# MediaSequence keys and functions reference
The documentation below will first provide an overview of using MediaSequence
for machine learning tasks. Then, the documentation will describe the function
prototypes used in MediaSequence for storing multimedia data in
SequenceExamples. Finally, the documentation will describe the specific keys for
storing specific types of data.
## Overview of MediaSequence for machine learning
The goal of MediaSequence is to provide a tool for transforming annotations of
multimedia into input examples ready for use with machine learning models in
TensorFlow. The most semantically appropriate data type for this task that can
be easily parsed in TensorFlow is
tensorflow.train.SequenceExamples/tensorflow::SequenceExamples.
Using SequenceExamples enables quick integration of new
features into TensorFlow pipelines, easy open sourcing of models and data,
reasonable debugging, and efficient TensorFlow decoding. For many machine
learning tasks, TensorFlow Examples are capable of fulfilling that role.
However, Examples can become unwieldy for sequence data, particularly when the
number of features per timestep varies, creating a ragged struction. Video
object detection is one example task that requires this ragged structure because
the number of detections per frame varies. SequenceExamples can easily encode
this ragged structure. Sequences naturally match the semantics of video as a
sequence of frames or other common media patterns. The interpretable semantics simplify debugging and decoding of
potentially complicated data. One potential disadvantage of SequenceExamples is
that keys and formats can vary widely. The MediaSequence library provides tools
for consistently manipulating and decoding SequenceExamples in Python and C++ in
a consistent format. The consistent format enables creating a pipeline for
processing data sets. A goal of MediaSequence as a pipeline is that users should
only need to specify the metadata (e.g. videos and labels) for their task. The
pipeline will turn the metadata into training data.
The pipeline has two stages. First, users must generate the metadata
describing the data and applicable labels. This process is
straightforward and described in the next section. Second, users run MediaPipe
graphs with the `UnpackMediaSequenceCalculator` and
`PackMediaSequenceCalculators` to extract the relevant data from multimedia
files. A sequence of graphs can be chained together in this second stage to
achieve complex processing such as first extracting a subset of frames from a
video and then extracting deep features or object detections for each extracted
frame. As MediaPipe is built to simply and reproducibly process media files,
the two stage approach separates and simplifies data management.
### Creating metadata for a new data set
Generating examples for a new data set typically only requires defining the
metadata. MediaPipe graphs can interpret this metadata to fill out the
SequenceExamples using the `UnpackMediaSequenceCalculator` and
`PackMediaSequenceCalculator`. This section will list the metadata required for
different types of tasks and provide a limited descripiton for the data filled
by MediaPipe. The input media will be referred to as video because that is a
common case, but audio files or other sequences could be supported. The function
calls in the Python API will be used in examples, and the equivalent C++ calls
are described below.
The video metadata is a way to access the video, using `set_clip_data_path` to
define the path on disk, and the time span to include using
`set_clip_start_timestamp` and `set_clip_end_timestamp`. The data path can be
absolute or can be relative to a root directory passed to the
`UnpackMediaSequenceCalculator`. The start and end timestamps should be valid
MediaPipe timestamps in microseconds. Given this information, the pipeline can
extract the portion of the media between the start and end timestamps. If you do
not specify a start time, the video is decoded from the beginning. If you do not
specify an end time, the entire video is decoded. The start and end times are
not filled if left empty.
The features extracted from the video depends on the MediaPipe graph that is
run. The documentation of keys below and in `PackMediaSequenceCalculator`
provide the best description.
The annotations including labels should be added as metadata. They will be
passed through the MediaPipe pipeline unchanged. The label format will vary
depending on the task you want to do. Several examples are included below. In
general, the MediaPipe processing is independent of any labels that you provide:
only the clip data path, start time, and end time matter.
#### Clip classification
For clip classification, e.g. is this video clip about basketball?, you
should use `set_clip_label_index` with the integer index of the correct class
and `set_clip_label_string` with the human readable version of the correct class.
The index is often used when training the model and the string is used for
human readable debugging. The same number of indices and strings need to be
provided. The association between the two is just their relative positions in
the list.
