没有合适的资源?快使用搜索试试~ 我知道了~
温馨提示
The book is focused on a fast-paced approach to exploring practical deep learning concepts with math and programming-friendly abstractions. You will learn to design, develop, train, validate, and deploy deep neural networks using the industry's favorite Keras framework.
资源推荐
资源详情
资源评论
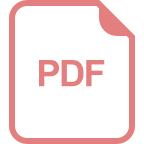
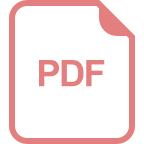
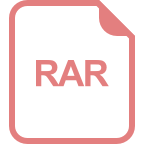
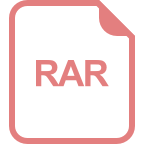
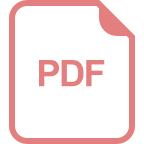
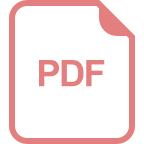
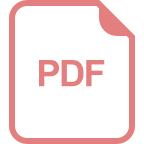
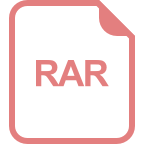
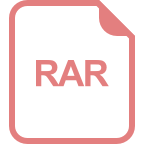
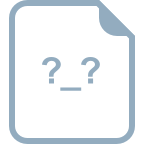
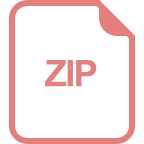
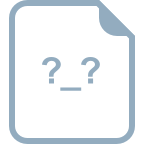
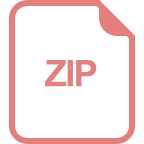
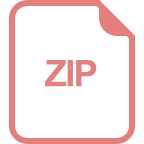
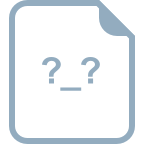
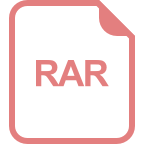
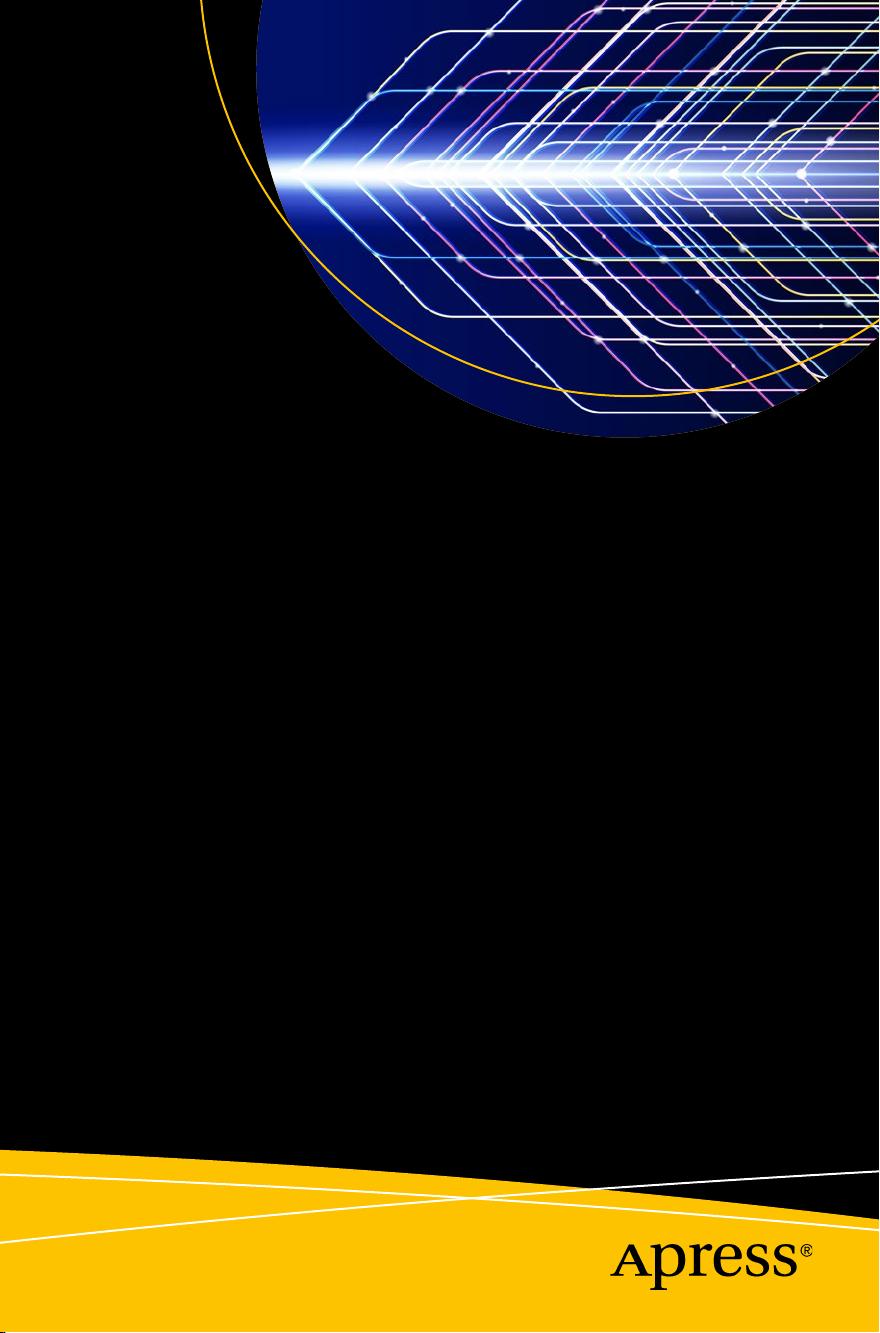
Learn Keras for
Deep Neural
Networks
