# Stable Diffusion Version 2



This repository contains [Stable Diffusion](https://github.com/CompVis/stable-diffusion) models trained from scratch and will be continuously updated with
new checkpoints. The following list provides an overview of all currently available models. More coming soon.
## News
**December 7, 2022**
*Version 2.1*
- New stable diffusion model (_Stable Diffusion 2.1-v_, [HuggingFace](https://huggingface.co/stabilityai/stable-diffusion-2-1)) at 768x768 resolution and (_Stable Diffusion 2.1-base_, [HuggingFace](https://huggingface.co/stabilityai/stable-diffusion-2-1-base)) at 512x512 resolution, both based on the same number of parameters and architecture as 2.0 and fine-tuned on 2.0, on a less restrictive NSFW filtering of the [LAION-5B](https://laion.ai/blog/laion-5b/) dataset.
Per default, the attention operation of the model is evaluated at full precision when `xformers` is not installed. To enable fp16 (which can cause numerical instabilities with the vanilla attention module on the v2.1 model) , run your script with `ATTN_PRECISION=fp16 python <thescript.py>`
**November 24, 2022**
*Version 2.0*
- New stable diffusion model (_Stable Diffusion 2.0-v_) at 768x768 resolution. Same number of parameters in the U-Net as 1.5, but uses [OpenCLIP-ViT/H](https://github.com/mlfoundations/open_clip) as the text encoder and is trained from scratch. _SD 2.0-v_ is a so-called [v-prediction](https://arxiv.org/abs/2202.00512) model.
- The above model is finetuned from _SD 2.0-base_, which was trained as a standard noise-prediction model on 512x512 images and is also made available.
- Added a [x4 upscaling latent text-guided diffusion model](#image-upscaling-with-stable-diffusion).
- New [depth-guided stable diffusion model](#depth-conditional-stable-diffusion), finetuned from _SD 2.0-base_. The model is conditioned on monocular depth estimates inferred via [MiDaS](https://github.com/isl-org/MiDaS) and can be used for structure-preserving img2img and shape-conditional synthesis.

- A [text-guided inpainting model](#image-inpainting-with-stable-diffusion), finetuned from SD _2.0-base_.
We follow the [original repository](https://github.com/CompVis/stable-diffusion) and provide basic inference scripts to sample from the models.
________________
*The original Stable Diffusion model was created in a collaboration with [CompVis](https://arxiv.org/abs/2202.00512) and [RunwayML](https://runwayml.com/) and builds upon the work:*
[**High-Resolution Image Synthesis with Latent Diffusion Models**](https://ommer-lab.com/research/latent-diffusion-models/)<br/>
[Robin Rombach](https://github.com/rromb)\*,
[Andreas Blattmann](https://github.com/ablattmann)\*,
[Dominik Lorenz](https://github.com/qp-qp)\,
[Patrick Esser](https://github.com/pesser),
[Björn Ommer](https://hci.iwr.uni-heidelberg.de/Staff/bommer)<br/>
_[CVPR '22 Oral](https://openaccess.thecvf.com/content/CVPR2022/html/Rombach_High-Resolution_Image_Synthesis_With_Latent_Diffusion_Models_CVPR_2022_paper.html) |
[GitHub](https://github.com/CompVis/latent-diffusion) | [arXiv](https://arxiv.org/abs/2112.10752) | [Project page](https://ommer-lab.com/research/latent-diffusion-models/)_
and [many others](#shout-outs).
Stable Diffusion is a latent text-to-image diffusion model.
________________________________
## Requirements
You can update an existing [latent diffusion](https://github.com/CompVis/latent-diffusion) environment by running
```
conda install pytorch==1.12.1 torchvision==0.13.1 -c pytorch
pip install transformers==4.19.2 diffusers invisible-watermark
pip install -e .
```
#### xformers efficient attention
For more efficiency and speed on GPUs,
we highly recommended installing the [xformers](https://github.com/facebookresearch/xformers)
library.
Tested on A100 with CUDA 11.4.
Installation needs a somewhat recent version of nvcc and gcc/g++, obtain those, e.g., via
```commandline
export CUDA_HOME=/usr/local/cuda-11.4
conda install -c nvidia/label/cuda-11.4.0 cuda-nvcc
conda install -c conda-forge gcc
conda install -c conda-forge gxx_linux-64==9.5.0
```
Then, run the following (compiling takes up to 30 min).
```commandline
cd ..
git clone https://github.com/facebookresearch/xformers.git
cd xformers
git submodule update --init --recursive
pip install -r requirements.txt
pip install -e .
cd ../stablediffusion
```
Upon successful installation, the code will automatically default to [memory efficient attention](https://github.com/facebookresearch/xformers)
for the self- and cross-attention layers in the U-Net and autoencoder.
## General Disclaimer
Stable Diffusion models are general text-to-image diffusion models and therefore mirror biases and (mis-)conceptions that are present
in their training data. Although efforts were made to reduce the inclusion of explicit pornographic material, **we do not recommend using the provided weights for services or products without additional safety mechanisms and considerations.
The weights are research artifacts and should be treated as such.**
Details on the training procedure and data, as well as the intended use of the model can be found in the corresponding [model card](https://huggingface.co/stabilityai/stable-diffusion-2).
The weights are available via [the StabilityAI organization at Hugging Face](https://huggingface.co/StabilityAI) under the [CreativeML Open RAIL++-M License](LICENSE-MODEL).
## Stable Diffusion v2
Stable Diffusion v2 refers to a specific configuration of the model
architecture that uses a downsampling-factor 8 autoencoder with an 865M UNet
and OpenCLIP ViT-H/14 text encoder for the diffusion model. The _SD 2-v_ model produces 768x768 px outputs.
Evaluations with different classifier-free guidance scales (1.5, 2.0, 3.0, 4.0,
5.0, 6.0, 7.0, 8.0) and 50 DDIM sampling steps show the relative improvements of the checkpoints:

