function [model,b,X,Y] = trainlssvm(model,X,Y)
% Train the support values and the bias term of an LS-SVM for classification or function approximation
%
% >> [alpha, b] = trainlssvm({X,Y,type,gam,kernel_par,kernel,preprocess})
% >> model = trainlssvm(model)
%
% type can be 'classifier' or 'function estimation' (these strings
% can be abbreviated into 'c' or 'f', respectively). X and Y are
% matrices holding the training input and output data. The i-th
% data point is represented by the i-th row X(i,:) and Y(i,:). gam
% is the regularization parameter: for gam low minimizing of the
% complexity of the model is emphasized, for gam high, good fitting
% of the training data points is stressed. kernel_par is the
% parameter of the kernel; in the common case of an RBF kernel, a
% large sig2 indicates a stronger smoothing. The kernel_type
% indicates the function that is called to compute the kernel value
% (by default RBF_kernel). Other kernels can be used for example:
%
% >> [alpha, b] = trainlssvm({X,Y,type,gam,[d p],'poly_kernel'})
% >> [alpha, b] = trainlssvm({X,Y,type,gam,[] ,'lin_kernel'})
%
% The kernel parameter(s) are passed as a row vector, in the case
% no kernel parameter is needed, pass the empty vector!
%
% The training can either be proceeded by the preprocessing
% function ('preprocess') (by default) or not ('original'). The
% training calls the preprocessing (prelssvm, postlssvm) and the
% encoder (codelssvm) if appropiate.
%
% In the remainder of the text, the content of the cell determining
% the LS-SVM is given by {X,Y, type, gam, sig2}. However, the
% additional arguments in this cell can always be added in the
% calls.
%
% If one uses the object oriented interface (see also A.3.14), the training is done by
%
% >> model = trainlssvm(model)
% >> model = trainlssvm(model, X, Y)
%
% The status of the model checks whether a retraining is
% needed. The extra arguments X, Y allow to re-initialize the model
% with this new training data as long as its dimensions are the
% same as the old initiation.
%
% The training implementation:
%
% * The Matlab implementation: a straightforward implementation
% based on the matrix division '\' (lssvmMATLAB.m).
%
%
% This implementation allows to train a multidimensional output
% problem. If each output uses the same kernel type, kernel
% parameters and regularization parameter, this is
% straightforward. If not so, one can specify the different types
% and/or parameters as a row vector in the appropriate
% argument. Each dimension will be trained with the corresponding
% column in this vector.
%
% >> [alpha, b] = trainlssvm({X, [Y_1 ... Y_d],type,...
% [gam_1 ... gam_d], ...
% [sig2_1 ... sig2_d],...
% {kernel_1,...,kernel_d}})
%
% Full syntax
%
% 1. Using the functional interface:
%
% >> [alpha, b] = trainlssvm({X,Y,type,gam,sig2})
% >> [alpha, b] = trainlssvm({X,Y,type,gam,sig2,kernel})
% >> [alpha, b] = trainlssvm({X,Y,type,gam,sig2,kernel,preprocess})
%
% Outputs
% alpha : N x m matrix with support values of the LS-SVM
% b : 1 x m vector with bias term(s) of the LS-SVM
% Inputs
% X : N x d matrix with the inputs of the training data
% Y : N x 1 vector with the outputs of the training data
% type : 'function estimation' ('f') or 'classifier' ('c')
% gam : Regularization parameter
% sig2 : Kernel parameter (bandwidth in the case of the 'RBF_kernel')
% kernel(*) : Kernel type (by default 'RBF_kernel')
% preprocess(*) : 'preprocess'(*) or 'original'
%
%
% * Using the object oriented interface:
%
% >> model = trainlssvm(model)
% >> model = trainlssvm({X,Y,type,gam,sig2})
% >> model = trainlssvm({X,Y,type,gam,sig2,kernel})
% >> model = trainlssvm({X,Y,type,gam,sig2,kernel,preprocess})
%
% Outputs
% model : Trained object oriented representation of the LS-SVM model
% Inputs
% model : Object oriented representation of the LS-SVM model
% X(*) : N x d matrix with the inputs of the training data
% Y(*) : N x 1 vector with the outputs of the training data
% type(*) : 'function estimation' ('f') or 'classifier' ('c')
% gam(*) : Regularization parameter
% sig2(*) : Kernel parameter (bandwidth in the case of the 'RBF_kernel')
% kernel(*) : Kernel type (by default 'RBF_kernel')
% preprocess(*) : 'preprocess'(*) or 'original'
%
% See also:
% simlssvm, initlssvm, changelssvm, plotlssvm, prelssvm, codelssvm
% Copyright (c) 2011, KULeuven-ESAT-SCD, License & help @ http://www.esat.kuleuven.be/sista/lssvmlab
%
% initialise the model 'model'
%
if (iscell(model)),
model = initlssvm(model{:});
end
%
% given X and Y?
