
# Fast Segment Anything
[[`ðPaper`](https://arxiv.org/pdf/2306.12156.pdf)] [[`ð¤HuggingFace Demo`](https://huggingface.co/spaces/An-619/FastSAM)] [[`Colab demo`](https://colab.research.google.com/drive/1oX14f6IneGGw612WgVlAiy91UHwFAvr9?usp=sharing)] [[`Replicate demo & API`](https://replicate.com/casia-iva-lab/fastsam)] [~~[`OpenXLab Demo`](https://openxlab.org.cn/apps/detail/zxair/FastSAM)~~] [[`Model Zoo`](#model-checkpoints)] [[`BibTeX`](#citing-fastsam)] [[`Video Demo`](https://youtu.be/yHNPyqazYYU)]

The **Fast Segment Anything Model(FastSAM)** is a CNN Segment Anything Model trained using only 2% of the SA-1B dataset published by SAM authors. FastSAM achieves comparable performance with
the SAM method at **50Ã higher run-time speed**.

**ð Updates**
- **`2023/11/28`** Recommendation: [Semantic FastSAM](https://github.com/KBH00/Semantic-Fast-SAM), which add the semantic class labels to FastSAM. Thanks to [KBH00](https://github.com/KBH00/Semantic-Fast-SAM) for this valuable contribution.
- **`2023/09/11`** Release [Training and Validation Code](https://github.com/CASIA-IVA-Lab/FastSAM/releases).
- **`2023/08/17`** Release [OpenXLab Demo](https://openxlab.org.cn/apps/detail/zxair/FastSAM). Thanks to OpenXLab Team for help.
- **`2023/07/06`** Added to [Ultralytics (YOLOv8) Model Hub](https://docs.ultralytics.com/models/fast-sam/). Thanks to [Ultralytics](https://github.com/ultralytics/ultralytics) for help ð¹.
- **`2023/06/29`** Support [text mode](https://huggingface.co/spaces/An-619/FastSAM) in HuggingFace Space. Thanks a lot to [gaoxinge](https://github.com/gaoxinge) for help ð¹.
- **`2023/06/29`** Release [FastSAM_Awesome_TensorRT](https://github.com/ChuRuaNh0/FastSam_Awsome_TensorRT). Thanks a lot to [ChuRuaNh0](https://github.com/ChuRuaNh0) for providing the TensorRT model of FastSAM ð¹.
- **`2023/06/26`** Release [FastSAM Replicate Online Demo](https://replicate.com/casia-iva-lab/fastsam). Thanks a lot to [Chenxi](https://chenxwh.github.io/) for providing this nice demo ð¹.
- **`2023/06/26`** Support [points mode](https://huggingface.co/spaces/An-619/FastSAM) in HuggingFace Space. Better and faster interaction will come soon!
- **`2023/06/24`** Thanks a lot to [Grounding-SAM](https://github.com/IDEA-Research/Grounded-Segment-Anything) for Combining Grounding-DINO with FastSAM in [Grounded-FastSAM](https://github.com/IDEA-Research/Grounded-Segment-Anything/tree/main/EfficientSAM) ð¹.
## Installation
Clone the repository locally:
```shell
git clone https://github.com/CASIA-IVA-Lab/FastSAM.git
```
Create the conda env. The code requires `python>=3.7`, as well as `pytorch>=1.7` and `torchvision>=0.8`. Please follow the instructions [here](https://pytorch.org/get-started/locally/) to install both PyTorch and TorchVision dependencies. Installing both PyTorch and TorchVision with CUDA support is strongly recommended.
```shell
conda create -n FastSAM python=3.9
conda activate FastSAM
```
