# yolov3_pytorch_ros
This package provides a ROS wrapper for [YOLOv3](https://pjreddie.com/darknet/yolo) based on [PyTorch-YOLOv3](https://github.com/eriklindernoren/PyTorch-YOLOv3). For consistency, the [messages](msg) are based on the [darknet_ros](https://github.com/leggedrobotics/darknet_ros) package. The package has been tested with Ubuntu 16.04 and ROS Kinetic on a NVIDIA Jetson TX2.
**Authors**: Vasileios Vasilopoulos (<vvasilo@seas.upenn.edu>), Georgios Pavlakos (<pavlakos@seas.upenn.edu>)
**Affiliation**: [University of Pennsylvania, GRASP Lab](https://www.grasp.upenn.edu)
## Prerequisites
To download the prerequisites for this package (except for ROS itself), use the [ROS-default `rosdep` to install the required dependencies](http://wiki.ros.org/ROS/Tutorials/rosdep):
```
cd /your/catkin_ws
rosdep install --from-paths src --ignore-src -y
```
Alternatively you can use the python standard `requirements.txt` file to install the dependencies as well. Navigate to the package folder and run:
```
$ sudo pip install -r requirements.txt
```
## Installation
Aftre making sure the required dependencies are installed following the instructions above, navigate to your catkin workspace and run:
```
$ catkin_make --pkg yolov3_pytorch_ros
```
## Basic Usage
1. First, make sure to put your weights in the [models](models) folder. This should automatically be done for the default configuration during the compilation process, however if you'd like to use your own make sure that they exist before you use them. For the **training process** in order to use custom objects, please refer to the original [YOLO page](https://pjreddie.com/darknet/yolo/).
By default during the build the following pre-trained weights are downloaded:
```
# download yolov3.weights
wget http://pjreddie.com/media/files/yolov3.weights
# dowload yolov3-tiny.weights
wget http://pjreddie.com/media/files/yolov3-tiny.weights
```
2. The default settings (using `yolov3.weights`) in the `launch/detector.launch` file should work, all you should have to do is change the image topic you would like to subscribe to:
```
roslaunch yolov3_pytorch_ros detector.launch image_topic:=/your/image/topic
```
You can also try out the `yolov3-tiny.weights` by simply passing in different arguments at launch. This is the recommended usage on a CPU for dramatically increased framerate with slightly reduced performance:
```
roslaunch yolov3_pytorch_ros detector.launch image_topic:=/your/image/topic config_name:=yolov3-tiny.cfg weights_name:=yolov3-tiny.weights confidence:=0.1
```
The `confidence` argument can be adjusted to set a threshold for detected objects to be ignored.
Alternatively you can modify the parameters in the [launch file](launch/detector.launch), recompile and launch it that way so that no arguments need to be passed at runtime.
### Node parameters
* **`image_topic`** (string)
Subscribed camera topic.
* **`weights_name`** (string)
Weights to be used from the [models](models) folder.
* **`config_name`** (string)
The name of the configuration file in the [config](config) folder. Use `yolov3.cfg` for YOLOv3, `yolov3-tiny.cfg` for tiny YOLOv3, and `yolov3-voc.cfg` for YOLOv3-VOC.
* **`classes_name`** (string)
The name of the file for the detected classes in the [classes](classes) folder. Use `coco.names` for COCO, and `voc.names` for VOC.
* **`publish_image`** (bool)
Set to true to get the camera image along with the detected bounding boxes, or false otherwise.
* **`detected_objects_topic`** (string)
Published topic with the detected bounding boxes.
* **`detections_image_topic`** (string)
Published topic with the detected bounding boxes on top of the image.
* **`confidence`** (float)
Confidence threshold for detected objects.
### Subscribed topics
* **`image_topic`** (sensor_msgs::Image)
Subscribed camera topic.
### Published topics
* **`detected_objects_topic`** (yolov3_pytorch_ros::BoundingBoxes)
Published topic with the detected bounding boxes.
* **`detections_image_topic`** (sensor_msgs::Image)
Published topic with the detected bounding boxes on top of the image (only published if `publish_image` is set to true).
## Citing
The YOLO methods used in this software are described in the paper: [You Only Look Once: Unified, Real-Time Object Detection](https://arxiv.org/abs/1506.02640).
If you are using this package, please add the following citation to your publication:
@misc{vasilopoulos_pavlakos_yolov3ros_2019,
author = {Vasileios Vasilopoulos and Georgios Pavlakos},
title = {{yolov3_pytorch_ros}: Object Detection for {ROS} using {PyTorch}},
howpublished = {\url{https://github.com/vvasilo/yolov3_pytorch_ros}},
year = {2019},
}

徐浪老师
- 粉丝: 8496
- 资源: 1万+
最新资源
- 基于GPRS的环境监测数据传输系统的研究
- 基于FPGA的TFT-LCD视频驱动系统设计
- 基于MSP430的智能家居系统的设计与实现
- 基于LabVIEW喷雾干燥机模糊控制系统的设计
- calibre-7.23.0.dmg
- 机械设计汽车车身底板装配线sw21全套设计资料100%好用.zip.zip
- 机械设计汽车玻璃延时料架设备(sw18可编辑+工程图+BOM)全套设计资料100%好用.zip.zip
- 机械设计平板主板整流罩贴泡棉机sw18可编辑全套设计资料100%好用.zip.zip
- 机械设计汽车天窗装配线sw16全套设计资料100%好用.zip.zip
- 机械设计汽车天窗底涂工作站(sw18可编辑+工程图+BOM)全套设计资料100%好用.zip.zip
- 机械设计汽车连接器插端包装一体机sw2016可编辑全套设计资料100%好用.zip.zip
- 机械设计汽车头枕盖自动去毛刺设备sw2016全套设计资料100%好用.zip.zip
- 机械设计全自动导管检测机(sw18可编辑+BOM)全套设计资料100%好用.zip.zip
- 机械设计全自动动平衡量测移载机stp全套设计资料100%好用.zip.zip
- 机械设计全自动上料双工位锁螺丝机stp全套设计资料100%好用.zip.zip
- 机械设计全自动端子插针机sw18全套设计资料100%好用.zip.zip
资源上传下载、课程学习等过程中有任何疑问或建议,欢迎提出宝贵意见哦~我们会及时处理!
点击此处反馈


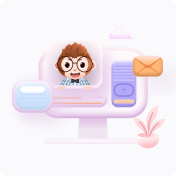