%
%model = codelssvm(model);
eval('model = changelssvm(model,''xtrain'',X);',';');
eval('model = changelssvm(model,''ytrain'',Y);',';');
eval('model = changelssvm(model,''selector'',1:size(X,1));',';');
%
% no training needed if status = 'trained'
%
if model.status(1) == 't',
if (nargout>1),
% [alpha,b]
X = model.xtrain;
Y = model.ytrain;
b = model.b;
model = model.alpha;
end
return
end
%
% control of the inputs
%
if ~((strcmp(model.kernel_type,'RBF_kernel') && length(model.kernel_pars)>=1) ||...
(strcmp(model.kernel_type,'lin_kernel') && length(model.kernel_pars)>=0) ||...
(strcmp(model.kernel_type,'MLP_kernel') && length(model.kernel_pars)>=2) ||...
(strcmp(model.kernel_type,'poly_kernel')&& length(model.kernel_pars)>=1)),
% eval('feval(model.kernel_type,model.xtrain(1,:),model.xtrain(2,:),model.kernel_pars);model.implementation=''MATLAB'';',...
% 'error(''The kernel type is not valid or to few arguments'');');
elseif (model.steps<=0),
error('steps must be larger then 0');
elseif (model.gam<=0),
error('gamma must be larger then 0');
% elseif (model.kernel_pars<=0),
% error('sig2 must be larger then 0');
elseif or(model.x_dim<=0, model.y_dim<=0),
error('dimension of datapoints must be larger than 0');
end
%
% coding if needed
%
if model.code(1) == 'c', % changed
model = codelssvm(model);
end
%
% preprocess
%
eval('if model.prestatus(1)==''c'', changed=1; else changed=0;end;','changed=0;');
if model.preprocess(1) =='p' && changed,
model = prelssvm(model);
elseif model.preprocess(1) =='o' && changed
model = postlssvm(model);
end
% clock
tic;
%
% set & control input variables and dimensions
%
if (model.type(1) == 'f'), % function
dyn_pars=[];
elseif (model.type(1) == 'c'), % class
dyn_pars=[];
end
% only MATLAB
if size(model.gam,1)>1,
model.implementation='MATLAB';
end
%
% output dimension > 1...recursive call on each dimension
%
if model.y_dim>1,
if (length(model.kernel_pars)==model.y_dim || size(model.gam,2)==model.y_dim || numel(model.kernel_type,2)==model.y_dim)
disp('multidimensional output...');
model = trainmultidimoutput(model);
%
% wich output is wanted?
%
if (nargout>1),
X = model.xtrain;
Y = model.ytrain;
b = model.b;
model = model.alpha;
else
model.duration = toc;
model.status = 'trained';
end
return
end
end
%
% call lssvmMATLAB.m
%
model = lssvmMATLAB(model);
%
% wich output is wanted?
