# Face_recognition_attendance_system
# The Basic Approach
This is my attempt to make a Face recognition system for classroom or office attendance. The system is based on a special type of cnn architecture known as a siamese network. Such a network is trained to generate a very accurate and almost unique 128 vector given that the images of face which a are fed to the network are properly aligned and cropped. <br>
Then another dense neural network is trained taking input these embeddings. The second neural network is only for classification purposes. Then the person who is identified by the system, his/her attendance in the system is incremented by 1.<br>
When the system is closed, a excel file consisting of attendance of all the students is generated.
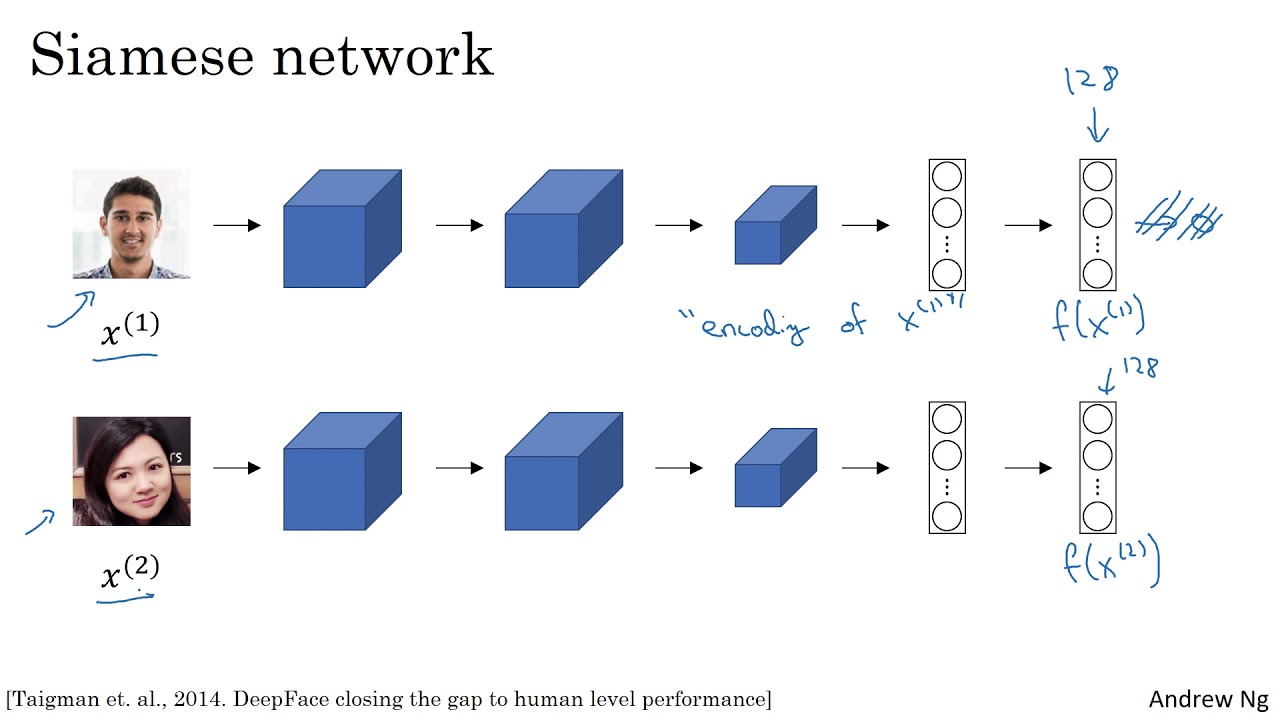
taken from DeepLearning.ai.<br>
You can watch these videos. Professor Andrew Ng gives an excellent explanation to these networks.
# Embedding Generator
I have download the pretrained facenet model from <a href="https://github.com/nyoki-mtl/keras-facenet"> nyoki-mtl githubu </a><br>
This network is pretrained on a pretty large dataset, and produces a unique 128 dimensional vector for a particular face given the images fed to it are cropped to only the face region and are alligned. The input size of image for this netowrk is 160X160X3
# Face Detection
Face detection is acheived by using haar cascades of opencv. Face detection haarcascade is used to detect the face and this detected region is fed to the embedding generator.