### Text-to-Image


Stable Diffusion 2 is a latent diffusion model conditioned on the penultimate text embeddings of a CLIP ViT-H/14 text encoder.
We provide a [reference script for sampling](#reference-sampling-script).
#### Reference Sampling Script
This script incorporates an [invisible watermarking](https://github.com/ShieldMnt/invisible-watermark) of the outputs, to help viewers [identify the images as machine-generated](scripts/tests/test_watermark.py).
We provide the configs for the _SD2-v_ (768px) and _SD2-base_ (512px) model.
First, download the weights for [_SD2.1-v_](https://huggingface.co/stabilityai/stable-diffusion-2-1) and [_SD2.1-base_](https://huggingface.co/stabilityai/stable-diffusion-2-1-base).
To sample from the _SD2.1-v_ model, run the following:
```
python scripts/txt2img.py --prompt "a professional photograph of an astronaut riding a horse" --ckpt <path/to/768model.ckpt/> --config configs/stable-diffusion/v2-inference-v.yaml --H 768 --W 768
```
or try out the Web Demo: [](https://huggingface.co/spaces/stabilityai/stable-diffusion).
To sample from the base model, use
```
python scripts/txt2img.py --prompt "a professional photograph of an astronaut riding a horse" --ckpt <path/to/model.ckpt/> --config <path/to/config.yaml/>
```
By default, this uses the [DDIM sampler](https://arxiv.org/abs/2010.02502), and renders images of size 768x768 (which it was trained on) in 50 steps.
Empirically, the v-models can be sampled with higher guidance scales.
Note: The inference config for all model versions is designed to be used with EMA-only checkpoints.
For this r
没有合适的资源?快使用搜索试试~ 我知道了~
《AI大模型》--stable-diffusion是一个支持文本生成图片的AI语言模型,功能异常强大,一起探索中.zip
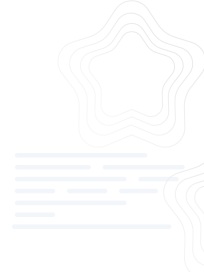
共74个文件
py:56个
yaml:10个
md:2个

