《核卷积神经网络研究与应用》这篇论文深入探讨了如何结合核函数的非线性映射优势与卷积神经网络(CNN)的特性,构建一个新的网络学习模型——核卷积神经网络(Kernel-CNN)。该模型旨在提高数据特征提取的能力,并在实际问题中实现更高效的分类效果。 在传统卷积神经网络的基础上,核卷积神经网络引入了核函数的概念,这允许网络在卷积过程中进行非线性变换。非线性映射是解决复杂数据模式的关键,因为它能够捕获数据中的高级抽象特征。通过这种方式,Kernel-CNN模型能够增强对数据的理解和表征,尤其是在处理高维度和非线性数据时,它的性能表现更佳。 论文中提到的实验部分,Kernel-CNN模型在MNIST手写数字数据库和MIT-BIH心律失常数据库上的分类准确率分别达到了98.5%和97%,这些结果显著优于传统的卷积神经网络和支持向量机(SVM)。这表明,Kernel-CNN在保持较高识别精度的同时,其损失值(LOSS)相对较小,这意味着它在训练过程中对数据的拟合程度更高,泛化能力更强。 MNIST手写数字库是一个广泛用于测试图像识别算法的标准数据集,而MIT-BIH心律失常数据库则是一个心脏健康监测数据集,用于评估模型在复杂生理信号分析中的性能。在这两个数据集上取得的成功,证明了Kernel-CNN在图像识别和生物医学信号处理等领域的潜力。 Kernel-CNN的工作流程包括数据预处理、特征提取和分类三个主要步骤。数据预处理是为了消除噪声并标准化输入数据,使其更适合模型的训练。接着,核卷积神经网络进行特征提取,通过核函数将原始数据转换到高维空间,从而揭示潜在的结构和模式。采用softmax分类器对提取出的特征进行分类,以得出最终的预测结果。 论文的贡献在于提出了一种新的深度学习模型,该模型结合了核函数的非线性映射和CNN的卷积特性,提升了模型在复杂任务上的性能。此外,通过实验证明了Kernel-CNN在处理非线性问题时的优越性,为机器学习和深度学习领域的研究提供了有价值的参考。 《核卷积神经网络研究与应用》这篇论文深入探讨了如何利用核函数提升卷积神经网络的性能,尤其是在特征提取和分类任务上,为未来的研究提供了新的思路和可能。这一工作不仅在理论上有重要价值,而且在实际应用中也展现出巨大的潜力,特别是在图像识别和生物医学信号分析等领域。
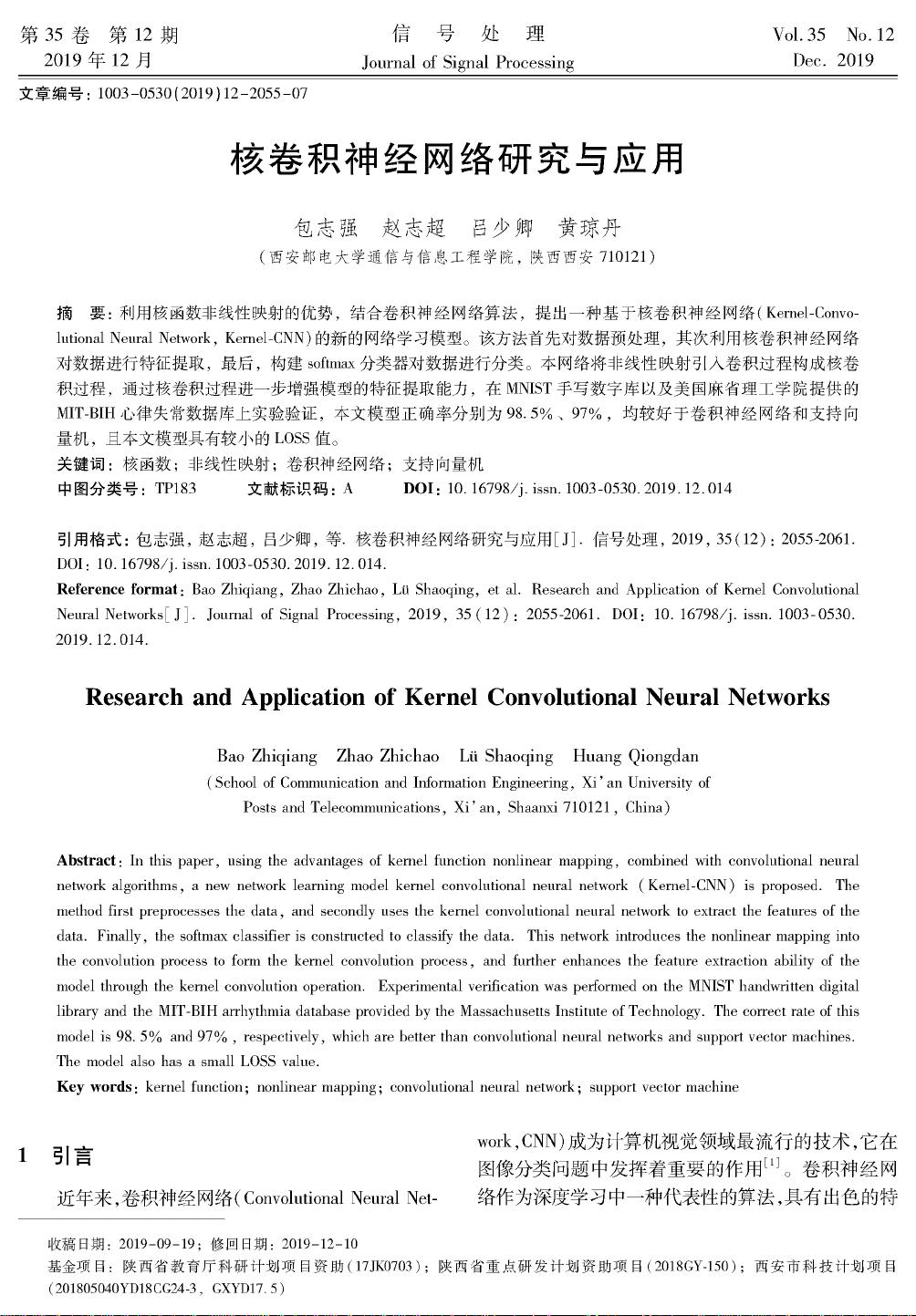
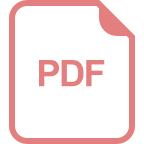
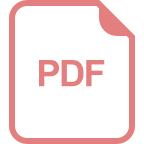
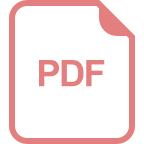
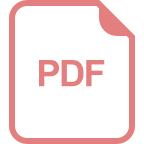
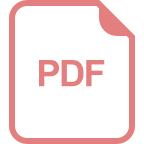
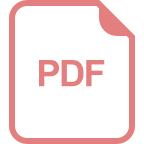
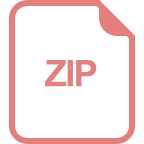
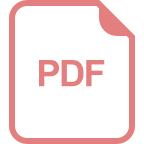
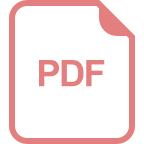
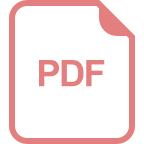
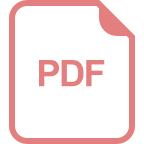
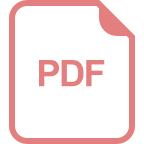
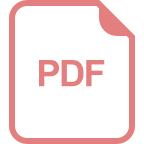
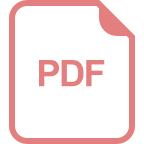
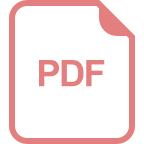

