# Eigen Tensors
Tensors are multidimensional arrays of elements. Elements are typically scalars,
but more complex types such as strings are also supported.
[TOC]
## Tensor Classes
You can manipulate a tensor with one of the following classes. They all are in
the namespace ```::Eigen.```
### Class Tensor<data_type, rank>
This is the class to use to create a tensor and allocate memory for it. The
class is templatized with the tensor datatype, such as float or int, and the
tensor rank. The rank is the number of dimensions, for example rank 2 is a
matrix.
Tensors of this class are resizable. For example, if you assign a tensor of a
different size to a Tensor, that tensor is resized to match its new value.
#### Constructor Tensor<data_type, rank>(size0, size1, ...)
Constructor for a Tensor. The constructor must be passed ```rank``` integers
indicating the sizes of the instance along each of the the ```rank```
dimensions.
// Create a tensor of rank 3 of sizes 2, 3, 4. This tensor owns
// memory to hold 24 floating point values (24 = 2 x 3 x 4).
Tensor<float, 3> t_3d(2, 3, 4);
// Resize t_3d by assigning a tensor of different sizes, but same rank.
t_3d = Tensor<float, 3>(3, 4, 3);
#### Constructor Tensor<data_type, rank>(size_array)
Constructor where the sizes for the constructor are specified as an array of
values instead of an explicitly list of parameters. The array type to use is
```Eigen::array<Eigen::Index>```. The array can be constructed automatically
from an initializer list.
// Create a tensor of strings of rank 2 with sizes 5, 7.
Tensor<string, 2> t_2d({5, 7});
### Class TensorFixedSize<data_type, Sizes<size0, size1, ...>>
Class to use for tensors of fixed size, where the size is known at compile
time. Fixed sized tensors can provide very fast computations because all their
dimensions are known by the compiler. FixedSize tensors are not resizable.
If the total number of elements in a fixed size tensor is small enough the
tensor data is held onto the stack and does not cause heap allocation and free.
// Create a 4 x 3 tensor of floats.
TensorFixedSize<float, Sizes<4, 3>> t_4x3;
### Class TensorMap<Tensor<data_type, rank>>
This is the class to use to create a tensor on top of memory allocated and
owned by another part of your code. It allows to view any piece of allocated
memory as a Tensor. Instances of this class do not own the memory where the
data are stored.
A TensorMap is not resizable because it does not own the memory where its data
are stored.
#### Constructor TensorMap<Tensor<data_type, rank>>(data, size0, size1, ...)
Constructor for a Tensor. The constructor must be passed a pointer to the
storage for the data, and "rank" size attributes. The storage has to be
large enough to hold all the data.
// Map a tensor of ints on top of stack-allocated storage.
int storage[128]; // 2 x 4 x 2 x 8 = 128
TensorMap<Tensor<int, 4>> t_4d(storage, 2, 4, 2, 8);
// The same storage can be viewed as a different tensor.
// You can also pass the sizes as an array.
TensorMap<Tensor<int, 2>> t_2d(storage, 16, 8);
// You can also map fixed-size tensors. Here we get a 1d view of
// the 2d fixed-size tensor.
Tensor<float, Sizes<4, 5>> t_4x3;
TensorMap<Tensor<float, 1>> t_12(t_4x3, 12);
#### Class TensorRef
See Assigning to a TensorRef below.
## Accessing Tensor Elements
#### <data_type> tensor(index0, index1...)
Return the element at position ```(index0, index1...)``` in tensor
```tensor```. You must pass as many parameters as the rank of ```tensor```.
The expression can be used as an l-value to set the value of the element at the
specified position. The value returned is of the datatype of the tensor.
// Set the value of the element at position (0, 1, 0);
Tensor<float, 3> t_3d(2, 3, 4);
t_3d(0, 1, 0) = 12.0f;
// Initialize all elements to random values.
for (int i = 0; i < 2; ++i) {
for (int j = 0; j < 3; ++j) {
for (int k = 0; k < 4; ++k) {
t_3d(i, j, k) = ...some random value...;
}
}
}
// Print elements of a tensor.
for (int i = 0; i < 2; ++i) {
LOG(INFO) << t_3d(i, 0, 0);
}
## TensorLayout
The tensor library supports 2 layouts: ```ColMajor``` (the default) and
```RowMajor```. Only the default column major layout is currently fully
supported, and it is therefore not recommended to attempt to use the row major
layout at the moment.