##### Example lines creating metadata for clip classification
```python
# Python: functions from media_sequence.py as ms
sequence = tf.train.SequenceExample()
ms.set_clip_data_path(b"path_to_video", sequence)
ms.set_clip_start_timestamp(1000000, sequence)
ms.set_clip_end_timestamp(6000000, sequence)
ms.set_clip_label_index((4, 3), sequence)
ms.set_clip_label_string((b"run", b"jump"), sequence)
```
```c++
// C++: functions from media_sequence.h
tensorflow::SequenceExample sequence;
SetClipDataPath("path_to_video", &sequence);
SetClipStartTimestamp(1000000, &sequence);
SetClipEndTimestamp(6000000, &sequence);
SetClipLabelIndex({4, 3}, &sequence);
SetClipLabelString({"run", "jump"}, &sequence);
```
#### Temporal detection
For temporal event detection or localization, e.g. classify regions in time
where people are playing a sport, the labels are referred to as segments. You
need to set the segment timespans with `set_segment_start_timestamp` and
`set_segment_end_timestamp` and labels with `set_segment_label_index` and
`set_segment_label_string`. All of these are repeated fields so you can provide
multiple segments for each clip. The label index and string have the same
meaning as for clip classification. Only the start and end timestamps need to
be provided. (The pipeline will automatically call `set_segment_start_index` to
