<p align="center">
<img height="150" src="https://raw.githubusercontent.com/pyg-team/pyg_sphinx_theme/master/pyg_sphinx_theme/static/img/pyg_logo_text.svg?sanitize=true" />
</p>
______________________________________________________________________
[![PyPI Version][pypi-image]][pypi-url]
[![Testing Status][testing-image]][testing-url]
[![Linting Status][linting-image]][linting-url]
[![Docs Status][docs-image]][docs-url]
[![Contributing][contributing-image]][contributing-url]
[![Slack][slack-image]][slack-url]
**[Documentation](https://pytorch-geometric.readthedocs.io)** | **[Paper](https://arxiv.org/abs/1903.02428)** | **[Colab Notebooks and Video Tutorials](https://pytorch-geometric.readthedocs.io/en/latest/get_started/colabs.html)** | **[External Resources](https://pytorch-geometric.readthedocs.io/en/latest/external/resources.html)** | **[OGB Examples](https://github.com/snap-stanford/ogb/tree/master/examples)**
**PyG** *(PyTorch Geometric)* is a library built upon [PyTorch](https://pytorch.org/) to easily write and train Graph Neural Networks (GNNs) for a wide range of applications related to structured data.
It consists of various methods for deep learning on graphs and other irregular structures, also known as *[geometric deep learning](http://geometricdeeplearning.com/)*, from a variety of published papers.
In addition, it consists of easy-to-use mini-batch loaders for operating on many small and single giant graphs, [multi GPU-support](https://github.com/pyg-team/pytorch_geometric/tree/master/examples/multi_gpu), [`torch.compile`](https://pytorch-geometric.readthedocs.io/en/latest/advanced/compile.html) support, [`DataPipe`](https://github.com/pyg-team/pytorch_geometric/blob/master/examples/datapipe.py) support, a large number of common benchmark datasets (based on simple interfaces to create your own), the [GraphGym](https://pytorch-geometric.readthedocs.io/en/latest/advanced/graphgym.html) experiment manager, and helpful transforms, both for learning on arbitrary graphs as well as on 3D meshes or point clouds.
**[Click here to join our Slack community!][slack-url]**
<p align="center">
<a href="https://medium.com/stanford-cs224w"><img style="max-width=: 941px" src="https://data.pyg.org/img/cs224w_tutorials.png" /></a>
</p>
______________________________________________________________________
- [Library Highlights](#library-highlights)
- [Quick Tour for New Users](#quick-tour-for-new-users)
- [Architecture Overview](#architecture-overview)
- [Implemented GNN Models](#implemented-gnn-models)
- [Installation](#installation)
## Library Highlights
Whether you are a machine learning researcher or first-time user of machine learning toolkits, here are some reasons to try out PyG for machine learning on graph-structured data.
- **Easy-to-use and unified API**:
All it takes is 10-20 lines of code to get started with training a GNN model (see the next section for a [quick tour](#quick-tour-for-new-users)).
PyG is *PyTorch-on-the-rocks*: It utilizes a tensor-centric API and keeps design principles close to vanilla PyTorch.
If you are already familiar with PyTorch, utilizing PyG is straightforward.
- **Comprehensive and well-maintained GNN models**:
Most of the state-of-the-art Graph Neural Network architectures have been implemented by library developers or authors of research papers and are ready to be applied.
- **Great flexibility**:
Existing PyG models can easily be extended for conducting your own research with GNNs.
Making modifications to existing models or creating new architectures is simple, thanks to its easy-to-use message passing API, and a variety of operators and utility functions.
- **Large-scale real-world GNN models**:
We focus on the need of GNN applications in challenging real-world scenarios, and support learning on diverse types of graphs, including but not limited to: scalable GNNs for graphs with millions of nodes; dynamic GNNs for node predictions over time; heterogeneous GNNs with multiple node types and edge types.
- **GraphGym integration**: GraphGym lets users easily reproduce GNN experiments, is able to launch and analyze thousands of different GNN configurations, and is customizable by registering new modules to a GNN learning pipeline.
## Quick Tour for New Users
In this quick tour, we highlight the ease of creating and training a GNN model with only a few lines of code.