The layout of a tensor is optionally specified as part of its type. If not
specified explicitly column major is assumed.
Tensor<float, 3, ColMajor> col_major; // equivalent to Tensor<float, 3>
TensorMap<Tensor<float, 3, RowMajor> > row_major(data, ...);
All the arguments to an expression must use the same layout. Attempting to mix
different layouts will result in a compilation error.
It is possible to change the layout of a tensor or an expression using the
```swap_layout()``` method. Note that this will also reverse the order of the
dimensions.
Tensor<float, 2, ColMajor> col_major(2, 4);
Tensor<float, 2, RowMajor> row_major(2, 4);
Tensor<float, 2> col_major_result = col_major; // ok, layouts match
Tensor<float, 2> col_major_result = row_major; // will not compile
// Simple layout swap
col_major_result = row_major.swap_layout();
eigen_assert(col_major_result.dimension(0) == 4);
eigen_assert(col_major_result.dimension(1) == 2);
// Swap the layout and preserve the order of the dimensions
array<int, 2> shuffle(1, 0);
col_major_result = row_major.swap_layout().shuffle(shuffle);
eigen_assert(col_major_result.dimension(0) == 2);
eigen_assert(col_major_result.dimension(1) == 4);
## Tensor Operations
The Eigen Tensor library provides a vast library of operations on Tensors:
numerical operations such as addition and multiplication, geometry operations
such as slicing and shuffling, etc. These operations are available as methods