the index of the image frame under the image/timestamp key that is closest in
time, and similarly for `set_segment_end_index`. Allowing the pipeline to fill
in the indices corrects for frame rate changes automatically.) The same number
of values must be present in each field. If the same segment would have
multiple labels, the segment start and end time must be duplicated.
##### Example lines creating metadata for temporal detection
```python
# Python: functions from media_sequence.py as ms
sequence = tf.train.SequenceExample()
ms.set_clip_data_path(b"path_to_video", sequence)
ms.set_clip_start_timestamp(1000000, sequence)
ms.set_clip_end_timestamp(6000000, sequence)
ms.set_segment_start_timestamp((2000000, 4000000), sequence)
ms.set_segment_end_timestamp((3500000, 6000000), sequence)
ms.set_segment_label_index((4, 3), sequence)
ms.set_segment_label_string((b"run", b"jump"), sequence)
```
```c++
// C++: functions from media_sequence.h
tensorflow::SequenceExample sequence;
SetClipDataPath("path_to_video", &sequence);
SetClipStartTimestamp(1000000, &sequence);
SetClipEndTimestamp(6000000, &sequence);
SetSegmentStartTimestamp({2000000, 4000000}, &sequence);
SetSegmentEndTimestamp({3500000, 6000000}, &sequence);
SetSegmentLabelIndex({4, 3}, &sequence);
SetSegmentLabelString({"run", "jump"}, &sequence);
```