### Train your own GNN model
In the first glimpse of PyG, we implement the training of a GNN for classifying papers in a citation graph.
For this, we load the [Cora](https://pytorch-geometric.readthedocs.io/en/latest/generated/torch_geometric.datasets.Planetoid.html) dataset, and create a simple 2-layer GCN model using the pre-defined [`GCNConv`](https://pytorch-geometric.readthedocs.io/en/latest/generated/torch_geometric.nn.conv.GCNConv.html):
```python
import torch
from torch import Tensor
from torch_geometric.nn import GCNConv
from torch_geometric.datasets import Planetoid
dataset = Planetoid(root='.', name='Cora')
class GCN(torch.nn.Module):
def __init__(self, in_channels, hidden_channels, out_channels):
super().__init__()
self.conv1 = GCNConv(in_channels, hidden_channels)
self.conv2 = GCNConv(hidden_channels, out_channels)
def forward(self, x: Tensor, edge_index: Tensor) -> Tensor:
# x: Node feature matrix of shape [num_nodes, in_channels]
# edge_index: Graph connectivity matrix of shape [2, num_edges]
x = self.conv1(x, edge_index).relu()
x = self.conv2(x, edge_index)
return x
model = GCN(dataset.num_features, 16, dataset.num_classes)
```
<details>
<summary>We can now optimize the model in a training loop, similar to the <a href="https://pytorch.org/tutorials/beginner/basics/optimization_tutorial.html#full-implementation">standard PyTorch training procedure</a>.</summary>
```python
import torch.nn.functional as F
data = dataset[0]
optimizer = torch.optim.Adam(model.parameters(), lr=0.01)
for epoch in range(200):
pred = model(data.x, data.edge_index)
loss = F.cross_entropy(pred[data.train_mask], data.y[data.train_mask])
# Backpropagation
optimizer.zero_grad()
loss.backward()
optimizer.step()
```
</details>
More information about evaluating final model performance can be found in the corresponding [example](https://github.com/pyg-team/pytorch_geometric/blob/master/examples/gcn.py).
### Create your own GNN layer
In addition to the easy application of existing GNNs, PyG makes it simple to implement custom Graph Neural Networks (see [here](https://pytorch-geometric.readthedocs.io/en/latest/tutorial/create_gnn.html) for the accompanying tutorial).
For example, this is all it takes to implement the [edge convolutional layer](https://arxiv.org/abs/1801.07829) from Wang *et al.*:
$$x_i^{\\prime} ~ = ~ \\max\_{j \\in \\mathcal{N}(i)} ~ \\textrm{MLP}\_{\\theta} \\left( \[ ~ x_i, ~ x_j - x_i ~ \] \\right)$$
```python
import torch
from torch import Tensor
from torch.nn import Sequential, Linear, ReLU
from torch_geometric.nn import MessagePassing
class EdgeConv(MessagePassing):
def __init__(self, in_channels, out_channels):
super().__init__(aggr="max") # "Max" aggregation.