Install the packages:
```shell
cd FastSAM
pip install -r requirements.txt
```
Install CLIP:
```shell
pip install git+https://github.com/openai/CLIP.git
```
## <a name="GettingStarted"></a> Getting Started
First download a [model checkpoint](#model-checkpoints).
Then, you can run the scripts to try the everything mode and three prompt modes.
```shell
# Everything mode
python Inference.py --model_path ./weights/FastSAM.pt --img_path ./images/dogs.jpg
```
```shell
# Text prompt
python Inference.py --model_path ./weights/FastSAM.pt --img_path ./images/dogs.jpg --text_prompt "the yellow dog"
```
```shell
# Box prompt (xywh)
python Inference.py --model_path ./weights/FastSAM.pt --img_path ./images/dogs.jpg --box_prompt "[[570,200,230,400]]"
```
```shell
# Points prompt
python Inference.py --model_path ./weights/FastSAM.pt --img_path ./images/dogs.jpg --point_prompt "[[520,360],[620,300]]" --point_label "[1,0]"
```
You can use the following code to generate all masks, make mask selection based on prompts, and visualize the results.
```shell
from fastsam import FastSAM, FastSAMPrompt
model = FastSAM('./weights/FastSAM.pt')
IMAGE_PATH = './images/dogs.jpg'
DEVICE = 'cpu'
everything_results = model(IMAGE_PATH, device=DEVICE, retina_masks=True, imgsz=1024, conf=0.4, iou=0.9,)
prompt_process = FastSAMPrompt(IMAGE_PATH, everything_results, device=DEVICE)
# everything prompt
ann = prompt_process.everything_prompt()
# bbox default shape [0,0,0,0] -> [x1,y1,x2,y2]
ann = prompt_process.box_prompt(bboxes=[[200, 200, 300, 300]])
# text prompt
ann = prompt_process.text_prompt(text='a photo of a dog')
# point prompt
# points default [[0,0]] [[x1,y1],[x2,y2]]
# point_label default [0] [1,0] 0:background, 1:foreground
ann = prompt_process.point_prompt(points=[[620, 360]], pointlabel=[1])
prompt_process.plot(annotations=ann,output_path='./output/dog.jpg',)
```
You are also welcomed to try our Colab demo: [FastSAM_example.ipynb](https://colab.research.google.com/drive/1oX14f6IneGGw612WgVlAiy91UHwFAvr9?usp=sharing).
## Different Inference Options
We provide various options for different purposes, details are in [MORE_USAGES.md](MORE_USAGES.md).
## Training or Validation
Training from scratch or validation: [Training and Validation Code](https://github.com/CASIA-IVA-Lab/FastSAM/releases).
## Web demo
### Gradio demo
- We also provide a UI for testing our method that is built with gradio. You can upload a custom image, select the mode and set the parameters, click the segment button, and get a satisfactory segmentation result. Currently, the UI supports interaction with the 'Everything mode' and 'points mode'. We plan to add support for additional modes in the future. Running the following command in a terminal will launch the demo:
```
# Download the pre-trained model in "./weights/FastSAM.pt"
python app_gradio.py
```
- This demo is also hosted on [HuggingFace Space](https://huggingface.co/spaces/An-619/FastSAM).
 
