# mAP (mean Average Precision)
[](https://github.com/Cartucho/mAP)
This code will evaluate the performance of your neural net for object recognition.
<p align="center">
<img src="https://user-images.githubusercontent.com/15831541/37559643-6738bcc8-2a21-11e8-8a07-ed836f19c5d9.gif" width="450" height="300" />
</p>
In practice, a **higher mAP** value indicates a **better performance** of your neural net, given your ground-truth and set of classes.
## Citation
This project was developed for the following paper, please consider citing it:
```bibtex
@INPROCEEDINGS{8594067,
author={J. {Cartucho} and R. {Ventura} and M. {Veloso}},
booktitle={2018 IEEE/RSJ International Conference on Intelligent Robots and Systems (IROS)},
title={Robust Object Recognition Through Symbiotic Deep Learning In Mobile Robots},
year={2018},
pages={2336-2341},
}
```
## Table of contents
- [Explanation](#explanation)
- [Prerequisites](#prerequisites)
- [Quick start](#quick-start)
- [Running the code](#running-the-code)
- [Authors](#authors)
## Explanation
The performance of your neural net will be judged using the mAP criterium defined in the [PASCAL VOC 2012 competition](http://host.robots.ox.ac.uk/pascal/VOC/voc2012/). We simply adapted the [official Matlab code](http://host.robots.ox.ac.uk/pascal/VOC/voc2012/#devkit) into Python (in our tests they both give the same results).
First (**1.**), we calculate the Average Precision (AP), for each of the classes present in the ground-truth. Finally (**2.**), we calculate the mAP (mean Average Precision) value.
#### 1. Calculate AP
For each class:
First, your neural net **detection-results** are sorted by decreasing confidence and are assigned to **ground-truth objects**. We have "a match" when they share the **same label and an IoU >= 0.5** (Intersection over Union greater than 50%). This "match" is considered a true positive if that ground-truth object has not been already used (to avoid multiple detections of the same object).
<img src="https://user-images.githubusercontent.com/15831541/37725175-45b9e1a6-2d2a-11e8-8c15-2fb4d716ca9a.png" width="35%" height="35%" />
Using this criterium, we calculate the precision/recall curve. E.g:
<img src="https://user-images.githubusercontent.com/15831541/43008995-64dd53ce-8c34-11e8-8a2c-4567b1311910.png" width="45%" height="45%" />
Then we compute a version of the measured precision/recall curve with **precision monotonically decreasing** (shown in light red), by setting the precision for recall `r` to the maximum precision obtained for any recall `r' > r`.
Finally, we compute the AP as the **area under this curve** (shown in light blue) by numerical integration.
No approximation is involved since the curve is piecewise constant.
#### 2. Calculate mAP
We calculate the mean of all the AP's, resulting in an mAP value from 0 to 100%. E.g:
<img src="https://user-images.githubusercontent.com/15831541/38933241-5f9556ae-4310-11e8-9d47-cb205f9b103b.png"/>
<img src="https://user-images.githubusercontent.com/15831541/38933180-366b6fca-4310-11e8-99b9-17ad4b159b86.png" />
## Prerequisites
You need to install:
- [Python](https://www.python.org/downloads/)
Optional:
- **plot** the results by [installing Matplotlib](https://matplotlib.org/users/installing.html) - Linux, macOS and Windows:
1. `python -mpip install -U pip`
2. `python -mpip install -U matplotlib`
- show **animation** by installing [OpenCV](https://www.opencv.org/):
1. `python -mpip install -U pip`
2. `python -mpip install -U opencv-python`
## Quick-start
To start using the mAP you need to clone the repo:
```
git clone https://github.com/Cartucho/mAP
```
## Running the code
Step by step:
1. [Create the ground-truth files](#create-the-ground-truth-files)
2. Copy the ground-truth files into the folder **input/ground-truth/**
3. [Create the detection-results files](#create-the-detection-results-files)
4. Copy the detection-results files into the folder **input/detection-results/**
5. Run the code:
```
python main.py
```
Optional (if you want to see the **animation**):
6. Insert the images into the folder **input/images-optional/**
#### PASCAL VOC, Darkflow and YOLO users
In the [scripts/extra](https://github.com/Cartucho/mAP/tree/master/scripts/extra) folder you can find additional scripts to convert **PASCAL VOC**, **darkflow** and **YOLO** files into the required format.
