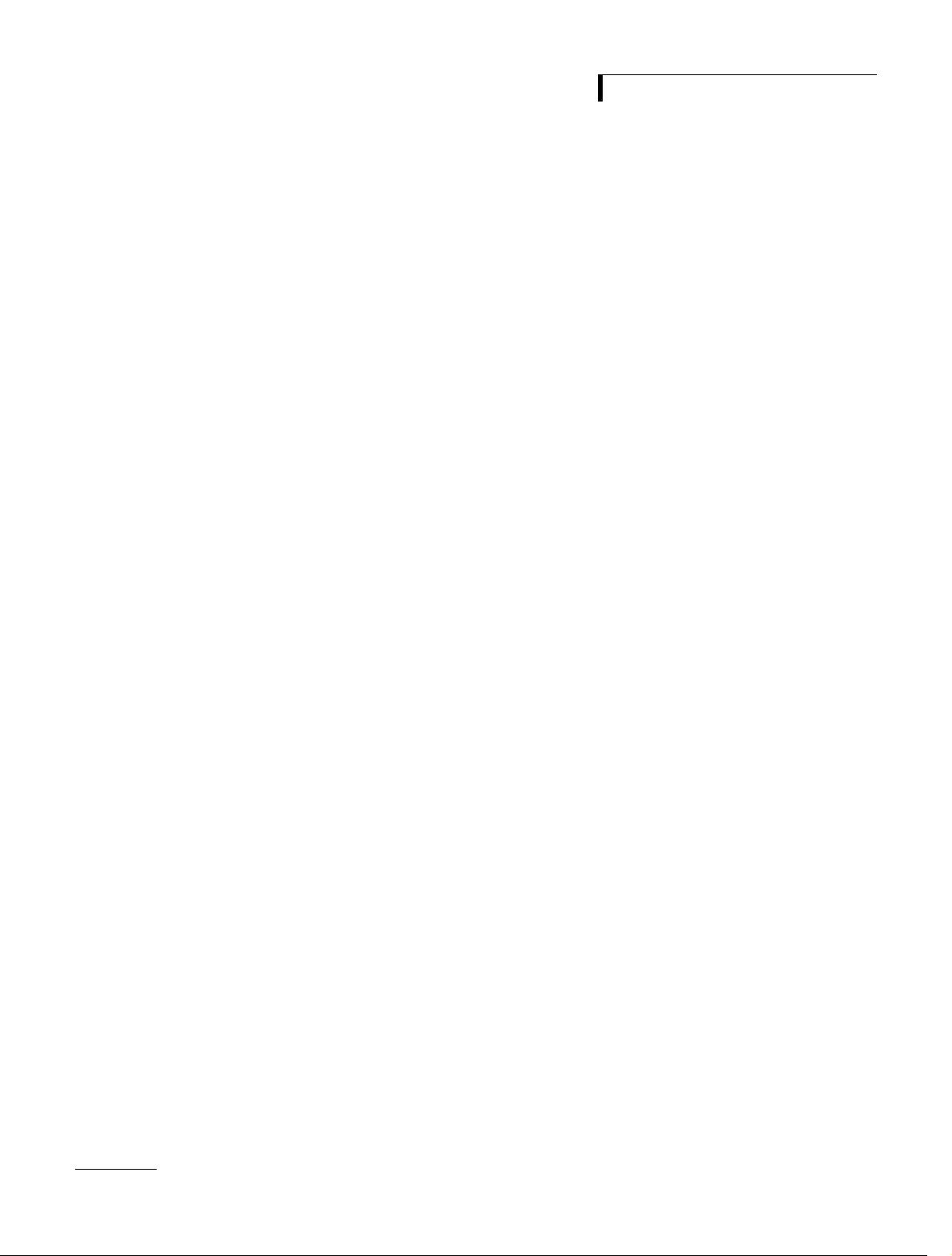
1
Artificial Intelligence for 6G Networks: Technology
Advancement and Standardization
Muhammad K. Shehzad, Luca Rose, M. Majid Butt, István Z. Kovács, Mohamad Assaad, and Mohsen Guizani
Abstract—With the deployment of 5G networks, standards
organizations have started working on the design phase for
sixth-generation (6G) networks. 6G networks will be immensely
complex, requiring more deployment time, cost and management
efforts. On the other hand, mobile network operators demand
these networks to be intelligent, self-organizing, and cost-effective
to reduce operating expenses (OPEX). Machine learning (ML),
a branch of artificial intelligence (AI), is the answer to many
of these challenges providing pragmatic solutions, which can
entirely change the future of wireless network technologies. By
using some case study examples, we briefly examine the most
compelling problems, particularly at the physical (PHY) and link
layers in cellular networks where ML can bring significant gains.
