gensim-3.7.3-cp35-cp35m-manylinux1_x86_64.whl.zip
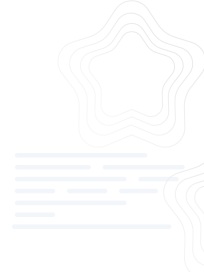

《 gensim 库的安装与应用详解》 gensim 是一个强大的Python库,主要用于处理文本数据,特别是主题建模和文档相似性分析。这个压缩包文件 "gensim-3.7.3-cp35-cp35m-manylinux1_x86_64.whl.zip" 内含 gensim 的特定版本3.7.3,适用于Python 3.5,且为64位系统。文件名中的 "cp35" 表示它兼容Python 3.5,"cp35m" 指的是针对该Python版本的优化构建,"manylinux1_x86_64" 则意味着它是针对多种Linux平台的优化版本,特别是x86_64架构的系统。 在Python环境中,通常我们使用pip来安装第三方库,但对于.whl这样的二进制文件,我们需要先解压后使用。使用解压缩工具打开 "gensim-3.7.3-cp35-cp35m-manylinux1_x86_64.whl.zip",解压后会得到一个名为 "gensim-3.7.3-cp35-cp35m-manylinux1_x86_64.whl" 的文件。接下来,通过pip安装: ```bash pip install gensim-3.7.3-cp35-cp35m-manylinux1_x86_64.whl ``` 安装完成后,你便可以导入gensim库并开始利用其功能。gensim主要提供了以下核心模块: 1. **Corpora**:处理文本数据的工具,包括分词、去除停用词等预处理步骤。 2. **Models**:提供了多种主题模型,如Latent Dirichlet Allocation (LDA)、Hierarchical Dirichlet Process (HDP),以及TF-IDF和LSI等。 3. **Similarity**:提供了一种基于余弦相似度的索引方法,用于快速查找文档间的相似性。 4. **Distributed**:支持多线程和分布式计算,提升大规模文本处理的效率。 5. ** Corpora and Documents**:包括字典(Dictionary)和文档集合(Corpus)的表示方式,是gensim处理文本的基础。 在 "使用说明.txt" 文件中,可能包含了详细的安装指南和使用示例,对于初学者来说非常有用。建议在安装完成后仔细阅读,以便更好地理解和运用gensim。 例如,你可以使用gensim创建一个TF-IDF模型,然后对新的文档进行向量化表示: ```python from gensim import corpora, models, similarities # 假设我们有以下文档数据 documents = ["Human machine interface for lab abc computer applications", "A survey of user opinion of computer system response time", "The EPS user interface management system", "System and human system engineering testing of EPS", "Relation of user perceived response time to error measurement", "The generation of random binary unordered trees", "The intersection graph of paths in trees", "Graph minors IV Widths of trees and well quasi ordering", "Graph minors A survey"] # 对文档进行预处理 texts = [[word for word in document.lower().split()] for document in documents] # 创建字典 dictionary = corpora.Dictionary(texts) # 将文档转换为向量 corpus = [dictionary.doc2bow(text) for text in texts] # 训练TF-IDF模型 tfidf = models.TfidfModel(corpus) # 创建相似性索引 index = similarities.MatrixSimilarity(tfidf[corpus]) # 对新文档进行处理 new_document = "Human computer interaction" new_bow = dictionary.doc2bow(new_document.lower().split()) # 转换新文档的向量 new_tfidf = tfidf[new_bow] # 查找新文档与原有文档的相似度 similarity_scores = index[new_tfidf] ``` 以上代码展示了如何使用gensim处理文本数据,构建TF-IDF模型,并进行文档相似性检索。gensim的强大之处在于它的灵活性和扩展性,可以根据具体需求调整模型参数或自定义模型。 gensim是一个强大的文本分析库,适合进行大规模文本数据的处理和分析。无论是做主题建模、文档相似性比较还是其他自然语言处理任务,gensim都能提供高效且易用的解决方案。对于Python开发者而言,掌握gensim的使用将极大地提升处理文本数据的能力。
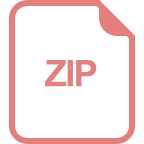
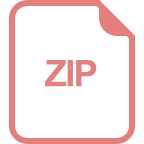
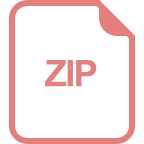
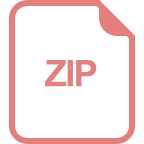
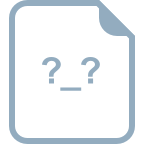
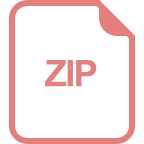
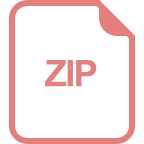
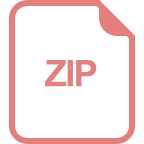
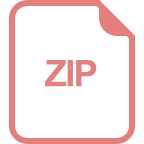
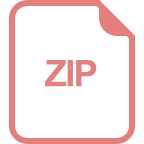
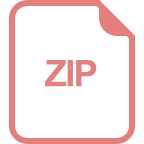
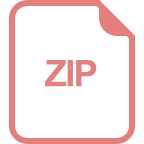
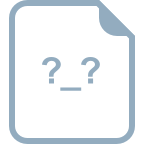
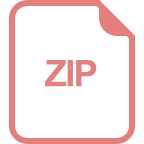
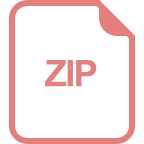
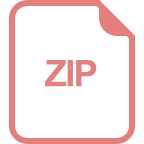
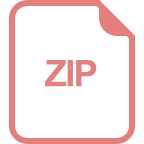
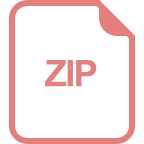
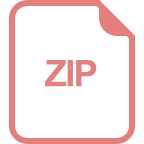
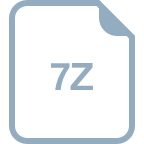
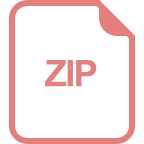
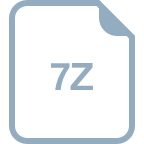
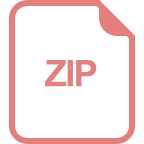
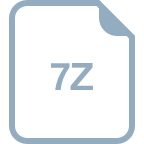

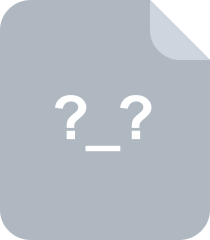
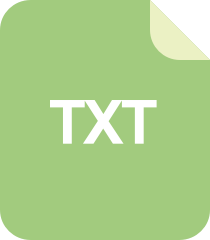
- 1
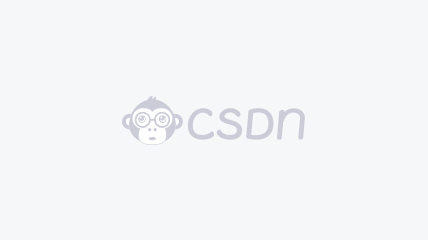

- 粉丝: 5115
- 资源: 7439
我的内容管理 展开
我的资源 快来上传第一个资源
我的收益
登录查看自己的收益我的积分 登录查看自己的积分
我的C币 登录后查看C币余额
我的收藏
我的下载
下载帮助

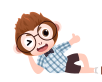
最新资源

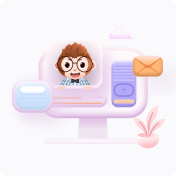