#### Create the ground-truth files
- Create a separate ground-truth text file for each image.
- Use **matching names** for the files (e.g. image: "image_1.jpg", ground-truth: "image_1.txt").
- In these files, each line should be in the following format:
```
<class_name> <left> <top> <right> <bottom> [<difficult>]
```
- The `difficult` parameter is optional, use it if you want the calculation to ignore a specific detection.
- E.g. "image_1.txt":
```
tvmonitor 2 10 173 238
book 439 157 556 241
book 437 246 518 351 difficult
pottedplant 272 190 316 259
```
#### Create the detection-results files
- Create a separate detection-results text file for each image.
- Use **matching names** for the files (e.g. image: "image_1.jpg", detection-results: "image_1.txt").
- In these files, each line should be in the following format:
```
<class_name> <confidence> <left> <top> <right> <bottom>
```
- E.g. "image_1.txt":
```
tvmonitor 0.471781 0 13 174 244
cup 0.414941 274 226 301 265
book 0.460851 429 219 528 247
chair 0.292345 0 199 88 436
book 0.269833 433 260 506 336
```
## Authors:
* **João Cartucho**
Feel free to contribute
[](https://github.com/Cartucho/mAP/graphs/contributors)
没有合适的资源?快使用搜索试试~ 我知道了~
温馨提示
