function [kernel_c1,kernel_c2,weight_c2,weight_full_1,weight_full_2,weight_arr2num]=CNN_upweight(Error,train_data,state_c1,state_s1,state_c2,state_s2_temp1,state_s2_temp2,state_s2,...
state_f1,state_f2,kernel_c1,kernel_c2,weight_c2,weight_full_1,weight_full_2,weight_arr2num,yita,state_c2_temp1)
%%% 完成参数更新,权值和卷积核
%% 结点数目
layer_f2_num=size(state_f2,2);
layer_f1_num=size(state_f1,2);
layer_c2_num=size(state_c2,3);
layer_c1_num=size(state_c1,3);
[c2_row,c2_col,~]=size(state_c2);
[c1_row,c1_col,~]=size(state_c1);
[s2_row,s2_col,~]=size(state_s2_temp1);
[kernel_row,kernel_col]=size(kernel_c1(:,:,1));
%% 保存网络权值
weight_full_2_temp=weight_full_2;
weight_full_1_temp=weight_full_1;
weight_arr2num_temp=weight_arr2num;
kernel_c2_temp=kernel_c2;
kernel_c1_temp=kernel_c1;
weight_c2_temp=weight_c2;
%% 更新weight_full_2
for n=1:layer_f2_num
delta_weight_full_2_temp(:,n)=2*Error(1,n)*state_f1';
end
size(delta_weight_full_2_temp);
weight_full_2_temp=weight_full_2_temp-yita*delta_weight_full_2_temp;
%% 更新weight_full_1
for n=1:layer_f2_num
for m=1:layer_f1_num
delta_weight_full_1_temp(:,m)=2*Error(1,n)*weight_full_2(m,n)*state_s2';
end
weight_full_1_temp=weight_full_1_temp-yita*delta_weight_full_1_temp;
end
%% 更新weight_arr2num
for m=1:layer_c2_num
for n=1:layer_f2_num
count_delta_weight_arr2num_temp=zeros(s2_row,s2_col);
for k=1:layer_f1_num
delta_weight_arr2num_temp(:,:,m)=2*Error(1,n)*weight_full_1(m,k)*weight_full_2(k,n)*exp(-state_s2_temp2(1,m))/(1+exp(-state_s2_temp2(1,m))).^2*state_s2_temp1(:,:,m);