### Replicate demo
- [Replicate demo](https://replicate.com/casia-iva-lab/fastsam) has supported all modes, you can experience points/box/text mode.
  
## <a name="Models"></a>Model Checkpoints
Two model versions of the model are available with different sizes. Click the links below to download the checkpoint for the corresponding model type.
- **`default` or `FastSAM`: [YOLOv8x based Segment Anything Model](https://drive.google.com/file/d/1m1sjY4ihXBU1fZXdQ-Xdj-mDltW-2Rqv/view?usp=sharing) | [Baidu Cloud (pwd: 0000).](https://pan.baidu.com/s/18KzBmOTENjByoWWR17zdiQ?pwd=0000)**
- `FastSAM-s`: [YOLOv8s based Segment Anything Model.](https://drive.google.com/file/d/10XmSj6mmpmRb8NhXbtiuO9cTTBwR_9SV/view?usp=sharing)
## Results
All result were tested on a single NVIDIA GeForce RTX 3090.
### 1. Inference time
Running Speed under Different Point Prompt Numbers(ms).
| method | params | 1 | 10 | 100 | E(16x16) | E(32x32\*) | E(64x64) |
|:------------------:|:--------:|:-----:|:-----:|:-----:|:----------:|:-----------:|:----------:|
| SAM-H | 0.6G | 446 | 464 | 627 | 852 | 2099 | 6972 |
| SAM-B | 136M | 110 | 125 | 230 | 432 | 1383 | 5417 |
| FastSAM | 68M | 40 |40 | 40 | 40 | 40 | 40 |
### 2. Memory usage
| Dataset | Method | GPU Memory (MB) |
| :-------: | :-----: | :-------------: |
| COCO 2017 | FastSAM | 2608 |
| COCO 2017 | SAM-H | 7060 |
| COCO 2017 | SAM-B | 4670 |
### 3. Zero-shot Transfer Experiments
#### Edge Detection
Test on the BSDB500 dataset.
|method | year| ODS | OIS | AP | R50 |
|:----------:|:
没有合适的资源?快使用搜索试试~ 我知道了~
快速目标分割:FastSAM
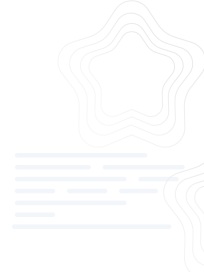
共53个文件
png:19个
py:14个
jpg:13个

1.该资源内容由用户上传,如若侵权请联系客服进行举报
2.虚拟产品一经售出概不退款(资源遇到问题,请及时私信上传者)
2.虚拟产品一经售出概不退款(资源遇到问题,请及时私信上传者)
版权申诉
0 下载量 106 浏览量
2024-02-05
16:29:01
上传
评论
收藏 39.52MB ZIP 举报
温馨提示
一个用于快速分割任何对象的项目。它提供了一种高效的算法和方法,能够在图像和视频中准确地进行目标分割,为计算机视觉和图像处理领域的研究人员和开发人员提供了有力的工具。
资源推荐
资源详情
资源评论
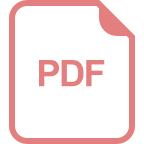
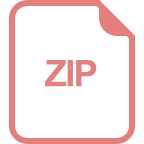
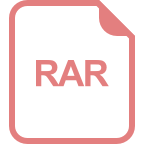
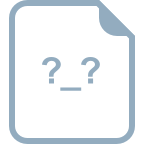
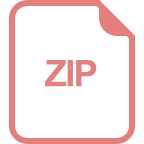
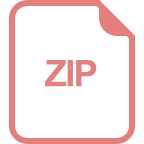
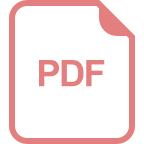
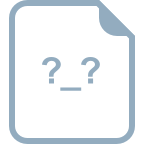
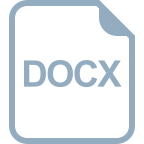
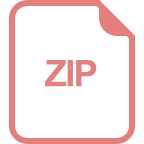
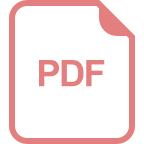
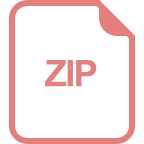
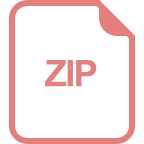
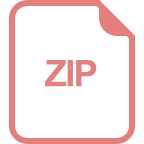
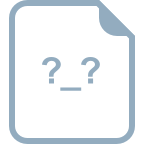
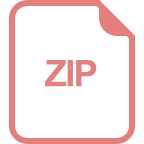
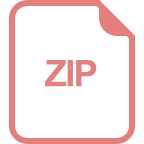
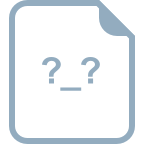
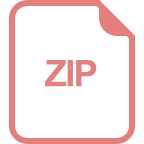
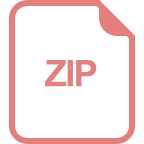
收起资源包目录