1、该资源内项目代码经过严格调试,下载即用确保可以运行! 2、该资源适合计算机相关专业(如计科、人工智能、大数据、数学、电子信息等)正在做课程设计、期末大作业和毕设项目的学生、或者相关技术学习者作为学习资料参考使用。 3、该资源包括全部源码,需要具备一定基础才能看懂并调试代码。 基于keras-yolov3进行烟火数据集训练及识别(python开发源码+项目说明).zip 基于keras-yolov3进行烟火数据集训练及识别(python开发源码+项目说明).zip 基于keras-yolov3进行烟火数据集训练及识别(python开发源码+项目说明).zip 基于keras-yolov3进行烟火数据集训练及识别(python开发源码+项目说明).zip 基于keras-yolov3进行烟火数据集训练及识别(python开发源码+项目说明).zip 基于keras-yolov3进行烟火数据集训练及识别(python开发源码+项目说明).zip 基于keras-yolov3进行烟火数据集训练及识别(python开发源码+项目说明).zip
资源推荐
资源详情
资源评论
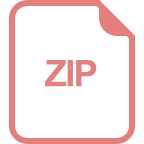
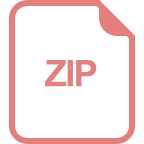
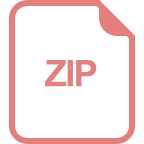
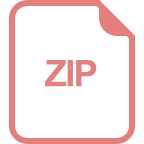
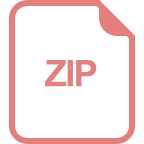
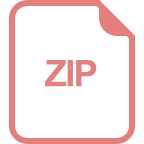
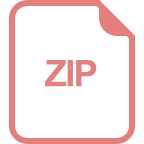
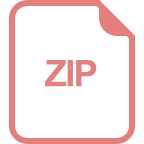
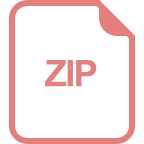
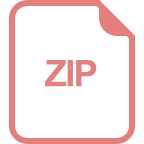
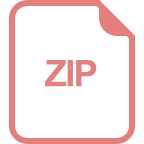
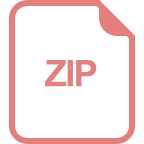
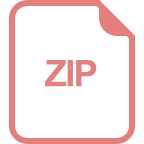
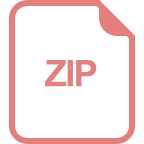
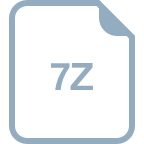
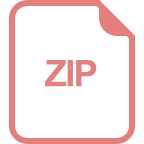
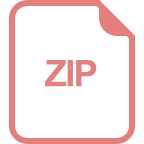
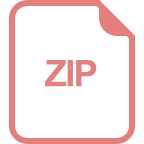
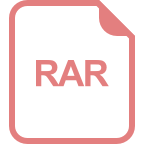
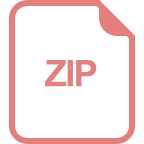