1.该资源内容由用户上传,如若侵权请联系客服进行举报
2.虚拟产品一经售出概不退款(资源遇到问题,请及时私信上传者)
2.虚拟产品一经售出概不退款(资源遇到问题,请及时私信上传者)
版权申诉
0 下载量 182 浏览量
2024-03-22
16:24:38
上传
评论
收藏 585KB ZIP 举报
温馨提示
人工智能学习总结成果,希望可以帮到大家,有疑问欢迎随时沟通~ 人工智能学习总结成果,希望可以帮到大家,有疑问欢迎随时沟通~ 人工智能学习总结成果,希望可以帮到大家,有疑问欢迎随时沟通~ 人工智能学习总结成果,希望可以帮到大家,有疑问欢迎随时沟通~ 人工智能学习总结成果,希望可以帮到大家,有疑问欢迎随时沟通~
资源推荐
资源详情
资源评论
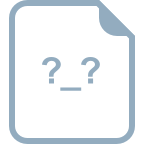
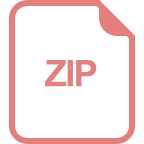
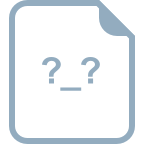
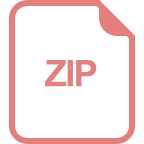
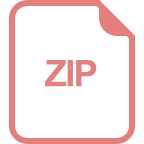
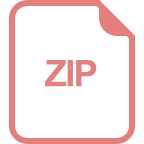
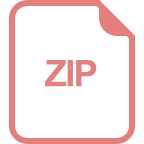
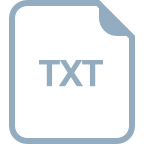
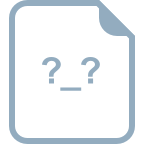
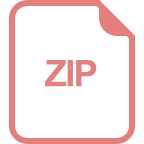
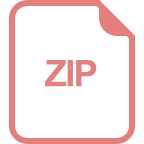
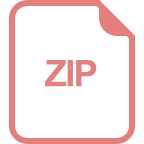
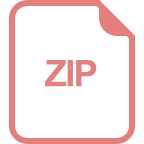
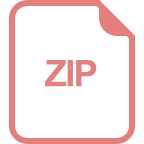
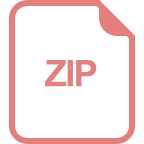
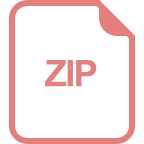
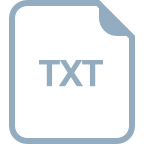
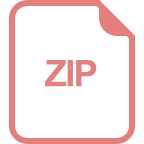
收起资源包目录


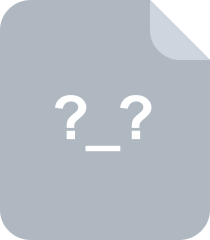

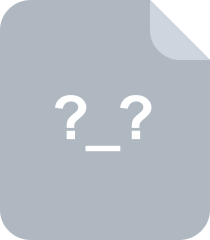
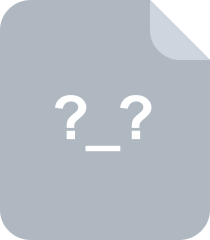


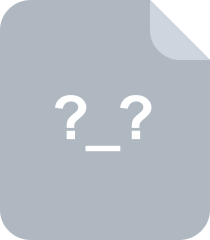
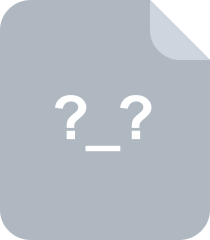
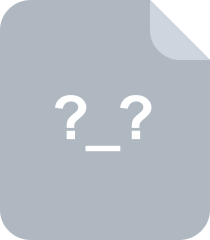

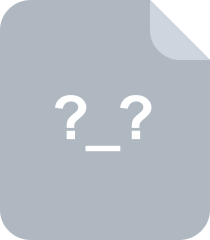
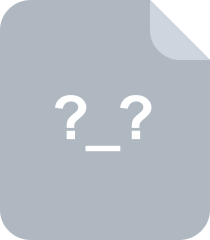

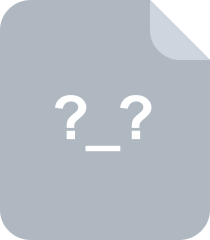

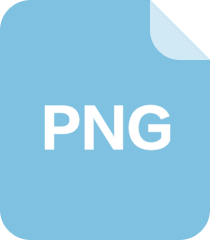
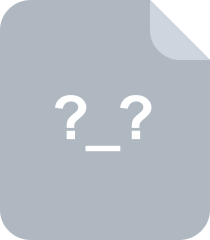
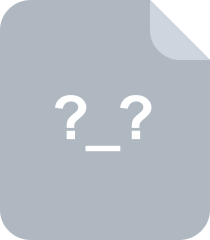
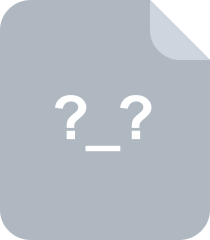