A Fast-Track Approach to Modern
Deep Learning with Python
—
Jojo Moolayil
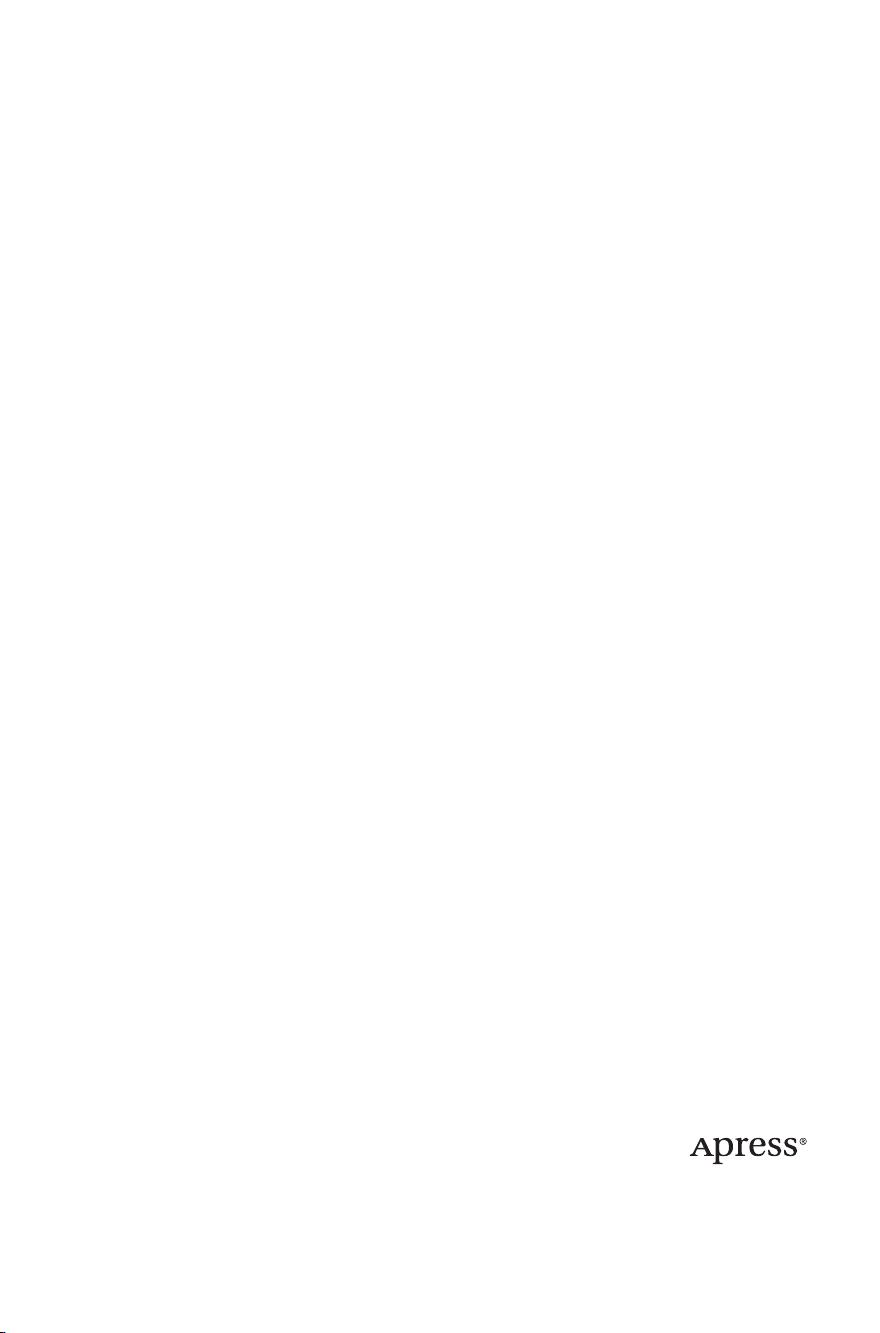
Learn Keras for Deep
Neural Networks
A Fast-Track Approach
to Modern Deep Learning
with Python
Jojo Moolayil
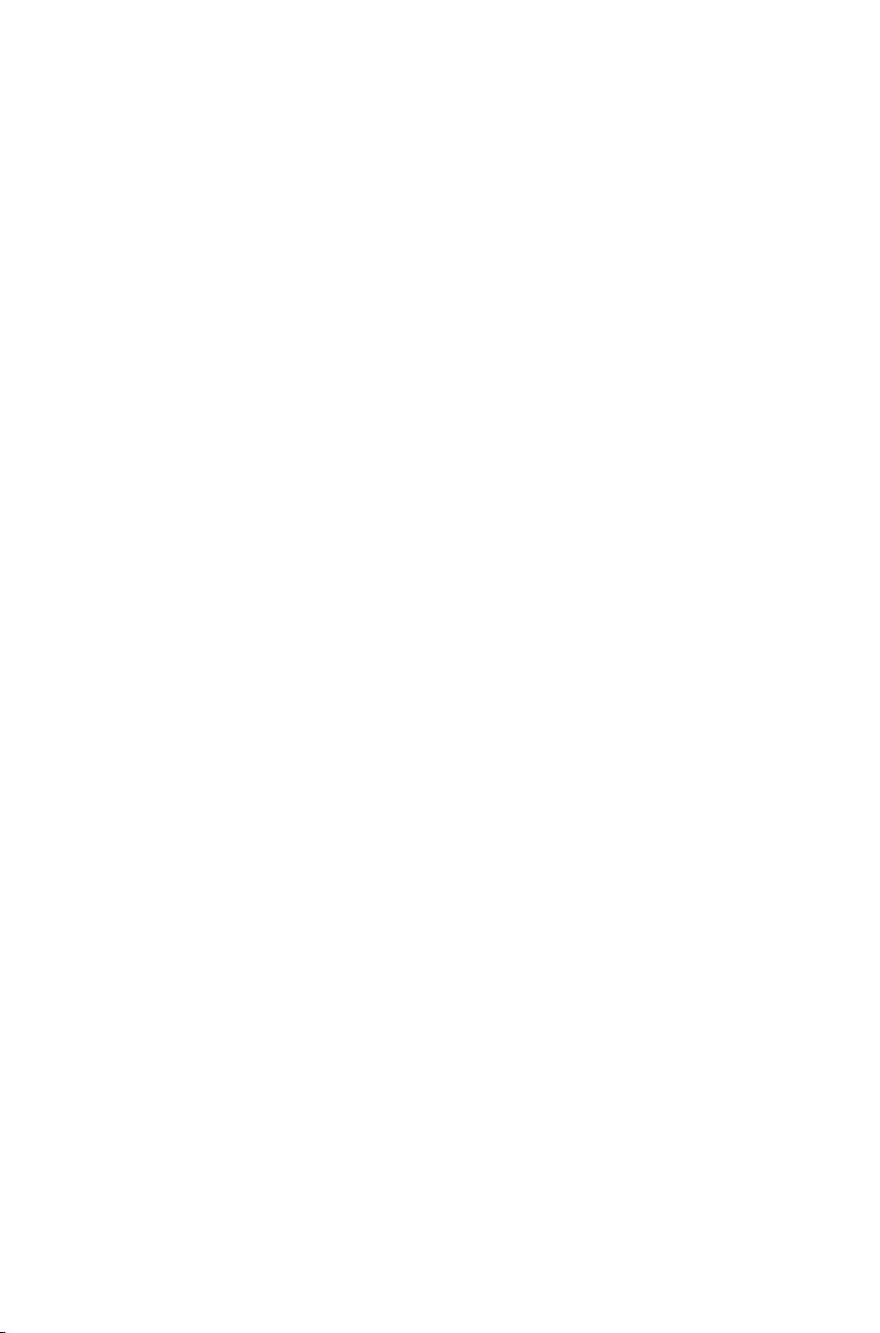
Learn Keras for Deep Neural Networks
ISBN-13 (pbk): 978-1-4842-4239-1 ISBN-13 (electronic): 978-1-4842-4240-7
https://doi.org/10.1007/978-1-4842-4240-7
Library of Congress Control Number: 2018965596
Copyright © 2019 by Jojo Moolayil
is work is subject to copyright. All rights are reserved by the Publisher, whether the whole or
part of the material is concerned, specically the rights of translation, reprinting, reuse of
illustrations, recitation, broadcasting, reproduction on microlms or in any other physical way,
and transmission or information storage and retrieval, electronic adaptation, computer software,
or by similar or dissimilar methodology now known or hereafter developed.
Trademarked names, logos, and images may appear in this book. Rather than use a trademark