of the Tensor classes, and in some cases as operator overloads. For example
the following code computes the elementwise addition of two tensors:
Tensor<float, 3> t1(2, 3, 4);
...set some values in t1...
Tensor<float, 3> t2(2, 3, 4);
...set some values in t2...
// Set t3 to the element wise sum of t1 and t2
Tensor<float, 3> t3 = t1 + t2;
While the code above looks easy enough, it is important to understand that the
expression ```t1 + t2``` is not actually adding the values of the tensors. The
expression instead constructs a "tensor operator" object of the class
TensorCwiseBinaryOp<scalar_sum>, which has references to the tensors
```t1``` and ```t2```. This is a small C++ object that knows how to add
```t1``` and ```t2```. It is only when the value of the expression is assigned
to the tensor ```t3``` that the addition is actually performed. Technically,
this happens through the overloading of ```operator=()``` in the Tensor class.
This mechanism for computing tensor expressions allows for lazy evaluation and
optimizations which are what make the tensor library very fast.
Of course, the tensor operators do nest, and the expression ```t1 + t2 *
0.3f``` is actually represented with the (approximate) tree of operators:
TensorCwiseBinaryOp<scalar_sum>(t1, TensorCwiseUnaryOp<scalar_mul>(t2, 0.3f))
### Tensor Operations and C++ "auto"
Because Tensor operations create tensor operators, the C++ ```auto``` keyword
does not have its intuitive meaning. Consider these 2 lines of code:
Tensor<float, 3> t3 = t1 + t2;
auto t4 = t1 + t2;
In the first line we allocate the tensor ```t3``` and it will contain the
result of the addition of ```t1``` and ```t2```. In the second line, ```t4```
is actually th
没有合适的资源?快使用搜索试试~ 我知道了~
资源推荐
资源详情
资源评论
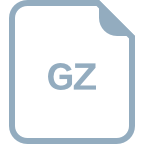
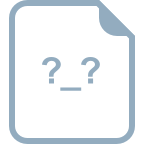
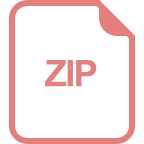
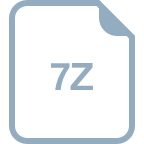
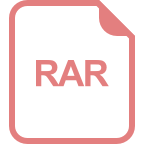
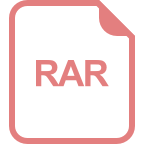
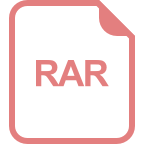
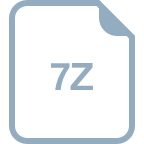
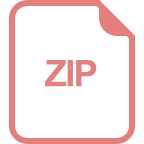
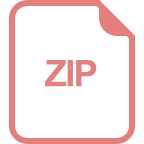
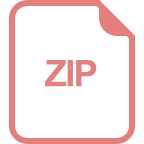
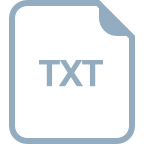
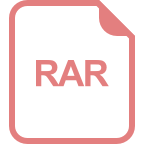
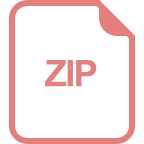
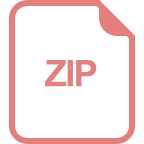
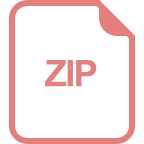
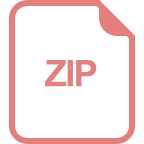
收起资源包目录

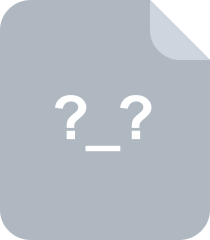
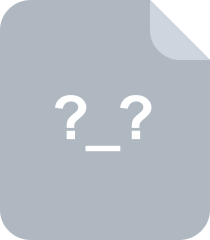
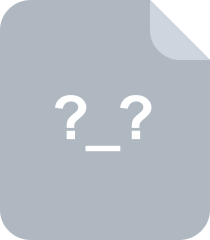
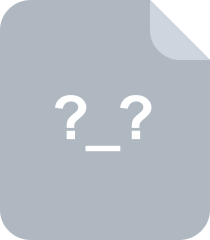
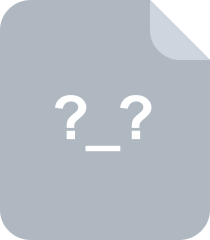
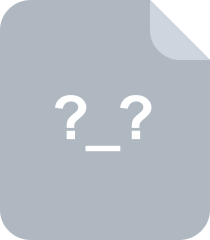
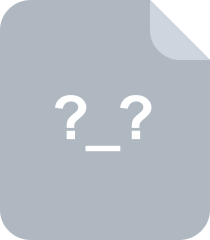
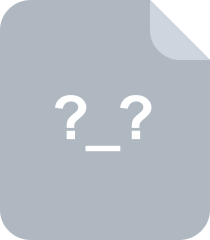
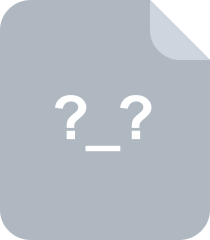
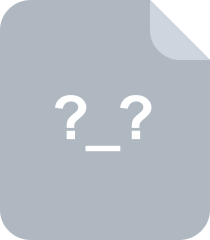
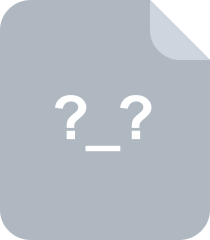
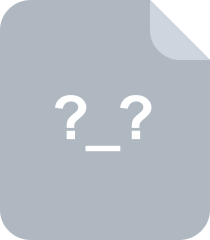