# The second neural net
The second neural network has a dense architecture and is used for classification. The second neural network take input the 128 dimensional vector and ouputs the probability of the face to be one of the student.The architecture of the second neural network is
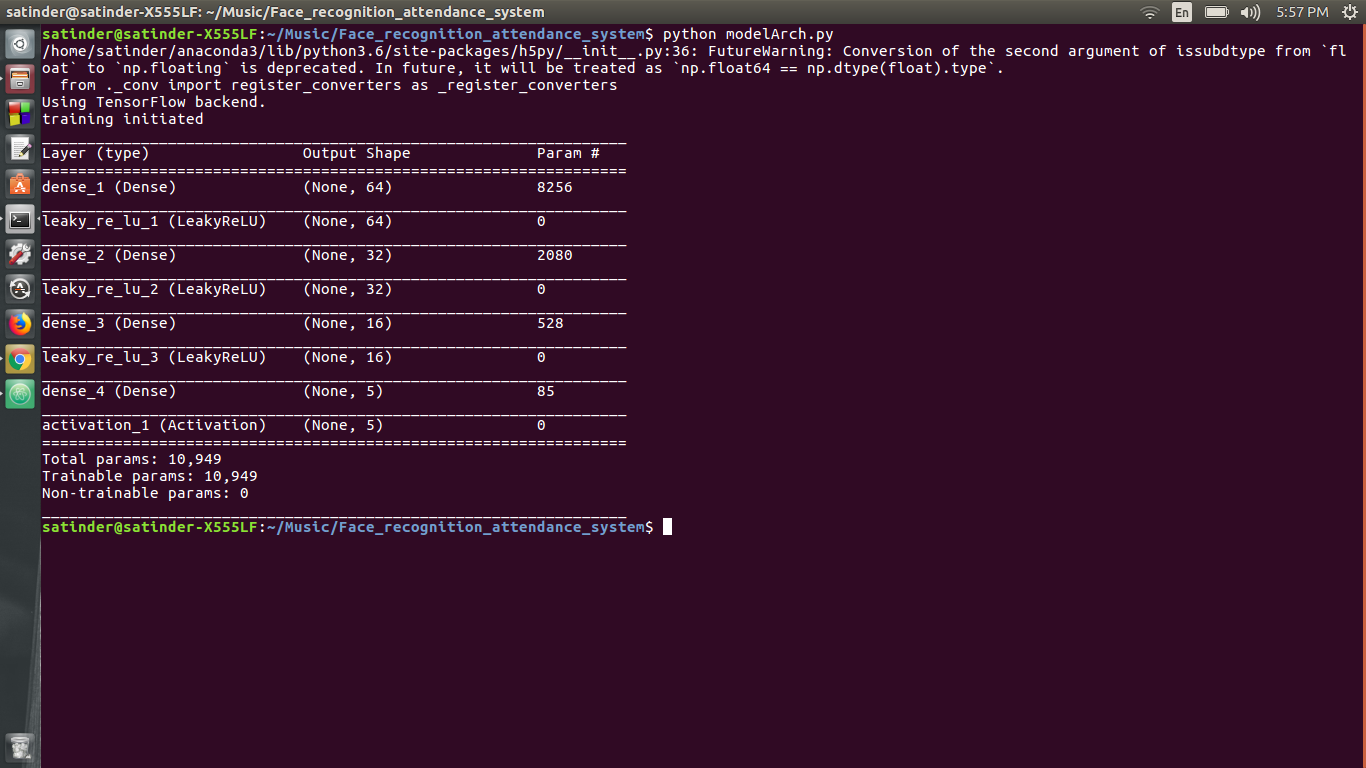
# Updation of attendance
The database used is mongodb. Pymongo is used to add, delete records and also increment the attendance of the particular student.
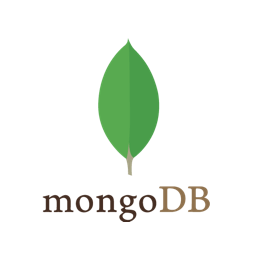
# csv file generation
After the application is closed, an excel file is generated. This excel file contains the attendance of all the student.
# Requiremnents
## Installing the requirements
1. Start your terminal of cmd depending on your os.
2. If you have a NVidia GPU then make sure you have the prerequisites for Tensorflow GPU installation (Refer to official site). Then use this commmand
pip install -r requirements_gpu.txt
3. In case you do not have a GPU then use this command
pip install -r requirements_cpu.txt
<br>
Apart from all this you also have to install mongodb in your system.