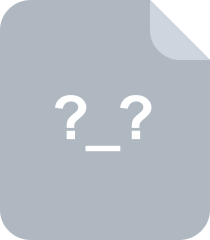
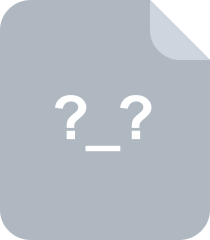
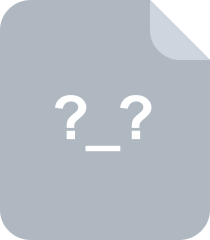

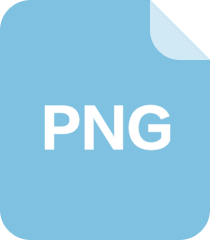
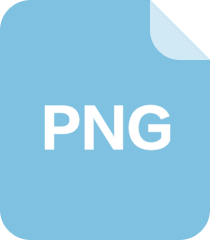

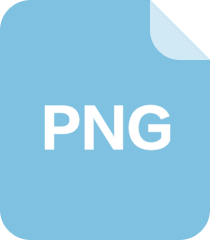
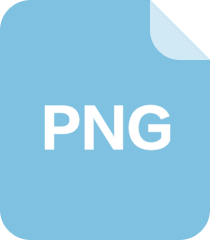
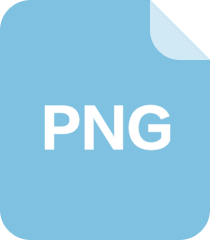
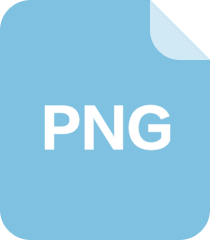
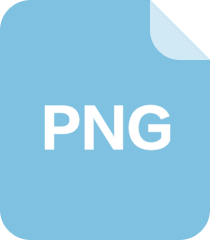
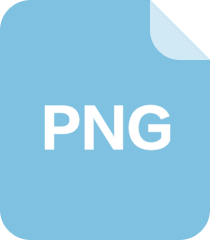
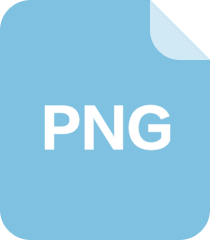
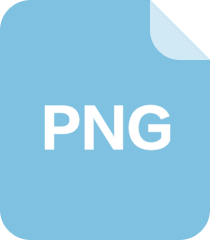
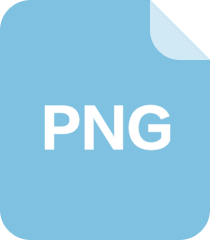
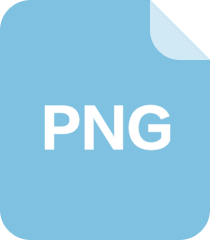
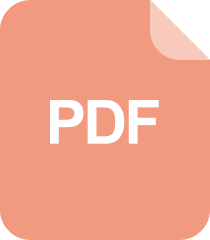
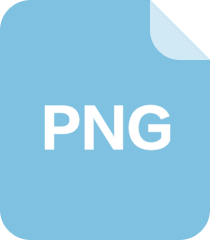
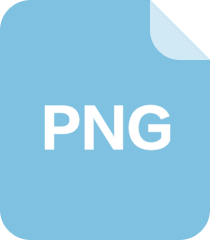
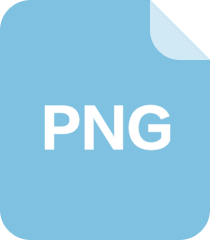
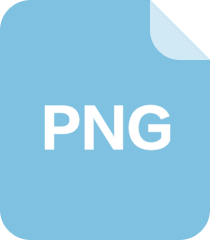
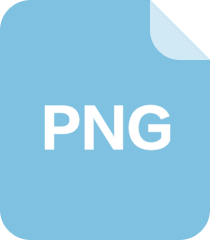
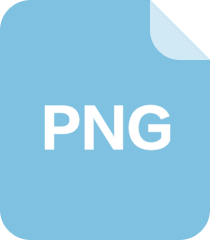
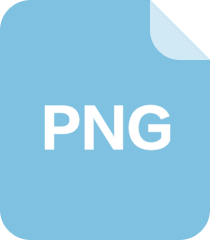
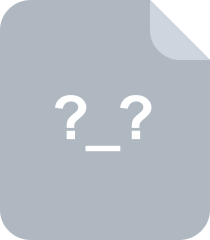
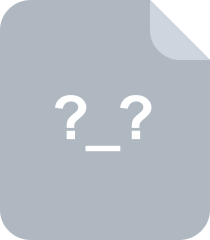
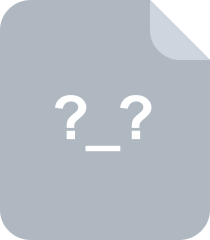