- 粉丝: 136
- 资源: 23万+
我的内容管理 展开
我的资源 快来上传第一个资源
我的收益
登录查看自己的收益我的积分 登录查看自己的积分
我的C币 登录后查看C币余额
我的收藏
我的下载
下载帮助

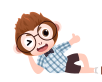
最新资源
- 基于Java和Vue的kopsoftKANBAN车间电子看板设计源码
- 影驰战将PS3111 东芝芯片TT18G23AIN开卡成功分享,图片里面画线的选项很重要
- 【C++初级程序设计·配套源码】第1期-语法基础
- 基于JavaScript、CSS、HTML的简易DOM版飞机游戏设计源码
- 基于Java开发的日程管理FlexTime应用设计源码
- SM2258XT-BGA144-4BGA180-6L-R1019 三星KLUCG4J1CB B0B1颗粒开盘工具 , EC, 3A, 94, 43, A4, CA 七彩虹SL300这个固件有用
- GJB 5236-2004 军用软件质量度量
- 30天开发操作系统 第 8 天 - 鼠标控制与切换32模式
- spice vd interface接口
- 安装Git时遇到找不到`/dev/null`的问题

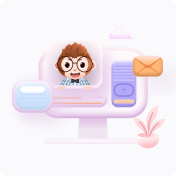

评论0