Interference Cancellation in Multi-User MIMO Networks
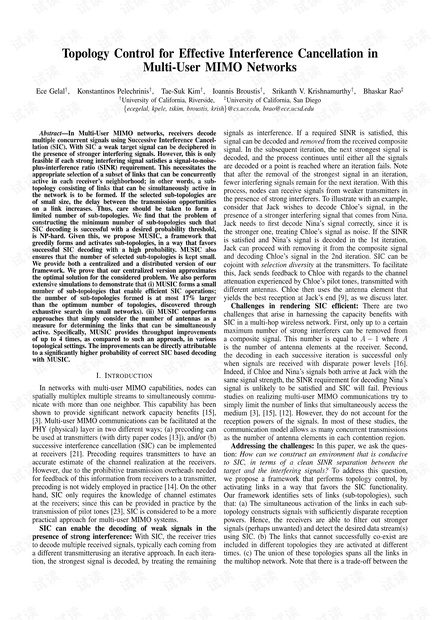

阐述很详细,适合研究生。 Abstract—In Multi-User MIMO networks, receivers decode multiple concurrent signals using Successive Interference Cancellation (SIC). With SIC a weak target signal can be deciphered in the presence of stronger interfering signals. However, this is only feasible if each strong interfering signal satisfies a signal-to-noiseplus- interference ratio (SINR) requirement. This necessitates the appropriate selection of a subset of links that can be concurrently active in each receiver’s neighborhood; in other words, a subtopology consisting of links that can be simultaneously active in the network is to be formed. If the selected sub-topologies are of small size, the delay between the transmission opportunities on a link increases. Thus, care should be taken to form a limited number of sub-topologies. We find that the problem of constructing the minimum number of sub-topologies such that SIC decoding is successful with a desired probability threshold, is NP-hard. Given this, we propose MUSIC, a framework that greedily forms and activates sub-topologies, in a way that favors successful SIC decoding with a high probability. MUSIC also ensures that the number of selected sub-topologies is kept small. We provide both a centralized and a distributed version of our framework. We prove that our centralized version approximates the optimal solution for the considered problem. We also perform extensive simulations to demonstrate that (i) MUSIC forms a small number of sub-topologies that enable efficient SIC operations; the number of sub-topologies formed is at most 17% larger than the optimum number of topologies, discovered through exhaustive search (in small networks). (ii) MUSIC outperforms approaches that simply consider the number of antennas as a measure for determining the links that can be simultaneously active. Specifically, MUSIC provides throughput improvements of up to 4 times, as compared to such an approach, in various topological settings. The improvements can be directly attributable to a significantly higher probability of correct SIC based decoding with MUSIC.
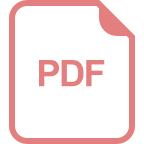
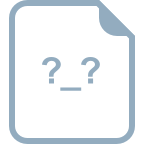
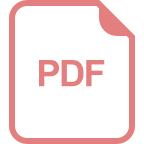
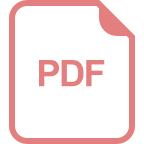
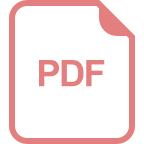
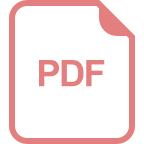
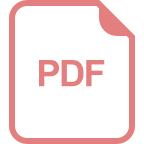
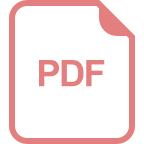
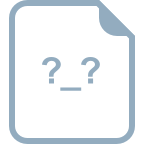
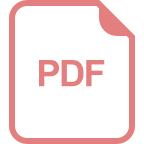
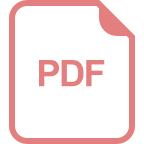
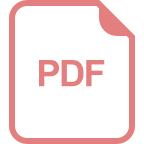
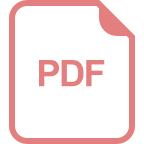
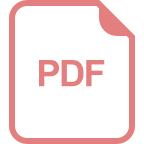
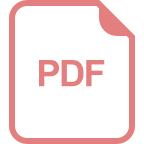
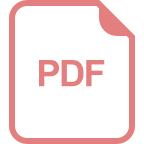
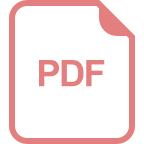
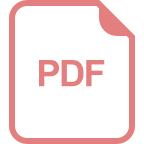
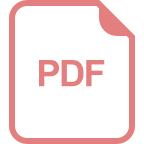
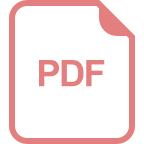
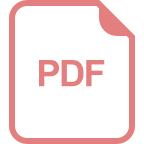
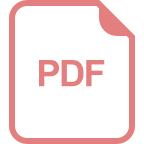
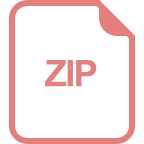
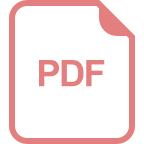
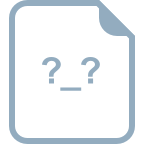
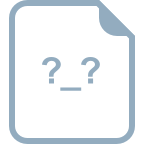
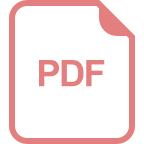
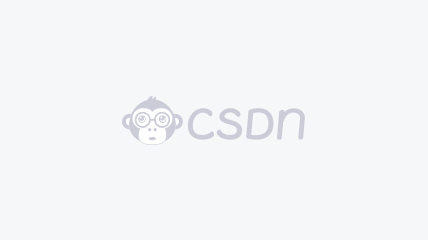

- 粉丝: 2
- 资源: 16
我的内容管理 展开
我的资源 快来上传第一个资源
我的收益
登录查看自己的收益我的积分 登录查看自己的积分
我的C币 登录后查看C币余额
我的收藏
我的下载
下载帮助

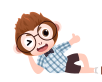
