# -*- coding: utf-8 -*-
"""
Created on Sun Mar 6 14:23:54 2022
Author: Fu Yangyang
@Our Final variables:
pool=['gender',
'housing',
'income',
'std_age',
'past_bad_credit',
'married',
'0',
'1',
'edu0',
'e1',
'e2',
'e3']
"""
import os,csv
import pandas as pd
import numpy as np
from sklearn.linear_model import LogisticRegression
import statsmodels.api as sm
from sklearn.metrics import roc_curve, auc
import matplotlib.pyplot as plt
#from tqdm import tqdm
from sklearn import preprocessing
from imblearn.over_sampling import SMOTE
path = r'C:\Users\hp\Desktop\MFIN7034 Problem set2'
#df=pd.read_csv(path+os.sep+'credit_risk.csv')
with open(path+os.sep+'credit_risk.csv', mode='r') as csv_file:
csv_reader = csv.reader(csv_file, delimiter=',')
line = 0
data_temp = []
for row in csv_reader:
if line == 0:
print("variable names",",".join(row))
var_names = row
line=line+1
else:
data_temp.append(list(map(float,row)))
line=line+1
csv_file.close()
data_temp_m = np.asmatrix(data_temp)
df = pd.DataFrame(np.array(data_temp), columns=var_names)
def draw_curve(fpr,tpr,roc_auc,save_name):
###make a plot of roc curve
plt.figure(dpi=150)
lw = 2
plt.plot(fpr, tpr, color='darkorange',
lw=lw, label='ROC curve (area = %0.2f)' % roc_auc)
plt.plot([0, 1], [0, 1], color='navy', lw=lw, linestyle='--')
plt.xlim([0.0, 1.0])
plt.ylim([0.0, 1.05])
plt.xlabel('False Positive Rate')
plt.ylabel('True Positive Rate')
plt.title(save_name)
plt.legend(loc="lower right")
plt.savefig(path+os.sep+save_name+'.jpg')
plt.show()
print('Figure was saved to ' + path)
#%%
#Question1 //Simple Logistic model
LR = LogisticRegression()
###simple example: predictors include income and past_bad_credit
X=df[['income','past_bad_credit']]
y=df['default_label']
###run logistic regression
lr_model = LR.fit(X,y)
###another way to run logistic regression
lr_model1 = sm.Logit(y,sm.add_constant(X)).fit()
###get a summary result of lr
print(lr_model1.summary())
###this is a two dimensional vector, prob d=0 and prob d=1, use the second one
predicted_prob = lr_model.predict_proba(X)
predicted_default_prob= predicted_prob[:,1]
###compute false positive rate and true positive rate using roc_curve function
fpr, tpr, _ = roc_curve(y, predicted_default_prob)
roc_auc = auc(fpr, tpr)
draw_curve(fpr,tpr,roc_auc,'2.1 Receiver operating characteristic example')
#%%
#Question2 // 2.2 Full Logistic Model
LR = LogisticRegression(penalty="l1",solver= 'liblinear',class_weight='balanced',tol=0.008,max_iter=100000)
#convert the gender, age and income
df['gender']=preprocessing.scale(df['gender'])
df['std_age']=preprocessing.scale(df['Age'])
df['std_income']=preprocessing.scale(df['income'])
#change the job_occupation to dummy
df['jo_0'] = pd.get_dummies(df['job_occupation'])[0]
df['jo_1'] = pd.get_dummies(df['job_occupation'])[1]
##change the edu to dummy
df['edu_0'] = pd.get_dummies(df['edu'])[0]
df['edu_1'] = pd.get_dummies(df['edu'])[1]
df['edu_2'] = pd.get_dummies(df['edu'])[2]
df['edu_3'] = pd.get_dummies(df['edu'])[3]
'''
#variables that we have tried
#df['dummy_edu']=list(map(lambda x: np.log(x),df['edu']))
#df['gender']=list(map(lambda x: np.log(x),df['gender']))
#df['ln_income']=list(map(lambda x: np.log(x),df['income']))
#df['std_ln_age']=preprocessing.scale(list(map(lambda x: np.log(x),df['Age'])))
#df['std_ln_income']=preprocessing.scale(list(map(lambda x: np.log(x),df['income'])))
#df['Age'] = df['Age']//10