%
if (nargout>1),
X = model.xtrain;
Y = model.ytrain;
b = model.b;
model = model.alpha;
else
model.duration = toc;
model.status = 'trained';
end
function model = trainmultidimoutput(model)
model.alpha = zeros(model.nb_data, model.y_dim);
model.b = zeros(1,model.y_dim);
for d=1:model.y_dim,
eval('gam = model.gam(:,d);','gam = model.gam(:);');
eval('sig2 = model.kerne
没有合适的资源?快使用搜索试试~ 我知道了~
温馨提示
Matlab实现SVM、MLP、LSSVM和 AdaBoost 模式识别(完整源码) 我们选择了分类任务中四种重要的机器学习算法,在 MATLAB 软件中实现了这些算法。 Matlab实现SVM、MLP、LSSVM和 AdaBoost 模式识别(完整源码) 我们选择了分类任务中四种重要的机器学习算法,在 MATLAB 软件中实现了这些算法。 Matlab实现SVM、MLP、LSSVM和 AdaBoost 模式识别(完整源码) 我们选择了分类任务中四种重要的机器学习算法,在 MATLAB 软件中实现了这些算法。 Matlab实现SVM、MLP、LSSVM和 AdaBoost 模式识别(完整源码) 我们选择了分类任务中四种重要的机器学习算法,在 MATLAB 软件中实现了这些算法。
资源推荐
资源详情
资源评论
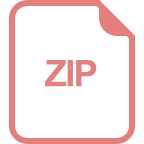
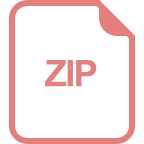
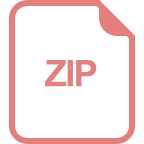
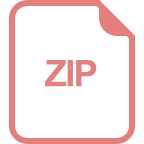
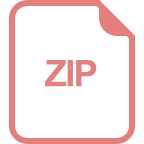
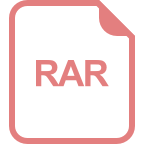
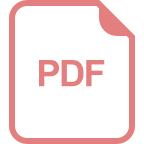
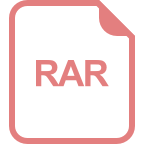
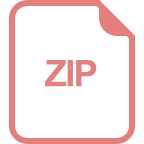
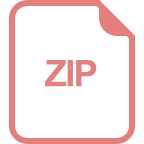
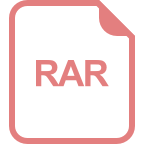
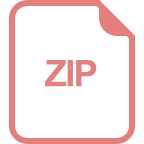
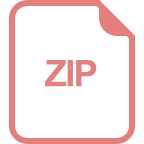