self.mlp = Sequential(
Linear(2 * in_channels, out_channels),
ReLU(),
Linear(out_channels, out_channels),
)
def forward(self, x: Tensor, edge_index: Tensor) -> Tensor:
# x: Node feature matrix of shape [num_nodes, in_channels]
# edge_index: Graph connectivity matrix of shape [2, num_edges]
return self.propagate(edge_index, x=x) # shape [num_nodes, out_channels]
def message(self, x_j: Tensor, x_i: Tensor) -> Tensor:
# x_j: Source node features of shape [num_edges, in_channels]
# x_i: Target node features of shape [num_edges, in_channels]
edge_features = torch.cat([x_i, x_j - x_i], dim=-1)
return self.mlp(edge_features)
没有合适的资源?快使用搜索试试~ 我知道了~
温馨提示
PyG (PyTorch Geometric) 是一个基于 PyTorch 构建的库,可轻松编写和训练图神经网络 (GNN),用于与结构化数据相关的各种应用程序。 它由各种已发表的论文中对图形和其他不规则结构进行深度学习的各种方法组成,也称为几何深度学习。 此外,它还包括易于使用的迷你批处理加载器,用于在许多小型和单个巨型图形上进行操作、多 GPU 支持、torch.compile 支持、DataPipe 支持、大量通用基准数据集(基于简单的界面创建自己的)、GraphGym 实验管理器和有用的转换,既可用于在任意图形上学习,也可用于在 3D 网格或点云上学习。
资源推荐
资源详情
资源评论
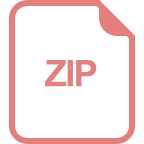
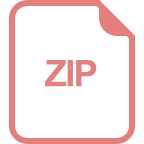
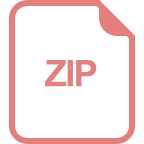
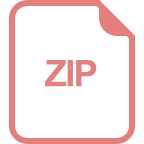
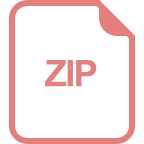
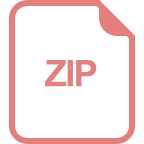
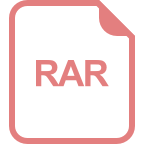