#### Tracking and spatiotemporal detection
For object tracking or detection in videos, e.g. classify regions in tim
没有合适的资源?快使用搜索试试~ 我知道了~
温馨提示
在import模型时,有时候因为网络问题无法加载mediapipe模型。可以将附件拷贝到对应的目录即可。具体报错如下:TimeoutError: [WinError 10060] 由于连接方在一段时间后没有正确答复或连接的主机没有反应,连 接尝试失败
资源推荐
资源详情
资源评论
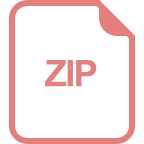
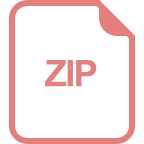
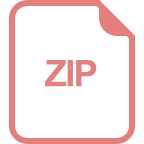
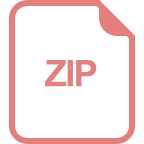
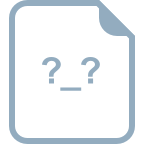
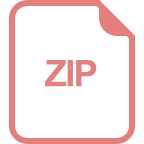
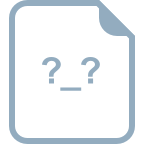
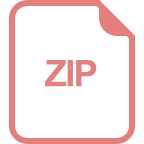
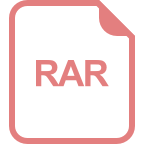
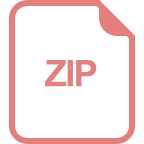
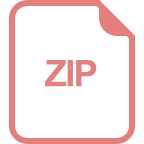
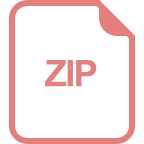
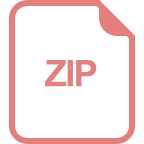
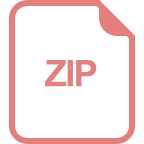
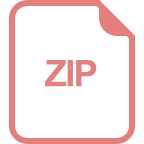
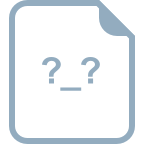
收起资源包目录

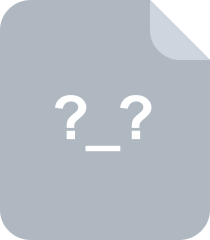
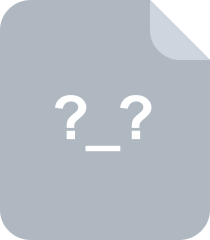
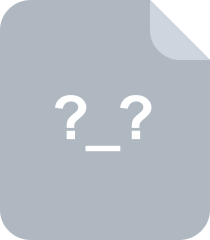
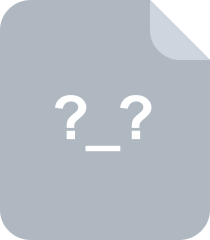
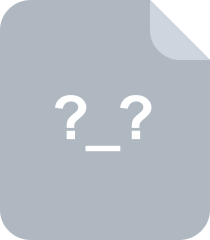
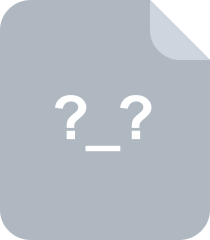
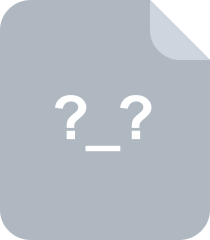
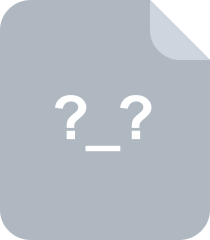
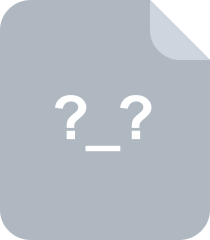
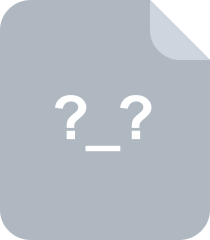
