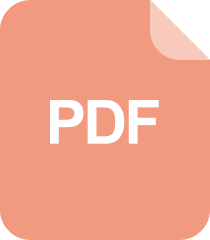
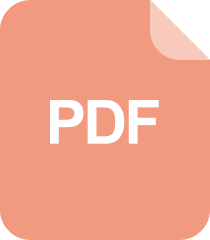
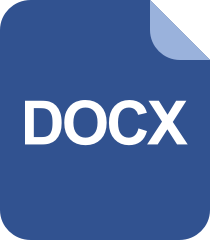

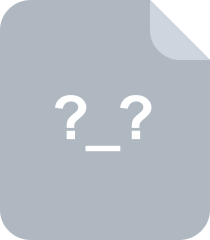
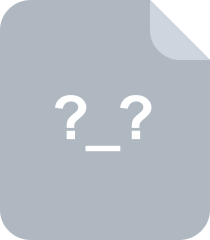
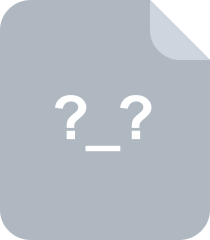
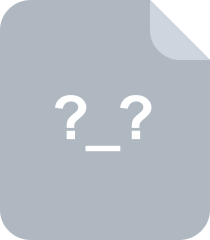
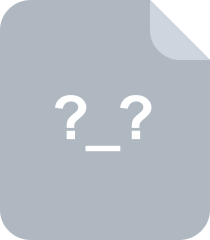
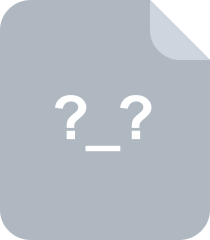


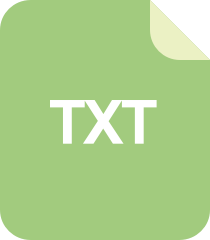
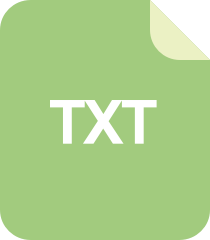

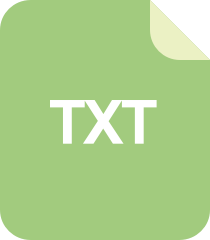
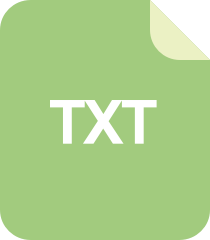
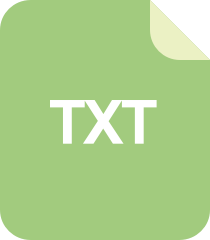
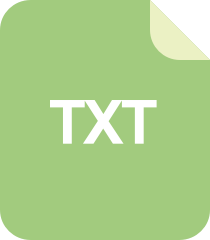

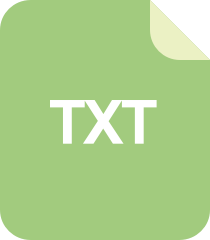
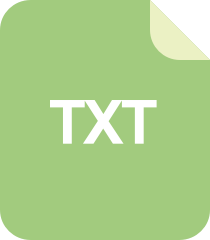

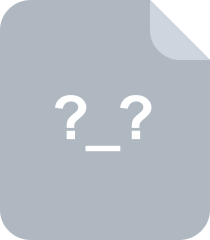
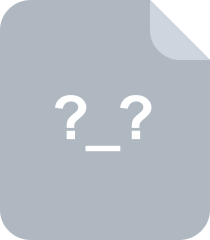
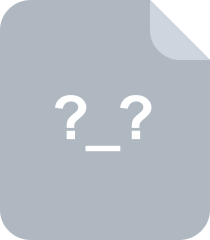
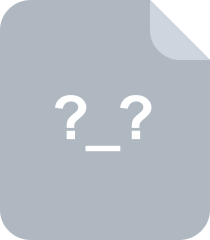


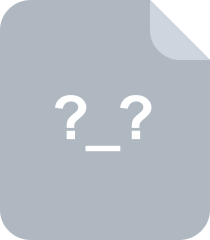
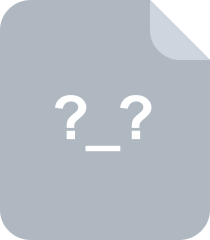
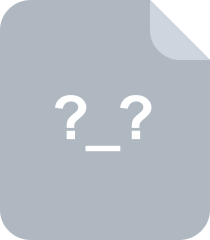
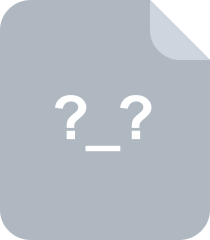
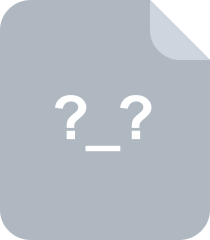
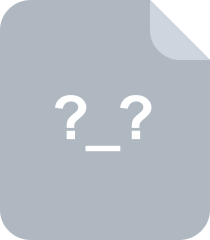
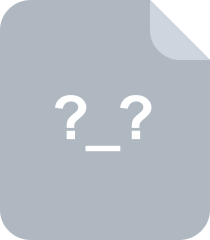
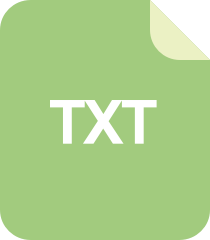
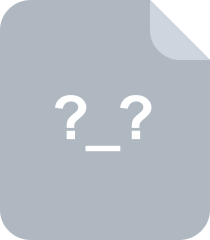