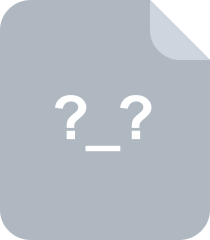
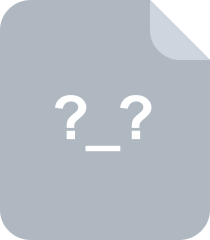
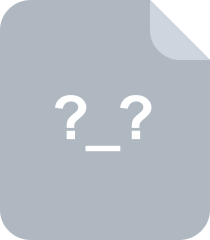
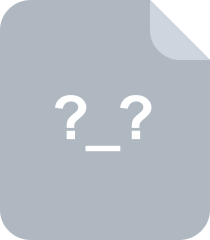
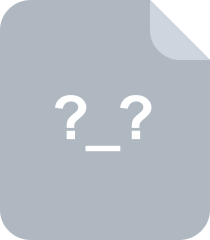
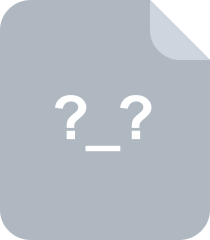
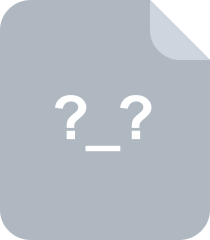
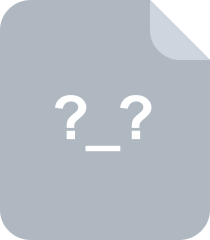
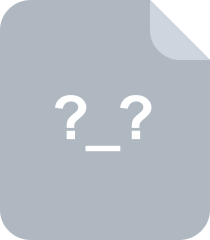
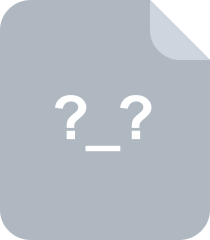
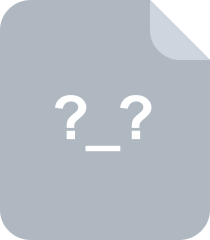
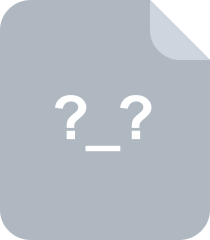
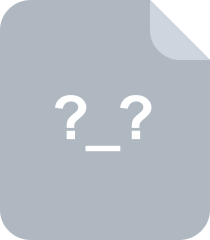
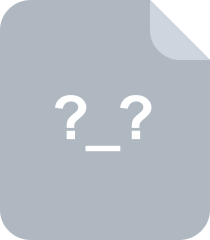
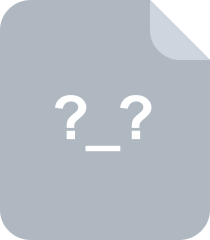
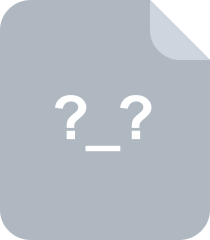
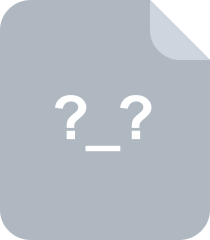
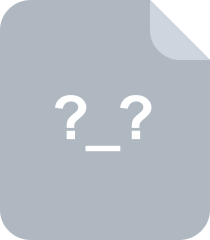
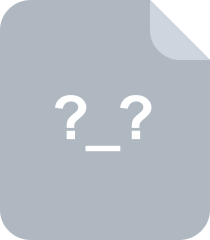
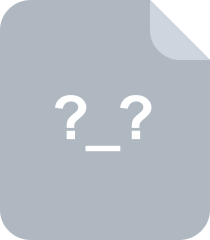
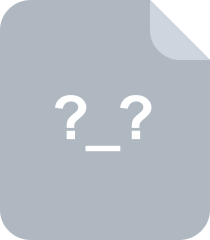
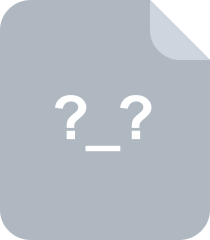
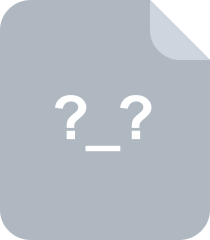
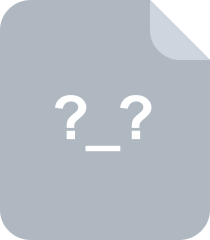
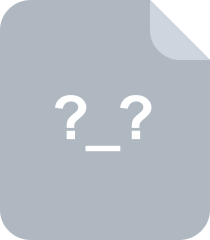
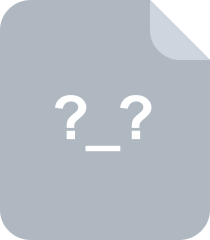
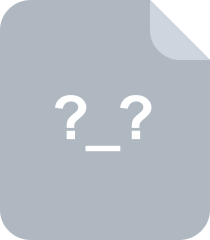
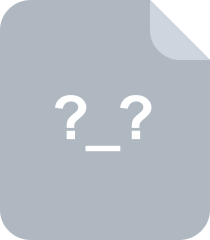
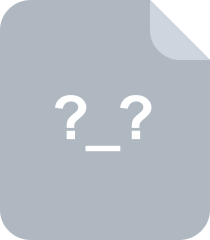
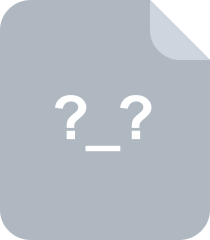
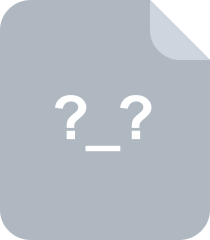
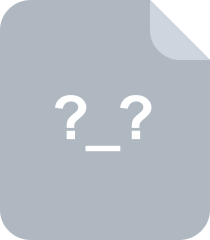
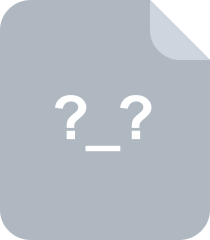
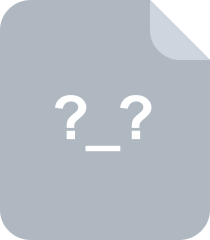
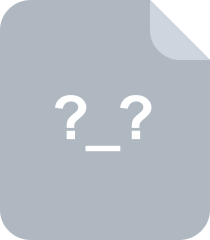