count_delta_weight_arr2num_temp=count_delta_weight_arr2num_temp+delta_weight_arr2num_temp(:,:,m);
end
weight_arr2num_temp(:,:,m)=weight_arr2num_temp(:,:,m)-yita*count_delta_weight_arr2num_temp;
end
end
%% 更新kernel_c2
for m=1:layer_c2_num
for n=1:layer_f2_num
count_delta_state_s2_temp1=zeros(s2_row,s2_col);
for k=1:layer_f1_num
delta_state_s2_temp1(:,:,m)=2*Error(1,n)*weight_full_1(m,k)*weight_full_2(k,n)*exp(-state_s2_temp2(1,m))/(1+exp(-state_s2_temp2(1,m))).^2*weight_arr2num(:,:,m);
count_delta_state_s2_temp1=count_delta_state_s2_temp1+delta_state_s2_temp1(:,:,m);
end
delta_state_c2=kron(count_delta_state_s2_temp1,ones(2,2)/4);
count=zeros(kernel_row,kernel_col);
for k_row=1:c2_row
for k_col=1:c2_col
count=count+state_c2_temp1(k_row:k_row+kernel_row-1,k_col:k_col+kernel_col-1)*delta_state_c2(k_row,k_col);
end
end
kernel_c2_temp(:,:,m)=kernel_c2_temp(:,:,m)-yita*count;
end
end
%% 更新 weight_c2
for n=1:layer_f2_num
for m=1:layer_c2_num
count_delta_state_s2_temp1=zeros(s2_row,s2_col);
for kk=1:layer_f1_num
delta_state_s2_temp1(:,:,m)=2*Error(1,n)*weight_full_1(m,kk)*weight_full_2(kk,n)*exp(-state_s2_temp2(1,m))/(1+exp(-state_s2_temp2(1,m))).^2*weight_arr2num(:,:,m);
count_delta_state_s2_temp1=count_delta_state_s2_temp1+delta_state_s2_temp1(:,:,m);
end
delta_state_c2=kron(count_delta_state_s2_temp1,ones(2,2)/4);
delta_state_c2_temp1(:,:,m)=conv2(delta_state_c2,kernel_c2(:,:,m),'full');
for k=1:layer_c1_num