# Want to run it on your own
1)Install all the requirements<br><br>
2)Make a folder named "people" without quotes<br><br>
3)Now run Generating_training_data.py, when this runs enter the name of the person followed by a index beginning from zero
for example, if I want to generate data for "ravi", I will write "ravi0" and for the next name write "secondname1", just make sure the index given to everybody is in increasing order. Now put all this folders into the people folder<br><br>
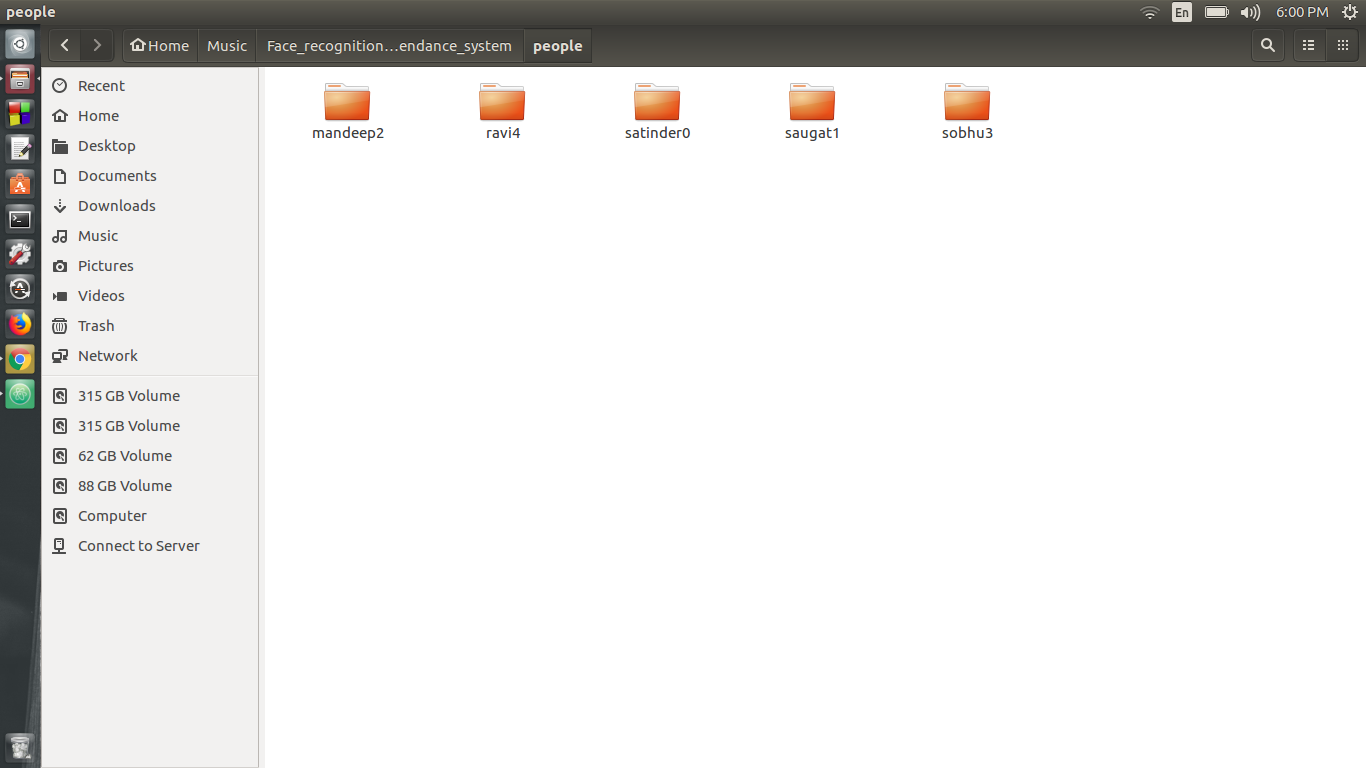
4)Now in trainer.py change the number of classes according to number of folder and then run trainer.py<br><br>
5)The model will be trained.<br><br>