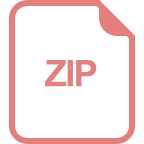
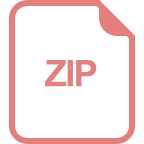
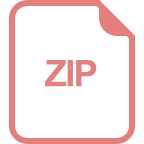
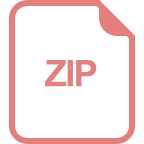
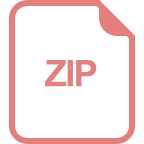
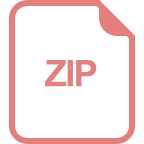
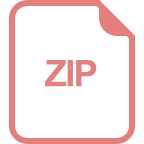
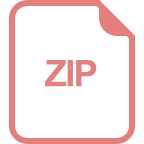
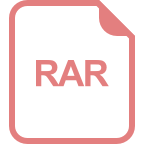
收起资源包目录

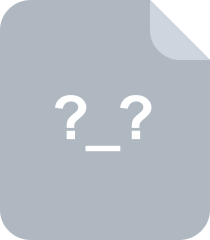
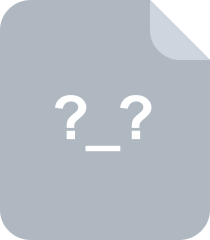
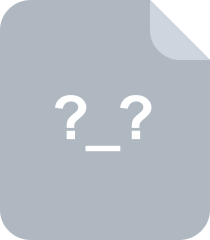
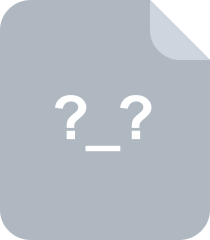
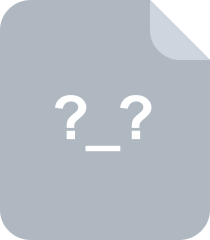
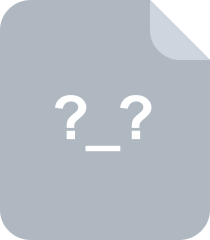
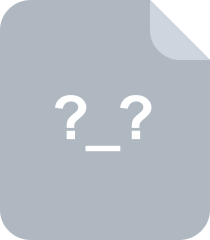
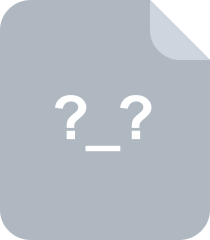
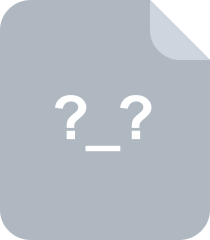
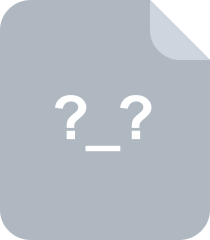
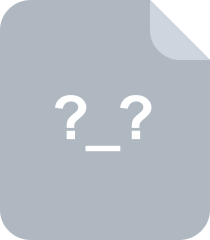
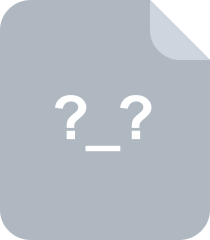
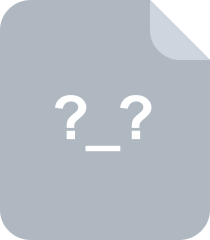
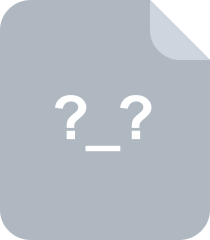
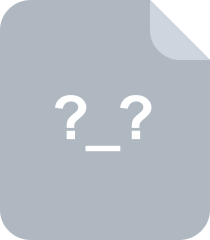
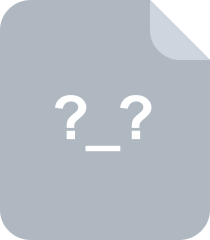
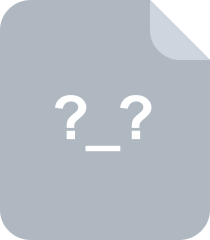
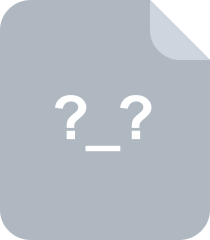
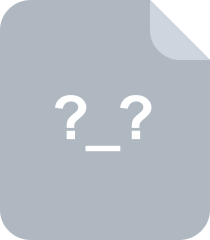
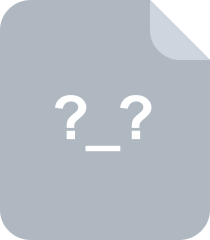
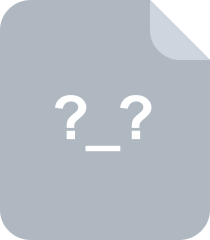