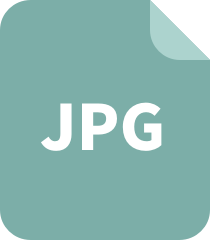
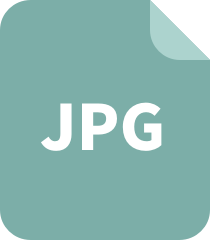
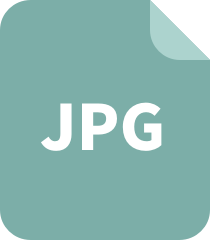
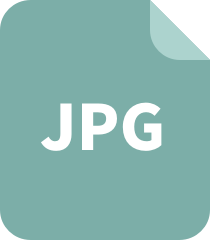
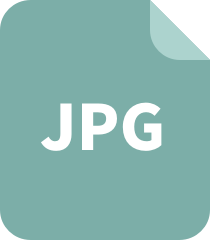
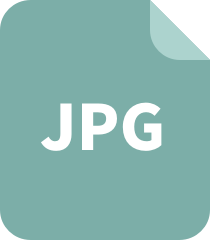
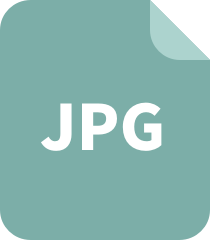
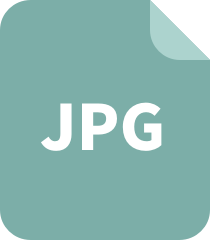
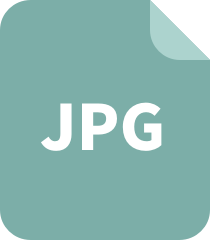

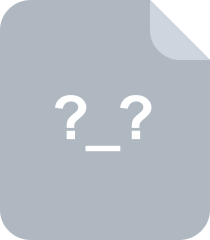
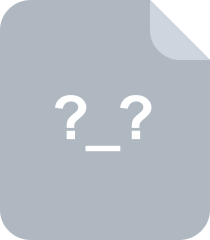
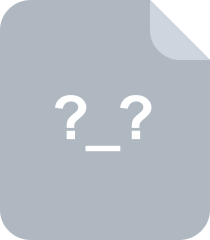

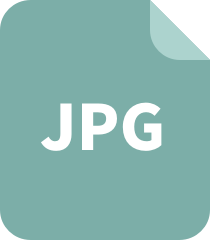
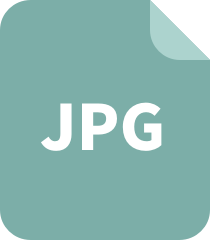
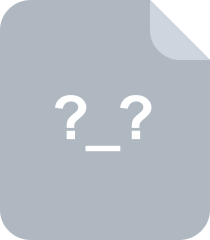