delta_weight_c2_temp(k,m)=sum(sum(delta_state_c2_temp1(:,:,m).*state_s1(:,:,k)));
end
end
weight_c2_temp=weight_c2_temp-yita*delta_weight_c2_temp;
end
%% 更新 kernel_c1
for n=1:layer_f2_num
for m=1:layer_c2_num
count_delta_state_s2_temp1=zeros(s2_row,s2_col);
for kk=1:layer_f1_num
delta_state_s2_temp1(:,:,m)=2*Error(1,n)*weight_full_1(m,kk)*weight_full_2(kk,n)*exp(-state_s2_temp2(1,m))/(1+exp(-state_s2_temp2(1,m))).^2*weight_arr2num(:,:,m);
count_delta_state_s2_temp1=count_delta_state_s2_temp1+delta_state_s2_temp1(:,:,m);
end
delta_state_c2=kron(count_delta_state_s2_temp1,ones(2,2)/4);
delta_state_c2_temp1(:,:,m)=conv2(delta_state_c2,kernel_c2(:,:,m),'full');
end
for k=1:layer_c1_num
[x,y,~]=size(state_c2_temp1);
count1=zeros(x,y);
for m=1:layer_c2_num
count_temp=weight_c2(k,m)*delta_state_c2_temp1(:,:,m);
count1=count1+count_temp;
end
delta_state_s1(:,:,k)=count1;
delta_state_c1(:,:,k)=kron(delta_state_s1(:,:,k),ones(2,2)/4);
%
count2=zeros(kernel_row,kernel_col);
for k_row=1:c1_row
for k_col=1:c1_col
count=count+train_data(k_row:k_row+kernel_row-1,k_col:k_col+kernel_col-1)*delta_state_c1(k_row,k_col,k);
end
end
kernel_c1_temp(:,:,k)=kernel_c1_temp(:,:,k)-yita*count;
end
end
%% 权值更新
weight_c2=weight_c2_temp;
kernel_c1=kernel_c1_temp;
kernel_c2=kernel_c2_temp;
weight_arr2num=weight_arr2num_temp;
weight_full_2=weight_full_2_temp;
weight_full_1=weight_full_1_temp;
end
没有合适的资源?快使用搜索试试~ 我知道了~
基于CNN卷积神经网络Matlab实现源码
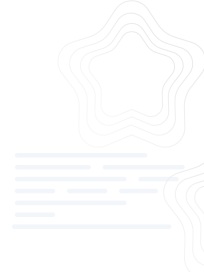
共2000个文件
bmp:1992个
m:8个

需积分: 5 9 下载量 143 浏览量