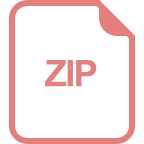
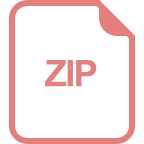
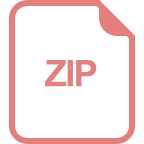
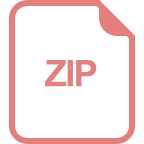
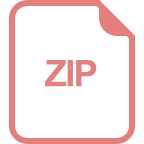
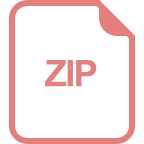
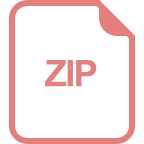
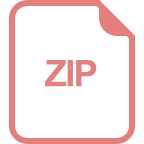
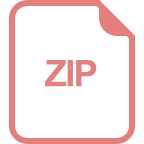
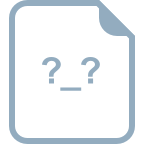
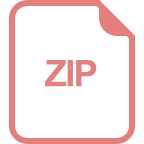
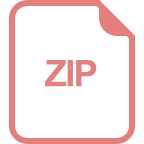
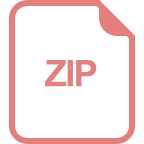
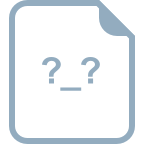
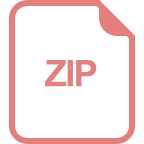
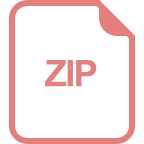
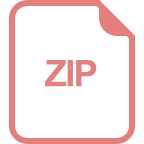
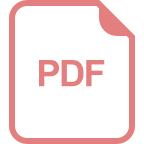
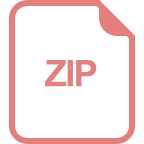
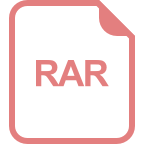
收起资源包目录

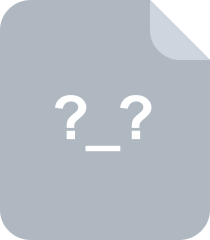
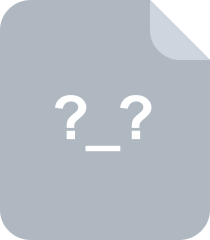
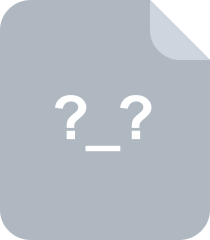
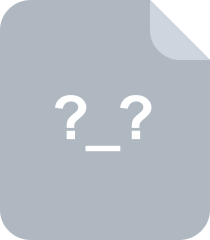
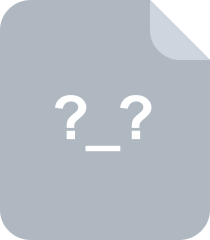
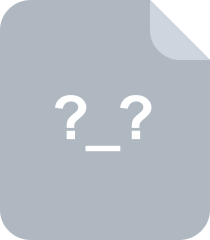
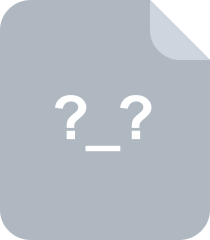
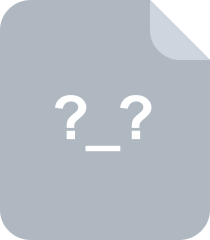
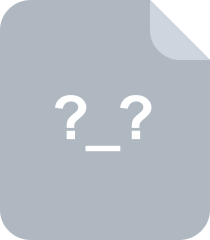
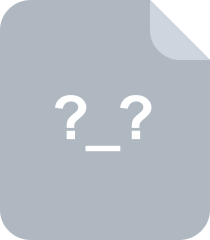