symbol with every occurrence of a trademarked name, logo, or image we use the names, logos,
and images only in an editorial fashion and to the benet of the trademark owner, with no
intention of infringement of the trademark.
e use in this publication of trade names, trademarks, service marks, and similar terms, even if
they are not identied as such, is not to be taken as an expression of opinion as to whether or not
they are subject to proprietary rights.
While the advice and information in this book are believed to be true and accurate at the date of
publication, neither the authors nor the editors nor the publisher can accept any legal
responsibility for any errors or omissions that may be made. e publisher makes no warranty,
express or implied, with respect to the material contained herein.
Managing Director, Apress Media LLC: Welmoed Spahr
Acquisitions Editor: Celestin Suresh John
Development Editor: Matthew Moodie
Coordinating Editor: Aditee Mirashi
Cover designed by eStudioCalamar
Cover image designed by Freepik (www.freepik.com)
Distributed to the book trade worldwide by Springer Science+Business Media NewYork,
233 Spring Street, 6th Floor, NewYork, NY 10013. Phone 1-800-SPRINGER, fax (201) 348-4505,
e-mail orders-ny@springer-sbm.com, or visit www.springeronline.com. Apress Media, LLC is a
California LLC and the sole member (owner) is Springer Science + Business Media Finance Inc
(SSBM Finance Inc). SSBM Finance Inc is a Delaware corporation.
For information on translations, please e-mail rights@apress.com, or visit http://www.apress.
com/rights-permissions.
Apress titles may be purchased in bulk for academic, corporate, or promotional use. eBook
versions and licenses are also available for most titles. For more information, reference our Print
and eBook Bulk Sales web page at http://www.apress.com/bulk-sales.
Any source code or other supplementary material referenced by the author in this book is available
to readers on GitHub via the book’s product page, located at www.apress.com/978-1-4842-4239-1.
For more detailed information, please visit http://www.apress.com/source-code.