2024-05-09
01:00:48
上传
评论 3
收藏 17.23MB ZIP 举报
温馨提示
基于CNN(卷积神经网络)的Matlab实现通常涉及构建、训练和使用卷积神经网络模型来处理图像数据或其他适合使用CNN的任务。以下是一份关于使用Matlab实现CNN的资源描述: 1. **Matlab环境**:Matlab是一个强大的数学计算软件,广泛用于算法开发、数据可视化、数据分析以及深度学习等领域。Matlab提供了对深度学习网络的直接支持,允许用户方便地构建和训练CNN模型。 2. **卷积神经网络(CNN)**:CNN是一种深度学习模型,特别适用于处理具有网格状拓扑结构的数据,如图像。CNN通过使用卷积层来提取图像特征,然后通常使用池化层(Pooling Layer)、全连接层(Fully Connected Layer)和激活函数来构建一个深层网络。 3. **Matlab实现步骤**: - **初始化**:在Matlab中设置CNN的层数、每层的神经元数量、激活函数等。 - **构建网络**:使用Matlab的`layerGraph`、`seriesNetwork`或`dagNetwork`等函数定义网络结构。 - **训练数据准备**:加载或预
资源推荐
资源详情
资源评论
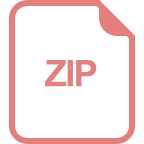
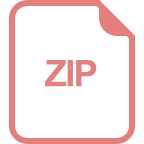
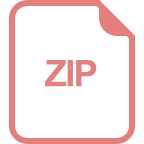
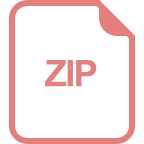
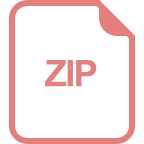
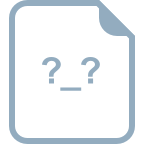
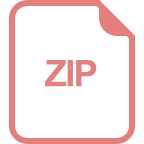
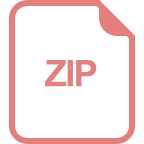
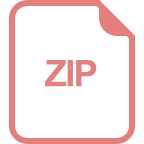
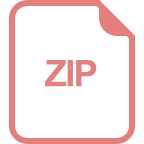
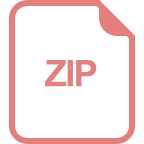
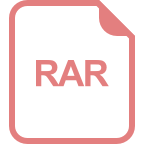
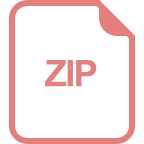
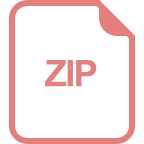
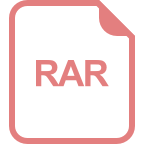
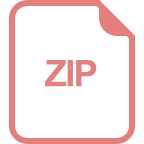
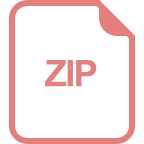
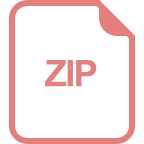
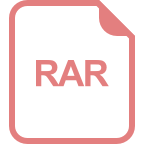
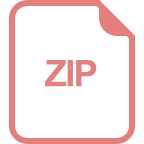
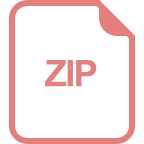
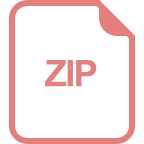
收起资源包目录