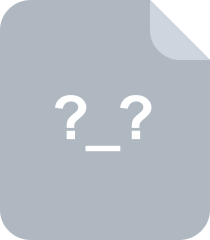
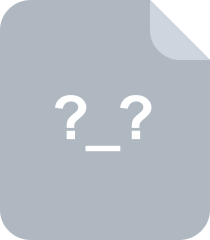
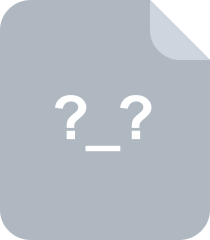
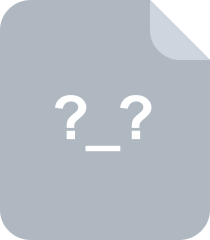
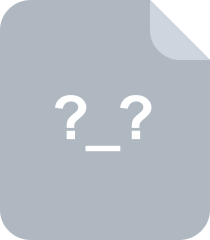
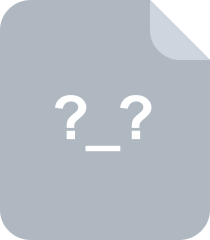
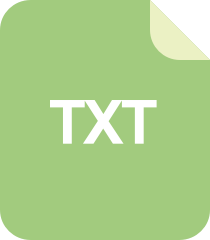
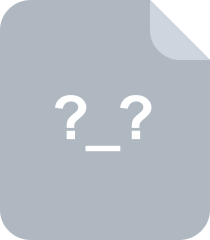
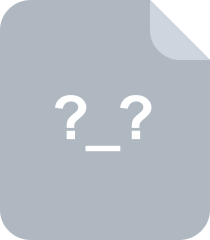

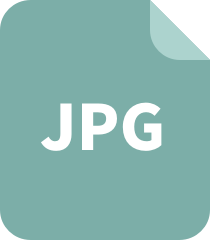
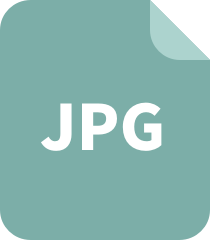
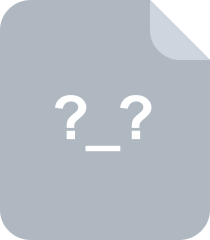
共 53 条
- 1
资源评论
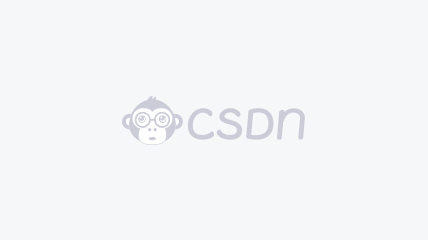

UnknownToKnown
- 粉丝: 1w+
- 资源: 627
上传资源 快速赚钱
我的内容管理 展开
我的资源 快来上传第一个资源
我的收益
登录查看自己的收益我的积分 登录查看自己的积分
我的C币 登录后查看C币余额
我的收藏
我的下载
下载帮助

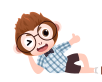
最新资源
- 实验1.1 访问控制列表.topo
- 办公效率:PDF-图片互转工具
- 一天的风格化规范化当时法国是
- 编译原理:正规式转NFA(有穷自动机)
- 通过 Navicat 备份文件读取连接信息和解析密码
- Linux下开箱即用的C++单元测试demo示例,public成员函数单元测试
- 连接Redis服务器 在使用Redis之前,首先需要使用redis-cli工具连接到Redis服务器 redis-cli是Re
- 连接Redis服务器 在使用Redis之前,首先需要使用redis-cli工具连接到Redis服务器 redis-cli是Red
- 连接Redis服务器 在使用Redis之前,首先需要使用redis-cli工具连接到Redis服务器 redis-cli是Red
- redis命令实践 详细教程
资源上传下载、课程学习等过程中有任何疑问或建议,欢迎提出宝贵意见哦~我们会及时处理!
点击此处反馈


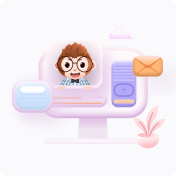
安全验证
文档复制为VIP权益,开通VIP直接复制