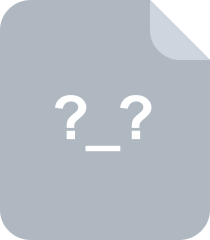
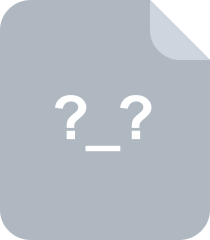
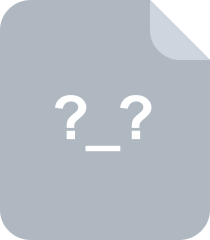

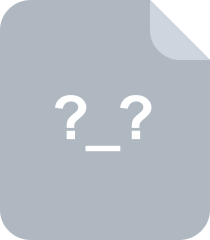
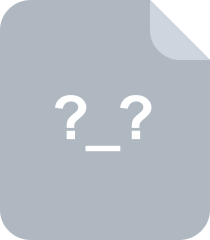
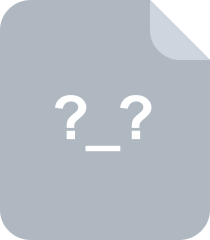
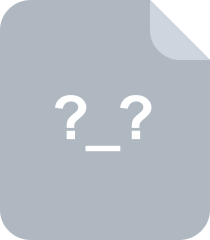
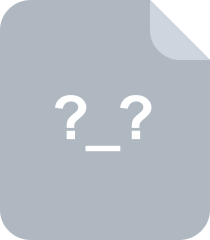
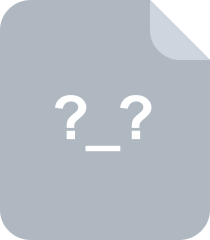
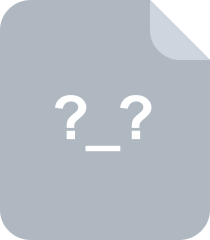
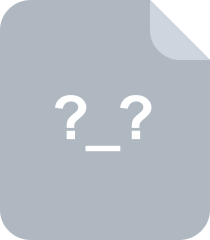

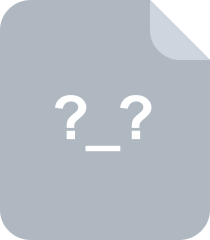
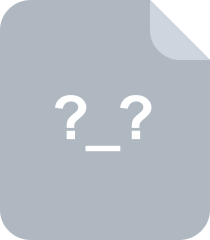
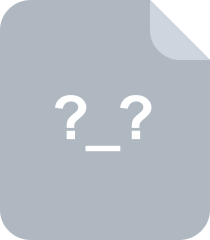
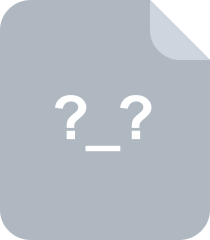
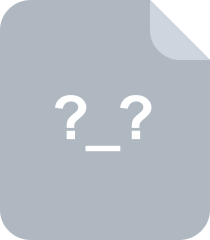
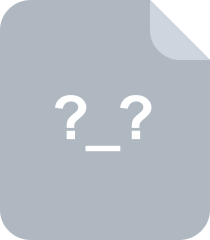

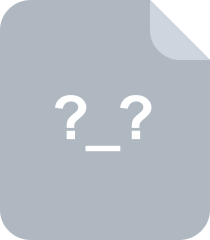
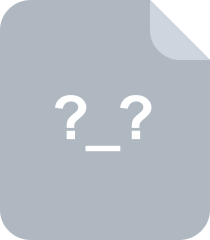
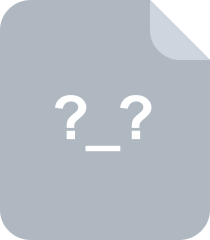
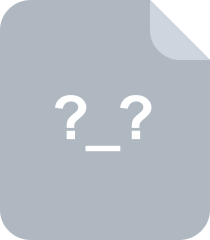
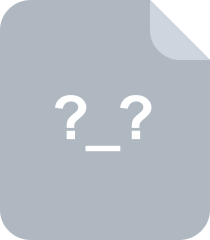

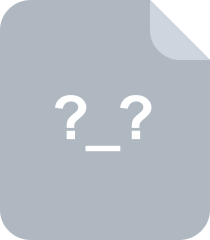

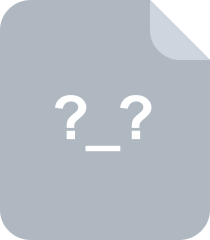
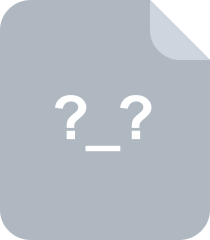
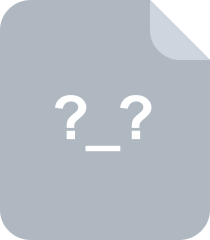
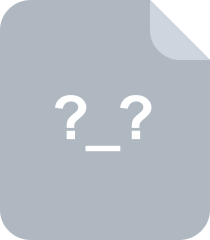