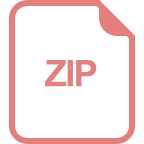
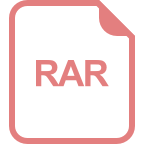
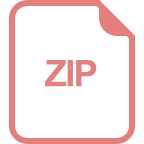
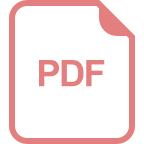
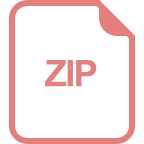
收起资源包目录

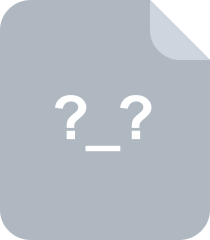
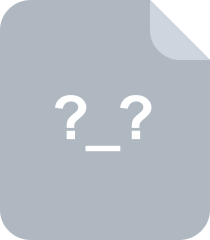
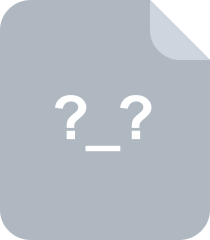
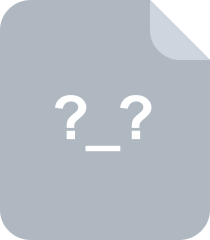
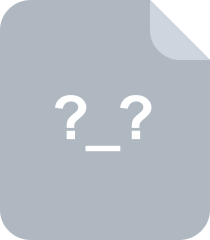
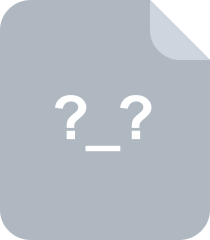
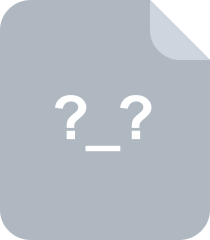
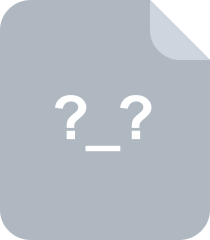
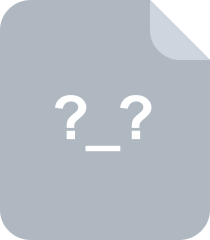
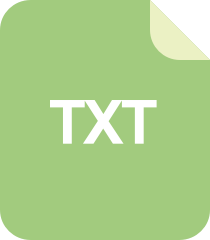
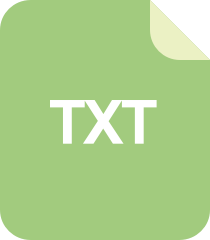
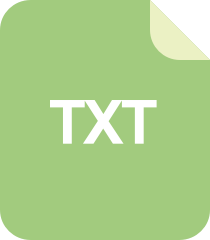
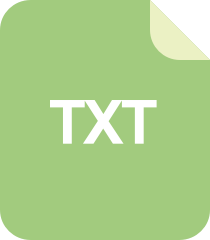
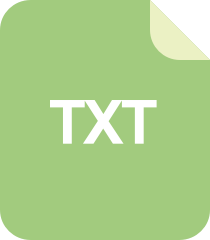
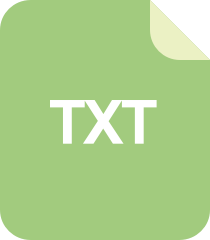
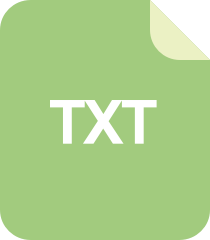
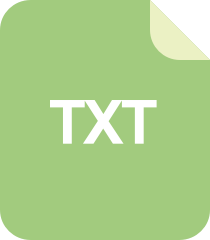
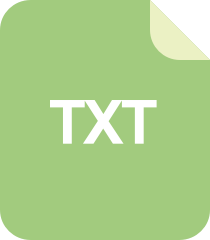
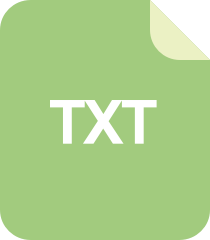
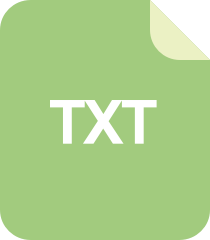
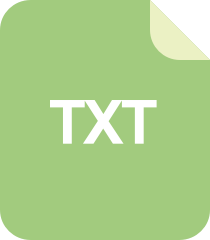
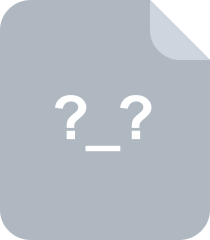
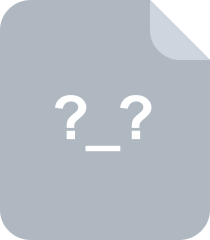
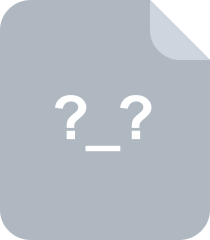
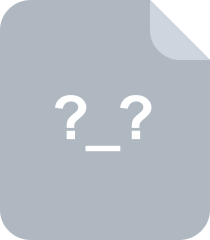