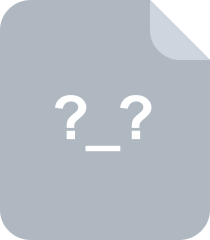
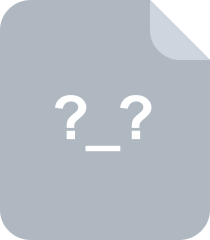
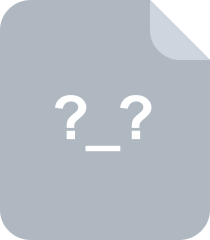
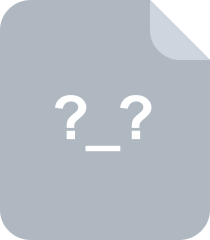
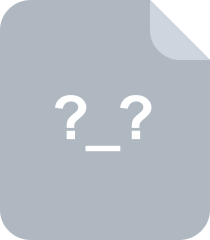
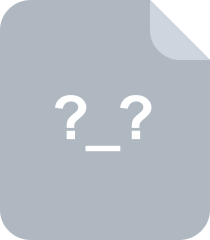
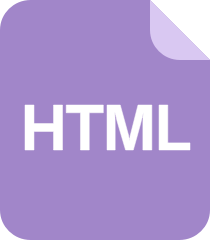
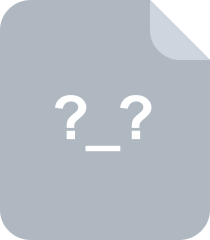
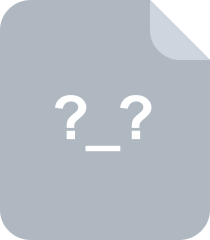
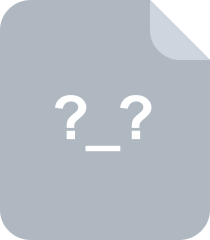
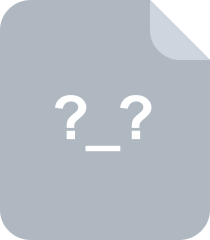
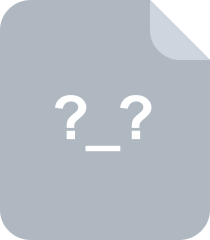
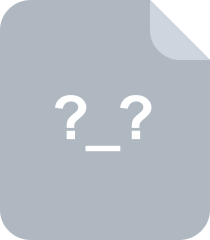
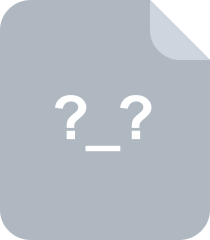
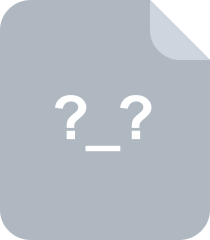
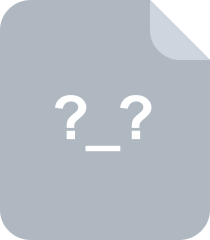
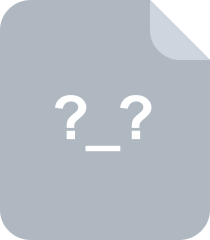
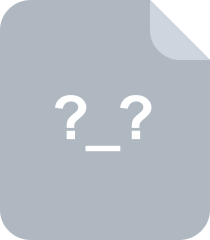
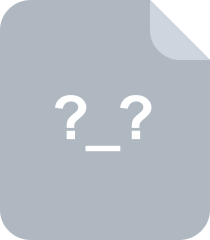
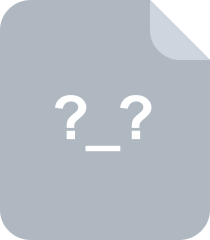
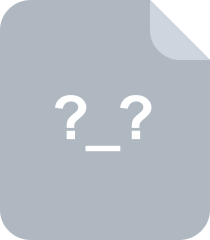
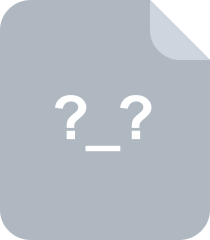
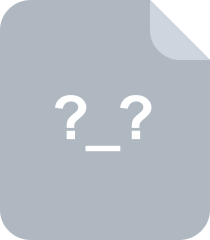
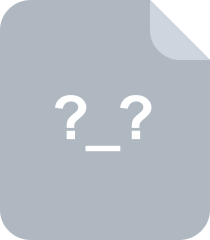
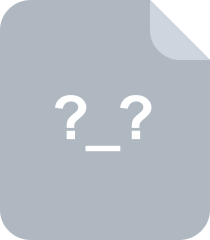
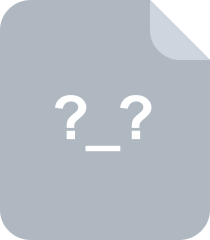
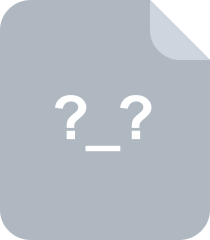
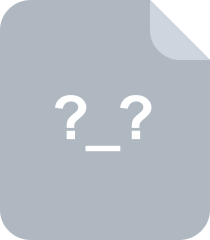
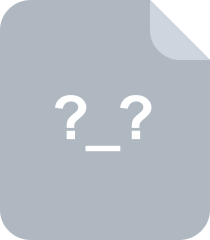
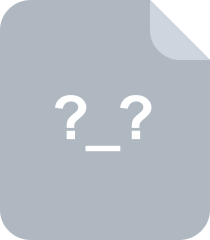