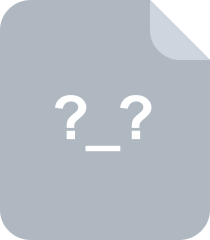
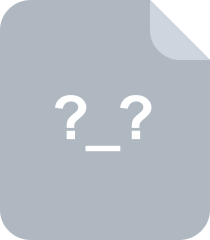
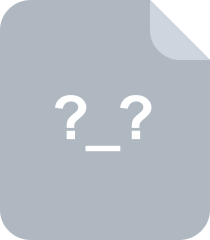
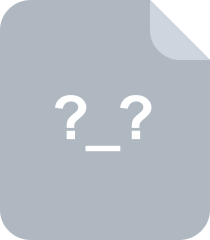
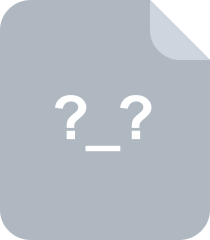
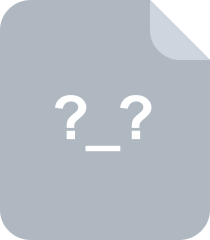
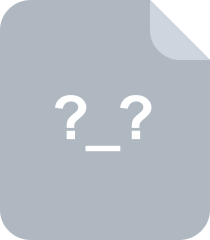
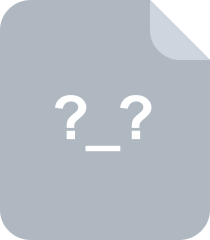
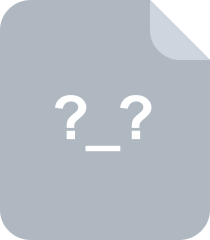
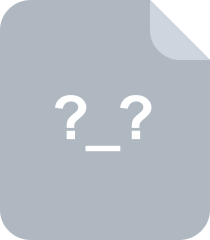
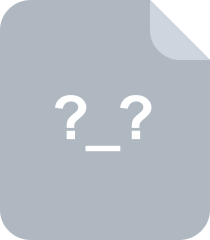
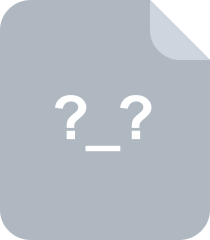
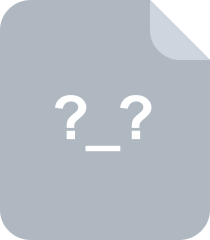
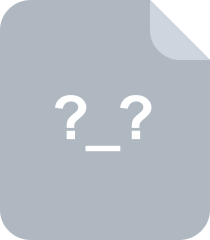
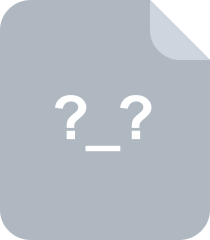
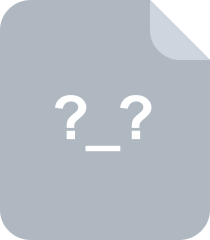
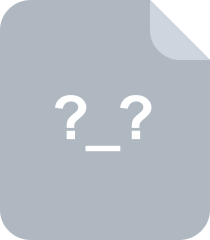
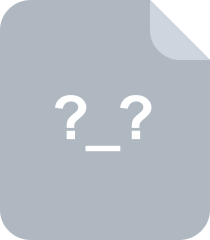
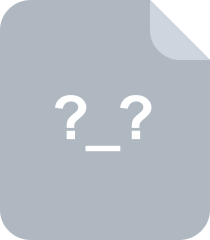
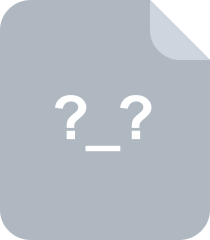
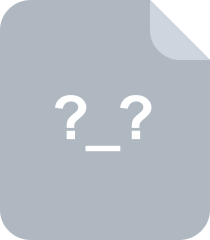
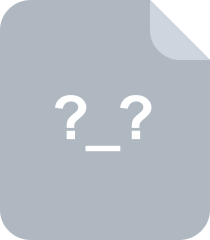
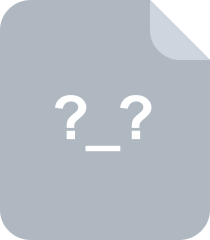
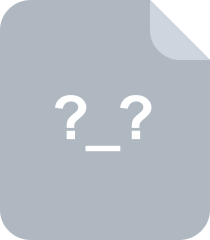
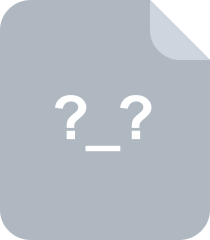
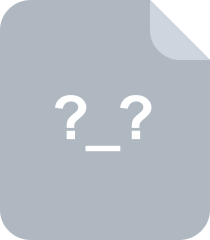
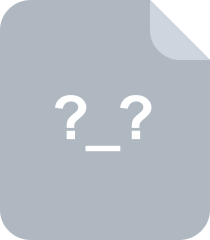
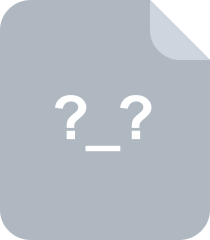
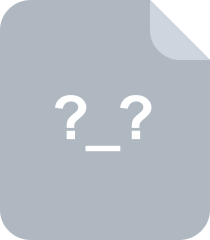
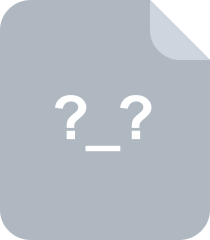
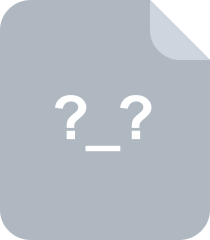
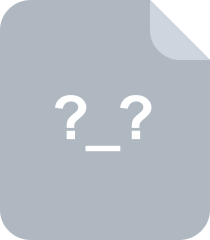
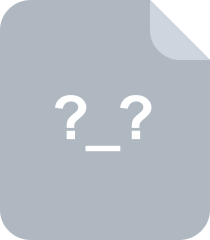
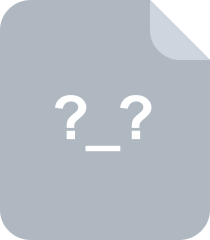
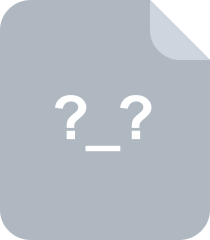
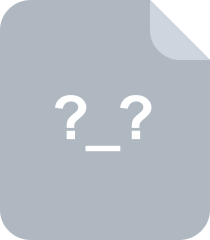