#df['std_edu']=preprocessing.scale(df['edu'])
#Use Exhaustive method to try every combination,but at last, we find the best combination is the pool list now
LR = LogisticRegression(penalty="l1",solver= 'liblinear',class_weight='balanced',tol=0.008,max_iter=100000)
df2=pd.DataFrame()
cbna_list=[] #save variables
auc_list=[] #save auc
variables=[] #save number of variables
for i in tqdm(range(7,len(pool)+1)): #Use up to len(pool) variables
for cbna in itertools.combinations(pool, i):
X=df[list(cbna)]
y=df['default_label']
x_smote, y_smote = smote.fit_resample(X, y)
lr_model = LR.fit(x_smote,y_smote)
predicted_prob = lr_model.predict_proba(x_smote)
predicted_default_prob= predicted_prob[:,1]
fpr, tpr, _ = roc_curve(y_smote, predicted_default_prob)
roc_auc = auc(fpr, tpr)
#save results
cbna_list.append(list(cbna))
variables.append(len(list(cbna)))
auc_list.append(roc_auc)
df2['Varibles']=cbna_list
df2['No. of variables used'] = variables
df2['auc value'] = auc_list
'''
#%%
#Continue Question 2
#Choose the combinantion that achieve highest auc
pool=['gender',
'housing',
'income',
'std_age',
'past_bad_credit',
'married',
'jo_0',
'jo_1',
'edu_0',
'edu_1',
'edu_2',
'edu_3']
smote = SMOTE()#use smote function to balance our data sample
X = df[pool]
y=df['default_label']
x_smote, y_smote = smote.fit_resample(X, y)
lr_model = LR.fit(x_smote,y_smote)
lr_model1 = sm.Logit(y_smote,sm.add_constant(x_smote)).fit()
predicted_prob = lr_model.predict_proba(x_smote)
predicted_default_prob= predicted_prob[:,1]
fpr, tpr, _ = roc_curve(y_smote, predicted_default_prob)
roc_auc = auc(fpr, tpr)
print(lr_model1.summary())
print('the best combination: ', list(X.columns))
print('used variables: ' , len(X.columns))
print('the auc value: ' , roc_auc)
draw_curve(fpr,tpr,roc_auc,'2.2 Full Logistic Model')
#%%
#Question3 // 2.3 SVM
from sklearn.svm import SVC
regressor = SVC(C=1.0, cache_size=200, class_weight=None, coef0=0.0,
decision_function_shape='ovr', degree=3, gamma='auto', kernel='rbf',
max_iter=-1, probability=True, random_state=None, shrinking=True,
tol=0.001, verbose=False)
X = x_smote
y = y_smote
regressor.fit(X, y)
predicted_prob = regressor.predict_proba(X)
predicted_default_prob= predicted_prob[:,1]
fpr, tpr, _ = roc_curve(y, predicted_default_prob)
roc_auc = auc(fpr, tpr)
draw_curve(fpr,tpr,roc_auc,'2.3 SVM')
#%%
#Question4 // 2.4 Out-of-Sample Test
from sklearn.model_selection import train_test_split
X_train, X_test, y_train, y_test = train_test_split(X,
y,
train_size=10000,
random_state=1)
LR = LogisticRegression()
lr_model = LR.fit(X_train,y_train)
predicted_prob = lr_model.predict_proba(X_test)
predicted_default_prob= predicted_prob[:,1]
fpr, tpr, _ = roc_curve(y_test, predicted_default_prob)
roc_auc = auc(fpr, tpr)
draw_curve(fpr,tpr,roc_auc,'2.4 Out-of-Sample Test')

manylinux
- 粉丝: 4442
- 资源: 2491
最新资源
- bdwptqmxgj11.zip
- onnxruntime-win-x86
- onnxruntime-win-x64-gpu-1.20.1.zip
- vs2019 c++20 语法规范 头文件 <ratio> 的源码阅读与注释,处理分数的存储,加减乘除,以及大小比较等运算
- 首次尝试使用 Win,DirectX C++ 中的形状渲染套件.zip
- 预乘混合模式是一种用途广泛的三合一混合模式 它已经存在很长时间了,但似乎每隔几年就会被重新发现 该项目包括使用预乘 alpha 的描述,示例和工具 .zip
- 项目描述 DirectX 引擎支持版本 9、10、11 库 Microsoft SDK 功能相机视图、照明、加载网格、动画、蒙皮、层次结构界面、动画控制器、网格容器、碰撞系统 .zip
- 项目 wiki 文档中使用的代码教程的源代码库.zip
- 面向对象的通用GUI框架.zip
- 基于Java语言的PlayerBase游戏角色设计源码
资源上传下载、课程学习等过程中有任何疑问或建议,欢迎提出宝贵意见哦~我们会及时处理!
点击此处反馈


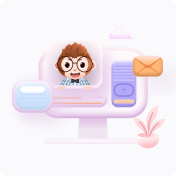