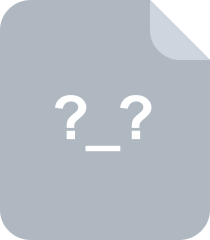
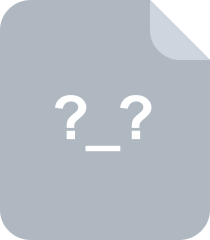
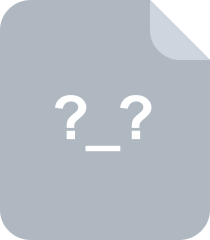
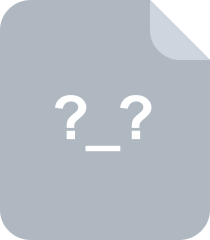
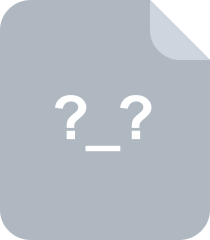
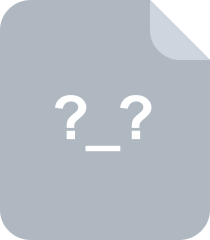
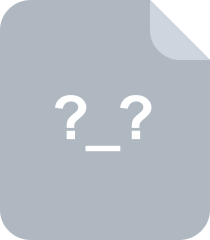
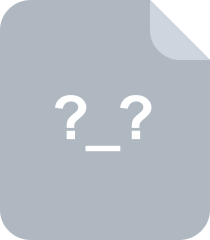

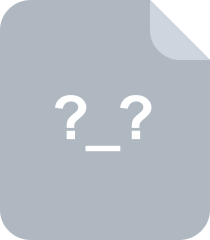

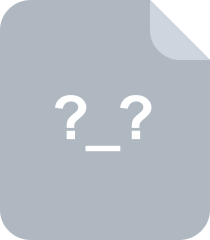
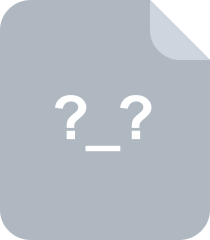
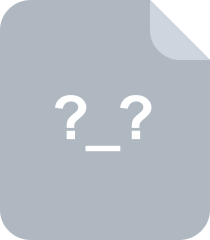

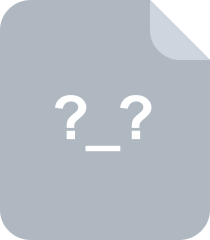
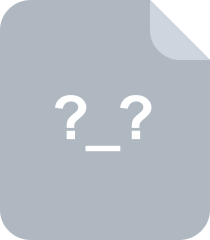
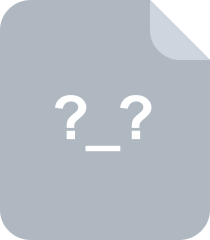
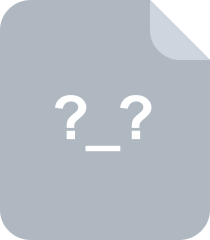
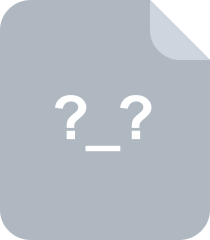
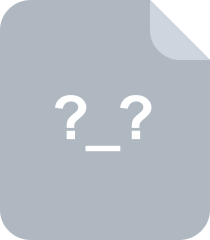
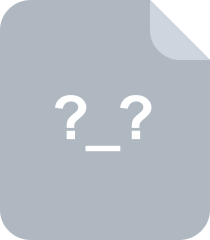
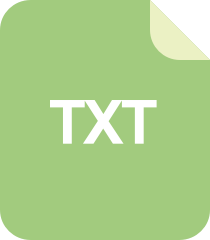
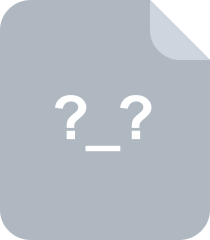
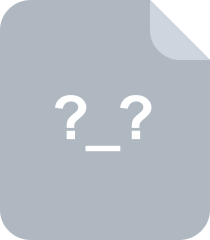
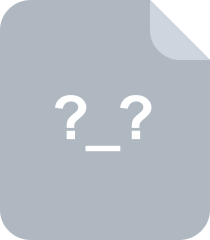