Printed on acid-free paper
JojoMoolayil
Vancouver, BC, Canada
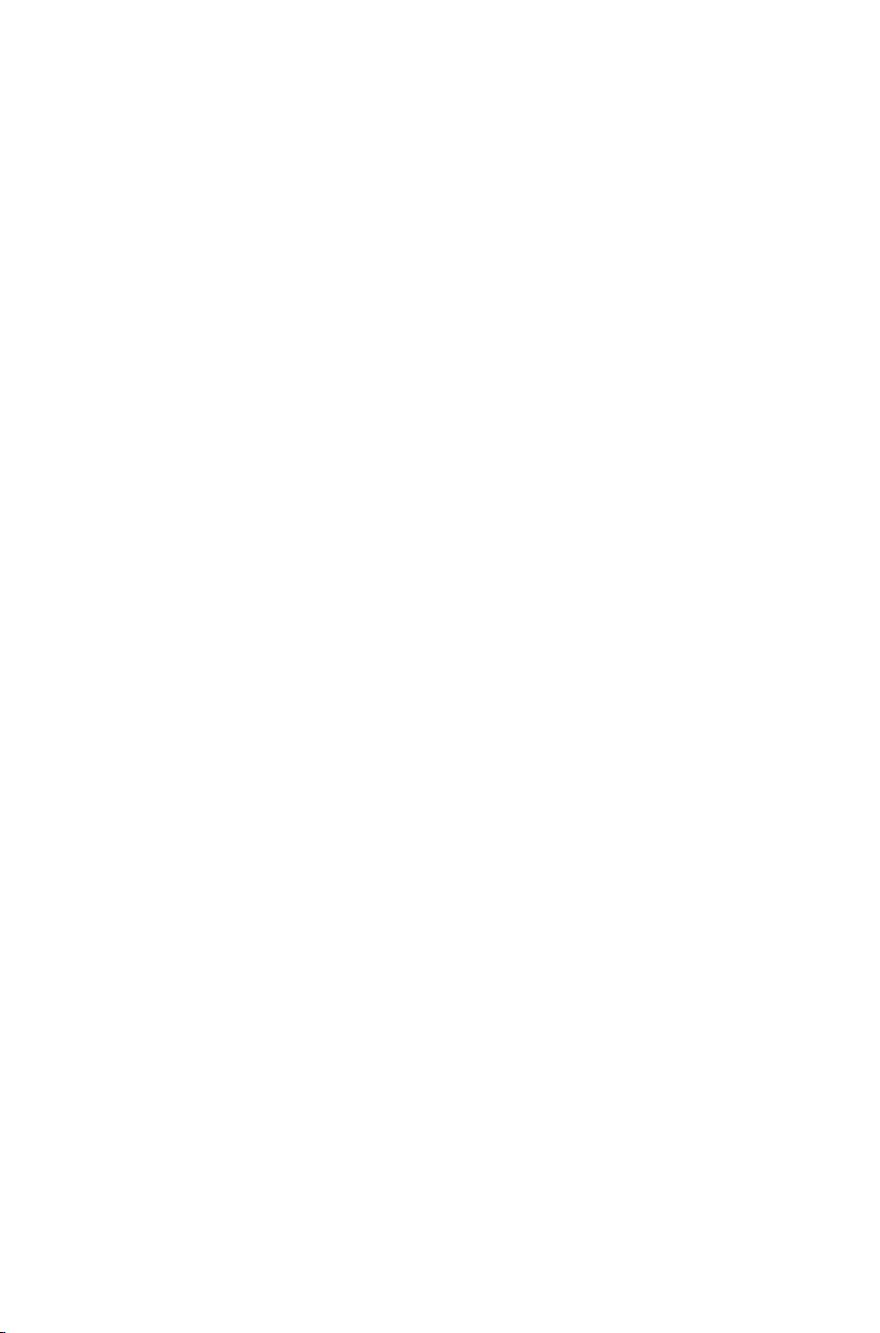
iii
About the Author ��������������������������������������������������������������������������������vii
About the Technical Reviewer �������������������������������������������������������������ix
Acknowledgments �������������������������������������������������������������������������������xi
Introduction ���������������������������������������������������������������������������������������xiii
Table of Contents
Chapter 1: An Introduction to Deep Learning and Keras ����������������������1
Introduction to DL �������������������������������������������������������������������������������������������������1
Demystifying the Buzzwords ���������������������������������������������������������������������������2
What Are Some Classic Problems Solved by DL in Today’s Market? ���������������5
Decomposing a DL Model �������������������������������������������������������������������������������� 5
Exploring the Popular DL Frameworks ������������������������������������������������������������������8
Low-Level DL Frameworks ������������������������������������������������������������������������������ 9
High-Level DL Frameworks ���������������������������������������������������������������������������11
A Sneak Peek into the Keras Framework ������������������������������������������������������������13
Getting the Data Ready ����������������������������������������������������������������������������������15
Dening the Model Structure ������������������������������������������������������������������������15
Training the Model and Making Predictions ��������������������������������������������������15
Summary�������������������������������������������������������������������������������������������������������������16
Chapter 2: Keras in Action ������������������������������������������������������������������17
Setting Up the Environment ��������������������������������������������������������������������������������17
Selecting the Python Version ������������������������������������������������������������������������� 17