6)Now create a database using mongodb. Enter all the names with their attendance. This can be acheived by <br>
a)create a data base named "new"<br>
b)create a collection named "pa"<br>
c)add the enteries. For eg db.pa.insert({"name":"satinder","attendance":0})<br><br>
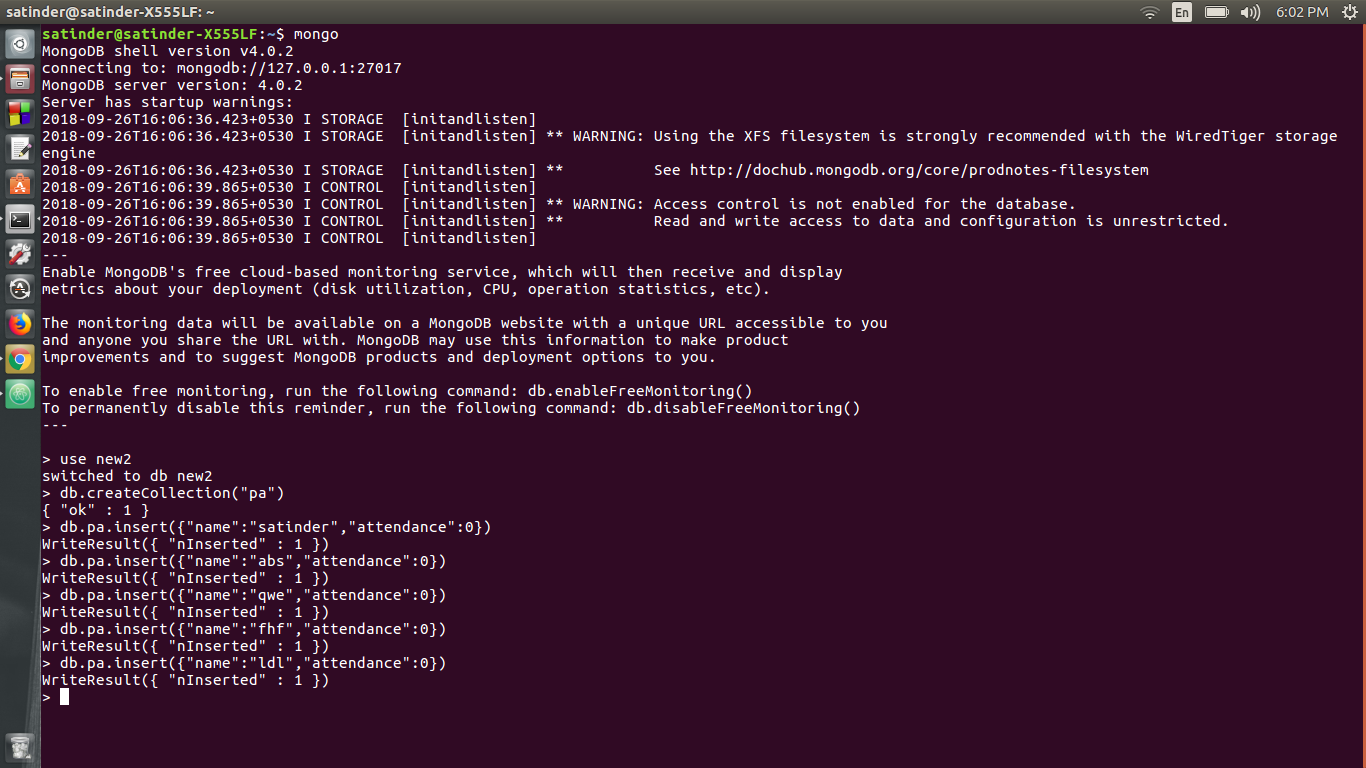
7)Now open recognizer.py and change the dictionary "a" and people according to your data. The key of array "a" is the index of the people and the data is a indicating variable which is used to indicate that in a particular session, if the person attendance has been taken.<br><br>
8)Dictionary "people" is self explanatory.<br><br>
9)Run recognizer.py to recognize people. Their attendance will be registered in the mongodb database.