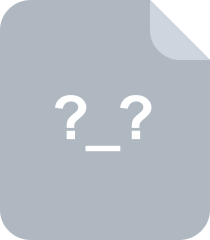
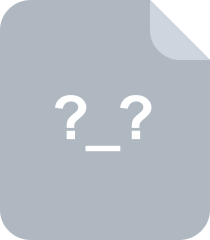
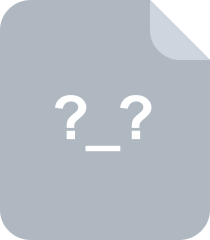
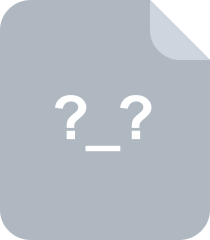
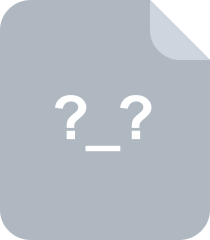
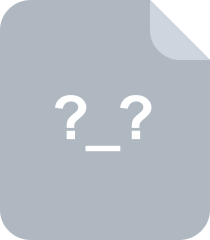
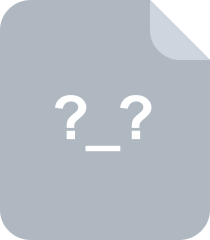
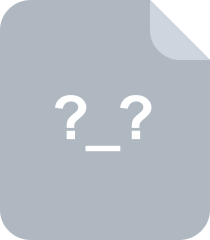
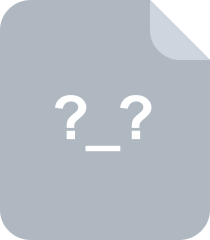
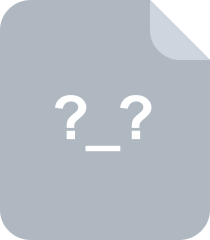
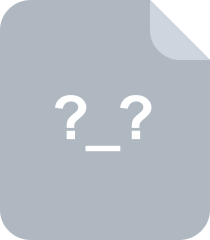
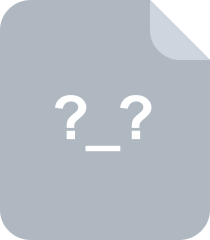
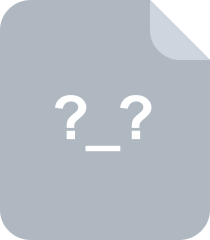
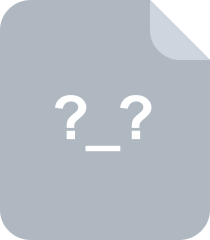
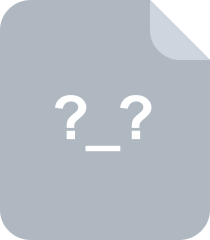
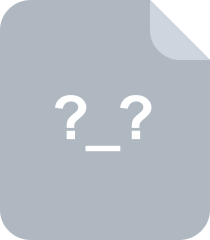
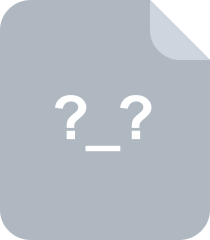
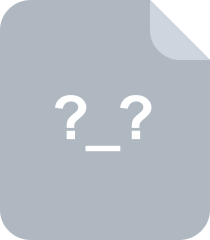
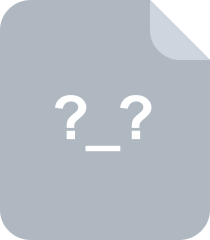
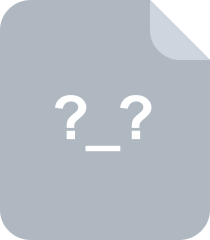
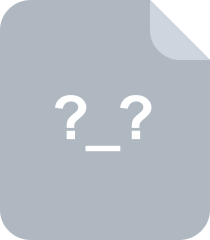
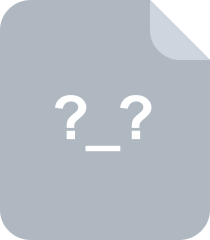
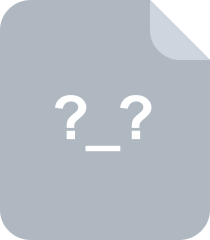
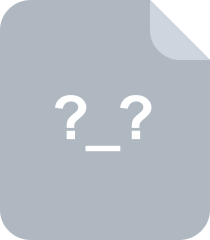
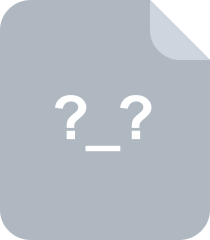
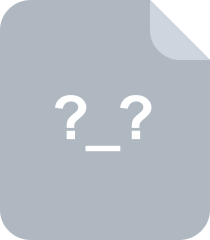
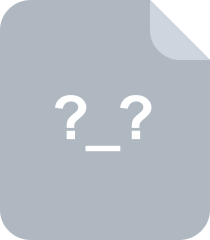
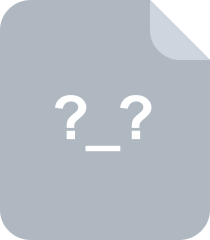
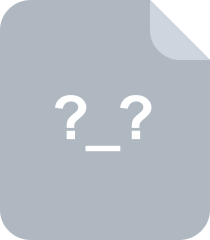
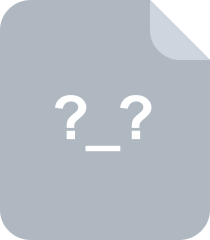
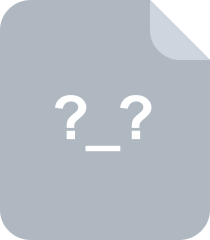