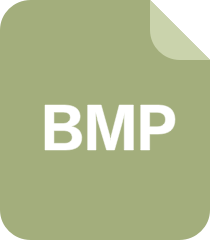
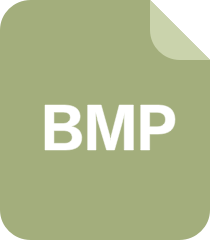
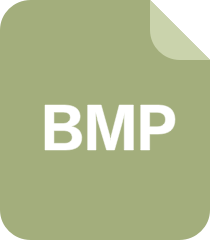
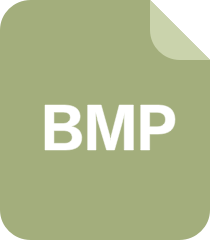
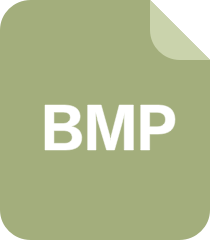
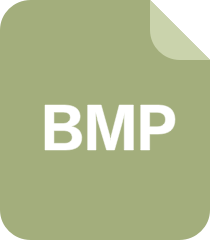
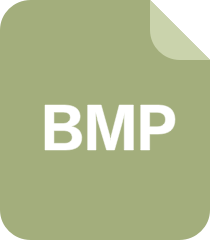
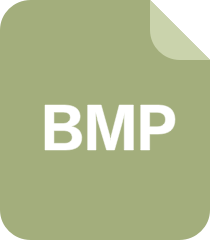
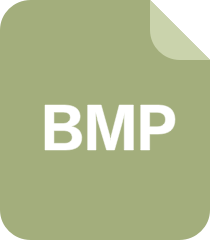
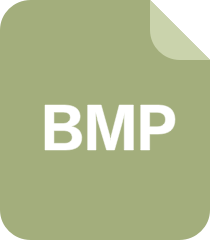
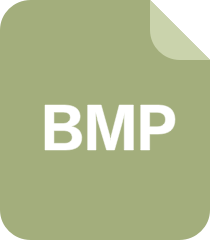
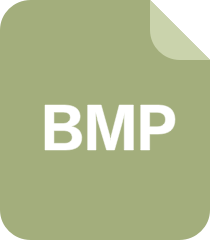
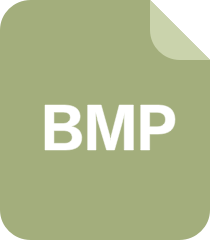
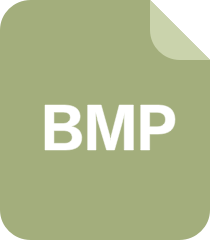
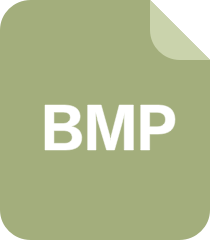
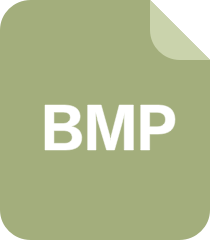
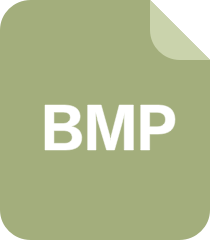
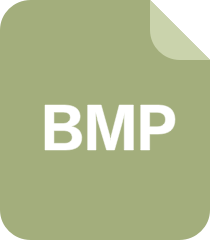
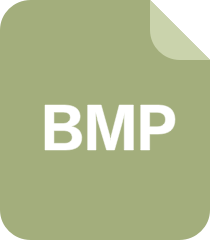
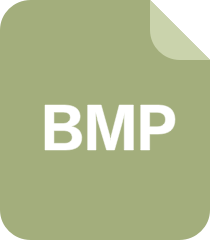
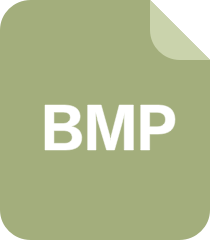
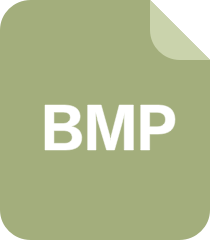
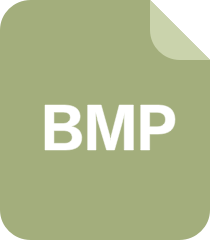
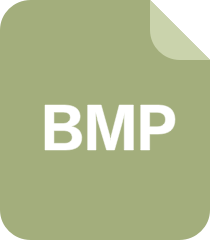
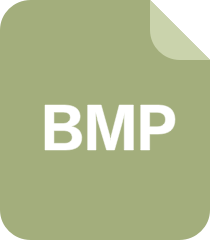
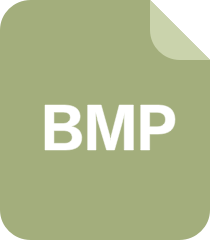
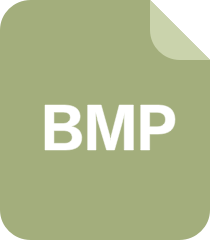
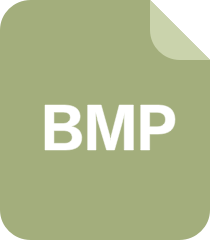
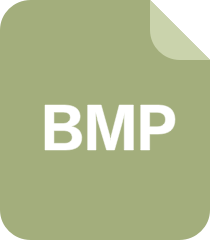
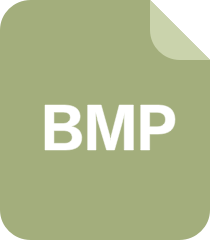
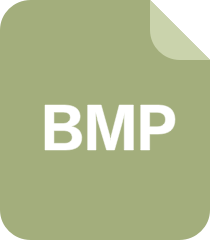
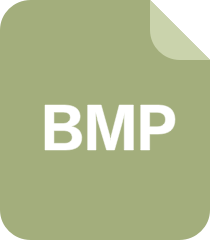
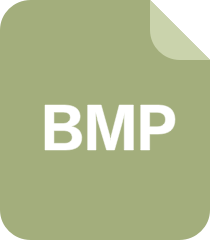
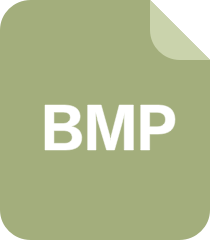
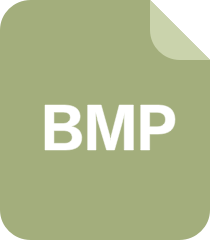
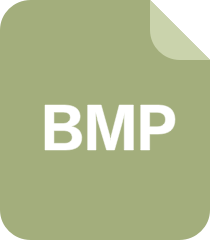
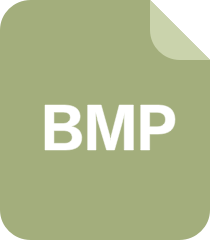