Installing Python for Windows, Linux, or macOS �������������������������������������������18
Installing Keras and TensorFlow Back End ���������������������������������������������������� 19
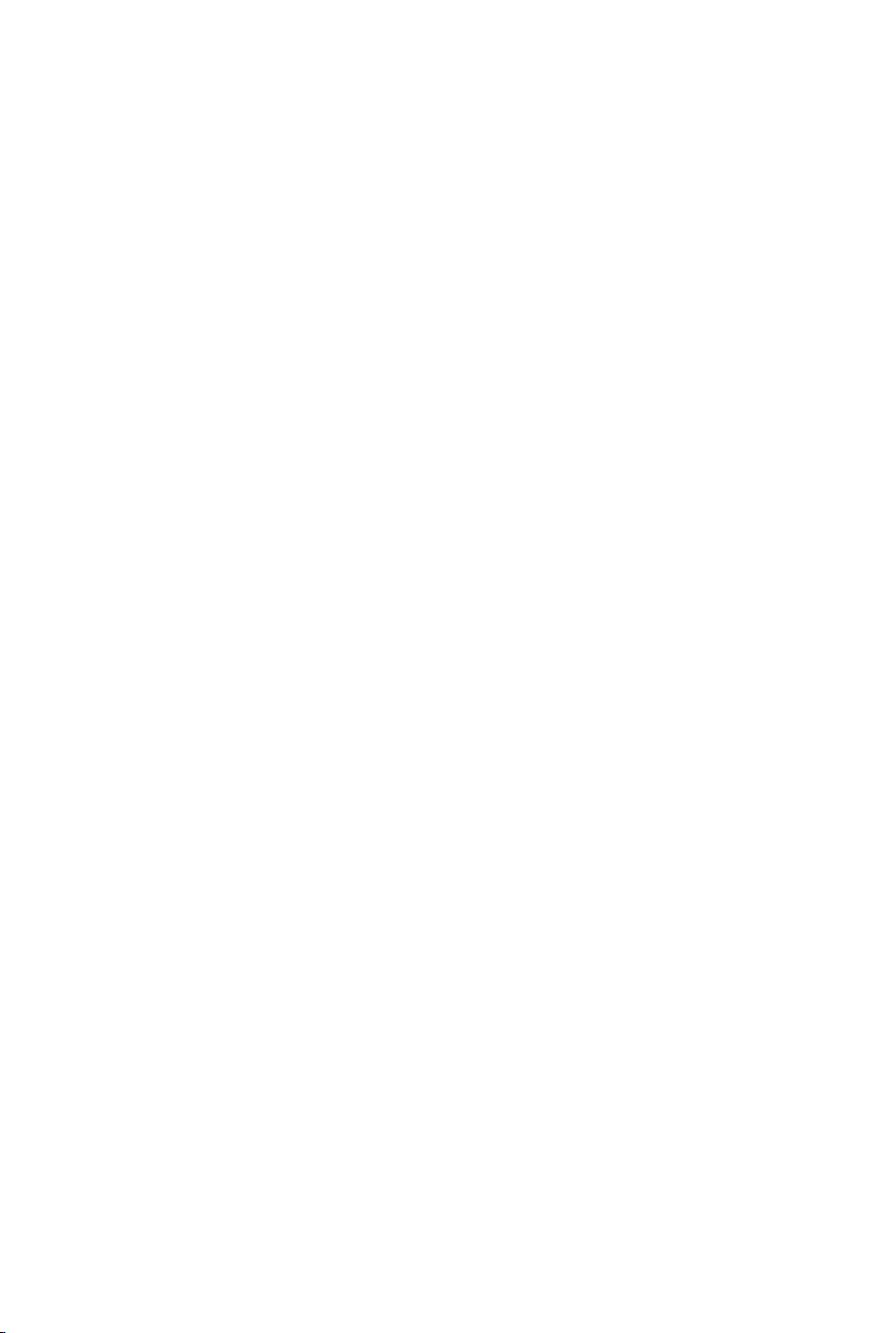
iv
Getting Started with DL in Keras �������������������������������������������������������������������������21
Input Data ������������������������������������������������������������������������������������������������������21
Neuron ����������������������������������������������������������������������������������������������������������� 23
Activation Function ���������������������������������������������������������������������������������������� 24
Sigmoid Activation Function ��������������������������������������������������������������������������25
Model �������������������������������������������������������������������������������������������������������������28
Layers ������������������������������������������������������������������������������������������������������������ 28
The Loss Function �����������������������������������������������������������������������������������������32
Optimizers �����������������������������������������������������������������������������������������������������35
Metrics ����������������������������������������������������������������������������������������������������������39
Model Conguration ��������������������������������������������������������������������������������������39
Model Training �����������������������������������������������������������������������������������������������40
Model Evaluation �������������������������������������������������������������������������������������������43
Putting All the Building Blocks Together �������������������������������������������������������������45
Summary�������������������������������������������������������������������������������������������������������������52
Chapter 3: Deep Neural Networks for Supervised Learning:
Regression ������������������������������������������������������������������������������������������53
Getting Started ���������������������������������������������������������������������������������������������������� 53