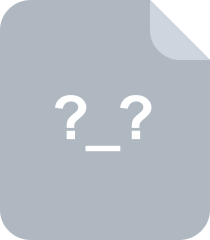
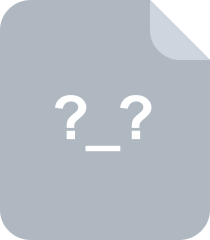
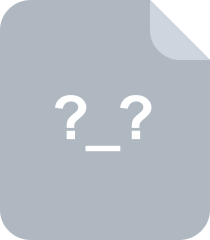
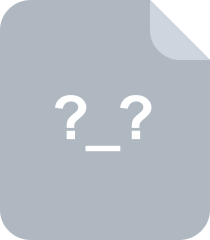
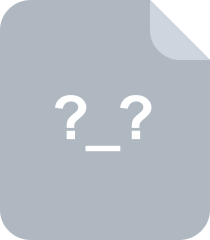
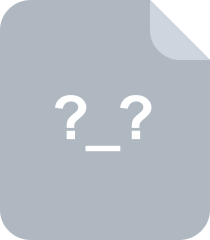
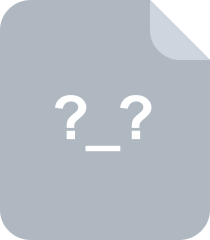
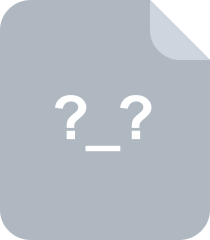
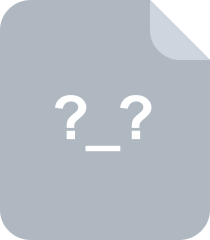
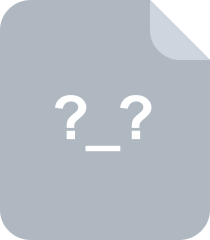
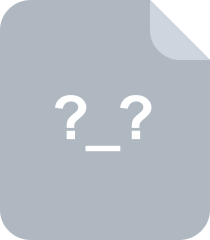
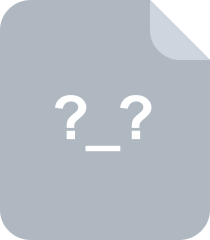
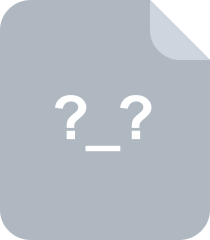
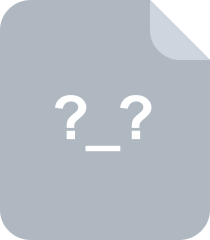
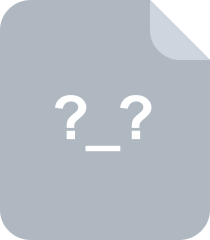
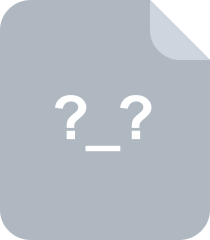
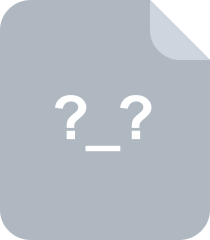
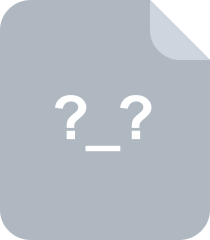
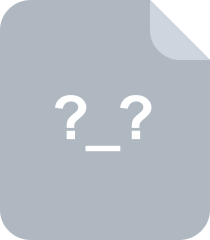
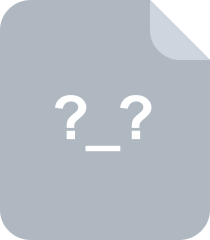
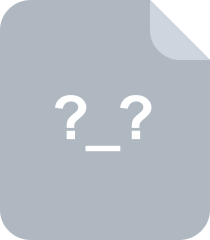
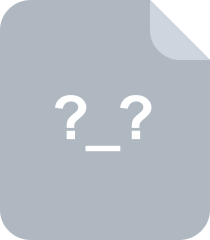
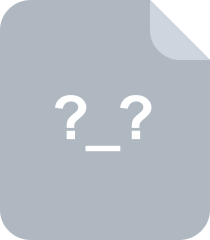
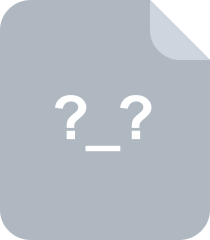
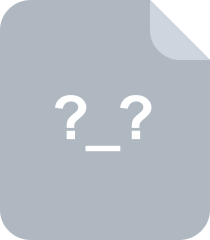
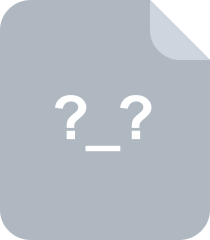
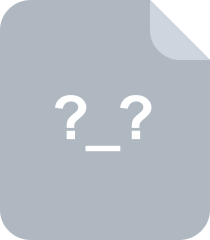
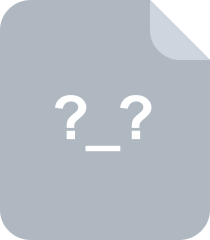
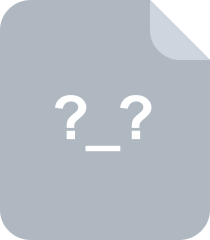
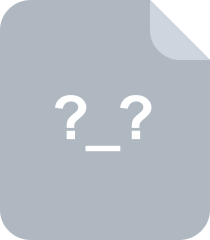
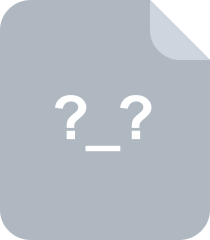