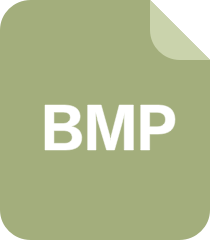
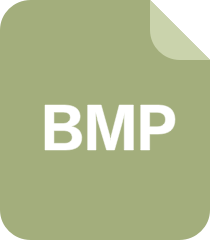
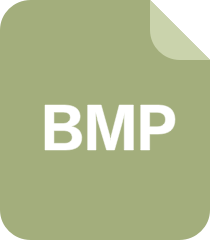
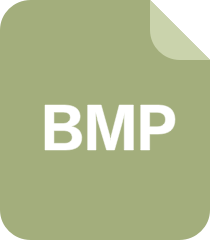
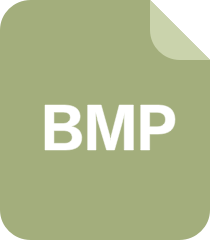
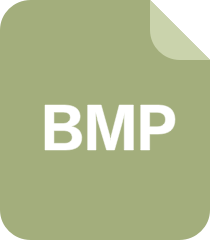
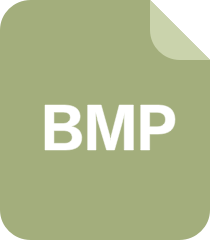
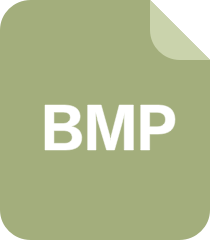
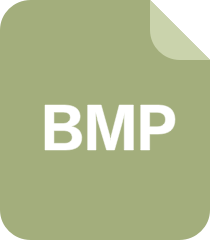
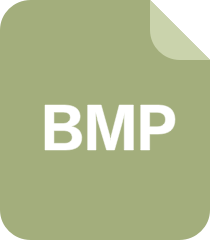
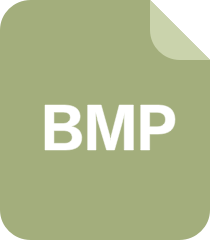
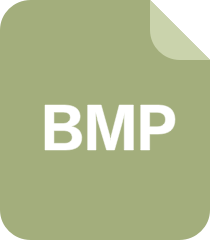
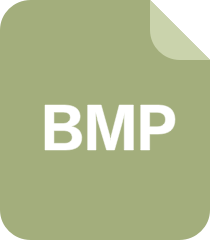
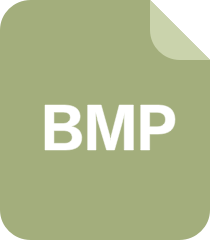
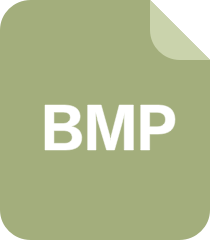
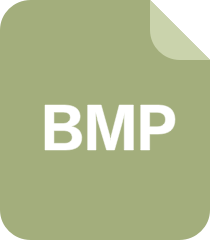
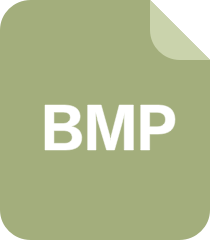
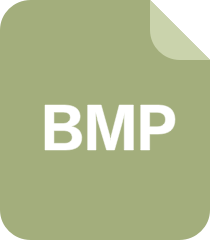
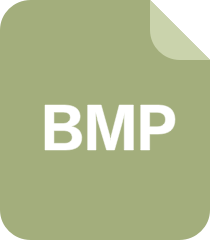
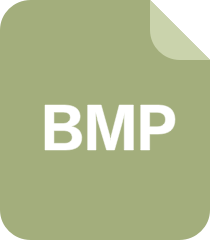
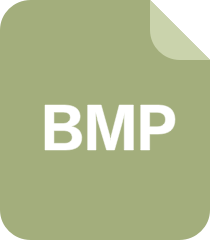
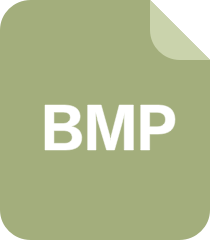
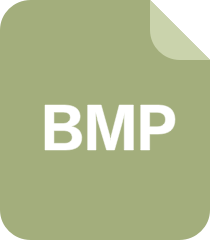
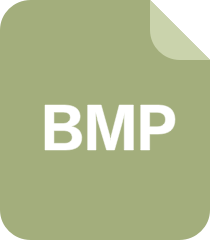
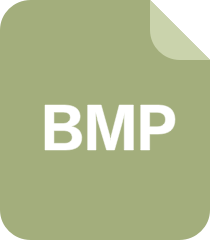
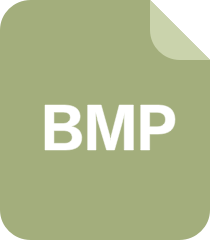
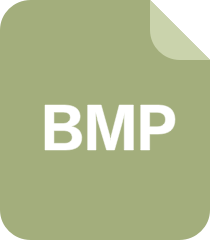
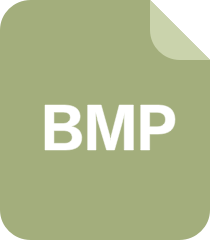
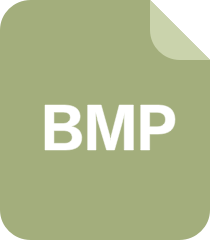
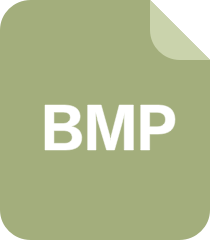
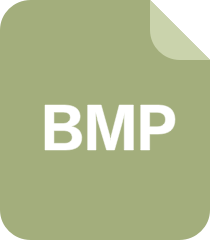
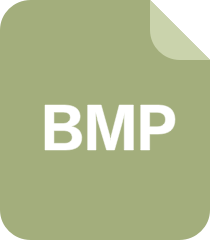
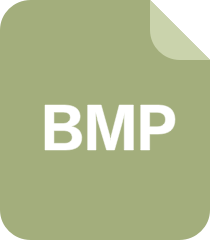
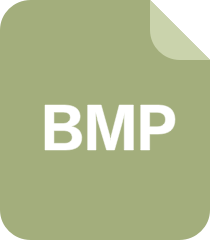
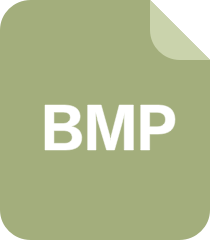
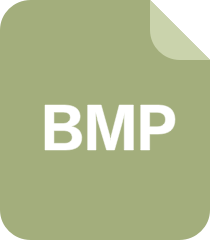