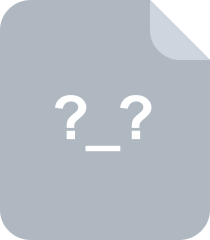
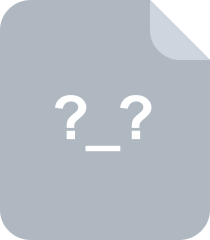
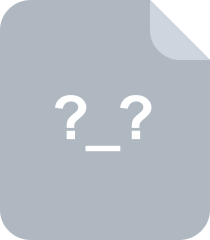
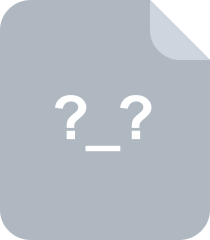
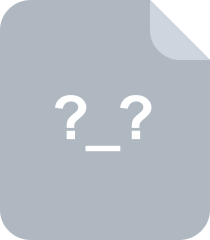
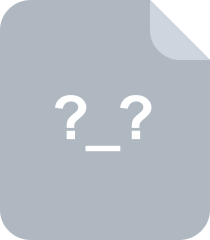
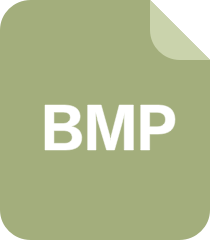
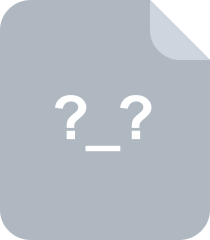
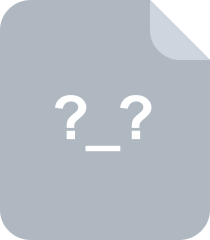
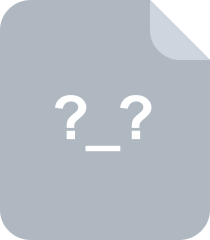
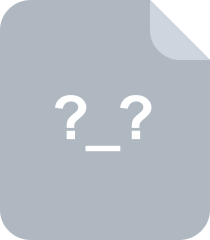
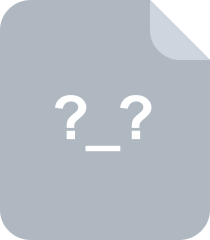
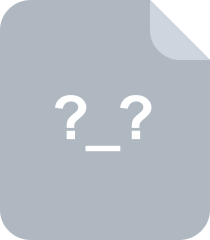
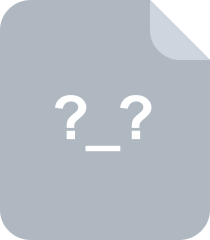
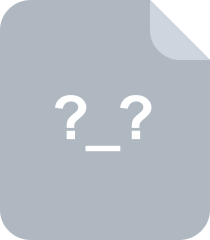
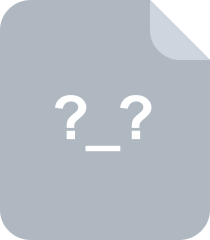
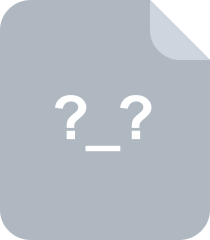
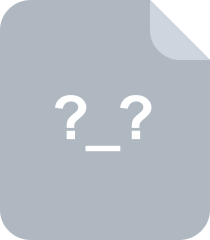
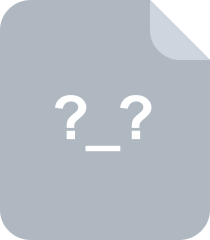
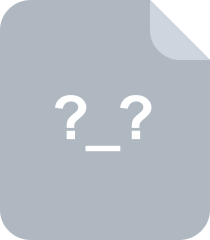
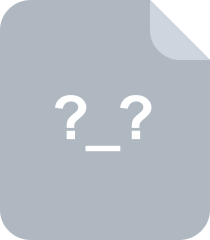
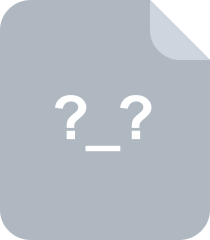
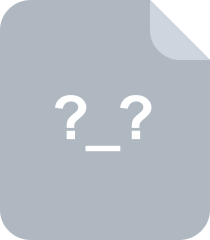
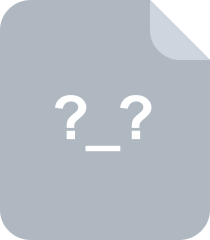
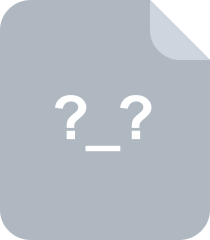
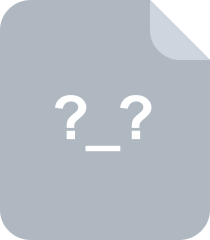
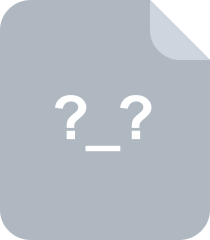
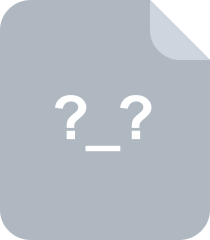
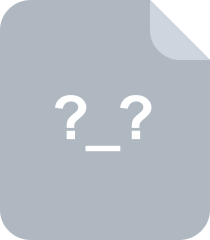
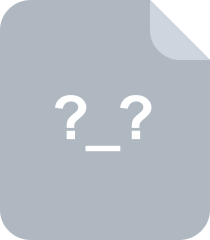
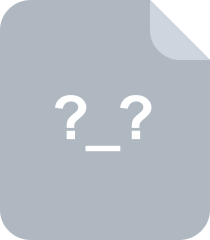
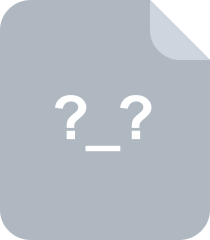
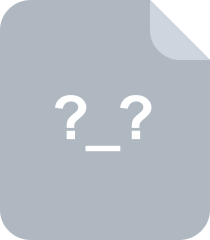