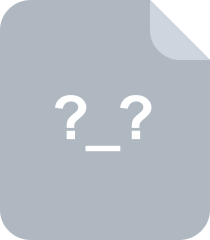
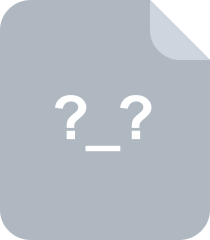
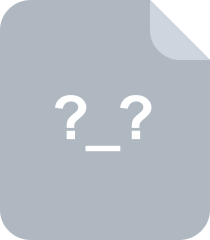
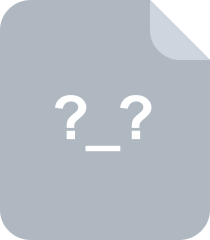
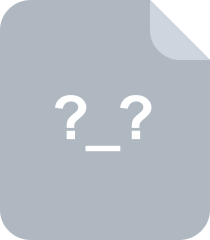
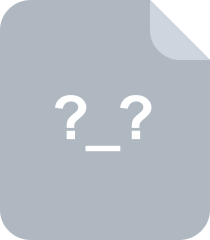
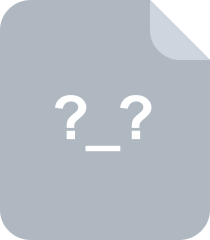
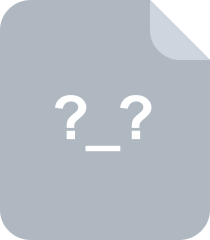
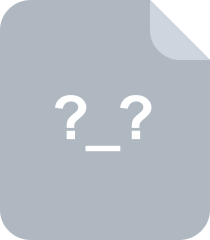
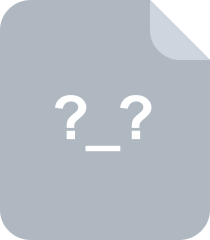
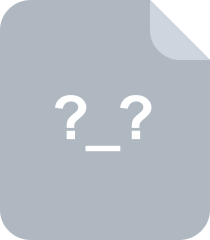
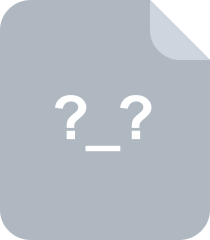
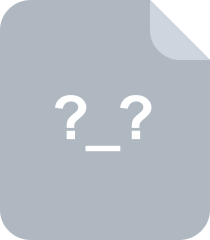
共 2000 条
- 1
- 2
- 3
- 4
- 5
- 6
- 20
资源评论
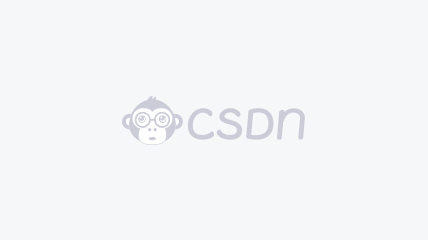


辣椒种子
- 粉丝: 4242
- 资源: 5837
上传资源 快速赚钱
我的内容管理 展开
我的资源 快来上传第一个资源
我的收益
登录查看自己的收益我的积分 登录查看自己的积分
我的C币 登录后查看C币余额
我的收藏
我的下载
下载帮助

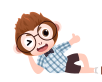
最新资源
- Windows检查电池健康度的批处理脚本实现
- 用HTML5和JavaScript实现动态过年鞭炮场景
- 快速排序在Go中的高效实现与应用
- 对象检测23-YOLO(v5至v11)、COCO、CreateML、Paligemma、TFRecord、VOC数据集合集.rar
- 云原生-k8s知识学习-CKA考前培训
- Python实现HTML压缩功能
- 完结26章Java主流分布式解决方案多场景设计与实战
- ECSHOP模板堂最新2017仿E宠物模板 整合ECTouch微分销商城
- Pear Admin 是 一 款 开 箱 即 用 的 前 端 开 发 模 板,提供便捷快速的开发方式,延续 Admin 的设计规范
- 51单片机仿真摇号抽奖机源程序12864液晶显示仿真+程序
资源上传下载、课程学习等过程中有任何疑问或建议,欢迎提出宝贵意见哦~我们会及时处理!
点击此处反馈


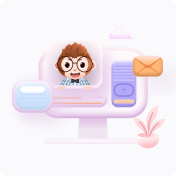
安全验证
文档复制为VIP权益,开通VIP直接复制