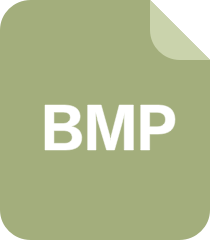
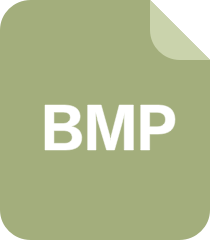
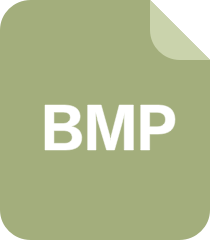
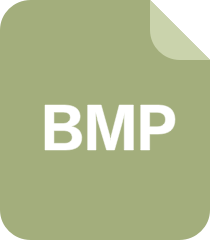
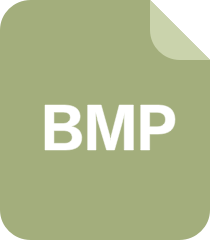
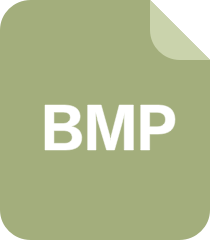
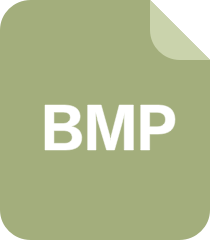
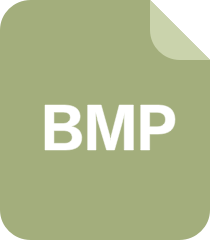
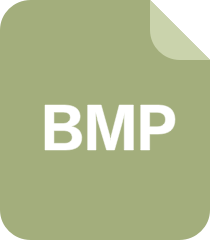
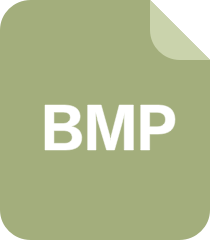
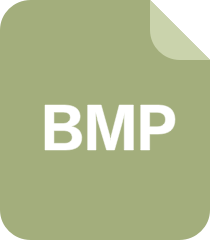
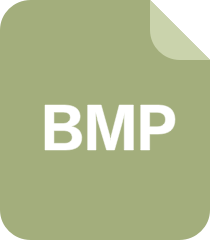
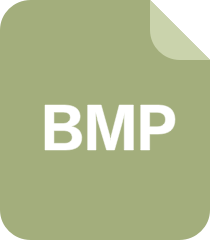
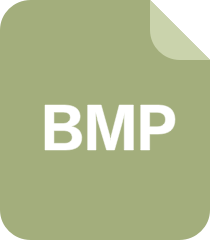
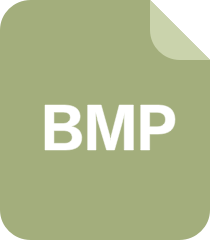
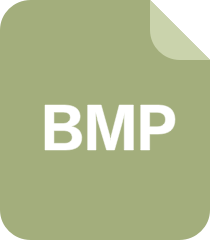
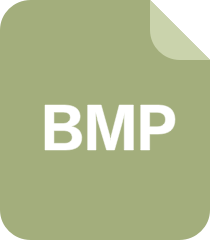
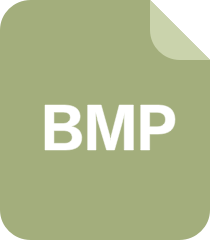
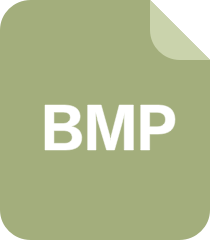
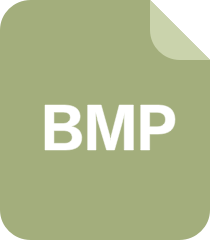
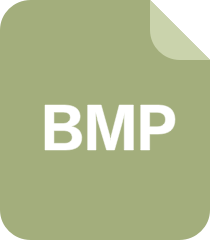
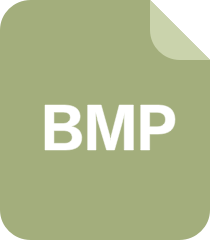
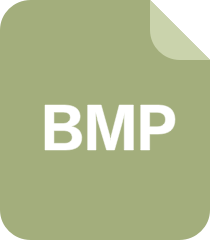
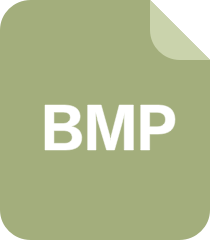
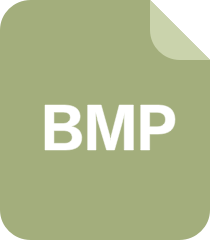
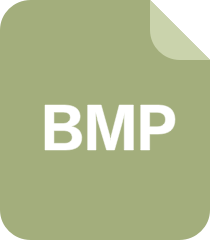
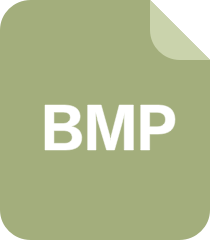
共 2000 条
- 1
- 2
- 3
- 4
- 5
- 6
- 20
资源评论
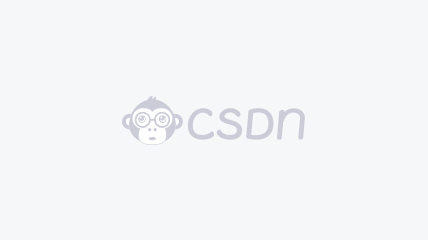

依邻依伴
- 粉丝: 3072
- 资源: 249
上传资源 快速赚钱
我的内容管理 展开
我的资源 快来上传第一个资源
我的收益
登录查看自己的收益我的积分 登录查看自己的积分
我的C币 登录后查看C币余额
我的收藏
我的下载
下载帮助

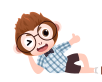
最新资源
资源上传下载、课程学习等过程中有任何疑问或建议,欢迎提出宝贵意见哦~我们会及时处理!
点击此处反馈


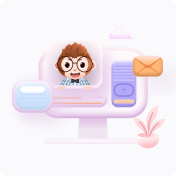
安全验证
文档复制为VIP权益,开通VIP直接复制