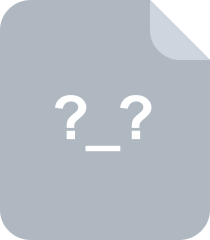
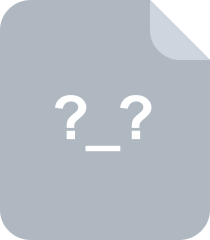
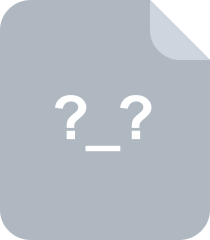
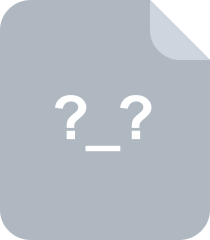
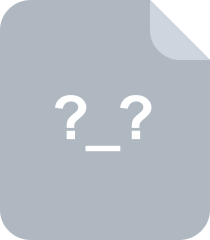
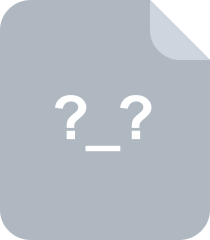
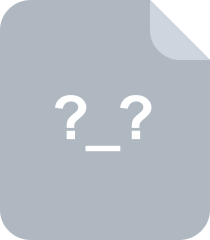
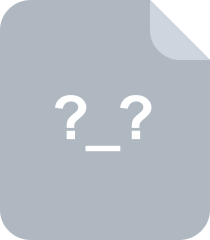
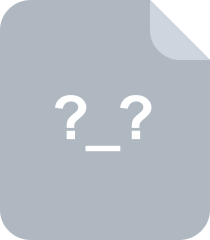
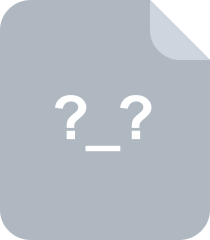
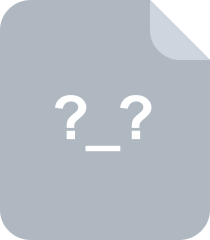
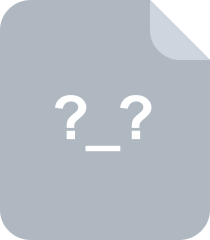
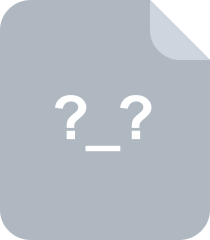
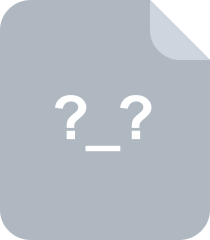
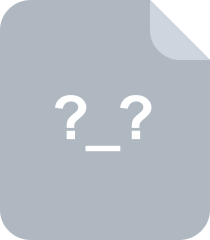
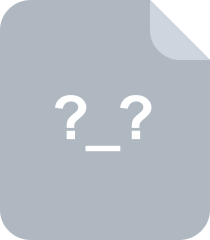
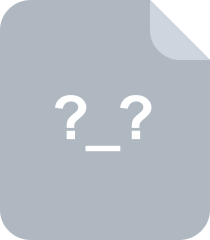
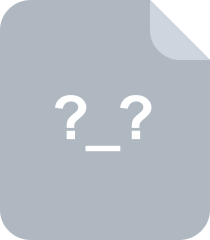
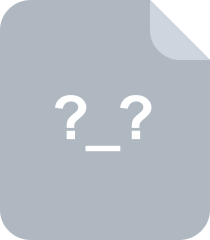
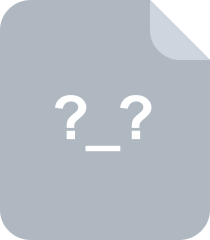
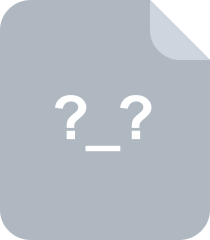
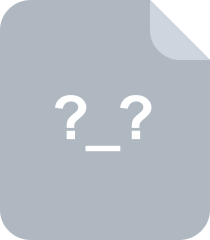
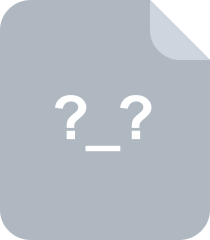
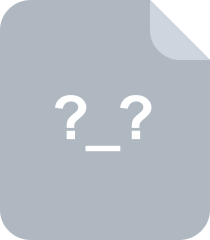
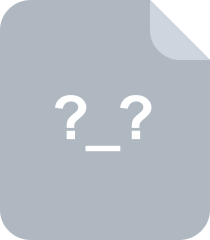
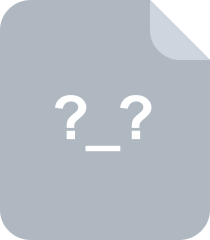
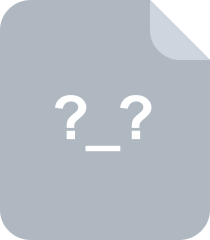
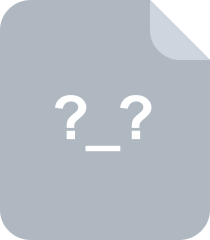
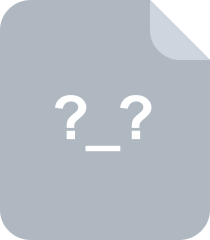
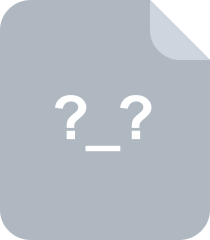
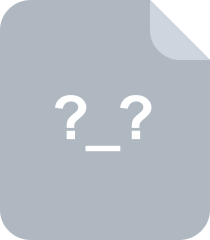
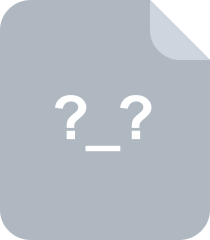