## Results
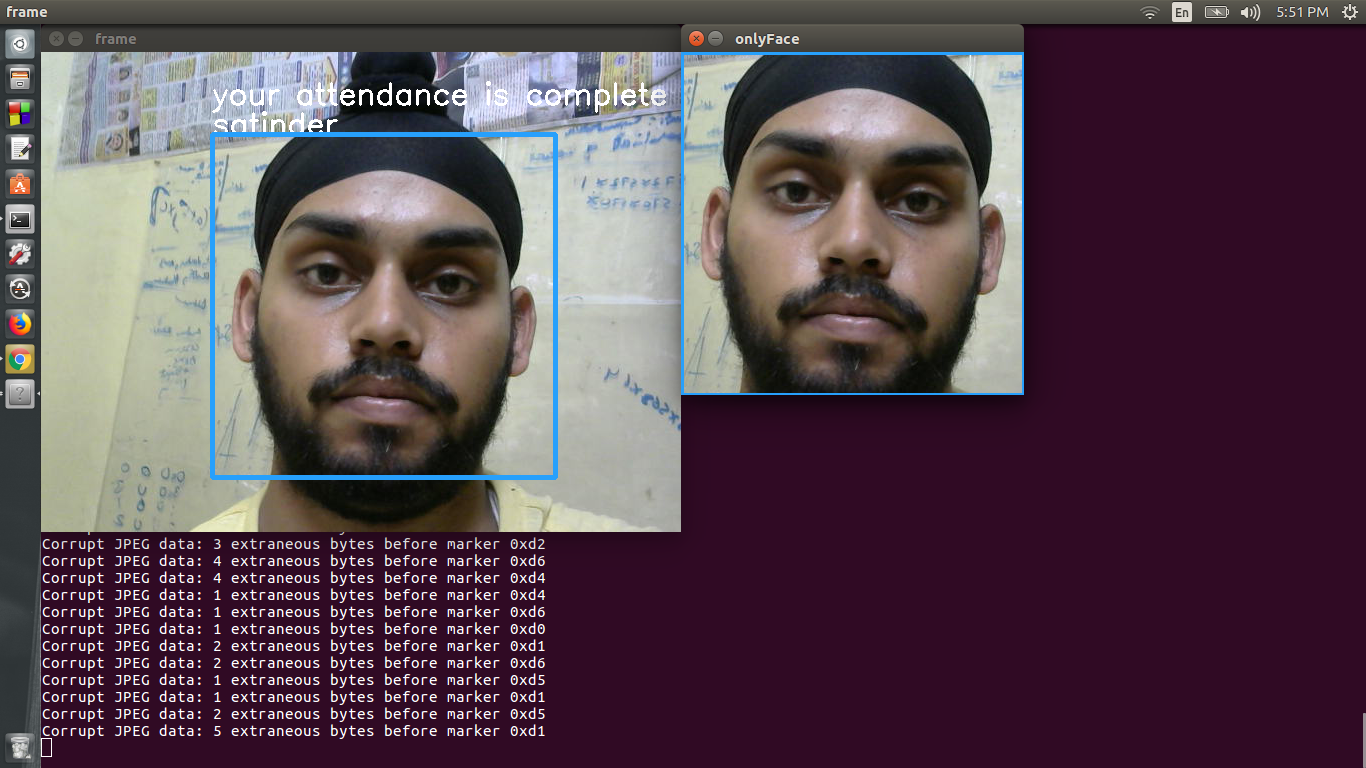
## Updated attendance in the database
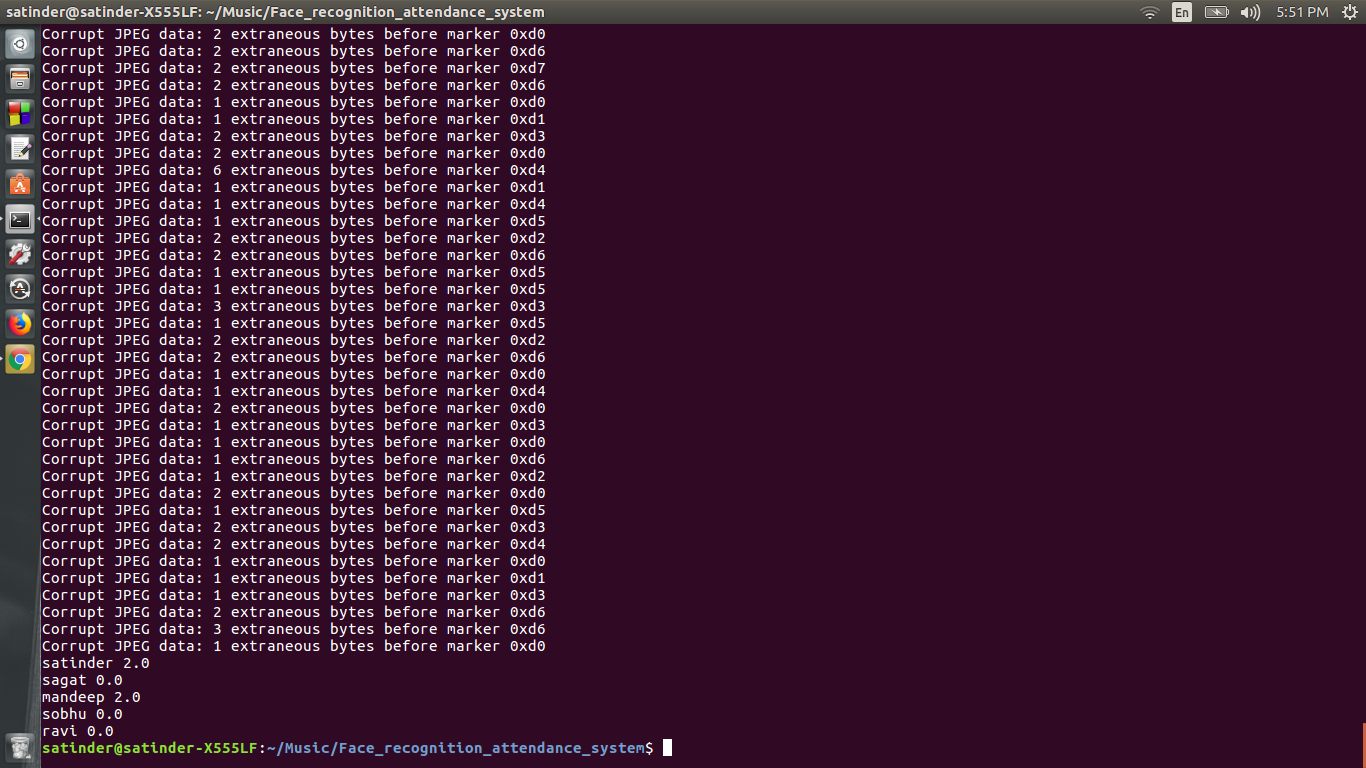
## Liked it
If you liked it you will surely like my other repos as well. You can also have a look at my youtube channel <a href="https://www.youtube.com/c/reactorscience">"reactor science"</a>. If you have any doubts you can contact me on my facebook page <a href="https://www.facebook.com/pg/reactorscience/about/">"reactor science"</a>
## References
1)Deep learning with python by Francois Chollet<br>
2)keras.io<br>
3)Deeplearning.ai by coursera(prof Andrew Ng)<br>
4)CS231n by stanford<br>
5)Pyimagesearch.com(Adrian Rosenberg)<br>
6)Brandon Amos(github:https://github.com/bamos)
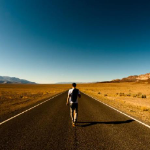