We also review standardization activities in relation to the use
of ML in wireless networks and future timeline on readiness
of standardization bodies to adapt to these changes. Finally, we
highlight major issues in ML use in the wireless technology,
and provide potential directions to mitigate some of them in 6G
wireless networks.
Index Terms—AI, ML, Wireless networks, 3GPP, 6G.
I. INTRODUCTION
Unprecedented growth in the global cellular traffic (as
shown in Fig. 1) and immense data rate demands have become
a challenge, leading wireless industry to the next-generation,
called 6G. 6G-era will bring digital, physical and biological
worlds together with the goal to improve human experience
and well-being. 6G will be operating in TeraHertz (THz)
frequencies (0.1-10 THz), hence beneficial for multiple use
cases in industrial applications, providing immense data rates
(≈ 1 Tb/s), accelerating internet-of-things, and wider network
coverage. AI/ML will pave the way for THz communications
at different layers [2], e.g., supporting channel acquisition [3]
and modulation classification [4] at PHY. Similarly, at the
link layer, beamforming design and channel allocation can
exploit ML [2]. In THz systems, a channel can significantly
vary at a micrometer scale, resulting in a tremendous increase
in channel estimation frequency and corresponding overhead.
ML algorithms can counter this issue by using, e.g., improved
channel prediction techniques [3], [5].
Muhammad K. Shehzad (corresponding author) is with Nokia
Bell-Labs, France, and Laboratoire des Signaux et Systèmes,
CentraleSupelec, CNRS, University of Paris-Saclay, France.
({muhammad.shehzad}@nokia.com/centralesupelec.fr).
Luca Rose, and M. Majid Butt are with Nokia Bell-Labs, France.
({luca.rose, majid.butt}@nokia-bell-labs.com).
István Z. Kovács is with Nokia Standards, Aalborg, Denmark. (ist-
van.kovacs@nokia.com).
Mohamad Assaad is with Laboratoire des Signaux et Systèmes,
CentraleSupelec, CNRS, University of Paris-Saclay, France.
(mohamad.assaad@centralesupelec.fr).
Mohsen Guizani is with Mohamed Bin Zayed University of Artificial
Intelligence (MBZUAI), Abu Dhabi, UAE. (mguizani@ieee.org).
2020 2021 2022 2023 2024 2025 2026 2027 2028 2029 2030
Year
0
10
20
30
40
50
60
70
80
90
100
Billion
M2M Users
MBB Users
Fig. 1. Estimation of global mobile subscriptions in machine-to-machine
(M2M) and mobile broadband (MBB) from 2020 to 2030. Source: ITU-R
Report M.2370-0 [1].
.
Recently, fast-growing deployment of 5G has opened up
many challenges, including massive complexity in network
architecture, low latency, high cost, power consumption, and
deployment of hybrid Long-Term Evolution (LTE) new radio
(NR), leading to difficulties in network optimization. In such
a complex scenario, the network intelligence has become
a major focus as it will play a pivotal role in complex
problem solving [6], e.g., self-healing, self-optimization, and
self-configuration of a network [7].
Future networks will become “cognitive” in a way that
many aspects such as spectrum sensing/sharing, slicing, radio
resource management (RRM), and mobility management, will
be ML-based. Further, it is expected that ML will impact
6G air interface fundamentally and it will be designed to
support ML natively [8]. Several recent research attempts,
e.g., [9], propose different road maps for 6G, but they do not
address standardization timeline and related issues regarding
application of ML in 6G. Albeit, to some extent, [10] gives an
overview of ML and standardization; nevertheless, ML-related
technical challenges and its applications from an industrial and
standardization perspective are not addressed.
Reconfigurable intelligent surface (RIS) and non-orthogonal
multiple access (NOMA) are two key technologies for 6G [11].
RIS can re-engineer electromagnetic waves, hence beneficial to
deliver the information where obstacles block the destination.
RIS can be integrated with ML, allowing RIS to acquire envi-
arXiv:2204.00914v1 [eess.SP] 2 Apr 2022