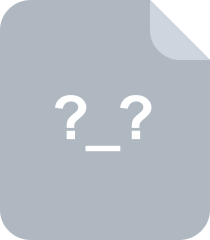
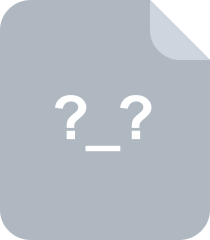
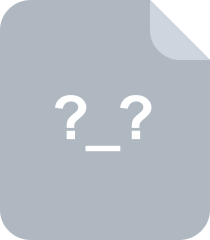
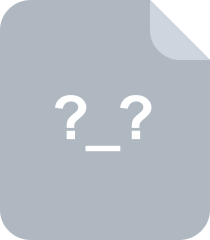
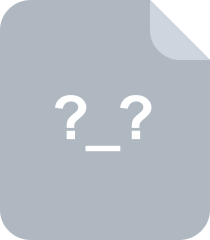
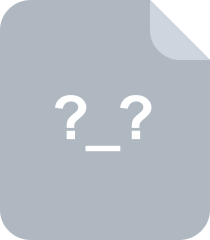
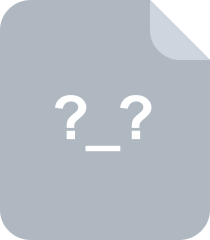
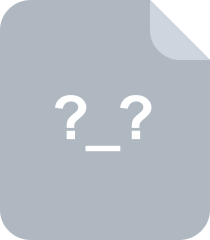
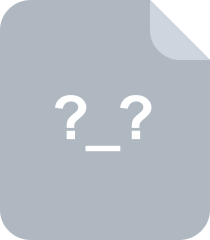
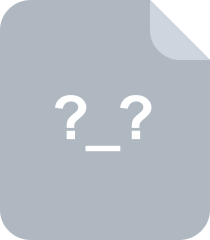
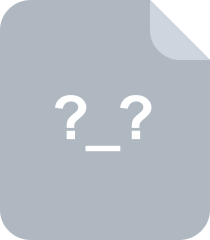
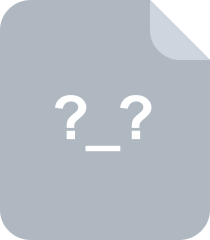
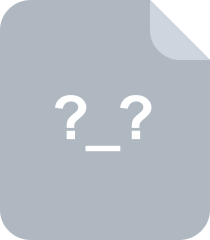
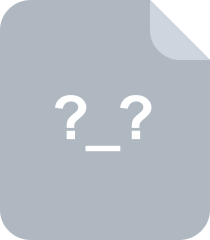
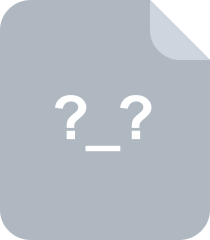
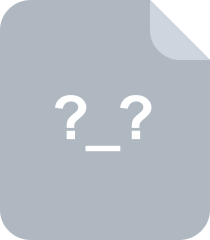
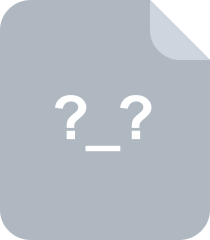
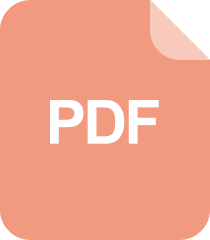
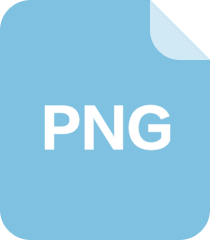
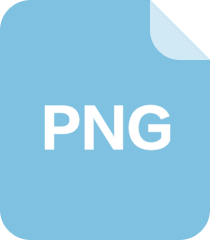
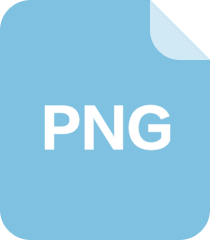
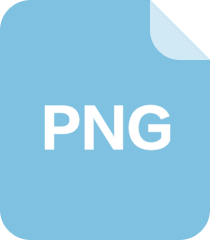
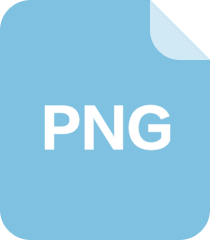
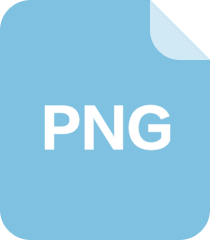
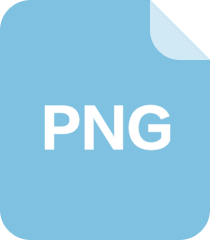
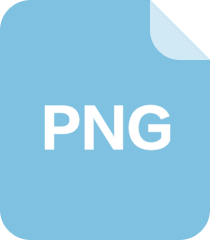
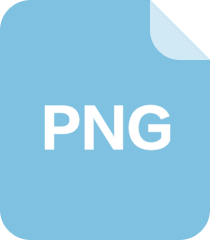
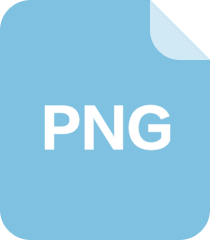
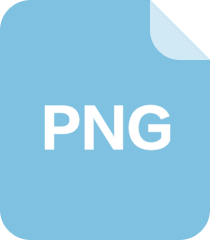
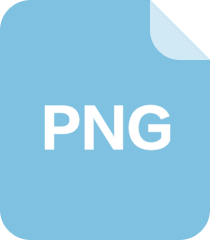