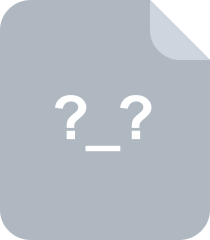
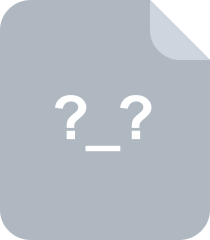
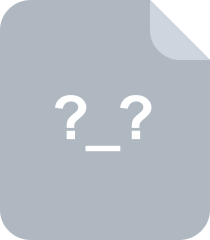
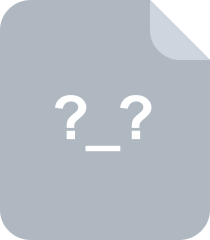
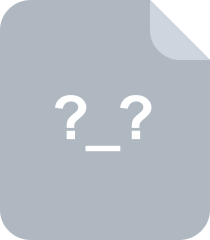
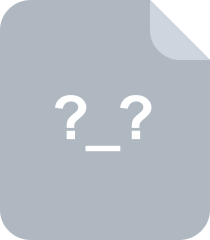
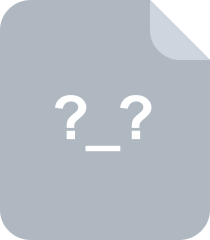
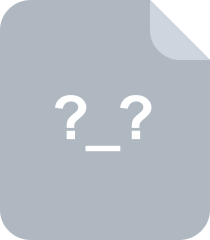
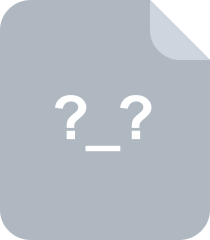
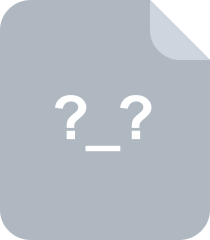
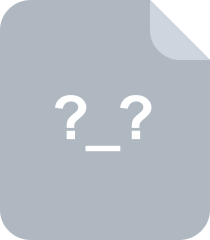
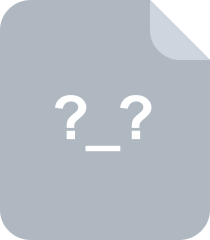
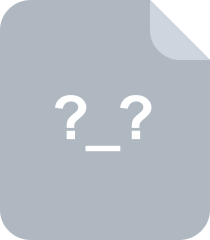
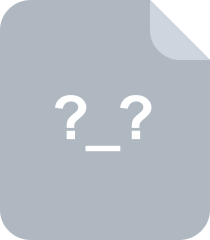
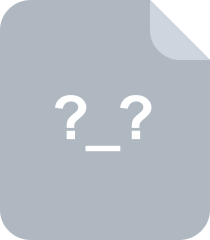
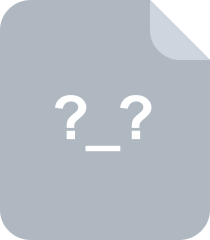
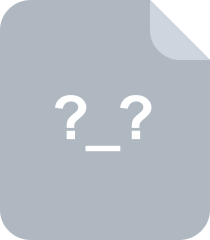
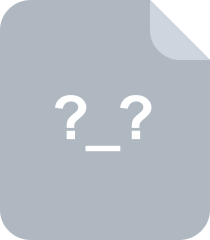
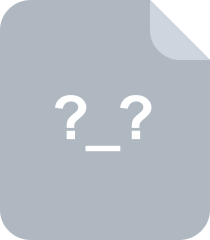
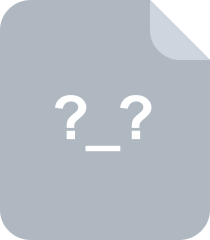
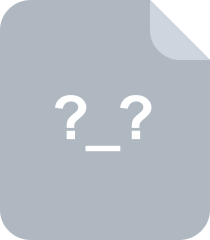
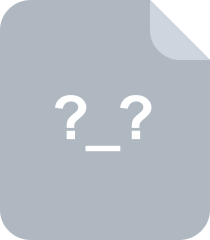
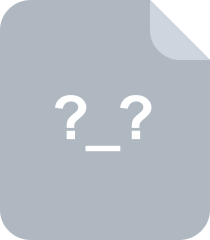
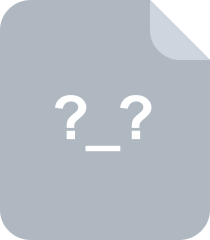
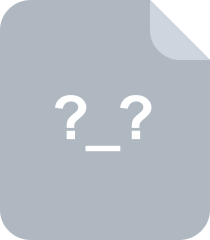
共 2386 条
- 1
- 2
- 3
- 4
- 5
- 6
- 24


lzscan
- 粉丝: 2
- 资源: 2
上传资源 快速赚钱
我的内容管理 展开
我的资源 快来上传第一个资源
我的收益
登录查看自己的收益我的积分 登录查看自己的积分
我的C币 登录后查看C币余额
我的收藏
我的下载
下载帮助

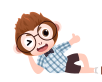
最新资源
资源上传下载、课程学习等过程中有任何疑问或建议,欢迎提出宝贵意见哦~我们会及时处理!
点击此处反馈


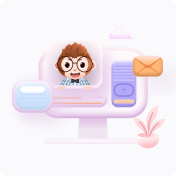
安全验证
文档复制为VIP权益,开通VIP直接复制

- 1
- 2
前往页