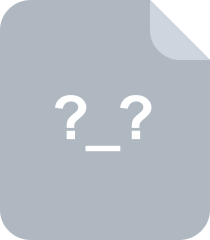
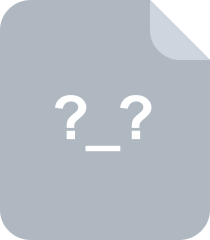
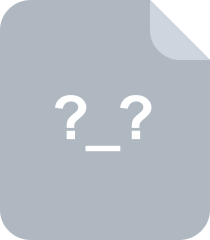
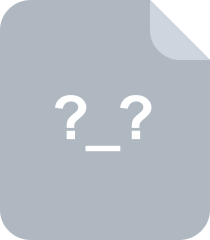
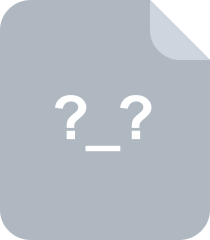
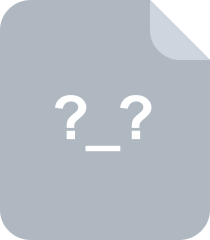
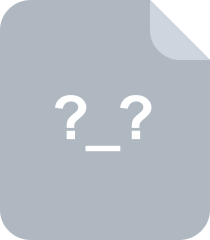
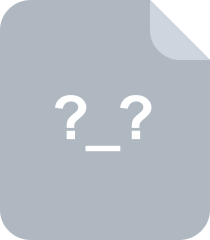
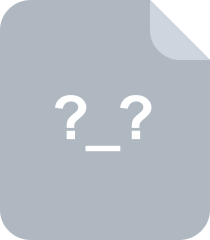
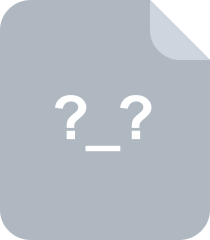
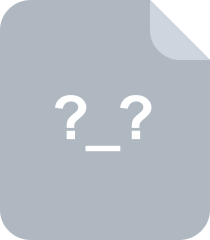
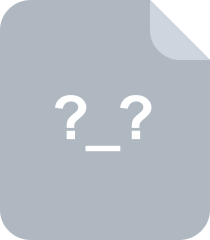
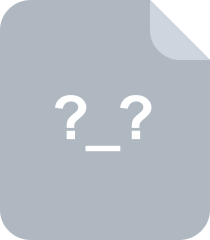
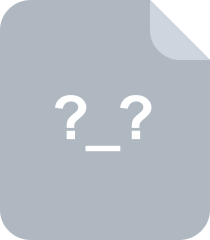
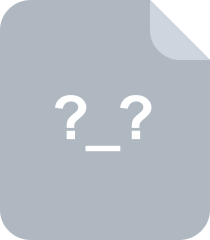
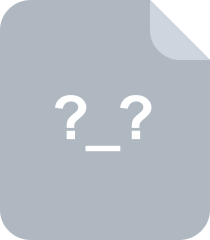
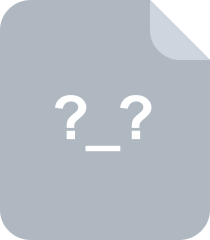
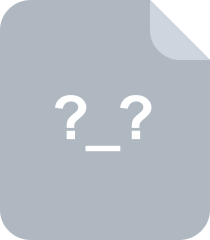
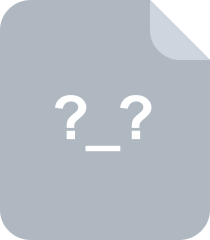
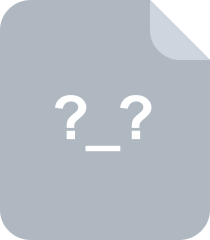
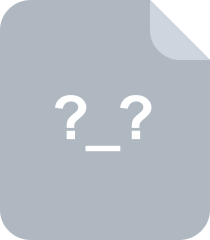
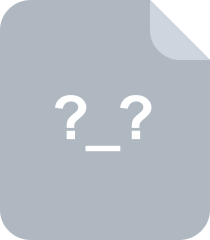
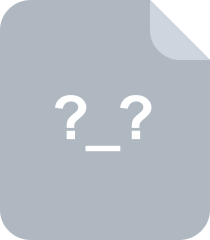
共 44 条
- 1
资源评论
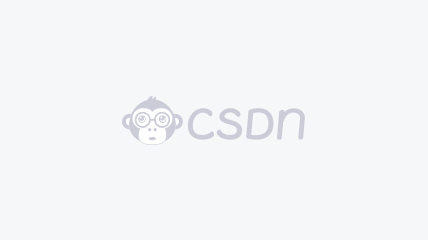

机器学习之心
- 粉丝: 2w+
- 资源: 1033
上传资源 快速赚钱
我的内容管理 展开
我的资源 快来上传第一个资源
我的收益
登录查看自己的收益我的积分 登录查看自己的积分
我的C币 登录后查看C币余额
我的收藏
我的下载
下载帮助

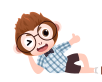
最新资源
资源上传下载、课程学习等过程中有任何疑问或建议,欢迎提出宝贵意见哦~我们会及时处理!
点击此处反馈


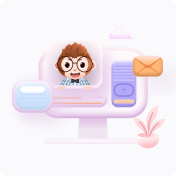
安全验证
文档复制为VIP权益,开通VIP直接复制