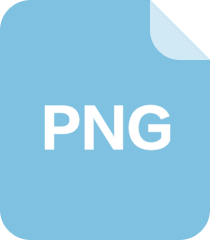
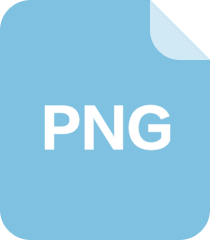
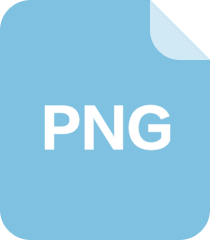
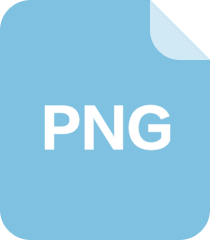
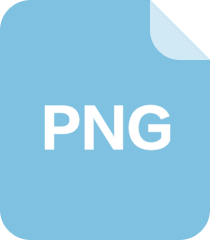
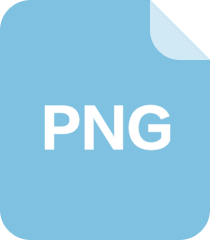
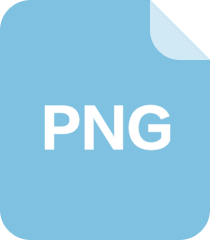
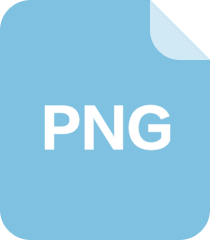
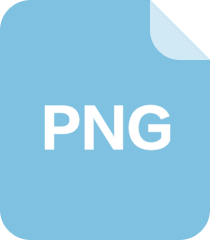
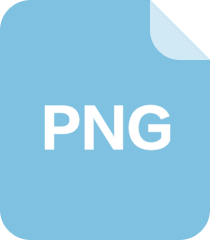
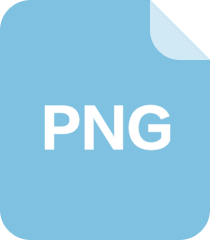
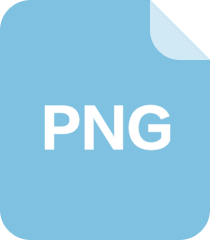
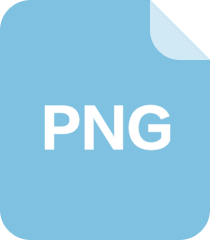
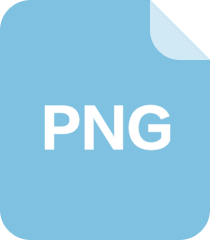
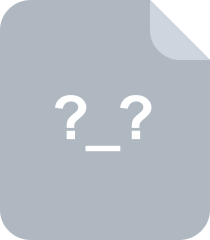
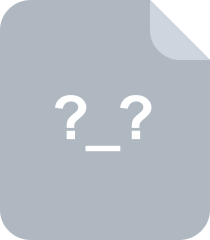
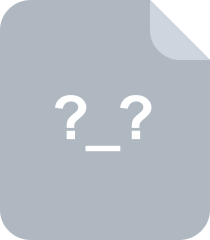
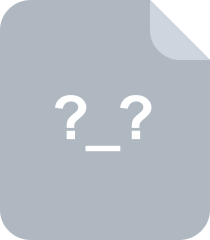
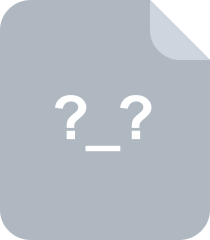
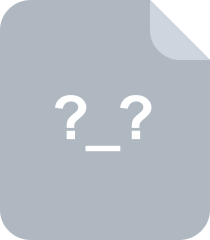
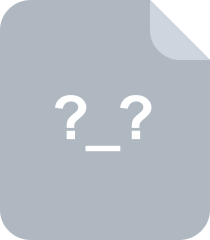
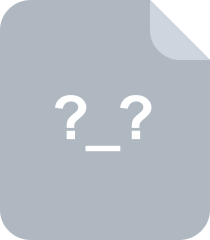
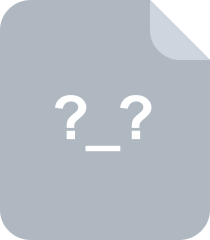
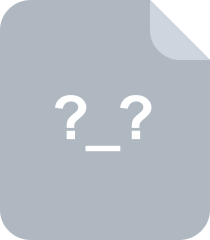
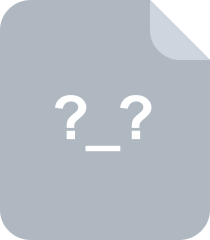
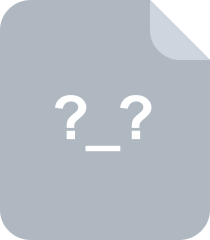
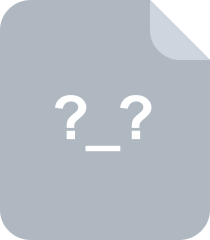
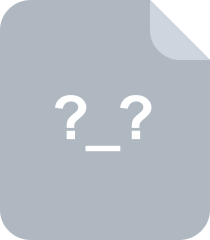
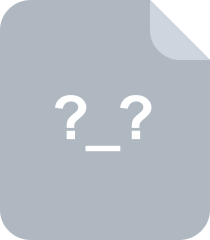
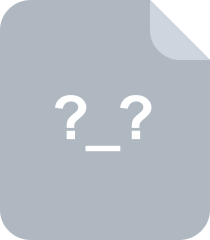
共 1430 条
- 1
- 2
- 3
- 4
- 5