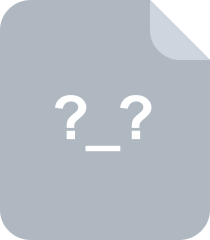
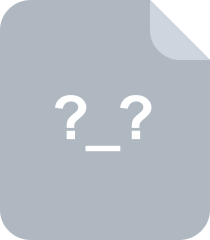

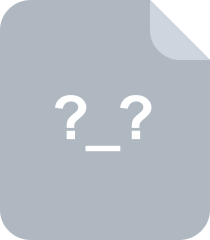
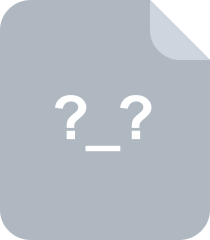

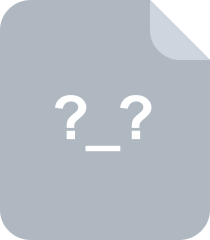
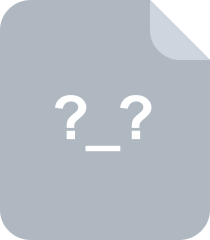
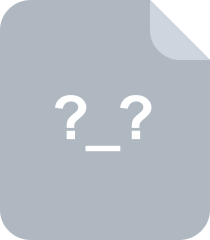
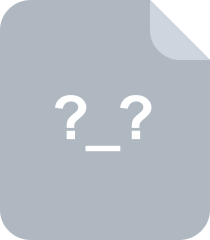

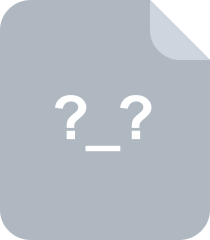
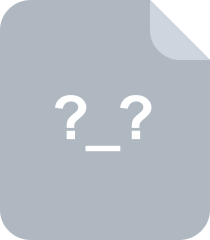
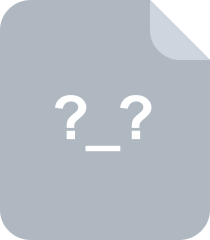
共 74 条
- 1
资源评论
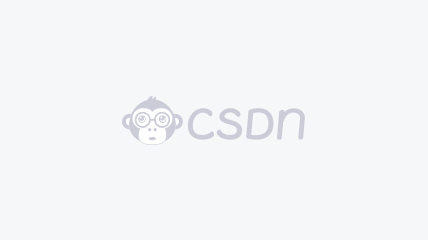

季风泯灭的季节
- 粉丝: 2067
- 资源: 3370
上传资源 快速赚钱
我的内容管理 展开
我的资源 快来上传第一个资源
我的收益
登录查看自己的收益我的积分 登录查看自己的积分
我的C币 登录后查看C币余额
我的收藏
我的下载
下载帮助

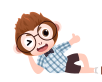
最新资源
- 大话5G.epub
- 电商数据分析与数据化运营-767f0da0bf87.epub
- 大数据、数据挖掘与智慧运营.epub
- 数据分析实战.epub
- Word Excel PPT 2016 高效办公实战.epub
- SDUCS汇编语言实验代码-MASM
- 永磁同步电机的MTPA最大转矩电流比控制算法的仿真模型 有详细的算法设计文档 提供永磁同步电机的矢量控制原理说明,采用最大转矩电流比控制和弱磁控制,调制采用SVPWM;
- 使用 Python 和 moviepy 库实现视频转音频及音频相似度计算
- 消防服全球市场研究报告:2024年全球消防服市场销售额为18.3亿美元
- 【Web网页设计制作-毕业设计期末大作业源码】木纹背景宽屏家居行业html5模板5605.zip
- 4-Attention 升级面.pdf
- 7-相似度函数篇.pdf
- 10-LLMs 训练经验帖.pdf
- 11-大模型(LLMs)langchain 面.pdf
- 13-基于langchain RAG问答应用实战.pdf
- 15-大模型 RAG 经验面.pdf
资源上传下载、课程学习等过程中有任何疑问或建议,欢迎提出宝贵意见哦~我们会及时处理!
点击此处反馈


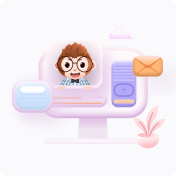
安全验证
文档复制为VIP权益,开通VIP直接复制