Problem Statement ���������������������������������������������������������������������������������������������55
Why Is Representing a Problem Statement with a Design Principle
Important? �����������������������������������������������������������������������������������������������������56
Designing an SCQ ������������������������������������������������������������������������������������������57
Designing the Solution ����������������������������������������������������������������������������������59
Exploring the Data �����������������������������������������������������������������������������������������������60
Looking at the Data Dictionary ����������������������������������������������������������������������63
Finding Data Types ����������������������������������������������������������������������������������������66
Working with Time �����������������������������������������������������������������������������������������67
Predicting Sales ���������������������������������������������������������������������������������������������69
Table of ConTenTsTable of ConTenTs
剩余191页未读,继续阅读
资源评论
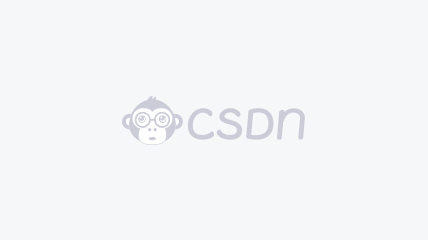

localsg
- 粉丝: 5
- 资源: 77
上传资源 快速赚钱
我的内容管理 展开
我的资源 快来上传第一个资源
我的收益
登录查看自己的收益我的积分 登录查看自己的积分
我的C币 登录后查看C币余额
我的收藏
我的下载
下载帮助

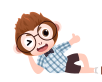
最新资源
- 基于redis全站抓取资料齐全+文档+源码.zip
- 基于pybullet和stable baseline3 的法奥机械臂的强化学习抓取训练代码资料齐全+文档+源码.zip
- 基于Redis实现的一套分布式定向抓取工程。资料齐全+文档+源码.zip
- 基于RSS订阅自动抓取文章生成站点,这是个实验性功能。资料齐全+文档+源码.zip
- 基于scrapy+selenium+phantomjs的爬虫程序,用于抓取多个学校的学术报告信息资料齐全+文档+源码.zip
- 基于scrapy的danbooru图片抓取工具资料齐全+文档+源码.zip
- 基于scrapy的上市公司信息抓取工具资料齐全+文档+源码.zip
- 基于Scrapy框架,用于抓取新浪微博数据,主要包括微博内容,评论以及用户信息资料齐全+文档+源码.zip
- 基于scrapy的时尚网站商品数据抓取资料齐全+文档+源码.zip
- 基于scrapy框架使用redis实现对shopee商城的增量抓取资料齐全+文档+源码.zip
- 基于Scrapy爬虫对某守望先锋网站数据的动态抓取资料齐全+文档+源码.zip
- 基于scrapy实现几大主流司法拍卖网站抓取资料齐全+文档+源码.zip
- 基于Selelium图片抓取资料齐全+文档+源码.zip
- 基于swoole扩展的爬虫,php多进程多线程抓取资料齐全+文档+源码.zip
- 基于Thinkphp5实现数据信息抓取、基于整理的API接口 + 招聘信息抓取(前程无忧智联招聘boss直聘拉勾网)数据接口 + 新闻分类(头条军事娱乐体
- FSCapture Ver. 8.9:屏幕截图与录制工具,图像编辑与快捷键支持,支持全屏、窗口、区域截图,滚动截图与视频录制,自动上传与FTP上传,适用于教学、设计、技术支持与文档制作
资源上传下载、课程学习等过程中有任何疑问或建议,欢迎提出宝贵意见哦~我们会及时处理!
点击此处反馈


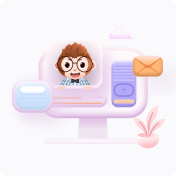
安全验证
文档复制为VIP权益,开通VIP直接复制