- 6
- 15
资源评论
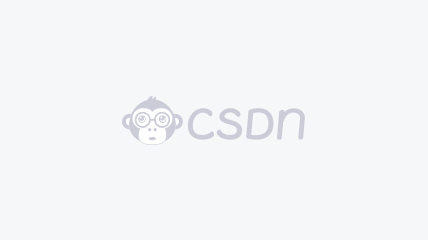
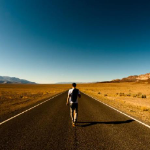
程序员无锋
- 粉丝: 3698
- 资源: 2563

下载权益

C知道特权

VIP文章

课程特权

开通VIP
上传资源 快速赚钱
我的内容管理 展开
我的资源 快来上传第一个资源
我的收益
登录查看自己的收益我的积分 登录查看自己的积分
我的C币 登录后查看C币余额
我的收藏
我的下载
下载帮助

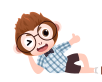
最新资源
- 基于Springboot+Vue的疗养院管理系统的设计与实现-毕业源码案例设计(源码+项目说明+演示视频).zip
- 基于Springboot+Vue的旅游推荐系统设计与实现-毕业源码案例设计(高分毕业设计).zip
- 11种概率分布的拟合与ks检验,可用于概率分析,可靠度计算等领域 案例中提供11种概率分布,具体包括:gev、logistic、gaussian、tLocationScale、Rayleigh、Log
- 基于Springboot+Vue的贸易行业crm系统-毕业源码案例设计(95分以上).zip
- 基于Springboot+Vue的秒杀系统设计与实现-毕业源码案例设计(高分项目).zip
- 西门子1200和三菱FXU通讯程序
- 基于Springboot+Vue的名城小区物业管理系统-毕业源码案例设计(高分毕业设计).zip
- 欧美风格, 节日主题模板
- 基于Springboot+Vue的民族婚纱预定系统的设计与实现-毕业源码案例设计(高分毕业设计).zip
- 基于Springboot+Vue的农商订单跟踪售后交流对接系统-毕业源码案例设计(源码+数据库).zip
- 海面目标检测跟踪数据集.zip
- 基于Springboot+vue的人力资源管理系统-毕业源码案例设计(高分毕业设计).zip
- 基于Springboot+Vue的商业辅助决策系统的设计与实现-毕业源码案例设计(95分以上).zip
- 基于Springboot+Vue的企业资产管理系统-毕业源码案例设计(源码+论文).zip
- 准Z源光伏并网系统MATLAB仿真模型,采用了三次谐波注入法SPWM调制,具有更高的电压利用效率 并网部分采用了电压外环电流内环 电池部分采用了扰动观察法,PO Z源并网和逆变器研究方向的同学可
- 基于Springboot+Vue的实习管理系统-毕业源码案例设计(高分项目).zip
资源上传下载、课程学习等过程中有任何疑问或建议,欢迎提出宝贵意见哦~我们会及时处理!
点击此处反馈


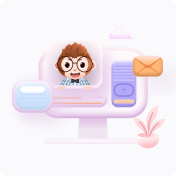
安全验证
文档复制为VIP权益,开通VIP直接复制