程序员无锋
- 粉丝: 3702
- 资源: 2564
最新资源
- 学习用资源方便随时用,感觉挺方便
- 以太网发展及测试方法解析
- 备战19届全国大学生智能汽车竞赛源码+文档说明.zip
- BLDC无刷直流电机和PMSM永磁同步电机 基于stm32F1的有传感器和无传感驱动 直流无刷电机有传感器和无传感驱动程序, 无传感的实现是基于反电动势过零点实现的,有传感是霍尔实现 永磁同步电机
- 基于opencv文档识别扫描OCR识别(完整代码python)
- 从基础理论到实际应用的MIPI C-PHY简介
- 碳交易机制下考虑需求响应的综合能源系统优化运行 综合能源系统是实现“双碳”目标的有效途径,为进一步挖掘其需求侧可调节潜力对碳减排的作用,提出了一种碳交易机制下考虑需求响应的综合能源系统优化运行模型
- 大学数学实验期末大作业指南:探究性与实践性实验要求详解
- 元旦快乐烟花动画效果代码解析(基于canvas画布实现)
- 重庆文理学院大数据技术原理及实践课程期末项目-学前教育大数据平台构建与应用
- python+Flask+SQLite制作的一个网页博客系统
- 中国2014-2020年石油加工产品产量数据处理及可视化分析
- 2024-2025学年《社交网络分析》大论文提交与评估指南
- 实现10负荷点的配电网蒙特卡洛可靠性计算matlab程序,代码有注释
- MikroTik RouterOS 7.16.2版本开始支持使用img镜像安装版,授权全部教程
- 基于QCM传感器的五种醇类分类实验与数据分析
资源上传下载、课程学习等过程中有任何疑问或建议,欢迎提出宝贵意见哦~我们会及时处理!
点击此处反馈


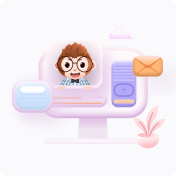