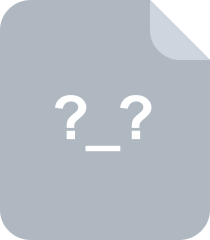
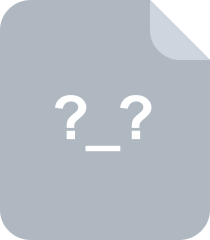
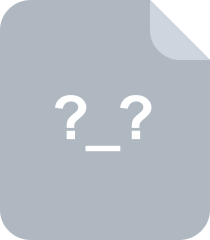
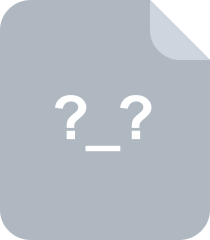

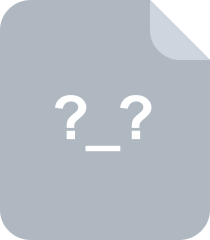
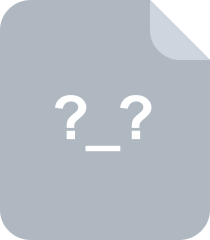
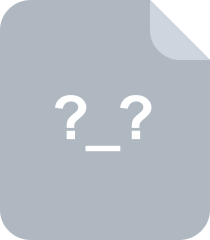
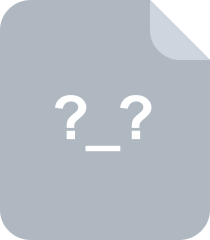
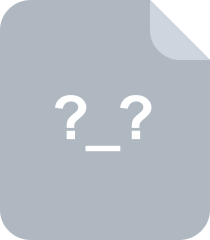
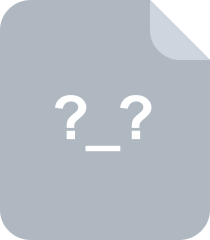
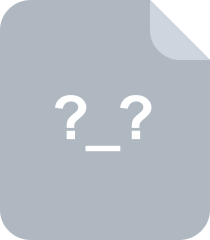
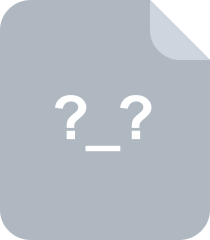
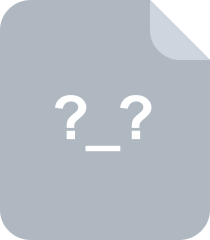

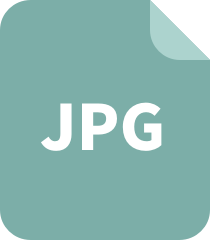
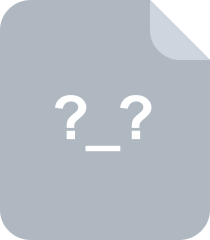
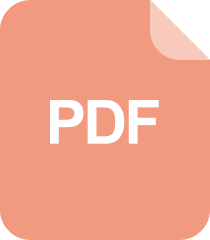
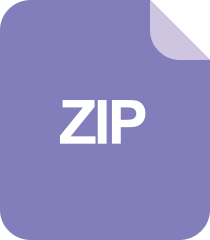
共 48 条
- 1
资源评论
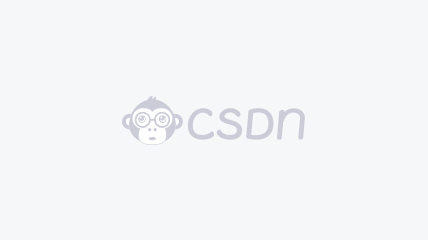

R-G-B
- 粉丝: 1822
- 资源: 113
上传资源 快速赚钱
我的内容管理 展开
我的资源 快来上传第一个资源
我的收益
登录查看自己的收益我的积分 登录查看自己的积分
我的C币 登录后查看C币余额
我的收藏
我的下载
下载帮助

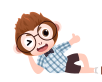
最新资源
- 基于树莓派的3D全息电子宠物嵌入式计算课程设计详细文档+全部资料+高分项目+源码.zip
- 基于指纹识别和指静脉识别技术的嵌入式门禁系统,DSP硬件平台详细文档+全部资料+高分项目+源码.zip
- FGT-80C-v400-build0458-FORTINET.out
- javascript各种算法源代码最全的算法技术资料.zip
- FGT-80C-v400-build0441-FORTINET.out
- 2025元旦倒计时雪花背景特效源码
- python-geohash-0.8.5-cp37-cp37m-win-amd64
- js各种算法源代码最全的算法技术资料.zip
- 实现财富自由的路径PPT
- go语言各种排序算法源代码最全的算法技术资料.zip
- 如何实现财富自由的分析PPT
- 电脑端微信自动锁定2.0
- 个人自我介绍、风采展示PPT
- python语法合集-python语法知识PDF
- Python数据可视化之Seaborn库详解与使用实例
- 俄罗斯大学录取数据集,大学招收数据集(5568行)
资源上传下载、课程学习等过程中有任何疑问或建议,欢迎提出宝贵意见哦~我们会及时处理!
点击此处反馈


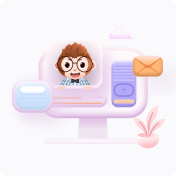
安全验证
文档复制为VIP权益,开通VIP直接复制