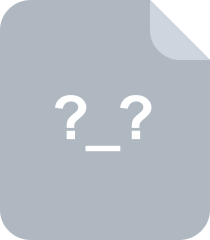
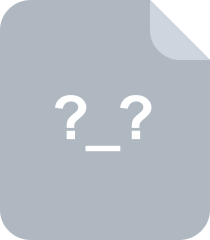
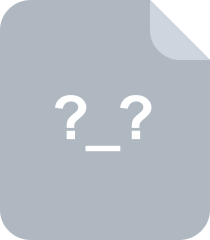
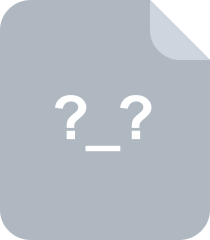
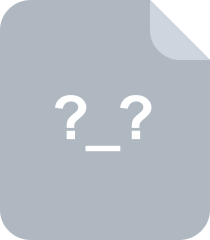
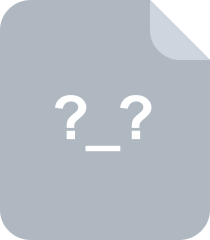
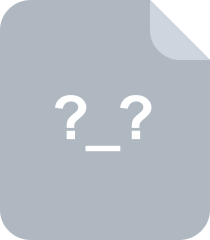
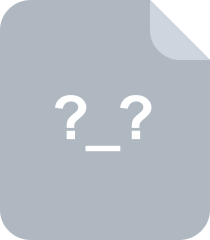
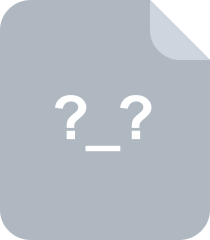
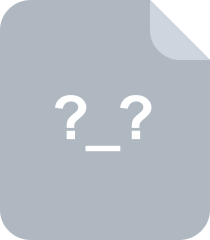
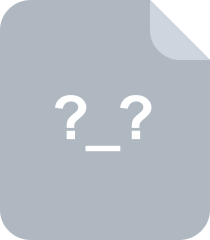
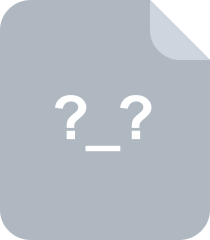
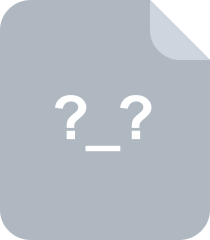
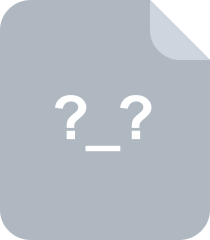
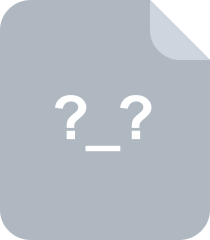
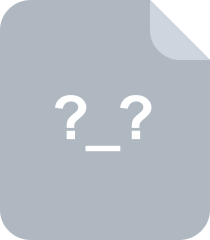
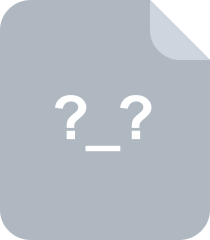
共 7210 条
- 1
- 2
- 3
- 4
- 5
- 6
- 73
资源评论
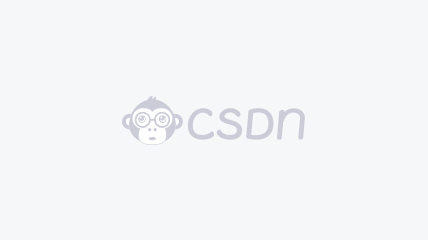

qauzy
- 粉丝: 150
- 资源: 15
上传资源 快速赚钱
我的内容管理 展开
我的资源 快来上传第一个资源
我的收益
登录查看自己的收益我的积分 登录查看自己的积分
我的C币 登录后查看C币余额
我的收藏
我的下载
下载帮助

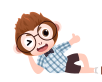
最新资源
- 基于Java开发的日程管理FlexTime应用设计源码
- SM2258XT-BGA144-4BGA180-6L-R1019 三星KLUCG4J1CB B0B1颗粒开盘工具 , EC, 3A, 94, 43, A4, CA 七彩虹SL300这个固件有用
- GJB 5236-2004 军用软件质量度量
- 30天开发操作系统 第 8 天 - 鼠标控制与切换32模式
- spice vd interface接口
- 安装Git时遇到找不到`/dev/null`的问题
- 标量(scalar)、向量(vector)、矩阵(matrix)、数组(array)等概念的深入理解与运用
- 数值计算复习内容,涵盖多种方法,内容为gpt生成
- 标量(scalar)、向量(vector)、矩阵(matrix)、数组(array)等概念的深入理解与运用
- 网络综合项目实验12.19
资源上传下载、课程学习等过程中有任何疑问或建议,欢迎提出宝贵意见哦~我们会及时处理!
点击此处反馈


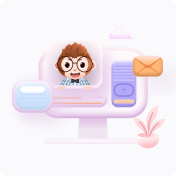
安全验证
文档复制为VIP权益,开通VIP直接复制
